Abstract
Natural gas production in the Groningen field in the Netherlands is causing induced earthquakes that have raised concerns regarding the safety of the local population given that the exposed building stock (which is predominantly unreinforced masonry residential housing) has not been designed and constructed considering seismic loading. Significant effort has been invested to date in assessing the safety risk of these buildings within a probabilistic framework. This paper describes the efforts that have since been made to extend this framework for probabilistic damage assessment of the buildings, for slight non-structural, slight structural and moderate structural damage. Fragility functions for non-structural damage have been developed considering the observed damage from damage reports, rather than from damage claims due to a number of issues with the latter, as described herein. Structural damage has been estimated using analytical models that have been calibrated through extensive in situ data collection and experimental testing. The probabilistic damage assessment is presented in terms of F-N curves, which plot the annual frequency of exceedance against number of buildings reaching each damage state.
Similar content being viewed by others
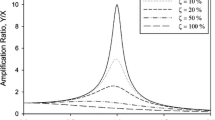
Avoid common mistakes on your manuscript.
1 Introduction
Gas production in the Groningen field in the northern Netherlands is inducing earthquakes, the largest of which to date was the magnitude ML 3.59 (M 3.53: Dost et al. 2018) Huizinge event of August 2012. In response to this induced seismicity, NAM (Nederlandse Aardolie Maatschappij B.V.) has been developing a comprehensive seismic hazard and risk model for the region, which comprises the entire gas field plus a 5 km buffer zone onshore (see Fig. 1a).
The initial focus of this hazard and risk assessment was on safety risk, and the estimation of local personal risk (LPR), defined as the annual probability of fatality for a hypothetical person who is continuously present without protection inside or near a building. The details of this probabilistic risk model have been described in van Elk and Doornhof (2017), whereas the methodology for developing collapse fragility and consequence models for the estimation of LPR in the Groningen field are provided in Crowley et al. (2017). In 2016 the Dutch Ministry of Economic Affairs (MEA) also requested the forecast of group risk for damage (so-called Maatschappelijk Risico [Schade]). To meet this request, F-N curves that present the annual frequency of exceedance against number of damaged buildings have been calculated for both non-structural and structural damage states. This paper thus describes the development of damage fragility functions (which provide the probability of exceeding a given damage state, conditional on a level of input ground motion) for each structural system that has been identified within the region, for the calculation of the aforementioned F-N damage curves.
There are approximately 250,000 residential, commercial and industrial buildings in the Groningen field, and the location and characteristics (structural and architectural) of these buildings have been stored in an exposure database (Arup 2018). These buildings have been classified into 54 different structural systems using the GEM Building Taxonomy (Brzev et al. 2013). Only the occupied buildings (of which there are around 150,000) have been considered in the damage and risk assessment, the majority which, as shown in Fig. 1b, have been constructed in unreinforced masonry.
The next section of this paper describes the damage data that has been collected after induced earthquakes in the northern Netherlands in recent years, and the difficulty in using damage claims data for the forecast of future damage. The methodology to develop fragility functions for non-structural damage, based on damage reports, and for structural damage, based on calibrated numerical models, is presented in Sect. 3. Finally, the methodology used to calculate F-N damage curves and the results for the region shown in Fig. 1a are presented.
2 Observed damage and damage claims
2.1 Preliminary fragility functions based on observed damage
A study of the building damage from induced earthquakes in the Netherlands first started in 2006 as part of a study commissioned by five oil and gas companies [NAM BV, BP Nederland Energy BV (later TAQA), Vermilion Oil & Gas Netherlands BV and Wintershall Noordzee BV] (TNO 2009). Damage data reports from induced earthquakes in the Groningen and Roswinkel gas fields (with ML between 3.0 and 3.5) were retrieved and the buildings were grouped into three main categories: farmhouses, low-rise unreinforced masonry housing constructed before 1940 and low-rise unreinforced masonry housing constructed after 1940 (see Fig. 2 for examples of each of these categories).
For each earthquake, a number of rings at different distances from the epicentre were defined, and the median peak ground velocity (PGV) within each ring was associated with the percentage of damaged buildings inside the ring, for each structural category. Each ring was defined by ensuring the same number of buildings per category would be found in each ring. The PGV was calculated using a ground-motion prediction equation (GMPE) developed by the Royal Netherlands Meteorological Institute (KNMI) using measured accelerometer and borehole seismometry data from the northern Netherlands (Dost et al. 2004). The use of PGV as the intensity measure was felt to be justified, given that empirical evidence (e.g. Bommer and Alarcón 2006) has shown that low levels of building damage correlate strongly with peak ground velocity (PGV) and most guidelines for tolerable shaking levels—implying disturbance to occupants and/or low damage levels—are specified in terms of PGV. The final percentage of damaged buildings (taken to represent the probability of damage) for the three building typologies was plotted against the median PGV.
The EMS damage scale (Grunthal et al. 1998) describes 5 damage states for unreinforced masonry (URM) buildings, where the first damage grade (DS1 herein) refers to no structural damage and slight non-structural damage which is manifested through hairline cracks in walls and damage to plaster (see Fig. 3). The majority of the collected damage data is understood to have corresponded to DS1. The most fragile building type was shown to be the farmhouses, which is not surprising given the lower stiffness of the long façades found in these buildings, compared to the relatively short façades of the low-rise dwellings.
EMS damage grade 1 (DS1) for URM buildings (Grunthal et al. 1998)
Several international norms exist to help assess vibration levels and their impact on buildings such as e.g. DIN-4150 in Germany and SBR-2017 in the Netherlands. The latter guidelines define a minimum threshold vibration velocity value of 5 mm/s for typical dwellings (i.e. the low-rise buildings considered herein) and 3 mm/s for other buildings that are more sensitive to vibrations, such as the farmhouses. Experience has shown that if such thresholds are complied with, damage that reduces the serviceability of the building will not occur. The damage data analysed by TNO (2009) showed that the probability of reaching or exceeding DS1 under the aforementioned peak ground velocities was in the order of 1 and 2%, which is consistent with the recommendations of the Dutch regulations. However, it is worth noting that these thresholds of PGV are low compared to e.g. British Standards, which indicate appreciably higher thresholds for ‘cosmetic damage’, starting at a minimum of over 15 mm/s for weak structures (BSI 1993).
2.2 Comparison of empirical fragility functions and damage claims
The damage data described in the previous section was used to estimate the number of damaged buildings for two events in the Groningen field: the Huizinge (August 16, 2012) and Hellum (September 30, 2015) earthquakes. The relationship for farmhouses, the weakest of the three typologies, has been conservatively applied to all buildings and the peak ground velocities that the buildings were exposed to have been estimated with an updated empirical ground motion prediction equation developed specifically for the Groningen field (Bommer et al. 2016). The estimated numbers of damaged buildings have then been compared with the number of damage claims received within 10 weeks after each seismic event.
The Huizinge earthquake had a magnitude of ML = 3.59 and affected a large area of the Groningen province. Figure 4 (left) shows in yellow the area where the buildings would have had at least a 1% probability of damage based on the empirical fragility function for farmhouses. This area corresponds quite well with the area from which damage claims were received. Also, it is noted that the forecast number of damaged buildings (around 2500) was very close to the number of damage claims in the 10 weeks following the earthquake (around 2000).
Map of the probability of DS1 (left) compared with the spatial extent of actual damage claims (right) for the Huizinge earthquake (NAM 2016)
The same methodology has been applied to the Hellum earthquake of 2015 (ML = 3.1), but in this case the comparison was found to be very different. Due to the lower energy released during the Hellum earthquake (around 5.5 times less than Huizinge), the area where the earthquake was predicted to have potentially caused damage was much smaller, as shown by the reduced yellow area in Fig. 5 (left). However, the area from which damage claims were received in the 10 weeks after the event was much larger than that of Huizinge: there were almost 7000 damage claims, but only 200 buildings were predicted to have reached or exceeded DS1 using the empirical fragility function.
Map of the probability of DS1 (left) compared with the spatial extent of actual damage claims (right) for the Hellum earthquake (NAM 2016) (note the location of Groningen city has been signalled in both plots to aid comparison)
Thus, for the Huizinge earthquake, the analysis showed a strong correlation between the predicted building damage and the actual damage claims. That correlation was instead much weaker for the Hellum earthquake, where the number of damage claims was much higher than the predicted building damage. The reason for the difference in damage claims between the Huizinge and Hellum earthquakes is likely to be due to the increased attention given to building damage following the Huizinge earthquake (which has been the largest earthquake recorded to date in the field). This increased attention to earthquake activity could have led homeowners to study more attentively their houses, and they may have identified damage that had already been there, possibly caused by other phenomenon such as differential settlement or lack of maintenance. Similar findings have been observed in the geothermal field in Basel, Switzerland, where the insurance payments from an induced event that occurred in December 2006 did not necessarily reflect extensive damage, but were likely to have been influenced by pre-existing non-structural damage as well as some cases of what the insurance industry refers to as ‘moral hazard’ (Bommer et al. 2015).
Figure 6 shows the cumulative number of damage claims since the Huizinge earthquake, where the increasing rate of received damage claims can clearly be observed. After the Huizinge earthquake (16th August 2012) the rate at which damage claims were received initially rose sharply, but tailed off after some weeks. However, during 2013 and the first three quarters of 2014, an average of 210 damage claims were received each week. In September 2014, this rate doubled to around 560 damage claims each week. The vertical lines in Fig. 6 indicate the occurrence of earthquakes with a magnitude above ML = 2.0, and although the claim rate does seem to increase after some earthquakes, this causes only a small deviation from the general (linear) trend, and a sharp increase for the whole of 2015 is observed without the presence of any earthquakes, which suggests that there may have been other reasons for the increase in damage claims.
Cumulative number of damage claims over time. The red vertical lines indicate earthquakes with local magnitude greater than 2.0 (NAM 2017)
The outcome of this study illustrates the difficulty of using claims data to forecast future damage, and highlights the importance and usefulness of studies such as the one carried out by TNO, whereby damage reports of individual buildings are used. These empirical fragility functions are particularly important as there is limited experience in using experimental data and analytical models to estimate non-structural damage (which is typically cracking in the plaster of the walls). The damage data supplied by TNO (assumed by the authors to correspond to DS1) has thus been re-evaluated and expanded for use in NAM’s probabilistic damage assessment, as described in the next section, and has been combined with experimental and analytical modelling for structural damage (DS2 and DS3), given that the latter damage states have not been sufficiently observed in the field.
3 Development of fragility functions for DS1, DS2 and DS3
3.1 Non-structural damage (DS1)
The process that has been selected for the development of fragility functions in the Groningen hazard and risk assessment is mainly based on numerical modelling, calibrated using a wide range of data, from in situ material properties to shake table tests of full-scale buildings taken to collapse (Crowley et al. 2017; Crowley and Pinho 2017). Limited attention was however given to the experimental testing of DS1 in unreinforced masonry buildings; the majority of the test specimens were not constructed with plaster finish. Therefore, for DS1 it was instead decided to update the empirical fragility functions described in the previous section through the consideration of damage data from earthquakes in the Groningen field that were not included in the original TNO (2009) study, together with the use of an updated field-specific ground-motion prediction equation for PGV that is valid for the magnitude range ML 1.8–4.0 (Bommer et al. 2017a).
The following Groningen field related earthquakes have thus been considered as input, based on data provided by TNO:
-
Hoeksmeer 2003 ML = 3.0
-
Stedum 2003 ML = 3.0
-
Westeremden 2006 ML = 3.5
-
Huizinge 2012 ML = 3.6
Fragility functions for DS1 have been developed using the aforementioned damage data and the median PGV associated with each group of damaged buildings (based on the ‘rings’ approach described previously). Each fragility curve is defined as a lognormal function with a median value of the hazard demand parameter (in this case PGV) that corresponds to the threshold of the damage state and by the variability associated with that damage state. The conditional probability of exceeding DS1 given the PGV is then given by the following function:
where θ is the median value of PGV at which the threshold of damage state DS1 is reached, β is the standard deviation of the natural logarithm of PGV for DS1 and Φ is the standard normal cumulative distribution function. The DS1 fragility functions have been fitted for each of the typologies defined in the TNO report using maximum likelihood estimation, leading to the functions shown in Fig. 7 and reported in Table 1.
3.2 Slight and moderate structural damage (DS2 and DS3)
The fragility functions for slight and moderate structural damage (DS2 and DS3) have been developed using analytical models, the majority of which have been calibrated using the results of a large experimental testing campaign. The following sections summarise the process that has been followed to produce these analytical fragility functions and the reader is referred to Crowley et al. (2017) and Crowley and Pinho (2017) for the full details of the methodology.
3.2.1 Transformation of MDOF models to SDOF models
Real representative buildings from the region have been identified for each building class (so-called index buildings) and the structural drawings of these buildings have been used to develop multi-degree-of-freedom (MDOF) numerical models of their structural systems, together with the predominant non-structural elements (such as partition walls and external façade walls).
Nonlinear dynamic analysis (using a set of 11 triaxial ground motions) of the majority of the index buildings has then been undertaken using LS-DYNA (LSTC—Livermore Software Technology Corporation 2013) and ELS (ASI 2017) for the URM buildings and SeismoStruct (Seismosoft 2017) for reinforced concrete (RC), steel and timber buildings. For some of the stronger buildings (i.e. those constructed in reinforced concrete, steel or timber), nonlinear static analysis has been performed. The results of these analyses are fully presented in Arup (2017) and Mosayk (2015, 2017). The results of a large number of in situ and laboratory tests (Graziotti et al. 2016a, b, 2017a, b, 2018; Tomassetti et al. 2017; Correia et al. 2018; Kallioras et al. 2018; Sharma et al. 2018; Brunesi et al. 2018a, b, c) have been used to validate and/or calibrate the aforementioned software tools as well as the inputs to the structural models (as described in Mosayk 2014; Arup et al. 2015, 2016a, b, 2017; Avanes et al. 2018a, b; Malomo et al. 2018a, b, c; Montalbini et al. 2018).
SDOF backbone capacity curves were then defined for each model using either the static pushover curves directly or the points from the dynamic hysteresis loops representing the peak base shear and corresponding attic floor (i.e. highest level in the building before the roof) displacement in each direction of the building (Fig. 8). It is noted that shear and attic floor displacement response time-histories of MDOF structural systems are not necessarily fully in-phase, particularly when multiple modes of vibration or failure mechanisms are activated during the response of a given structure (a phenomenon that is further accentuated when the structure is pushed highly into the nonlinear inelastic response range). This effectively implies the presence of a time-lag between the moment when the peak value of base shear is observed and the instant at which the corresponding attic floor displacement is recorded; the latter typically arriving with a slight delay with respect to the former. In the definition of the SDOF backbone capacity curves, this time-lag obviously needs to be removed (since it has no physical meaning within a SDOF representation of the response), this being the reason why the black dots in the plots in Fig. 8 (representing the max shear-displacement points with the time-lag removed) do not necessarily always appear on top of the hysteretic curves (where the time-lag is instead present).
Example static pushover analysis (left) and hysteresis loops of the 11 dynamic analyses (right) for two index buildings (Crowley and Pinho 2017)
The points of peak base shear and corresponding attic displacement from each nonlinear dynamic/static analysis have been transformed to equivalent SDOF properties using the transformation methodology presented in Casarotti and Pinho (2007). Figure 9 shows an example backbone SDOF curve obtained by transforming the points of peak base shear and corresponding attic displacement from each nonlinear dynamic/static analysis. These backbone curves, together with a hysteresis model (e.g. Takeda) and springs that represent the stiffness and damping due to foundation flexibility and radiation damping, comprise the SDOF models (see Crowley et al. 2017; Crowley and Pinho 2017 for more details). Once again it is noted that these SDOF models represent the global behaviour of the structure, but have been derived from MDOF models that include both in-plane and out-of-plane response (in the case of URM buildings).
Example SDOF backbone curve (Crowley and Pinho 2017)
3.2.2 Nonlinear dynamic ‘cloud’ analysis
A model of the probabilistic relationship between the ground motion intensity and the nonlinear structural response of the SDOF system has been developed using the cloud method (Jalayer 2003), due to its simplicity. Sufficiency of the selected intensity measures (i.e. independence with respect to other properties of the accelerograms, as discussed in Luco and Cornell 2007) has been checked to avoid the need to use hazard-consistent ground motions. The hazard model that has been developed for the field is dependent on the production scenario (see e.g. Bourne et al. 2015; Bourne and Oates 2017), and as the latter has been under significant fluctuation over recent years, this has made it more challenging to derive hazard consistent ground motions.
The cloud method has thus been employed using a large suite of records to ensure that a wide range of nonlinear structural response (from pre-yield to collapse) has been captured for all structural models, with limited need to scale records. The nonlinear dynamic analyses of each SDOF system have been undertaken in OpenSees (McKenna et al. 2000) and multivariate linear regression has been applied to the maximum nonlinear displacement response data for a range of scalar/vector intensity measures. The expected value of the natural logarithm of the displacement response (D) given the scalar/vector intensity measure (IM) is thus modelled by a linear regression equation (Eq. 2) with parameters b0 and bi (i = 1, …, m), whilst the standard deviation or dispersion (Eq. 3) is estimated by the standard error of the regression:
An example cloud data plot and associated regression is shown in Fig. 10, and it is noted that the observations with displacements greater than the collapse capacity have been plotted at the collapse displacement capacity value. A censored regression (Stafford 2008) has been applied to correctly treat these values for which the displacement response is not reliably predicted, but is known to exceed the displacement collapse capacity (see Crowley et al. 2017 for more details).
The sufficiency of four different scalar/vector IMs has been checked with respect to magnitude, distance and ground shaking duration: (1) spectral acceleration at an initial period (Sa[T1]), (2) spectral acceleration at T1 and 5–75% significant duration (DS5–75), (3) spectral acceleration at two periods T1 and T2, (4) spectral acceleration at two periods T1 and T2 and 5–75% significant duration. The final IM has been selected as that which is both sufficient and highly efficient (i.e. has the lowest standard deviation according to Eq. 3), and with a low Pearson coefficient (i.e. low correlation between the IMs).
3.2.3 Structural fragility functions
The regression analyses described in the previous section allow equations to be derived that relate the level of shaking with an estimate of the displacement response of an equivalent SDOF system. By identifying the thresholds to specific damage states in terms of SDOF displacements (or drifts, obtained by dividing the SDOF displacement by the effective height of the SDOF), it is possible to produce fragility functions that describe the probability of exceeding a number of distinct damage/collapse states. The variability in these damage/collapse state thresholds (βDL) should be accounted for in the dispersion of the response, and can be combined with the record-to-record variability (βR) and an additional building-to-building variability to account for changes in stiffness and strength of the backbone curve across the building class (βBB):
The damage/collapse state threshold variability has been assumed constant, with a value of 0.3, based on studies in the literature (e.g. Dymiotis et al. 1999; Borzi et al. 2008), the building-to-building variability has been taken as 0.1 and the record-to-record variability has been obtained directly from the cloud analyses.
The probability of exceeding the limit displacement to each structural damage or collapse state under a given level of ground shaking is thus calculated as follows:
where ln ηD|IM is given by Eq. (6) and Φ() is the cumulative distribution function of the standard normal distribution, DL is the displacement limit of each damage state, and βs is the total dispersion from Eq. 4 (i.e. due to record-to-record variability, backbone stiffness and strength variability and damage/collapse state threshold variability).
The following section describes the definition of DL for damage states DS2 and DS3 for each building class.
3.2.4 Damage state thresholds
Two structural damage states, similar to DS2 and DS3 in the EMS98 damage scale, have been identified for each structural system.
The drift levels at which damage occurs in the URM buildings has been informed by the large testing campaign that has been carried out on components and structures that match the construction practices and materials used in the Groningen field. A specific report focusing on the damage observed in the numerous tests has been compiled (Graziotti et al. 2017b), and its results in terms of the damage descriptions and levels of attic displacement have been used to identify SDOF drift limits to damage. The damage states have been defined as follows:
-
DS2: minor structural damage. This has been determined as the onset of cracking in primary resisting elements. The observed damage could be easily repaired.
-
DS3: significant structural damage. This level of performance was associated with a damage observed in all the piers contributing to the in-plane response of the building.
After each stage of the shake-table testing sequence, detailed surveys were carried out to obtain the maximum achieved top floor (attic) drift (%) at which a given level of damage was not observed. These values have been used to identify the attic limit state displacements for each damage state (DLi), which have then been transformed to SDOF drift levels by dividing by both the SDOF transformation factor and the effective height (Table 2). These drift limits have been found to be slightly lower than those proposed in the literature; for example, Borzi et al. (2008) assume a value of 0.13% for DS2 and 0.35% for DS3. These values are certainly conservative limits to damage, given that they have been obtained from a series of shake table tests wherein damage accumulates. However, given that the buildings in the Groningen field have already been subjected to a number of low magnitude events and in some cases they already have pre-existing damage from differential foundation settlement, it is assumed that it is appropriate to use these lower drift values for the damage assessment.
The drift levels at which damage is predicted to occur in wall–slab–wall reinforced concrete buildings typical of the region has been informed by the cyclic tests on cast-in-place and precast RC specimens (see Brunesi et al. 2018a, b). The results in terms of the damage descriptions and levels of attic displacement and associated SDOF drift have been used to identify the appropriate damage limits for the fragility functions.
The damage states that have been observed for the cast-in-place RC specimen are as follows:
-
DS2: full-depth hairline cracks at base of walls, and also cracks appearing at wall-slab joints.
-
DS3: Hairline cracks lengthen and extend, though with limited opening. Strength degradation begins.
whereas the damage states observed in the precast RC specimen are instead described as:
-
DS2: narrow cracks initiate around the wall connectors.
-
DS3: sliding of the slabs above walls and permanent flexural deformation in the connectors leading to residual displacements. Strength degradation initiates.
The values of SDOF drift at which each of the aforementioned damage states were reached in the cast-in-place (EUC-BUILD3) and pre-cast (EUC-BUILD4) RC cyclic tests are presented in Table 3.
For all other typologies (which are mainly RC, timber and steel frames) the recommended drift values for slight and moderate damage given in FEMA (2004) have been used.
3.2.5 Parameters of DS2 and DS3 fragility functions
A total of 54 structural systems have been identified in the Groningen field, and the parameters for the fragility functions of all these building types have been provided in Crowley and Pinho (2017). Herein, a summary of the fragility functions for the 5 most common URM building types and the 2 most common RC building types (in terms of number of buildings) is provided instead. These 7 structural systems make up over 70% of the building stock (see Table 4) and thus inevitably provide the largest contribution to the group damage plots presented in the next section. The parameters that have been obtained to compute the fragility functions according to Eq. 5 for these building types are presented in Table 5.
3.2.6 Consistency checks
As a consistency check on the performance of the URM fragility functions in Table 5, a comparison with the results of other fragility studies of European brick masonry buildings has been undertaken. A comparison of different fragility functions is never straightforward due to differences in the details of the building classes, the intensity measures used, and the definitions of the damage states. Many existing fragility functions for URM buildings are in terms of peak ground acceleration (PGA), whereas as described previously, the fragility functions derived herein are in terms of sufficient vector intensity measures, including spectral ordinates and significant duration.
Further, the majority of the URM buildings in the Groningen field are low rise (between 1 and 2 storeys) and are constructed with bricks, and a distinction has been made herein on the lateral load resisting system, the rigidity of the diaphragm and the presence of large openings at the ground floor (see descriptions in Table 4), whilst most of the European URM fragility functions found in GEM’s global vulnerability databaseFootnote 1 distinguish only between the type of masonry (brick or stone) and the number of storeys. There are two studies in the latter database that can be compared with the URM buildings presented herein, and they are both for 2-storey URM clay brick buildings with a low percentage of voids (Ahmad et al. 2011; Borzi et al. 2008). Both of these studies have derived fragility functions in terms of PGA, and the same limit state drifts have been assumed for the first two damage states (as presented previously in Sect. 3.2.4), though they are referred to as LS1 (light damage) and LS2 (significant damage) by Borzi et al. (2008) and slight and moderate by Ahmad et al. (2011).
In order to reconcile the different intensity measure types, the three sets of fragility functions have been combined with the uniform hazard spectra (UHS) with a 2475 year return period that have been developed for the area (van Elk et al. 2017) to produce maps of the conditional probability of exceedance of DS2 and DS3. The five URM types have been combined at each location by assuming a weighted average based on the total number of each typology in the field (Table 4). For type URM4L, the 5–75% significant duration is an input parameter, and it has been assumed to be equal to 1.5 s (based on the significant duration GMPE developed for the field by Bommer et al. 2018), though it is noted that the assumed value has not been found to significantly change the damage distribution. The maps for DS2 are presented in Fig. 11 and those for DS3 in Fig. 12.
These results show that the fragility functions for DS2 developed herein are similar to those from Borzi et al. (2008), though they predict higher values of DS2 exceedance at the edge of the exposure model. The DS2 limit state is a pre-yield limit state and thus the damage estimations are influenced by the elastic response of the masonry models. The fact that the DS2 drift limit used herein is lower than that of the other two studies (see Sect. 3.2.4) suggests that the capacity of the models used in this study have a higher initial stiffness (leading to lower displacement demands) than the models of Borzi et al. (2008). On the other hand, despite the lower drift limits for DS3, the forecast is lower than either of the other two studies, though in this case it is closer to that based on the fragility functions of Ahmad et al. (2011). As the DS3 damage state is taken to occur post-yield, the displacement demand and thus damage estimation is influenced by the base shear capacity of the models, and the lower results suggest that the strength of the URM models developed herein (which have been calibrated against experimental test results of full-scale building prototypes) is higher than that predicted in the other studies.
4 Probabilistic damage assessment
The probabilistic damage assessment is evaluated by Monte Carlo sampling of the aleatory variability within the causal sequence of conditional probability models and builds on the engine developed for a previous hazard model (Bourne et al. 2015). The steps of this engine are described in detail in van Elk and Doornhof (2017), and are illustrated in Fig. 13. In summary, a given production scenario is assumed, and then earthquake catalogues are simulated from the input probability distributions of total seismic moment, number of events and event epicentres, and for every simulated earthquake exceeding a given threshold, the ground motion model (Bommer et al. 2017b, 2018) forecasts the probability distribution of near-surface amplifications for every surface location within a dense surface grid of observation points.
Schematic diagram of probabilistic damage calculation process (van Elk and Doornhof 2017)
For the assessment of aggregate risk metrics (such as F-N curves for damage), spatial correlation is important and in the present model rules are applied in the sampling of the variability distributions such that near-perfect correlation of the motions at all grid points within zones of uniform site classification are produced, and zero correlation is assumed between zones. This simple model provides a good first order approximation to a more realistic model for the variation of spatial correlation with separation distance. The period-to-period correlation of spectral ordinates and correlation with significant duration is, on the other hand, explicitly modelled (Stafford et al. 2018), which is important given that the intensity measures (IM) of the fragility functions vary between building classes, and some make use of vector-based IMs, as described in Sect. 3.2.2. Given the simulated catalogue of ground motions, the structural fragility model is used to forecast the probability of exceedance of each damage state for each building class at each observation point. The aggregate number of buildings (N) that simultaneously reach or exceed DS2 or DS3 across the field (or within specific communities) for each simulation is then calculated and plotted against the annual frequency of exceedance (F).
For DS1, synthetic earthquake catalogues have only been generated for events between ML 1.8 and 4.0 (i.e. all events above 4.0 have been removed from the catalogue). The limitation on magnitude range is because the PGV ground-motion prediction equation (Bommer et al. 2017a) can only be used with confidence to estimate peak ground velocity in the Groningen field for earthquakes with magnitudes from 1.8 to 3.6. The model has been extrapolated slightly beyond the upper limit to 4 (in which range the extrapolation is most likely slightly conservative), but cannot be extrapolated further due to the purely linear magnitude scaling in the model, which would not be appropriate for a broader magnitude range. The equation can be applied with confidence up to 30 km and probably with reasonable confidence to 50 km from the epicentre. In all damage calculations it is assumed that any resulting building damage is repaired after the event and before the next one (i.e. ‘instant repair’).
Figure 14 shows the F-N curves in terms of the aggregate number of buildings (N) which simultaneously reach DS1, DS2 or DS3 across the field against the annual frequency of exceedance (F). These calculations have been undertaken for various gas depletion scenarios (van Elk and Doornhof 2017; Uilenreef et al. 2018), and only the results for the 24 bcm per year gas production scenario over a 5-year period from 2018 to 2022 are shown herein. As can be seen in Fig. 14, the exceedance curve for DS1 crosses that of the other two damage states, which is not realistic. The reason for this is due to the fact that the events larger than ML = 4.0 were removed from the catalogue (as the PGV GMPE was not valid for larger events) and because the DS1 fragility function is only well constrained for the data that is available at low levels of PGV (which have high frequencies of exceedance). There is a large level of variability in this empirical data (possibly due to the grouping of all buildings into just three categories), and this leads to high values of dispersion (see Table 1), which in turn lead to lower probabilities of exceedance at higher levels of ground shaking. Hence, the data shown in Fig. 14 are only reliable for the high frequencies of exceedance, and analytical fragility functions should be developed to constrain the probability of exceedance under levels of ground shaking that are higher than those that have been observed so far in the field.
A history check of the structural fragility functions has been carried out by estimating the F-N curves using the actual earthquake events with ML greater than 2.5 that have been observed in the field between 1995 and 2018. By calculating the expected value of the F-N damage curve and multiplying this by the number of years considered, it was found that the model predicts an average number of buildings with DS2 of 98 buildings, and with DS3 of 3 buildings. The same calculations have been undertaken using the seismicity between the years 2012–2018, and the average number of buildings with DS2 over this period was found to be 82 and with DS3 was calculated as 3. The prediction that the majority of damage occurred in the period 2012-2018 is expected, given that the two largest earthquakes in the field have occurred during this time period.
There is limited public data available on the number of buildings that have experienced DS2 and DS3 over the time periods considered in these history checks, and inspections that have been carried out have not just considered earthquake damage but also other sources of damage such as differential foundation settlement and lack of maintenance. However, the authors are aware that in the years following the Huizinge earthquake, the number of buildings that experienced damage greater than DS1 was less than a few hundred buildings, and thus the fragility models developed herein appear to provide consistent results with respected to actual structural damage observations.
5 Conclusions and future developments
This paper has presented the recent developments that have been undertaken to extend the risk assessment of the Groningen field for the assessment of damage in terms of group damage curves (known as F-N curves). For this purpose, fragility functions for non-structural and structural damage states have been estimated considering a combination of observed damage data, experimental testing and numerical modelling. The structural fragility functions have been compared with studies from the literature on similar building types, and have been found to give consistent results. The non-structural fragility functions are based on observed damage reports and whilst they are thus appropriate for use over the range of ground motions that have been experienced in the field, it has been shown that their extrapolation to higher ground motions leads to an underestimation of the damage. Future developments of this work will therefore look at deriving analytical fragility functions for DS1 calibrated using experimental test results of URM walls with plaster finish (and thus ensuring that all damage state fragility functions use the same intensity measures). Another aspect that will require more attention in future updates is the modelling of the various sources of correlation (e.g. spatial correlation of ground motions and building damage), which will influence the tails of the F-N curve and in particular the values of the group damage curves at low frequencies of exceedance.
References
Ahmad N, Crowley H, Pinho R (2011) Analytical fragility functions for reinforced concrete and masonry buildings and buildings aggregates of euro-Mediterranean regions—UPAV methodology. Internal Report, Syner-G Project 2009/2012
Arup (2017) Typology modelling: analysis results in support of fragility functions—2017 batch results. 229746_031.0_REP2005, NAM Platform, November 2017
Arup (2018) Data Documentation EDB V5. 229746_052.0_REP2014, Rev.0.09 ISSUE_DEF, NAM Platform
Arup, TU Delft, Eucentre (2015) Eucentre shake-table test of terraced house modelling predictions and analysis cross validation. NAM Platform, November 2015
Arup, TU Delft, Eucentre, Arcadis (2016a) EUC-BUILD2: modelling predictions and analysis cross validation of detached single-storey URM building. NAM Platform September 2016
Arup, TU Delft, Eucentre (2016b) Laboratory component testing: modelling post-test predictions and analysis cross validation. NAM Platform, February 2016
Arup, TU Delft, Eucentre, Mosayk (2017) LNEC-BUILD1: modelling predictions and analysis cross validation. NAM Platform, September 2017
ASI (2017) Extreme loading for structures v5. Applied Science International LLC, Durham
Avanes C, Fusco C, Mooneghi MA, Huang Y, Palmieri M, Sturt R (2018a) LS-DYNA numerical simulation of full scale masonry cavity wall terraced house tested dynamically. In: Proceedings of the 16th European conference in earthquake engineering, paper no. 10342, Thessaloniki
Avanes C, Montalbini G, Huang Y, Sturt R, Palmieri M (2018b) LS-DYNA numerical simulation of solid unreinforced masonry detached house tested dynamically. In: Proceedings of the 16th European conference in earthquake engineering, paper no. 10343, Thessaloniki
Bommer JJ, Alarcón JE (2006) The prediction and use of peak ground velocity. J Earthq Eng 10(1):1–31
Bommer J, Crowley H, Pinho R (2015) A risk-mitigation approach to the management of induced seismicity. J Seismolog 19:623–646
Bommer J, Stafford PJ, Ntinalexis M (2016) Empirical ground-motion prediction equations for peak ground velocity from small-magnitude earthquakes in the Groningen field using multiple definitions of the horizontal component of motion, NAM Platform, November 2016
Bommer JJ, Stafford PJ, Ntinalexis M (2017a) Empirical ground-motion prediction equations for peak ground velocity from small-magnitude earthquakes in the Groningen field using multiple definitions of the horizontal component of motion, NAM Platform, November 2017
Bommer JJ, Stafford PJ, Edwards B, Dost B, van Dedem E, Rodriguez-Marek A, Kruiver P, van Elk J, Doornhof D, Ntinalexis M (2017b) Framework for a ground-motion model for induced seismic hazard and risk analysis in the Groningen gas field, The Netherlands. Earthq Spectra 33(2):481–498
Bommer JJ, Edwards B, Kruiver P, Rodriguez-Marek A, Stafford PJ, Dost B, Ntinalexis M, Ruigrok E, Spetzler J (2018) v5 ground-motion model for the Groningen field. Revision 1. NAM Platform
Borzi B, Crowley H, Pinho R (2008) Simplified pushover-based earthquake loss assessment (SP-BELA) method for masonry buildings. Int J Archit Herit 2(4):353–376
Bourne SJ, Oates SJ (2017) Extreme threshold failures within a heterogeneous elastic thin-sheet account for the spatial–temporal development of seismicity induced by fluid extraction from a subsurface reservoir, Submitted to J Geophys Res Solid Earth
Bourne SJ, Oates SJ, Bommer JJ, Dost Β, van Elk J, Doornhof D (2015) A Monte Carlo method for probabilistic seismic hazard assessment of induced seismicity due to conventional gas production. Bull Seismol Soc Am 105(3):1721–1738
Brunesi E, Peloso S, Pinho R, Nascimbene R (2018a) Cyclic testing and analysis of a full-scale cast-in-place reinforced concrete wall–slab–wall structure. Bull Earthq Eng. https://doi.org/10.1007/s10518-018-0374-0
Brunesi E, Peloso S, Pinho R, Nascimbene R (2018b) Cyclic testing of a full-scale two-storey reinforced precast concrete wall–slab–wall structure. Bull Earthq Eng. https://doi.org/10.1007/s10518-018-0359-z
Brunesi E, Peloso S, Pinho R, Nascimbene R (2018c) Shake-table testing of a full scale two-story precast wall–slab–wall structure. Submitted to Earthq Spectra
Brzev S, Scawthorn C, Charleson AW, Allen L, Greene M, Jaiswal K, Silva V (2013) GEM Building Taxonomy Version 2.0. GEM Technical Report 2013-02 V1.0.0, GEM Foundation, Pavia. https://doi.org/10.13117/gem.ext-mod.tr2013.02
BSI (1993) Evaluation and measurements for vibration in buildings—part 2: guide to damage levels due to groundborne vibrations. BS 7385-2, British Standards Institute
Casarotti C, Pinho R (2007) An adaptive capacity spectrum method for assessment of bridges subjected to earthquake action. Bull Earthq Eng 5:377–390
Correia AA, Tomassetti U, Campos Costa A, Penna A, Magenes G, Graziotti F (2018) Collapse shake-table test on a URM-timber roof structure. In: Proceedings of the 16th European conference on earthquake engineering, paper no. 11929, Thessaloniki
Crowley H, Pinho R (2017) Report on the v5 fragility and consequence models for the Groningen field. NAM Platform, October 2017
Crowley H, Polidoro B, Pinho R, van Elk J (2017) Framework for developing fragility and consequence models for inside local personal risk. Earthq Spectra 33(4):1325–1345
Dost B, van Eck T, Haak H (2004) Scaling of peak ground acceleration and peak ground velocity recorded in the Netherlands. Boll Geofis Teorica ed Appl 45(3):153–168
Dost B, Edwards B, Bommer JJ (2018) The relationship between M and ML—a review and application to induced seismicity in the Groningen gas field, the Netherlands. Seismol Res Lett 89(3):1062–1074
Dymiotis C, Kappos AJ, Chryssanthopoulos MK (1999) Seismic reliability of RC frame with uncertain drift and member capacity. J Struct Eng 125(9):1038–1047
FEMA (2004) HAZUS-MH Technical Manual. Federal Emergency Management Agency, Washington
Graziotti F, Rossi A, Mandirola M, Penna A, Magenes G (2016a) Experimental characterization of calcium-silicate brick masonry for seismic assessment. In: Proceedings of the 16th international brick and block masonry conference (IBMAC), Padua, Italy, pp 1619–1627
Graziotti F, Tomassetti U, Penna A, Magenes G (2016b) Out-of-plane shaking table tests on URM single leaf and cavity walls. Eng Struct 125:455–470
Graziotti F, Tomassetti U, Kallioras S, Penna A, Magenes G (2017a) Shaking table test on a full scale URM cavity wall building. Bull Earthq Eng 15(12):5329–5364
Graziotti F, Tomassetti U, Penna A, Magenes M (2017b) Tests on URM clay and calcium-silicate masonry structures: identification of damage states. Eucentre Foundation, Pavia. Working Version of October 2017. www.eucentre.it/nam-project
Graziotti F, Tomassetti U, Sharma S, rottoli L, Magenes G (2018) Experimental response of URM single leaf and cavity walls in out-of-plane two-way bending generated by seismic excitation. Constr Build Mater, accepted for publication
Grunthal G, Musson RMW, Schwarz J, Stucchi M (1998) European Macroseismic Scale 1998. Centre Europeen de Geodynamique et de Seismologie, ISBN No2-87977-008-4
Jalayer F (2003) Direct probabilistic seismic analysis: implementing non-linear dynamic assessments. Ph.D. Dissertation, Stanford University
Kallioras S, Guerrini G, Tomassetti U, Marchesi B, Penna A, Graziotti F, Magenes G (2018) Experimental seismic performance of a full-scale unreinforced clay-masonry building with flexible timber diaphragms. Eng Struct 161:231–249
LSTC—Livermore Software Technology Corporation (2013) LS-DYNA—a general-purpose finite element program capable of simulating complex problems. Livermore
Luco N, Cornell CA (2007) Structure-specific scalar intensity measures for near-source and ordinary earthquake ground motions. Earthq Spectra 23(2):357–392
Malomo D, Pinho R, Penna A (2018a) Using the applied element method to model calcium-silicate brick masonry subjected to in-plane cyclic loading. Earthq Eng Struct Dyn 47:1610–1630
Malomo D, Comini P, Pinho R, Penna A (2018b) The applied element method and the modelling of both in-plane and out-of-plane response of URM walls. In: Proceedings of the 16th European conference in earthquake engineering, paper no. 10691, Thessaloniki
Malomo D, Pinho R, Penna A (2018c) Using the applied element method to simulate the dynamic response of full-scale URM houses tested to collapse or near-collapse conditions. In: Proceedings of the 16th European conference in earthquake engineering, paper no. 10692, Thessaloniki
McKenna F, Fenves GL, Scott MH, Jeremic B (2000) Open system for earthquake engineering simulation (OpenSees). Pacific Earthquake Engineering Research Center, University of California, Berkeley
Montalbini G, Avanes C, Alessi C, Huang Y, Palmieri M, Stuart R (2018) Numerical simulation of reinforced concrete tunnel-built structure tested bi-directionally under quasi-static cyclic loading. In: Proceedings of the 16th European conference in earthquake engineering, paper no. 10272, Thessaloniki
Mosayk (2014) Software verification against experimental benchmark data. NAM Platform, November 2014
Mosayk (2015) Structural modelling of non-URM buildings—v2 risk model update. NAM Platform, October 2015
Mosayk (2017) Nonlinear dynamic analysis of index buildings for v5 fragility and consequence models. NAM Platform, October 2017
NAM (2016) Technical Addendum to the Winningsplan Groningen 2016, Part V Damage and Appendices, NAM Platform, April 2016
NAM (2017) Methodology prognosis of building damage and study and data acquisition plan for building damage. NAM Platform, February 2017
SBR (2017) SBR Trillingsrichtlijn A: Schade aan bouwwerken:2017. SBRCURnet, Delft November 2017
Seismosoft (2017) SeismoStruct v2016—a computer program for static and dynamic nonlinear analysis of framed structures. http://www.seismosoft.com
Sharma S, Tomassetti U, Grottoli L, Graziotti F (2018) Out-of-plane two-way bending shaking table tests on single leaf and cavity walls. Report n. EUC137/2018U, Eucentre Foundation, Pavia, Italy. Available from NAM Platform
Stafford PJ (2008) Conditional prediction of absolute durations. Bull Seismol Soc Am 98(3):1588–1594
Stafford PJ, Zurek BD, Ntinalexis M, Bommer JJ (2018) Extensions to the Groningen ground-motion model for seismic risk calculations: component-to-component variability and spatial correlation. Bull Earthq Eng, this issue
TNO (2009) Kalibratiestudie schade door aardbevingen, TNO-034-DTM-2009-04435
Tomassetti U, Correia AA, Graziotti F, Marques AI, Mandirola M, Candeias PX (2017) Collapse shaking table test on a URM cavity wall building representative of a Dutch terraced house. Eucentre Foundation, Pavia, Italy and LNEC, Lisbon, Portugal, September 2017
Uilenreef J, van Elk J, Mar-Or A (2018) Assessment of building damage based on production scenario “Basispad Kabinet” for the Groningen field. NAM Platform
van Elk J, Doornhof D (2017) Assessment of hazard, building damage and risk. NAM Platform, November 2017
van Elk J, Doornhof D, Bommer JJ, Bourne SJ, Oates SJ, Pinho R, Crowley H (2017) Hazard and risk assessments for induced seismicity in Groningen. Neth J Geosc 96(5):s259–s269
Acknowledgements
The authors are grateful to TNO for providing access to the geo-referenced damage data, and special thanks are due to Chris Geurts and Huibert Borsje. The Groningen probabilistic seismic hazard and risk model described herein has been developed in collaboration with Julian Bommer, Stephen Bourne and Steve Oates, whose contributions to the study presented herein, through data contribution, model checking and many fruitful discussions, are thus gratefully acknowledged. Many of the details of the fragility model described in this paper were presented to an Assurance Panel comprising Jack Baker, Ihsan Engin Bal, Matjaz Dolsek, Paolo Franchin, Ron Hamburger, Nico Luco, Markus Schotanus and Dimitrios Vamvatsikos, whose valuable feedback and recommendations are thus gratefully acknowledged. Discussions, at different stages of this work, with Guido Magenes, Raphael Steenbergen, Peter Stafford, Iunio Iervolino, Francesco Graziotti, and Andrea Penna, were also very beneficial. Further, the contributions of the exposure model team at Arup, of the field/laboratory testing team at Eucentre, and of the structural modelling teams at Arup, Eucentre and Mosayk, are gratefully acknowledged. The authors would finally like to thank the two anonmymous reviewers for their constructive comments which helped improve the clarity of this manuscript.
Author information
Authors and Affiliations
Corresponding author
Rights and permissions
About this article
Cite this article
Crowley, H., Pinho, R., van Elk, J. et al. Probabilistic damage assessment of buildings due to induced seismicity. Bull Earthquake Eng 17, 4495–4516 (2019). https://doi.org/10.1007/s10518-018-0462-1
Received:
Accepted:
Published:
Issue Date:
DOI: https://doi.org/10.1007/s10518-018-0462-1