Abstract
In the domain of self-assessment, researchers have begun to draw distinctions between summative self-assessment activities (i.e., making an overall judgment of one’s ability in a particular domain) and self-monitoring processes (i.e., an “in the moment” awareness of whether one has the necessary knowledge or skills to address a specific problem with which one is faced). Indeed, previous research has shown that, when responding to both short answer and multiple choice questions, individuals are able to assess the likelihood of answering questions correctly on a moment-by-moment basis, even though they are not able to generate an accurate self-assessment of overall performance on the test. These studies, however, were conducted in the context of low-stakes tests of general “trivia”. The purpose of the present study was to further this line of research by investigating the relationship between self-monitoring and performance in the context of a high stakes test assessing medical knowledge. Using a recent administration of the Medical Council of Canada Qualifying Examination Part I, we examined three measures intended to capture self-monitoring: (1) the time taken to respond to each question, (2) the number of questions a candidate flagged as needing to be considered further, and (3) the likelihood of changing one’s initial answer. Differences in these measures as a function of the accuracy of the candidate’s response were treated as indices of each candidate’s ability to judge his or her likelihood of responding correctly. The three self-monitoring indices were compared for candidates at three different levels of overall performance on the exam. Relative to correct responses, when examinees initially responded incorrectly, they spent more time answering the question, were more likely to flag the question for future consideration, and were more likely to change their answer before committing to a final answer. These measures of self-monitoring were modulated by candidate performance in that high performing examinees showed greater differences on these indices relative to poor performing examinees. Furthermore, reliability analyses suggest that these difference measures hold promise for reliably differentiating self-monitoring at the level of individuals, at least within a given content area. The results suggest that examinees were self-monitoring their knowledge and skills on a question by question basis and altering their behavior appropriately in the moment. High performing individuals showed stronger evidence of accurate self-monitoring than did low performing individuals and the reliability of these measures suggests that they have the potential to differentiate between individuals. How these findings relate to performance in actual clinical settings remains to be seen.
Similar content being viewed by others
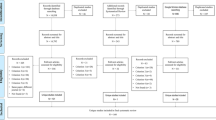
Explore related subjects
Discover the latest articles, news and stories from top researchers in related subjects.Avoid common mistakes on your manuscript.
Introduction
Education researchers have, for decades, emphasized the importance of accurate self-assessment in health professionals. As commonly expressed in the literature, self-assessment is thought to enable physicians to assess their own behaviours and performance in order to identify areas of relative strengths and weaknesses (Handfield-Jones et al. 2002). Unfortunately, it is now well established in the literature that summative self-assessment, that is, the ability to create a global judgment of one’s ability in a particular area, is quite poor (for reviews, see Gordon 1991 and Davis et al. 2006). More recently, however, researchers have suggested that the traditional “guess your grade” (Colliver et al. 2005) models of self-assessment might be problematic not only from a methodological perspective (Colliver et al. 2005; Ward et al. 2002) but also in their conceptualization of the importance of self-assessment in the self-regulatory process (Colliver et al. 2005; Regehr et al. 1996). Eva and Regehr (2005, 2007) theorized that the distinction between summative self-assessment and self-monitoring is particularly significant in the healthcare community, as physicians’ ability to self-monitor their performance in the moment of action is likely more important for the practice of safe and effective health care than the accuracy of their self-assessments of their overall ability. That is, it is possible that a physician could assess whether or not he has the necessary skills to perform a specific procedure for a particular patient, even if he is unable to create an accurate summative judgment of his overall ability on that procedure. Consequently, some researchers have started to draw distinctions between self-assessment as an overall summative evaluation of one’s performance (i.e., summative self-assessment) and self-assessment as an awareness, in the moment, of whether one has the necessary skills and knowledge to act in a specific situation (i.e., self-monitoring; Colliver et al. 2005; Eva and Regehr 2005, 2007, 2008, 2010; Moulton et al. 2007; Regehr and Eva 2006).
In one line of research related to this approach, Moulton and colleagues (Moulton et al. 2010a, b) explored the self-monitoring process within a complex, real-world clinical situation, demonstrating that experienced surgeons monitored their intra-operative activities and were able to avoid potential errors by appropriately paying more attention (i.e., “slowing down”) to a task when unusual or complicated circumstances arose. In a related line of research using a lab-based analog of the phenomenon described by Moulton et al. (2010a, b; Eva and Regehr 2007, 2010) provided quantitative evidence of the theoretical and functional distinction between summative self-assessment and self-monitoring. To quantify individuals’ capacity to self-monitor, Eva and Regehr had participants answer a series of general knowledge questions. Participants were told to answer questions only when they felt confident in their answer, and to defer answering when they were unsure of the correct response. Therefore, when presented with a question, participants had to consciously assess whether they had the knowledge to answer the question accurately. After completing the first round of questions and answering those questions they felt they could, participants were shown the deferred questions and were asked to take their best guess at the correct answer. In addition, participants were also asked to estimate how many questions they thought they would/did answer correctly, thereby providing a traditional measure of participants’ summative self-assessments. The authors found that participants were more likely to defer answering questions and, when they chose to answer, took longer to make this decision for questions that they ultimately answered incorrectly. These data suggest that participants were reasonably accurate, in the moment, in their assessment of whether or not they had the knowledge to answer a particular question. Despite this evidence of effective self-monitoring, participants’ overall prediction of how many questions they would/did answer correctly was poorly correlated with their actual performance, thereby replicating the results of previous self-assessment studies. Based on these results, the authors argued that moment-by-moment self-monitoring is not only distinct from summative self-assessment, but also a substantially more accurate process.
Results from studies such as Moulton et al. (2010a, b) and Eva and Regehr (2007, 2010) are encouraging because they suggest that individuals are able to effectively self-monitor their performance on a moment-by-moment basis, even though they are not very good at mentally aggregating performance across several events. Yet the situations represented by the two sets of studies are widely disparate not only in the task being performed, but also in the stakes of the decisions being made. The goal of the present research, therefore, was to act as a bridge between the high stakes, clinical context of Moulton’s work and the low stakes, non-clinical context of Eva and Regehr’s work. To this end, we examined self-monitoring in the context of the Medical Council of Canada (MCC) Qualifying Examination Part I (MCCQE Part I). The MCCQE Part I is a high-stakes, computer based examination that assesses the competence of medical students who wish to enter into supervised clinical practice in postgraduate training programs in Canada and is typically written at the end of medical school. Therefore, the study described here used quantitative behavioral indices of self-monitoring similar to those employed in Eva and Regehr’s research, but in a high-stakes situation relevant to clinical medicine.
Apart from measuring self-monitoring in a high-stakes medical licensure examination, the present study also differed from Eva and Regehr’s in three main ways. First, in addition to using behavioural indices of self-monitoring analogous to those used by Eva and Regehr (i.e., response time and response deferring), we also examined whether response changing could capture moment-by-moment self-monitoring. The effects of response changing on test performance have been thoroughly examined in the literature (i.e., Ferguson et al. 2002; Fischer et al. 2005; Geiger 1997; Higham and Gerrard 2005), and generally, the results are consistent with the notion that this activity may be an indicator of accurate self-monitoring. For example, as Ferguson et al. (2002) describe, when responses are changed on a test, the new answer tends to be more accurate than the original answer, although, the effect is slightly smaller for lower-performing students than higher-performing students. Further, students generally spend more time on questions for which they change the answers, and are more likely to change answers for items that have a higher difficulty index (Ferguson et al. 2002). The current study will seek to replicate and re-explore these findings in a self-monitoring framework. Second, we also examined the relationship between self-monitoring and candidate ability. Several researchers have shown that poor performers tend to overestimate their test performance relative to high performers (Di Milia 2007; Hodges et al. 2001; Kruger and Dunning 1999), and we wanted to determine whether these findings were specific to summative self-assessment or, as implied by the answer-changing literature, were generalizable to our measures of self-monitoring. And finally, we were interested in whether stable individual differences exist in the capacity to demonstrate self-monitoring in a given context. In contrast to previously published work, the large number of candidates writing the MCCQE Part I enabled us to examine the internal consistency of the self-monitoring indices and calculate the reliability of each index on the examination.
Method
Participants
Scores from 3,597 examinees who completed a recent administration of the MCCQE Part I were used in this study. All examinees were categorized into one of three ability levels based on their overall performance on the examination. Examinees were classified as “High Performers” if their total score was greater than half a standard deviation above the established passing score, as “Borderline Performers” if their total score was within half a standard deviation above or below the passing score, and as “Low Performers” if their total score was greater than half a standard deviation below the passing score.
Design
The MCCQE Part I is a one-day computer-based examination designed to assess candidates’ medical knowledge and clinical skills. The examination includes two components, one that consists of multiple-choice questions (MCQs) and another that consists of clinical decision making (CDM) scenarios. Only scores from the MCQ component were used in this study. The MCQ component of the examination is a multi-stage computer adaptive examination that assesses candidates’ knowledge in six medical disciplines: internal medicine, pediatrics, surgery, psychiatry, obstetrics and gynecology, and population health, ethics, legal and organizational aspects of medicine. A seventh discipline, family medicine, is made of relevant questions from the previous six disciplines. The MCQ component is presented to participants in seven sections, each containing 28 questions for a total of 196 questions (144 counting towards the examinee’s final score and 52 pilot questions). The first section of the examination is a routing section with an identical set of questions from all six disciplines presented to all examinees. The selection of the items in the subsequent six sections is adaptive in that an examinee’s performance within a discipline in a section determines the difficulty level of items from that discipline that the examinee will see in the next section. For example, examinees who perform well on surgery and poorly on pediatrics in one section will receive more difficult surgery questions but easier pediatric questions in the subsequent section.
In addition to the fixed questions of section 1 and the adaptive questions in the subsequent sections (all of which are used to determine the examinee’s final score), each examinee also receives 52 pilot questions of unknown difficulty that are scattered systematically throughout the 7 sections. Eight predetermined sets of 52 questions are created, and for each candidate one of the eight sets of pilot questions is randomly selected for presentation with the restriction that each form is presented to roughly the same number of examinees. Note that although, there are pilot questions on the MCCQE Part I, if these questions meet specified levels of quality in terms of their item statistics, they are calibrated and treated as scored items on the current examination. Because the MCCQE Part I examination consists of 120 adaptive questions and 76 non-adaptive questions (24 fixed questions in section 1 seen by all candidates and one of eight sets of 52 pilot questions each seen by one-eighth of the examinees), we initially conducted all analyses separately for adaptive and non-adaptive questions. By doing so, we hoped to examine self-monitoring skills when (1) the difficulty of questions was tailored to the ability of the examinee, and (2) the difficulty of questions varied consistently across all examinees, regardless of their overall performance. The results of these analyses revealed identical patterns for adaptive and non-adaptive items. As a result, we collapsed across adaptive and non-adaptive items and the final analyses were conducted on the entire examination (i.e., 196 questions) in order to maximize the stability of the scores for each person.
Dependent variables
To examine candidates’ capacity to self-monitor, we measured three dimensions of performance: (1) response time, (2) the proportion of questions flagged by a candidate, and (3) the proportion of questions for which the initial answer was changed. Each is described below.
Response time
Questions were presented individually to candidates on a computer screen and the computer recorded how long each question remained on the screen. In instances where the candidate returned to a previous question, the timer began timing where it left off and continued to measure the time spent on the question. This total response time per question was measured in seconds.
Question flagging
Examinees were given the opportunity to “flag” a question for future consideration or reconsideration. That is, an examinee could click on the flagging option, which resulted in that question being highlighted, to act as a reminder to the candidate to return to that question. The computer recorded which questions were flagged by each examinee.
Response changing
Each question consisted of a stem and five options, of which only one was correct. To select an option, the candidate clicked on the radio button next to the option or on the text of the option. The computer recorded each time an examinee selected a given option. If an examinee sequentially selected different options before committing to a final answer, the computer recorded both the identity and the number of response changes made by the candidate.
For each of these dimensions of performance, we calculated a candidate’s “score” separately for the questions answered correctly and questions answered incorrectly based on the initial response selected. In instances where only one response was made, the initial response was the final response. In instances where more than one response was made to a given question, we used the accuracy of the candidates’ initial response to determine whether the question was answered “correctly” or not. We made this decision because we theorized that flagging an item or changing the answer for an item with an initially incorrect response best represents instances in which a candidate was aware, either consciously or unconsciously, that the initial answer may not be correct. Thus, for the dimensions of question flagging and response changing, the use of initial response was determined to be a better mechanism for assessing self-monitoring than final response accuracy. Despite this theoretical reasoning, we also conducted the analyses using “final response” to define correct versus incorrect. The pattern of data did not change. So, because we believe, conceptually, that it is more appropriate to use initial response accuracy to determine our measures of self-monitoring, we report only the “initial response” comparisons in the results below.
Data analysis
As mentioned above, each examinee was classified as a “Low Performer”, “Borderline Performer”, or “High Performer”. For each of the three dependent variables, we conducted a two-way mixed design ANOVA, with examinee performance-level as the between subject variable and accuracy (score for correctly answered questions vs. score for incorrectly answered questions) as the within subject variable. Bonferroni corrections were applied to all multiple pairwise comparisons.
Our reason for creating ability groups based on the score distribution and applying ANOVA statistics rather than treating performance level as a continuous variable, was twofold. First, we wanted to present our results in a fashion similar to previous studies that examined the relationship between ability and summative self-assessment. Both Kruger and Dunning (1999) and Hodges et al. (2001) grouped candidates according to their overall score. Second, trichotomizing the ability variable allowed us to present our results graphically in a clear manner, which would not have been possible had we conducted a multiple regression analysis. That being said, we analyzed the data using both factorial ANOVA and multiple regression methods and the same conclusions could be drawn from the two analyses. Furthermore, given that three variables were used as measures of self-monitoring, we considered conducting a multivariate analysis of variance (MANOVA), as it would enable us to analyze the three dependent variables simultaneously. However, further investigations of the data revealed that our three measures of self-monitoring were poorly correlated with one another (r range: 0.04–0.21) and therefore, there was no additional value in using a MANOVA model over separate ANOVAs.
Finally, we calculated reliability coefficients to examine self-monitoring at the individual level. To do this, we created self-monitoring indices for each candidate by subtracting their mean score on each dependent variable for all correct responses from their mean score on the same variable for all incorrect responses. The three self-monitoring indices were calculated separately for each of the six test domains (i.e., medicine, pediatrics, surgery, psychiatry, obstetrics and gynecology, and population health). Each of the six difference scores was then treated as an item in a 6-item Cronbach’s alpha that was calculated for each dependent variable to investigate the internal consistency of these self-monitoring indices across the six test domains.
Ethics
Ethics approval was received from McMaster University’s Faculty of Health Sciences Research Ethics Board and MCC protocols to protect the identity of the candidates were followed.
Results
In total, 61.4% of the examinees were classified as high performers, 25.5% as borderline performers, and 13.1% as poor performers.
Response time measures
Overall, examinees spent less time on questions that were answered correctly relative to questions answered incorrectly [56.5 s vs. 68.3 s, respectively; F(1, 3,594) = 7,242, P < 0.001, η 2p = 0.67]. The performance-level of the examinee was also significantly related to response times, albeit with substantially lower effect sizes [F(2, 3,594) = 22.5, P < 0.001, η 2p = 0.01]. High performers answered questions more quickly than borderline performers [61.5 s vs. 62.6 s; t(3, 123) = 4.6, P < 0.001, d = 0.17], who, in turn, responded more quickly than poor performers [62.6 s vs. 63.0 s; t(1,388) = 2.2, P = 0.01, d = 0.11]. Accuracy and performance-level were also found to interact with one another to influence mean response times [F(2, 3,594) = 181.7, P < 0.001, η 2p = 0.09]. As can be seen in Fig. 1, the difference in response times between correct and incorrect responses was smallest, though still significant, in the poor performance group [t(471) = 26.0, P < 0.001, d = 1.20], was in the middle range for borderline performers [t(917) = 58.0, P < 0.001, d = 1.75], and was largest for high performers [t(2,206) = 102.0, P < 0.001, d = 2.17]. In other words, participants appeared to be slowing down, showing appropriate caution, on questions for which they were less likely to have the correct answer and the sensitivity of this self-monitoring process was somewhat modulated by the overall ability of the examinee.
Flagging examination questions
Examinees were given the opportunity to flag examination questions, indicating that they intended to revisit the question at a later time. Overall, examinees were more likely to flag questions that were answered incorrectly relative to questions that were answered correctly [10.0% vs. 5.8%; F(1, 3,594) = 769.6, P < 0.001, η 2p = 0.78]. Whether or not, an individual flagged a question was also influenced by his or her performance level [F(2, 3,594) = 106.8, P < 0.001, η 2p = 0.07]. Individuals with high performance were more likely to flag a given question than those with borderline performance [12.4% vs. 7.3%; t(3,123) = 9.6, P < 0.001, d = 0.35], and borderline performers, in turn, demonstrated a greater tendency to flag questions than examinees with low performance [4.2%; t(1,388) = 5.5, P < 0.001, d = 0.29]. Again, an interaction was observed in that the tendency to flag incorrect questions more often than correct questions was modulated by the ability of the examinee [F(2, 3,594) = 131.8, P < 0.001, η 2p = 0.07]. As Fig. 2 illustrates, high performing individuals were more likely to flag questions that were initially answered incorrectly relative to questions that were answered correctly [t(2,206) = 39.2, P < 0.001, d = 0.84]. Borderline individuals also showed a significant difference in question flagging for correct and incorrect answers [t(917) = 18.0, P < 0.001, d = 0.59], although, this difference was substantially smaller than the high performing examinees. And lastly, individuals with the poorest performance were still more likely to flag an incorrect question compared to a correct question [t(471) = 9.8, P < 0.001, d = 0.45], but the difference was smaller than for the other two ability groups. These results suggest that participants were able to recognize instances where the probability of an error was high and that the tendency to flag such questions appropriately was greatest for the high performers and smallest for the poor performers.
Response changing
Examinees were able to change their responses throughout the examination. Overall, responses to questions that were initially answered incorrectly were more likely to be changed relative to questions initially answered correctly [24.0% vs. 8.4%; F(1, 3,594) = 6,234, P < 0.001, η 2p = 0.63]. The proportion of response changes was related to exam performance [F(2,3,594) = 52.4, P < 0.001, η 2p = 0.03], in that high performers made more response changes than borderline performers [18.5% vs. 15.8%; t(3,123) = 7.4, P < 0.001, d = 0.29]. The difference in response changing behaviour for borderline and poor performers was also significant [15.8% vs. 14.4%; t(1,388) = 2.6, P = 0.01). And finally, performance-level and accuracy interacted with one another to influence the proportion of changed answers [F(2, 3,594) = 181.6, P < 0.001, η 2 p = 0.09]. As illustrated in Fig. 3, high performing individuals were more likely to change responses to questions that were initially answered incorrectly relative to questions that were initially answered correctly [t(2,206) = 91.5, P < 0.001, d = 1.95]. Borderline performers also showed a significant difference in response changing for correct and incorrect answers [t(917) = 49.5, P < 0.001, d = 1.64], although, this difference was substantially smaller among than the high performing examinees. Lastly, individuals with the poorest performance were more likely to change an initially incorrect question compared to an initially correct question, but the difference was smaller than it was in the other two ability groups [t(471) = 28.7, P < 0.001, d = 1.37]. The discovery that the higher performing candidates were more likely than lower performing candidates to change their responses to questions that were initially answered incorrectly suggests that higher performers were more aware, in the moment, of when they were making an error in their initial response to a particular question.
Reliability of the self-monitoring indices
While group comparisons are informative, it is also valuable to determine the extent to which self-monitoring can be used to differentiate between specific individuals. To study this issue, we created self-monitoring indices by calculating difference scores for each individual on each dependent measure by subtracting mean values for their correct responses from mean values for their incorrect responses. These indices were calculated separately for each of the six test domains.
The reliability coefficients for the three dependent measures are shown in Table 1. The reliability of the difference score for a single content domain was 0.09 for response time, 0.31 for question flagging, and 0.38 for response changing. The reliability of the average difference score across the six content domains (Cronbach’s alpha) was 0.38 for response time, 0.73 for question flagging, and 0.79 for response changing. By comparison, the same reliabilities for actual test scores across the six test domains were 0.57 for a single domain score and 0.88 for the average of the 6 domain scores. Taken together, these analyses demonstrate poor reliability for response time indices, but moderate to good reliability for flagging and response changing indices. The correlations between the individual indices and performance within each test domain were minimal (r < |0.24| in each instance).
Discussion
Eva and Regehr (2008) have argued that healthcare educators need to draw theoretical and methodological distinctions between summative self assessment (i.e., overarching judgments of one’s ability) and self-monitoring (i.e., moment-by-moment awareness of performance during a task). While previous research suggests that people are not effective at summative self-assessment (Dunning et al. 2004), the results from the current study yield further optimism that individuals are capable of monitoring themselves on a moment-by-moment basis, thereby replicating and extending the work of Eva and Regehr (2007, 2010). In the context of a high stakes examination, when the probability of making an initially erroneous response was high relative to other questions, examinees were more likely to (1) take additional time to respond, (2) flag the question as one for which they were uncertain of their response, and (3) alter their initial response before committing to a final answer. These results are also consistent with previous research showing that physicians’ take more time to provide a dermatological diagnosis when their eventual diagnosis is incorrect relative to when it is correct (Norman et al. 1989) and with the response changing literature which suggests that the decision to change responses is sensitive to the presence of initial errors (e.g., Ferguson et al. 2002).
A second major finding in the current study is that our measures of self-monitoring were modulated by the ability of the examinees. While all three performance groups showed sensitivity to their likelihood of being incorrect, the difference between correct and incorrect responses tended to be larger for better performers compared to poorer performers for all three dependent measures. These patterns of results suggest that poor performers are less likely than high performers to adjust their behavior to act more cautiously when in danger of making an error. Previous research has also shown that ability can modulate more traditional measures of self-assessment. For example, Kruger and Dunning 1999); see also Hodges et al. 2001) found that, relative to the best performers, the poorest performers were less likely to adjust their self-assessed performance scores appropriately after having been shown the performances of others on the same task. Further, again, these findings are consistent with the response changing literature, which shows similar modulating effects of overall ability on the value of response changes for overall score (Ferguson et al. 2002).
Finally, we were interested to see whether or not we could reliably differentiate between individuals based on indices of their self-monitoring success. Our analyses revealed poor reliability for response time indices, but moderate to good reliability for flagging and response changing indices. The reduced reliability for response time measures may be related to high intra-individual variability often associated with this measure (Salthouse and Berish 2005). These results may also have been affected by the timed nature of the test such that later response times may have been affected by an increased urgency to complete the test. Overall, however, our reliability analyses suggest that certain self-monitoring indices can be used to reliably differentiate between individuals, at least within the context of a specific task such as the MCCQE Part I. This suggests that stable individual differences may exist in the capacity to demonstrate self-monitoring in a given context.
This conclusion, however, must be bracketed with several caveats. First, we would note that the correlation between even our more reliable self-monitoring indices was quite low, suggesting that there are likely more subtle issues in play than a simple global self-monitoring ability that each of these measures taps into. Further, while the results of the reliability analyses suggest that we can reliably differentiate those who showed large differences in self-monitoring indices from those who showed small differences, this is not to say that self-monitoring is a context free skill that an individual either has or does not have. That is, we are not arguing that candidates who showed “greater” self-monitoring indices are in fact better at self-monitoring in all contexts. The efficiency of self-monitoring is almost certainly dependent on the both the content and context of the particular situation. Therefore, effective self-monitoring requires that an individual be sensitive to relevant situational cues and this likely depends on the individual’s competency within a particular context (Eva and Regehr 2008). Thus, rather than interpreting our findings as demonstrating that better self-monitoring ability is a vehicle for improving ability in a domain, it seems more likely that high performers are simply more competent in a wider range of medical domains and, therefore, are provided with more opportunity to demonstrate good self-monitoring relative to poor performers.
It is also important to note that the extent to which the current results generalize to clinical settings is unknown. Several researchers have suggested that self-monitoring is a critical aspect of patient safety (i.e., Colliver et al. 2005; Eva and Regehr 2005; Moulton et al. 2007, 2010a, b), arguing that healthcare professionals must continuously monitor the demands of each patient interaction to determine whether their current strategies are working effectively and whether they have the necessary skills, knowledge, and abilities to treat the patient. Such self-monitoring, which involves the ability to respond effectively to often subtle situational cues, requires an awareness that is sensitive to the particular content and context of a given problem Moulton et al. (2007, 2010a, b). Thus, the extent to which the quantification of the processes we observed in the context of test taking activities can be meaningfully transferable to clinical settings is at best speculative. Indeed, patterns of data similar to those we found have been interpreted in other literatures as “test-taking strategies” rather than self-monitoring (Rogers and Bateson 1991). That is, the efficient application of test-tasking strategies appears to be related to the overall knowledge base of the examinee, which may account for the present data. Clearly more research is needed to understand if and how “test-taking strategies” are conceptually different from self-monitoring activities before the results of such lab-based models of self-monitoring can be extrapolated to real-world, patient-safety strategies.
Despite these caveats, however, the present study has the potential to contribute to a more optimistic view of self-assessment relative to studies that operationalize self-assessment as an overall judgment of one’s ability. While individuals may not be good at rating their general strengths and weaknesses, they appear more capable of monitoring their skills and knowledge in the moment to determine whether or not they are able to address specific problems (or answer particular questions). Further, the ability to quantify this process in the context of high stakes performance has the potential to add an important tool in our efforts to understand and exploit this critical self-monitoring process in daily professional practice.
References
Colliver, J. A., Verhulst, S. J., & Barrows, H. S. (2005). Self-assessment in medical practice: A further concern about the conventional research paradigm. Teaching and Learning and Medicine, 17, 200–201.
Davis, D. A., Mazmanian, P. E., Fordis, M., Harrison, R. V., Thorpe, K. E., & Perrier, L. (2006). Accuracy of physician self-assessment compared with observed measures of competence: A systematic review. JAMA, 296, 1094–1102.
Di Milia, L. (2007). Benefits from multiple-choice exams: The positive impact of answer switching. Educational Psychology, 27, 607–615.
Dunning, D., Heath, C., & Suls, J. (2004). Flawed self-assessment: Implications for health, education, and the workplace. Psychological Science in the Public Interest, 5, 69–106.
Eva, K. W., & Regehr, G. (2005). Self-assessment in the health professions: A reformulation and research agenda. Academic Medicine, 80, S46–S54.
Eva, K. W., & Regehr, G. (2007). Knowing when to look it up: A new conception of self-assessment ability. Academic Medicine, 82, S81–S84.
Eva, K. W., & Regehr, G. (2008). I’ll never play professional football and other fallacies of self-assessment. Journal of Continuing Education in the Health Professions, 28, 14–19.
Eva, K. W., & Regehr, G. (2010). Exploring the divergence between self-assessment and self-monitoring. Advances in Health Sciences Education [epub ahead of publication].
Ferguson, K. J., Kreiter, C. D., Peterson, M. W., Roawt, J. A., & Elliott, S. T. (2002). Is that your final answer? Relationship of changed answers to overall performance on a computer-based medical school course examination. Teaching and Learning in Medicine, 14, 20–23.
Fischer, M. R., Herrmann, S. M., & Kopp, V. (2005). Answering multiple choice questions in high-stakes medical examinations. Medical Education, 39, 890–894.
Geiger, M. A. (1997). An examination of the relationship between answer changing, testwiseness, and examination performance. Journal of Experimental Education, 66, 49–60.
Gordon, M. J. (1991). A review of the validity and accuracy of self-assessments in health professions training. Academic Medicine, 66, 762–769.
Handfield-Jones, R. S., Mann, K. V., Challis, M. E., Hobma, S. O., Klass, D. J., McManus, I. C., et al. (2002). Linking assessment to learning: A new route to quality assurance in medical practice. Medical Education, 36, 949–958.
Higham, P. A., & Gerrard, C. (2005). Not all errors are created equal: Metacognition and changing answers on multiple-choice tests. Canadian Journal of Experimental Psychology, 59, 28–34.
Hodges, B., Regehr, G., & Martin, D. (2001). Difficulties in recognizing one’s own incompetence: Novice physicians who are unskilled and unaware of it. Academic Medicine, 76, S87–S89.
Kruger, J., & Dunning, D. (1999). Unskilled and unaware of it: Difficulties in recognizing one’s own incompetence lead to inflated self-assessments. Journal of Personality and Social Psychology, 77, 1121–1134.
Moulton, C. E., Regehr, G., Mylopoulos, M., & MacRae, H. M. (2007). Slowing down when you should: A new model of expert judgment. Academic Medicine, 82, S109–S116.
Moulton, C. E., Regehr, G., Lingard, L., Merritt, C., & MacRae, H. (2010a). ‘Slowing down when you should’: Initiators and influences of the transition from the routine to the effortful. Journal of Gastrointestinal Surgery, 14, 1019–1026.
Moulton, C. E., Regehr, G., Lingard, L., Merritt, C., & MacRae, H. (2010b). Slowing down to stay out of trouble in the operating room: Remaining attentive in automaticity. Academic Medicine, 85, 1571–1577.
Norman, G. R., Rosenthal, D., Brooks, L. R., Allen, S. W., & Muzzin, L. J. (1989). The development of expertise in dermatology. Archives of Dermatology, 125, 1063–1068.
Regehr, G., & Eva, K. W. (2006). Self-assessment, self-direction, and the self-regulating professional. Clinical Orthopaedics and Related Research, 449, 34–38.
Regehr, G., Hodges, B., Tiberius, R., & Lofchy, J. (1996). Measuring self-assessment skills: An innovative relative ranking model. Academic Medicine, 71, S52–S54.
Rogers, W. T., & Bateson, D. J. (1991). Verification of a model of test-taking behaviour of high school seniors. Journal of Experimental Education, 59, 331–350.
Salthouse, T. A., & Berish, D. E. (2005). Correlates of within-person (across-occasion) variability in reaction time. Neuropsychology, 19, 77–87.
Ward, M., Gruppen, L., & Regehr, G. (2002). Measuring self-assessment: Current state of the art. Advances in Health Sciences Education, 7, 63–80.
Author information
Authors and Affiliations
Corresponding author
Rights and permissions
About this article
Cite this article
McConnell, M.M., Regehr, G., Wood, T.J. et al. Self-monitoring and its relationship to medical knowledge. Adv in Health Sci Educ 17, 311–323 (2012). https://doi.org/10.1007/s10459-011-9305-4
Received:
Accepted:
Published:
Issue Date:
DOI: https://doi.org/10.1007/s10459-011-9305-4