Abstract
Airborne pollen are largely studied to obtain information about the atmospheric content of natural allergens. Aerobiological monitoring networks have been established to provide reliable data that facilitate the timely initiation of preventive actions aimed at minimizing allergic symptoms. Airborne pollen are usually identified and counted using an optical microscope, but as such procedures are extremely time-consuming, more expedient options are being explored. We have assessed the potential of Fourier transform infrared (FT-IR) spectroscopy as an alternative method for the rapid and reliable identification of allergenic pollen using six well-known allergenic pollen taxa and obtaining the respective FT-IR spectra. In doing this, a first IR spectral library has been created. The spectra of unknown pollen were compared to those of the reference library, and two pollen taxa of a mixed sample were identified.
Similar content being viewed by others
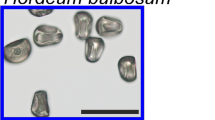
Avoid common mistakes on your manuscript.
1 Introduction
The prevalence of respiratory allergic diseases has increased dramatically during the last 20 years, especially in industrialized countries (Verlato et al. 2003). Pollen grains are recognized as important agents causing allergies in susceptible subjects (D’Amato et al. 1991; Verini et al. 2001; Johansson 2002).
Pollen grains, which contain the male gametes, represent the male partner in sexual reproduction of seed plants. The transfer of pollen from the pollen-producing part of the plant, the anther, to the female reproductive part, which is the stigma in angiosperms and the seminal primordia in gymnosperms, can be achieved through the mediation of biotic or abiotic agents. The most allergenic pollen grains are wind-pollinated, and airborne pollen grains are produced and shed into the atmosphere in very great quantities (Nilsson and Praglowski 1992).
The airborne pollen spectrum (types and amounts) at any given moment and place basically depends on the composition of the vegetation of the area (Faegri and Iversen 1989) and, consequently, presents a strong seasonality due to the typical flowering times of various species. Quantitative variations in airborne pollen are due to the amounts of pollen produced, which in turn depend on plant rhythms (Khanduri and Sharma 2002), and to meteorological conditions before (Latalowa et al. 2002) and during the flowering period (Corden et al. 2002; Riera et al. 2002).
Although exposure to natural allergens is almost impossible to avoid, it is possible to adopt protective and preventive measures to reduce allergic symptoms. These measures are possible only when accurate information is available on the atmospheric content of biologic particulate and its composition, abundance and period of presence (Levetin and Van de Water 2003; Chuine and Belmonte 2004). The constant monitoring of airborne biological particles is therefore extremely important, not only for providing such accurate information and thereby facilitating decisions on timely and adequate prophylaxis measures, but also for gaining an understanding of the factors associated with pollinosis. Reliable information for a specific area can best be obtained by means of an adequate number of aerobiological monitoring stations that monitor the phytogeographical and vegetational characteristics of the interested area. To this end, the national aerobiological networks provide monitoring of airborne pollen and publish bulletins and forecasts (see http://www.polleninfo.org for the European network).
Pollen grains are usually collected with an Hirst-type volumetric spore trap and are identified on the basis of morphological features and patterning (size, shape, surface, apertures of grains) following examination under an optical microscope (Faegri and Iversen 1989; Moore et al. 1991). In particular, the aerobiological center of the Italian Network follows a standardized protocol for pollen sampling and treatment, which has become regulation UNI 11108 in August 2004 (Mandrioli, 2004). Such methods, however, are largely time-consuming and require specific knowledge. In some cases the number of sampling sites and the development of an adequate monitoring network are necessarily hindered by these difficulties. Therefore, a faster and highly automated method would be very useful. To this end, a number of alternative approaches to pollen analysis have been reported. A semi-automatic system for pollen recognition and counting, based on the use of three-dimensional image processing, was proposed by Boucher et al. (2002). Other approaches involve the chemical characterization of the pollen grains, performed either by means of Raman spectroscopy (Laucks and Davis 1998; Laucks et al. 2000; Sengupta et al. 2005) or Raman microscopy (Ivleva et al. 2005). Fourier transform infrared (FT-IR) spectroscopy seems to be another useful methodology for pollen identification (Pappas et al. 2003).
FT-IR spectroscopy has been shown to be a very useful technique in several research fields and, in particular, for the analysis of organic material (Nabet and Pezolet 1997; Petibois et al. 2006). It provides information on the chemical bonding or molecular structure of materials. The basis of the technique is the property of chemical bonds and groups of bonds to vibrate at characteristic frequencies: a molecule that is exposed to infrared rays absorbs infrared energy at frequencies specifically characteristic of that molecule. During FT-IR analysis, a spot on the specimen is subjected to a modulated IR beam. The specimen’s transmittance and reflectance of the infrared rays at different frequencies are translated into an IR absorption plot consisting of reverse peaks. The resulting FT-IR spectral pattern is then analyzed and matched with known signatures of identified materials in a FT-IR library (from http://www.siliconfareast.com/FTIR.htm).
If the research is focused on particular class of materials, it is common practice to build an FT-IR library in the laboratory starting from pure substances. An IR spectrum represents a fingerprint of a sample, with absorption peaks corresponding to the frequencies of vibrations between the bonds of the atoms making up the material. Because each material is made up of a unique combination of atoms, it is impossible to find two different compounds ever producing exactly the same IR spectrum. Therefore, IR spectroscopy can positively identify (qualitative analysis) each unique kind of material – with the extra advantage of not destroying the sample (non-destructive method). In addition, the size of the peaks in the spectrum is a direct indication of the amount of material analyzed (quantitative analysis) (from: http://www.thermonicolet.com), and it can provide a precise measurement without external calibration. Finally, FT-IR measurements do not require prior complex sample preparation.
FT-IR spectroscopy has been successfully used in the past for the characterization and identification of microorganisms (Orsini et al. 2000; Mariey et al. 2001; Miguel Gomez et al. 2003; Pastuszka et al. 2005; Al-Holy et al. 2006).
The first scientists to apply FT-IR spectroscopy for pollen identification were Pappas et al. (2003). They created a library consisting of the spectra of 20 pollen types, both in diffuse reflectance and KBr pellet. For each FT-IR spectrum, they identified a fingerprint region, between 1500–800 cm−1, which then enabled them to discriminate the pollen of different species. Their high match values were verified by comparing spectra of unknown pollen with the corresponding library.
We report here the first step in the development of an alternative fast, easy and reliable method for qualitative–quantitative analysis of airborne pollen. The objectives of our investigation were:
-
to create a FT-IR reference spectral library of well-known allergenic pollen grains;
-
to explore and improve the potentiality of FT-IR spectroscopy in allergenic pollen identification.
2 Materials and methods
2.1 Pollen samples
Samples of allergy-relevant pollen were collected at flowering time from the following plants: Artemisia vulgaris L. (mugwort), Betula pendula Roth (silver birch), Castanea sativa Mill. (sweet chestnut), Corylus avellana L. (hazel), Fraxinus ornus L. (manna-ash) and Ostrya carpinifolia Scop. (hop hornbeam). Pollen samples were sieved through a mesh (50-μm pore size) and stored in small vials in a desiccator at +5°C until use. Each sample was used as a standard for the reference spectra library construction.
A mixture of two pollen taxa was prepared using silver birch (10% in weight) and hop hornbeam (90%) for a first attempt at identifying the pollen components of a composite sample. We selected these two species because their pollen could be found in the atmosphere during the study period and because it is difficult to distinguish these two pollen types under a light microscope.
Pre-hydrated pollen samples of the six different plant species were observed, checked and photographed with an environmental scanning electron microscope (ESEM). ESEM is a particularly useful technology when working with delicate biological samples as it does not involve conductive coatings (Collins et al. 1993), and it is not necessary to work in low vacuum, thereby avoiding collapse of the pollen grain. In addition, pollen samples can be observed without the deposition of a gold layer, which is necessary when using a traditional SEM.
2.2 FT-IR spectroscopy
Pollen spectra were obtained in by means of FT-IR spectroscopy using the Bio-Rad model FTS-165 instrument. The analysis were performed in an attenuated total reflectance (ATR) mode on diamond single reflection, in the frequency range of 4000–500 cm−1. Win-IR software by Galactic Industries was used to analyze the spectra and build a spectral library of the six pollen taxa studied. An area of 0.6 mm2 and a thickness of about 2–3 μm were scanned during the IR analysis of each pollen grain; for each pollen grain sampled, 64 scans were made.
Following the construction of the pollen library, the spectrum of “unknown” pollen (selected among those used for the library construction) was determined and subsequently compared with the library for identification. The identification was performed by means of Bio-Rad Search software that evaluates the similarity between an unknown spectrum and the library using the Euclidean distance algorithm. The algorithm calculates an Hit Quality Index (HQI) that ranges between 000 and 999. The HQI number is obtained from the fitting, and the program approximates to the whole number. Values behind the decimal points are not visualized, but they taken into consideration in producing the list of probable substances. This procedure produces a list of taxa with the relative match value (HQI): the larger the numerical value for the HQI, the better the match between the unknown and the reference spectrum (Bio-Rad Search manual, Technical Publications, Bio-Rad Laboratories, Cambridge, Mass.). When two or more substances show the same HQI, it is necessary to consider the order in the show list: the most probable substance is indicated at the first position of the list.
The final steps of our evaluation consisted of: (1) analyzing the two-taxa mixture and comparing its spectrum with the library, which allowed us to identify the main component; (2) subtracting the spectrum of the previously identified pollen from the mixture spectrum; (3) comparing once again the remaining spectrum with the library for the identification of the second component of the mixture. From the intensity of the peaks it was also possible to obtain information on the relative quantity of pollen in the sample.
3 Results
Morphological observation of the pollen samples performed with an ESEM confirmed their botanical origin. This procedure was adopted to ensure the construction of a reliable spectral library. ESEM photographs of of the pollen species studied are shown in Fig. 1. The main morphological features of the pollen grains that allow their identification are, in brief:
-
Artemisia vulgaris L. (mugwort) pollen grains are trizonocolpate and have an echinate exine with short spinules;
-
Betula pendula Roth (silver birch) pollen grains are trizonoporate with microechinate exine;
-
Castanea sativa Mill. (sweet chestnut) pollen grains are trizonocolporate with psilate-rugulate exine;
-
Corylus avellana L. (hazel) pollen grains are trizonoporate with a scabrate exine;
-
Fraxinus ornus L. (manna-ash) pollen grains are trizonocolpate and have a reticulate exine;
-
Ostrya carpinifolia Scop. (hop hornbeam) pollen grains are trizonoporate with psilate-scabrate exine.
It should be noted that hazel, silver birch and hop hornbeam pollen grains all have three pores and are often difficult to discriminate, particularly when using an optical microscope. Therefore, an alternative method for pollen analysis could be useful for resolving doubts in pollen identification.
The recorded FT-IR spectra of the six pollen species are shown in Fig. 2: the typical wave number range, between 4000 and 500 cm−1, are reported, and the most important peaks are indicated on the spectra. To identify these peaks, we first studied the typical chemical bonds present in the pollen matter and used the typical chemical bond peaks of the fitting program. We then studied the bonds in the range between 1800 and 500 cm−1, paying particular attention to differences in the peaks’ signals. In this particular region, in fact, the relative FT-IR peaks' signals, called the “fingerprint”, are typical of each taxon.
Fourier transform infrared (FT-IR) spectra of the six pollen taxa studied: (A) Artemisia vulgaris L. (mugwort), (B) Betula pendula Roth (silver birch), (C) Castanea sativa Mill. (sweet chesnut), (D) Corylus avellana L. (hazel), (E) Fraxinus ornus L. (manna-ash), (F) Ostrya carpinifolia Scop. (hop hornbeam)
The most important chemical bonds highlighted by FT-IR spectroscopy and the typical frequencies are listed in Table 1.
The comparison of “unknown” pollen spectra with those of the reference library allowed to identify all pollen samples. Figure 3 shows the example of an “unknown” pollen spectrum and the fitting result obtained by comparison with the library. In the lower part of the figure, the list of the most likely taxa with the correspondent HQI values is shown: it is possible to observe how the fitting program successfully individuates the right species among those with the same match values.
Figure 4a shows the spectrum of the two-pollen mixture and the fitting spectrum selected from the created library as being the most alike; the list below the spectrum displays the taxa identified from the fitting program, with the first one representing the larger component of the mixture (Ostrya carpinifolia Scop., hop hornbeam). The hop hornbeam spectrum was then subtracted from the sprectrum of the mix; the resulting spectrum is shown in Fig. 4b.
Identification of a mixed pollen sample. (A) Comparison of the measured FT-IR spectrum to the library and recognition of the main component of the mixture (hop hornbeam). (B) Subtraction of the hop hornbeam spectrum from the mixture spectrum. (C) Comparison of the remaining spectrum to the library and identification of the second component (silver birch). HQI values and the relative positions in the list are also reported
A second research fitting was then carried out: Fig. 4c shows the corresponding spectrum. The fitting program recognized the second pollen type as silver birch among the available spectra in the library (the one with the higher HQI value and at the first position in the list).
Subtraction of the spectrum of the first component (taxon) identified in the spectrum of the mixture could have resulted in the partial subtraction of peaks associated with the second taxon in areas that they overlapped. For this reason, in some regions, the obtained curve does not show a perfect correspondence with that selected from the library and its HQI value was lower than expected (Fig. 4c). Despite this drawback, FT-IR spectroscopy resulted in the correct identification of both mixture components.
4 Discussion and conclusions
The results of our investigation show that FT-IR spectroscopy provides specific spectra for different pollen species and that it enables the rapid identification of unknown pollen, thereby confirming the results of Pappas et al. (2003). The reliability of this method was demonstrated by its power to discriminate two different pollen taxa in a simple mixture. However, we must state that we have to take in account that the two-component mixture was intentionally unbalanced, with the proportion of one pollen taxon being much greater (90%) than that of the other (10%). In this way, the relative spectrum was probably very similar to that of the more abundant pollen, favoring its identification.
We can assume that a mixture spectrum is different from that of the sum total of single spectrum of every taxa that compose it. Therefore, each mixture curve should depend on the quantitative ratio of the pollen grains. With the aim of improving the pollen spectral library, future investigations should collect data on different mixtures of pollen by varying both the number of pollen taxa and their relative weight in the mixture. This would enable calibration models to be set up that could be used to determine unknown mixtures. Nevertheless, the characterization of aerobiological samples often occur in samples where many different pollen taxa are present, so it could be very difficult to simulate actual complexity in order to create an exhaustive spectra library. One possible solution could be the use of the FT-IR microscopy as this technology enables spectra from single grains to be analyzed, as performed by Raman microscopy (Ivleva et al. 2005). However, this approach could be time-expensive because it requires, for each pollen, the acquisition of the relative spectrum, the comparison with all spectra in the reference library and the calculation of the match value. We therefore suggest that future work be devoted either to improving the spectra library of reference pollen or to developing a method to obtain quantitative as well as qualitative analysis of single pollen grains.
The creation of libraries could be also obtained through the cooperation of different research units. As in other research fields (for example the Irug database in the art, architecture and archaeological field: http://www.irug.org), the posting of pollen FT-IR spectra on the Web could be a very useful and economic tool and may facilitate collaborations with different research centers.
The primary objective of the study reported here was to evaluate an alternative method to analyzing airborne pollen associated with allergic diseases. However, the study of pollen grains can make a significant contribution to a large range of other scientific fields (Moore et al. 1991), including taxonomy, melissopalinology, forensic science, paleopalinology, human impacts on vegetation, among others. In comparison to three-dimensional image processing (Boucher et al. 2002), FT-IR spectroscopy seems to be easier in terms of knowledge acquisition necessary for the construction of reference data sets as well as sample analysis, requiring no specific palynological skills. Therefore, FT-IR spectroscopy could be a very helpful method for rapid pollen identification in different disciplines and in a large number of studies.
References
Al-Holy, M. A., Lin, M., Al-Qadiri, H., Cavinato, A. G., & Rasco B. A. (2006). Classification of foodborne pathogens by Fourier transform infrared spectroscopy and pattern recognition techniques. Journal of Rapid Methods and Automation in Microbiology, 14, 189–200.
Boucher, A., Hidalgo, P., Thonnat, M., Belmonte, J., Galan, C., & Bonton, P. (2002). Development of a semi-automatic system for pollen recognition. Aerobiologia, 18, 195–201.
Chuine, I., & Belmonte, J. (2004). Improving prophylaxis for pollen allergies: Predicting the time course of the pollen load of the atmosphere of major allergenic plants in France and Spain. Grana, 43, 65–80.
Collins, S. P., Pope, R. K., Scheetz, R. W., Ray, R. I., Wagner, P. A., & Little, B. J. (1993). Advantages of environmental scanning electron microscopy in studies of microorganisms. Microscopy Research and Technique, 25, 398–405.
Corden, J. M., Stach, A., & Millington, W. M. (2002). A comparison of Betula pollen seasons at two European sites; Derby, United Kingdom and Poznan, Poland (1995–1999). Aerobiologia, 18, 45–53.
DΓÇÖAmato, G., Spieksma, F. Th. M., & Bonini, S. (1991). Allergenic pollen and pollinosis in Europe. Oxford, Great Britain: Blackwell Scientific Publications.
Faegri, K., & Iversen, J. (1989) Textbook of pollen analysis. London, Great Britain: John Wiley & Sons Ltd.
Ivleva, N. P., Niessner, R., & Panne U. (2005). Characterization and discrimination of pollen by Raman microscopy. Analytical and Bioanalytical Chemistry, 381, 261–267.
Johansson, S. G. O. (2002). Milestones in understanding allergy and its diagnosis. Clinical and Experimental Allergy: Review, 2, 2–7.
Khanduri, V. P., & Sharma, C. M. (2002). Pollen productivity variations. Pollen-ovule ratio and sexual selection in Pinus roxburghii. Grana, 41, 29–38.
Latalowa, M., Mietus, M., & Uruska, A. (2002). Seasonal variations in the atmospheric Betula pollen count in Gdansk (southern Baltic coast) in relation to meteorological parameters. Aerobiologia, 18, 33–43.
Laucks, M. L., & Davis, E. J. (1998). Chemical characterization of single pollen particles via fluorescence and raman spectroscopy. Journal of Aerosol Science, 29, s603–s604.
Laucks, M. L., Roll, G., Schweiger, G., & Davis, E. J. (2000). Physical and chemical (RAMAN) characterization of bioaerosols—pollen. Journal of Aerosol Science, 31, 307–319.
Levetin, E., & Van de Water, P. K. (2003). Pollen count forecasting. Immunology and Allergy Clinics of North America, 23, 423–442.
Mandrioli, P. (2004). Metodo di campionamento e conteggio dei granuli pollinici e delle spore fungine aerodisperse. UNI 11108.
Mariey, L., Signolle, J. P., Amiel, C., & Travert, J. (2001). Discrimination, classification, identification of microorganisms using FTIR spectroscopy and chemometrics. Vibrational Spectroscopy, 26, 151–159.
Miguel Gomez, M. A., Bratos Pérez, M. A., Martin Gil, M. A., Duenas Diez, A., Martin Rodriguez, J. F., Gutiérrez Rodriguez, P., Orduna Domingo, A., & Rodriguez Torres, A. (2003). Identification of species of Brucella using Fourier transform infrared spectroscopy. Journal of Microbiological Methods, 55, 121–131.
Moore, P. D., Webb, J. A., & Collinson, M. E. (1991). Pollen analysis. Oxford, Great Britain: Blackwell Scientific Publications.
Nabet, A., & Pezolet, M. (1997). Two-Dimensional FT-IR Spectroscopy: A Powerful Method to Study the Secondary Structure of Proteins Using H-D Exchange. Applied Spectroscopy, 51, 466–469.
Nilsson, S., & Praglowski, J. (1992). Erdtman’s Handbook of Palynology. Kobenhavn, Denmark: Munksgaard.
Orsini, F., Ami, D., Villa, A. M., Sala, G., Bellotti, M. G., & Doglia, S. M. (2000). FT-IR microspectroscopy for microbiological studies. Journal of Microbiological Methods, 42, 17–27.
Pappas, C. S., Tarantilis, P. A., Polissiou, M. G., & Harizanis, P. C. (2003). New method for pollen identification by FT-IR spectroscopy. Applied Spectroscopy, 57, 23–27.
Pastuszka, J. S., Talik, E., Hacura, A., Sloka, J., & Wlazlo, A. (2005). Chemical characterization of airborne bacteria using X-ray photoelectron spectroscopy (XPS) and Fourier transform infrared spectroscopy (FTIRS). Aerobiologia, 21, 181–192.
Petibois, C., Gionnet, K., Goncalves, M., Perromat, A., Moenner, M., & Déléris, G. (2006). Analytical performances of FT-IR spectrometry and imaging for concentration measurements within biological fluids, cells, and tissues. Analyst, 131, 640–647.
Riera, M. D., Cerdà, M. T., & Martin, J. (2002). A correlation study between airborne pollen and cases of pollinosis in humans. Aerobiologia, 18, 169–173.
Sengupta, A., Laucks, M. L., & James, D. E. (2005). Surface-enhanced Raman spectroscopy of bacteria and pollen. Applied Spectroscopy, 59, 1016–1023.
Verini, M., Rossi, N., Verrotti, A., Pelaccia, G., Nicodemo, A., & Chiarelli, F. (2001). Sensitization to environmental antigens in asthmatic children from a central Italian area. The Science of the Total Environment, 270, 63–69.
Verlato, G., Corsico, A., Villani, S., Cerveri, I., Migliore, E., Accordini, S., Carolei, A., Piccioni, P., Bugiani, M., Cascio, V., Marinoni, A., Poli, A., & De Marco, R. (2003). Is the prevalence of adult asthma and allergic rhinitis still increasing? Results of an Italian study. The Journal of Allergy and Clinical Immunology, 111, 1232–1238.
Author information
Authors and Affiliations
Corresponding author
Rights and permissions
About this article
Cite this article
Gottardini, E., Rossi, S., Cristofolini, F. et al. Use of Fourier transform infrared (FT-IR) spectroscopy as a tool for pollen identification. Aerobiologia 23, 211–219 (2007). https://doi.org/10.1007/s10453-007-9065-z
Received:
Accepted:
Published:
Issue Date:
DOI: https://doi.org/10.1007/s10453-007-9065-z