Abstract
Glyphosate is the most applied herbicide for weed control in agriculture worldwide. Excessive application of glyphosate induces water pollution. The transfer of glyphosate to freshwater and groundwater is largely controlled by glyphosate sorption to soils and sediments. Sorption coefficients are therefore the most sensitive parameters in models used for risk assessment. However, the variations in glyphosate sorption among soils and sediments are poorly understood. Here we review glyphosate sorption parameters and their variation with selected soils and sediment. We use this knowledge to build pedotransfer functions that allow predicting sorption parameters, Kd, Kf and n, for a wide range of soils and sediments. We gathered glyphosate sorption parameters, 101 Kf, n and equivalent Kd, and associated soil properties. These data were then used to perform stepwise multiple regression analyses to build the pedotransfer functions. The linear (Kd) and Freundlich (Kf, n) pedotransfer functions were benchmarked against experimental data. We found the following major points: (1) Under current environmental conditions, sorption is best predicted by the Kd pedotransfer function. (2) The pedotransfer function is Kd = 7.20*CEC − 1.31*Clay + 24.82 (Kd in L kg−1, CEC in cmol kg−1 and clay in %). (3) Cation exchange capacity (CEC) and clay content are the main drivers of Kd variability across soils and sediments. Freundlich parameters are additionally influenced by pH and organic carbon. This suggests that the formation of complexes between glyphosate phosphonate groups and soil-exchanged polyvalent cations dominates sorption across the range of analyzed soils.
Similar content being viewed by others
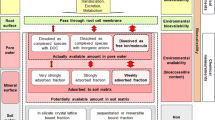
Explore related subjects
Discover the latest articles, news and stories from top researchers in related subjects.Avoid common mistakes on your manuscript.
Introduction
First introduced on the market in 1974, glyphosate [N-(phosphomethyl)glycine] rapidly became one of the most widely used broad-spectrum herbicides (Candela et al. 2007; Laitinen et al. 2008; Mazzei and Piccolo 2012; Székacs and Darvas 2012; Vereecken 2005). Since the 1990s, glyphosate has been the most heavily used pesticide worldwide for weed control in agriculture, forestry and urban landscapes, with postemergent applications (Candela et al. 2007; Kogan et al. 2003; Ololade et al. 2014; Rampoldi et al. 2011; Székacs and Darvas 2012). Despite glyphosate’s strong tendency to sorb to soils, its intensive use may be associated with a risk of freshwater and groundwater contamination. In fact, glyphosate and its main degradation product, aminomethyl phosphonic acid (AMPA), have been detected globally in freshwater ecosystems, wetlands and groundwater (Vereecken 2005; Villeneuve et al. 2011). These findings underline the need for the development of tools that enable the detection of zones with a risk of glyphosate dispersion.
The sorption of a given pesticide to soils and sediments after spraying and during its dispersion through landscapes largely controls its fate in the environment (Borggaard and Gimsing 2008; Gevao et al. 2000; Mamy and Barriuso 2005; Vereecken 2005). For example, sorption influences both the immobilization and the microbial degradation of a pesticide. This is demonstrated by the fact that strongly sorbing pesticides exhibit a lower availability for microbial degradation (Aparicio et al. 2013; Eberbach 1999; Strange-Hansen et al. 2004; Székacs and Darvas 2012; Vereecken 2005). Sorption parameters are therefore the main parameters used for risk assessment by regulatory agencies (Wauchope et al. 2002). They are also the most sensitive parameters in models used to predict pesticide dispersion in the soil (Wauchope et al. 2002). Sorption to soil is controlled by the chemical and physical properties of the pesticide and of the soil (Aparicio et al. 2013; Gevao et al. 2000). Accurate predictions of sorption coefficients for a given combination of pesticides, and soils require an understanding of the underlying mechanisms and associated soil properties involved in sorption (Ololade et al. 2014).
Glyphosate has three polar functional groups (amine, carboxylate and phosphonate) that can bind to metal polyvalent cations, placing it in the rarely represented class of chelating herbicides (Borggaard and Gimsing 2008; de Jonge and Wollesen de Jonge 1999; Morillo et al. 1997). Several mechanisms have been proposed to explain the sorption of glyphosate to soils and sediments. The formation of strong Fe–O–P(glyphosate) and Al–O–P(glyphosate) bonds by ligand exchange between the glyphosate phosphonate group and singly coordinated Al–OH and Fe–OH groups on the surfaces of variably charged soil minerals has been proposed as a possible sorption mechanism (Borggaard and Gimsing 2008; de Jonge et al. 2001; Dideriksen and Stipp 2003; Mamy and Barriuso 2005; Morillo et al. 1997; Nicholls and Evans 1991; Piccolo et al. 1994; Wang et al. 2006). This mechanism is deemed to occur preferentially on the broken edges of layer silicates, poorly ordered silicates or iron- and aluminum oxides (Borggaard and Gimsing 2008; Dideriksen and Stipp 2003; Laitinen et al. 2008; Morillo et al. 1997; Ololade et al. 2014; Piccolo et al. 1994). The formation of complexes between glyphosate phosphonate groups and the soil-exchanged polyvalent cations has also been suggested to be responsible for the strong sorption of glyphosate to soil (Borggaard and Gimsing 2008; de Jonge et al. 2001; de Jonge and Wollesen de Jonge 1999; Mamy and Barriuso 2005; Mcconnell and Hossner 1985; Vereecken 2005). The influence of the soil-exchanged cations on the sorption of glyphosate has been reported to follow the order of Na+<Mg2+<Ca2+<Zn2+<Mn2+<Fe3+<Al3+ (Borggaard and Gimsing 2008; de Jonge et al. 2001; Mcconnell and Hossner 1985). Furthermore, sorption to humic substances (HS) by hydrogen bonding at acidic or neutral pH or by formation of HS–Me–glyphosate complexes in which Me is a trivalent or divalent metal cation was reported to be a second-order sorption mechanism (Albers et al. 2009; Borggaard and Gimsing 2008; Mazzei and Piccolo 2012; Piccolo et al. 1992, 1996). However, due to its ability to block sorption sites, organic matter plays a dual role in glyphosate sorption (Borggaard and Gimsing 2008). Moreover, the soil pH may also be involved in sorption mechanisms. A rise in pH, leading to increased negatives charges of both soil and glyphosate may consequently result in greater electrostatic repulsion (Candela et al. 2007; de Jonge and Wollesen de Jonge 1999; Mcconnell and Hossner 1985; Morillo et al. 2000; Ololade et al. 2014; Wauchope et al. 2002). Finally, glyphosate and phosphate have been reported to exhibit similar sorption mechanisms, thereby leading to potential competition for sorption sites (Gimsing and Borggaard 2002). These sorption mechanisms are evidenced by the influence of clay content (Beltran et al. 1998; Borggaard and Gimsing 2008; da Cruz et al. 2007; Dion et al. 2001; Ololade et al. 2014; Pessagno et al. 2008; Singh et al. 2014; Xu et al. 2009), iron- and aluminum-oxide content (de Jonge et al. 2001; Gimsing et al. 2004, 2007; Mamy and Barriuso 2005; Morillo et al. 2000; Pessagno et al. 2008; Wang et al. 2005), pH (Accinelli et al. 2005; Al-Rajab et al. 2008; Borggaard and Gimsing 2008; da Cruz et al. 2007; de Jonge and Wollesen de Jonge 1999; Dousset et al. 2007; Gimsing et al. 2004; Mamy and Barriuso 2005; Xu et al. 2009; Zhou et al. 2004), phosphorus content (de Jonge et al. 2001; Gimsing and Borggaard 2002), organic carbon (Albers et al. 2009; da Cruz et al. 2007; Morillo et al. 2000; Ololade et al. 2014; Pessagno et al. 2008; Wang et al. 2005) and the cation exchange capacity (CEC) (da Cruz et al. 2007; de Jonge and Wollesen de Jonge 1999; Mamy and Barriuso 2005; Morillo et al. 1997; Wang et al. 2005; Xu et al. 2009) on the glyphosate sorption coefficients. Contrasting influences of these soil properties were reported among the studies, especially for organic carbon, reflecting variation in ranking among the aforementioned processes for various soil types.
The organic carbon sorption coefficient Koc that is generally used to normalize the sorption capacity of a given pesticide among various soils is not relevant for glyphosate. In fact, for this herbicide, the variability of Koc is greater than that of the linear sorption coefficient Kd (Autio et al. 2004; Mamy and Barriuso 2005). Despite the large number of studies reporting the sorption of glyphosate in different soil types, to our knowledge, no robust relationship has yet been developed between the glyphosate sorption coefficients and the aforementioned soil properties. For example, although Weber et al. (2004) proposed pedotransfer functions for predicting the Kd values of 57 pesticides, they did not include glyphosate in their study.
Accordingly, the objectives of this study were (i) to gather glyphosate sorption parameters and associated soil properties in a database, (ii) to investigate the soil properties responsible for the variation in sorption parameters among the soils and (iii) to build pedotransfer functions for the estimation of linear (Kd) and nonlinear (Kf, n) sorption parameters from the currently available soil properties.
Experimental
Physical and chemical properties of glyphosate
Glyphosate [N-(phosphomethyl)glycine] is a weak acid with strong hydrophilicity and very high water solubility (Table 1). Speciation of this zwitterionic molecule varies with the pH of the surrounding environment (Fig. 1). The main species within the soil pH range are GH2 − and GH2−, corresponding to net negative charges of one and two, respectively (Fig. 1) (Borggaard and Gimsing 2008).
Data mining
We extensively reviewed the literature to assemble a database of observed glyphosate sorption coefficients to both soils and sediments and the associated substrate properties (Table 2). We found 23 studies reporting sorption parameters for one or more soils or sediments. The soils or sediments for which glyphosate sorption measurements were carried out originated from four continents (Europe, Asia and North and South America) and exhibited highly varied texture and properties. The experimental conditions varied greatly as well. For example, the initial glyphosate concentrations in the liquid phase of the batch sorption experiments were distributed over a range of several orders of magnitude, from 0.01 to more than 1000 mg L−1.
Only coefficients of sorption to unmodified soils or sediments were included in the database. Indeed, a large body of the literature is based on either the addition or the removal of specific components of soils (e.g., organic matter) to evaluate the role of these components in the sorption mechanisms. Measured coefficients of sorption to organic soils were included in the database, but only those measured for sorption to mineral soils, i.e., with an organic matter content lower than 20 % (IUSS 2014) were used for the statistical analyses. Moreover, several studies have reported that sorption coefficients depend strongly on the background electrolyte (da Cruz et al. 2007; de Jonge et al. 2001; de Jonge and Wollesen de Jonge 1999; Mamy and Barriuso 2005). Therefore, only sorption coefficients obtained with classical background electrolyte, either Milli-Q water or CaCl2, were included in the database. Among the 101 sorption parameters registered in the database (Table 2), 69 were measured with CaCl2 as the background electrolyte. For a given soil, the use of CaCl2 as the background electrolyte, as recommended in the standardized method (OECD 2000), has been reported to increase glyphosate sorption compared to the use of Milli-Q water (de Jonge et al. 2001; de Jonge and Wollesen de Jonge 1999). To filter this potential bias, statistical analyses were only performed for the largest sample, i.e., sorption parameters measured with CaCl2 as the background electrolyte. This sample is designated as “sample A” in the following sections.
Sorption isotherms
Glyphosate sorption is usually described by either linear or Freundlich models (Eqs. 1 and 2). Freundlich models can be approximated by linear models for n values close to 1 (Wauchope et al. 2002). Here, we assumed linearity for Freundlich models with 0.98 < n < 1.02. The concentration dependence of the sorption processes is indicated by n values lower than 0.98 or higher than 1.02. Low n values (n < 0.98) indicate the saturation of sorption sites at high concentrations, whereas high n values (n > 1.02) suggest that the previously sorbed glyphosate increases the sorption power of the substrate (Wauchope et al. 2002).
where C s is the concentration of glyphosate in the soil (mg kg−1), C w is the concentration of glyphosate in the aqueous phase (mg L−1), C ini is the initial concentration of glyphosate in the aqueous phase (mg L−1) before equilibration with the solid phase, R is the solid/liquid ratio (g mL−1), Kd (L kg−1) is the linear sorption coefficient, Kf (L kg−1 n−1) is the nonlinear sorption coefficient and n is the nonlinearity parameter.
For sample A, approximately two-thirds of the sorption models were nonlinear Freundlich S-type, as shown by n values lower than 0.98 (Table 3). To establish a pedotransfer function for Kd, we approximated equivalent Kd values by linearizing the Freundlich models. In practice, most papers in the literature provided the fitted sorption model parameters but did not provide the basic batch data that were used to fit the models. Linearization of each Freundlich model was performed over the actual range of the initial aqueous concentrations of the batch experiment used for model fitting (Table 3). For this purpose, a set of initial concentrations distributed over the range of the actual batch concentrations was chosen among those presented in Table 4. For all the concentrations in the set, the solid and aqueous concentrations at equilibrium, namely C w and C s, were calculated by Eqs. 2 and 3 using a numerical solver (Microsoft Office 2010). The numerical solver adjusted C w and C s to minimize the difference between C ini resulting from Eqs. 2 and 3 and the target C ini (Table 4). Then, a linear model (Eq. 1) was fitted to the predicted C w/C s pairs corresponding to the selected set of initial concentrations. The relative difference between Kf and its equivalent, Kd (Kdeq), was approximately 30 % on average (data not shown).
Statistical analyses
Pedotransfer functions aim to predict the sorption parameters Kd, Kf and n from selected substrate properties. Some of the properties, especially CEC, iron- and aluminum oxides or phosphorus content, were not available for all soils or sediments (Table 2). This lack of data induced a subsampling of sample A for the establishment of pedotransfer functions for the Kd and Kf parameters. This sample is designated as “sample B” in the following sections and in Table 3. The sample used for the establishment of the pedotransfer function for the n parameter excluded sorption studies that investigated only one concentration and, thereby, did not consider the possibility that n differs from 1. This sample is designated as “sample C” in the following sections and in Table 3.
The statistical analyses were performed using version 3.1.0 of the R statistical computing software (Cran R organization 2015). Correlation analyses were performed using the default “lm” function of the R software. These analyses aimed at exploring the factors that potentially explain the variations in Kdeq, Kf and n across the range of investigated soils. Correlation analyses were also performed to detect covariation in the soil properties.
The three pedotransfer functions for the estimation of linear and nonlinear sorption models were established by forward and backward stepwise multiple regression analyses of the substrate properties and the Kdeq, Kf and n parameters. The stepwise multiple regression analyses were performed using the default “step” function of the R software. The root-mean-square error of prediction (RMSEP) of the regression pedotransfer functions was evaluated by leave-one-out cross-validation with the function cvFit of the cvTool package of R software version 3.1.0.
The validity of the Kd pedotransfer function is strongly supported by the fact that sorption processes do not depend on the pesticide concentration. However, in sample A, the n values ranged from 0.48 to 1.05, with a mean value of 0.83, indicating saturation of the sorption sites at high glyphosate concentrations. Such saturation may be induced by the experimental conditions, such as the solid-to-liquid ratio and the initial concentration range, or by the limited amount of sorption sites in the soil (Vereecken 2005; Wauchope et al. 2002). A complementary multiple regression between n, the substrate properties and the experimental conditions (C max and R) was therefore performed. The resulting equation (see Eq. 4 in the Results and Discussion section) indicates the linearity range under various conditions.
Finally, we evaluated the accuracy of the predicted equilibrium partitioning of glyphosate between the soil and water by using the sorption parameters provided by the Kd or Kf/n pedotransfer functions. The evaluation was performed for 11 initial concentrations (0.01, 0.04, 0.10, 0.40, 1, 4, 10, 40, 100, 400 and 1000 mg L−1) in the liquid phase by comparing the predicted soil-to-water glyphosate concentration ratios, as obtained by the pedotransfer-estimated sorption parameters, to those obtained by the batch-fitted sorption parameters. The aqueous and soil concentrations were calculated for all sample B soils and sediments using the numerical solver as described previously.
Results and discussion
Database and sample characteristics
The soils and sediments used in the glyphosate sorption measurements displayed great variability in their origins and properties. This variability was preserved in the subsampling of the database for pedotransfer function calibration, as seen in Table 3. Indeed, the three subsamples of the database displayed similar distributions of properties and parameters values. The 0.01–1000 mg L−1 concentration range (Table 4) was also preserved by the subsampling of the database. This range covers all possible environmental glyphosate concentrations from concentrations found during spraying to those found in runoff and groundwater (Székacs and Darvas 2012; Vereecken 2005; Villeneuve et al. 2011). It is interesting to note that the data presented in Table 5 exhibited highly significant correlations between some basic soil properties: The CEC was correlated with organic carbon or iron- and aluminum oxides, and the clay content was correlated with iron- and aluminum oxides. In contrast, there was no correlation between clay and CEC, suggesting a large influence of the within-sample variation in clay mineralogy (Table 5).
Glyphosate sorption: mechanisms and prediction
The Pearson correlation coefficients (Table 6) showed that the Kdeq and Kf values are primarily correlated with CEC and, secondarily, with organic carbon content and Feox–Alox content. They also show that n exhibits significant correlation with all of the selected soil properties, with the exception of CEC.
The multiple regression analysis (Table 7) provided pedotransfer functions that accurately fit the observed Kdeq, Kf and n values. The functions account for 48–62 % of the variation in the sorption parameters. Visual inspection of the disparity between the measured and predicted Kdeq, Kf and n sorption parameters did not reveal systematic departures from the regression, except for one outlier corresponding to high Kdeq and Kf values measured on a sediment containing a particularly high organic carbon content (Fig. 2). The multiple regression analyses highlighted the points that CEC is the main predictor of Kdeq and Kf variation and that clay is a useful predictor. Furthermore, we found that organic carbon was a predictor for Kf only. The analyses also revealed that clay and pH are significant predictors of n.
Multiple regression analysis of the sorption coefficients (Kd, Kf, n) and soil properties. The sorption coefficients predicted from the pedotransfer functions presented in Table 7 were plotted against the sorption coefficients (Kf, n) fitted from the experimental data and for Kdeq, against the linearized sorption coefficients
These results suggest that the formation of complexes between the glyphosate phosphonate groups and the soil-exchanged polyvalent cations is the dominating sorption mechanism across the entire range of analyzed soils. This is indicated by the primary role of CEC in controlling Kdeq and Kf variability. Indeed, several studies have highlighted the role of CEC in the strong sorption of glyphosate to soils (da Cruz et al. 2007; Mcconnell and Hossner 1985; Morillo et al. 1997; Xu et al. 2009), although others concluded that clay and Feox–Alox play an even more important role (Bergström et al. 2011; Rampazzo et al. 2013; Wang et al. 2005). Given the high correlation between CEC and Feox–Alox in our sample, it is likely that the influence of the latter property was masked by that of the former. Additionally, we found that clay content explained only approximately 5 % of the Kdeq and Kf variability (Tables 6, 7). This corroborates the hypothesis that for the sorption rate, the clay mineralogy and its associated properties, such as the specific surface area, saturating cation or isoelectric point, may be more important than the clay content (Borggaard and Gimsing 2008; da Cruz et al. 2007; Kogan et al. 2003; Mcconnell and Hossner 1985; Pessagno et al. 2008). Significant correlations were found between organic carbon and Kdeq or Kf (Table 6), although organic carbon only slightly increased the R 2 value obtained in the multiple regression analyses of Kf. Organic carbon appeared to be strongly correlated with CEC, indicating the significant contribution of organic matter to CEC; this correlation may explain the correlation of organic carbon with the sorption parameters. This finding is in agreement with the work of Yu and Zhou (2005), who found that the removal of organic matter drastically diminished the CEC of the soils and, consequently, sharply decreased the sorption coefficients. There is a general consensus that a rise in pH negatively affects the sorption of glyphosate (Accinelli et al. 2005; Al-Rajab et al. 2008; Borggaard and Gimsing 2008; de Jonge and Wollesen de Jonge 1999; Dousset et al. 2007; Gimsing et al. 2004; Mamy and Barriuso 2005; Mcconnell and Hossner 1985; Xu et al. 2009). However, the multiple regression analyses did not detect any influence of pH on Kdeq and Kf variability.
Here, pH and clay explained most of the n parameter variability (Tables 6 and 7). The positive correlation of n with pH may be related to the increased negative charges for both glyphosate (Fig. 1) and the soil, favoring the formation of complexes with soil-exchanged polyvalent cations. Despite the increasing electrostatic repulsion, a rise in pH appears to reduce the potential saturation of sorption sites for high initial concentrations by favoring cation bridging between glyphosate and the soil.
The variability of the sorption parameters that is not predicted by the multiple regressions may be largely attributed to the varying experimental conditions among the studies measuring glyphosate sorption to soils and sediments (Table 2). One source of experimental variation is the differences in the methods used to measure the soil properties, especially the pH, Feox–Alox content or CEC. Another source is the variations in the maximal initial concentration (C max) and in the soil-to-solution ratio (R) (Table 6). It is striking that Kf and n are correlated with these variables. Moreover, if these parameters are considered to be possible predictors in the multiple regressions, they enable a fit to a regression function (Eq. 4) with a better performance (R 2 = 0.69) than that of the regression using only basic soil properties as predictors for n.
A small R implies a limited amount of sorption sites. Combined with high concentrations, this feature leads to a rapid saturation of the sorption sites with increasing glyphosate concentrations (Al-Rajab et al. 2008; Litz et al. 2011; Wauchope et al. 2002). In addition to affecting the linearity of the processes, the experimental conditions also affect the adsorption rates (Wauchope et al. 2002). Wauchope et al. (2002) found that changing R from 1:1 to 1:33 caused a sorption decrease of 50 %. Correlations between Kf, Kdeq and the solid-to-liquid ratio or the maximal initial concentration (Table 6) are further evidence of the influence of the experimental conditions on the sorption. However, unlike the case of the n parameter, inclusion of the experimental conditions (C max, R) in the multiple regression analyses did not increase the predictive performance of the regression for Kdeq and Kf. The experimental conditions are usually determined based on analytical constraints and rarely reflect the actual environmental conditions. It must be noted that the pedotransfer functions could be improved with additional experimental sorption studies designed to closely mimic the environmental conditions and with pH and CEC analyzed with the standardized methods [pHH2O, Metson CEC (cmol kg−1)].
Use of pedotransfer functions for risk assessment
The linear sorption coefficient Kd can be predicted by a pedotransfer function requiring the knowledge of only two properties, the clay content and CEC. The prediction performance is good with an RMSEP of less than 10 % of the mean glyphosate Kdeq (Table 7). However, Fig. 3a shows that the errors in the predicted soil-to-water concentration ratios vary largely according to the initial concentration of water. The errors are moderate for initial liquid-phase concentrations below 10 mg L−1, indicating that the Kd pedotransfer function predicts sorption relatively accurately for concentrations below this threshold. The 10 mg L−1 may correspond to the threshold above which the concentration independence of the sorption process can no longer be assumed. This assumption can be checked by examining the variation in n given by Eq. 4. Figure 4 presents the departure from linearity assumed to occur when n is below 0.9 across a range of clay content values and initial glyphosate concentrations. Soil-to-solution ratios of 1:1 and 1:20 were chosen to represent structured soils (Wauchope et al. 2002) and sediment–water systems, respectively. For the 1:20 soil-to-solution ratio, which exhibits the lowest R among the experimental conditions of the results included in the database, the linearity is respected under 10 mg L−1 for clay contents higher than 10 %; this is the case for more than 50 % of the soils or sediments of sample A. For concentrations above 10 mg L−1, the sorption isotherms are linear only for soils or sediments with clay content higher than 20 %; this is the case for only approximately 25 % of the soils or sediments of sample A.
Distributions of the prediction errors for linear sorption isotherms and nonlinear sorption isotherms. Δ represents the difference (%) at a given initial concentration between the predicted and the measured ratio of concentrations between soil and water. a Ratios predicted by the Kd pedotransfer function (linear isotherm estimation) and b ratios predicted by the Kf and n pedotransfer function (nonlinear isotherm estimation)
Linearity range of sorption isotherms in relation to the clay content and initial glyphosate concentrations in the liquid phase. Plain green dots represent 0.9 < n < 1.05, and empty orange dots represent n values lower than 0.9 (bottom right). a n values were calculated from Eq. 4 with a solid-to-liquid ratio of 1:1 (g/ml). b n values were calculated from Eq. 4 with a solid-to-liquid ratio of 1:20 (g/ml). Note that for a solid-to-liquid ratio of 1:1, the saturation of sorption sites occurs at initial concentration higher than 100 mg/l for clay content varying between 0 and 10 %, whereas for the 1:20 ratio, the saturation for the same clay content starts at initial concentrations of approximately 0.1 mg/l. (Color figure online)
The Freundlich isotherms can be satisfactorily predicted by two pedotransfer functions requiring the knowledge of four properties, namely the organic carbon and clay contents, CEC and pH (Table 7). The RMSEP of predicted Kf values is greater than that for predicted Kd because it is approximately 16 % of the mean Kf; the RMSEP of n is smaller than 1 % of the mean n, demonstrating the high accuracy of the predicted n. As seen in Fig. 4, the prediction errors of the soil-to-water concentration ratio exceed 1000 % for concentrations between 0.01 and 0.40 mg L−1 and 500 % for concentrations up to 10 mg L−1 (Fig. 3b). Thus, the sorption estimated by the combination of n and Kf pedotransfer functions is significantly underestimated for initial concentrations below 10 mg L−1. This may be due to the multiplication of properties used to estimate the sorption parameters and the accumulation of inherent bias of the two pedotransfer functions. However, it must also be noted that for concentrations higher than 10 mg L−1, the predictions using the estimated Freundlich model parameters show slightly smaller errors than those using the estimated linear isotherms. The application of the Kf pedotransfer function is therefore only advisable for estimating sorption for very high liquid-phase concentrations, a condition that is relatively rarely found in the current environmental conditions.
Conclusion
Sorption to soils and sediments controls the fate of glyphosate in the environment and thus the potential risk of freshwater and groundwater contamination. Glyphosate sorption appeared to be controlled mainly by cation exchange capacity, clay and organic carbon content and pH. This suggests that the mechanism driving glyphosate sorption over the range of soil and sediment investigated is the complex formation between the phosphonate group of glyphosate and the soil-exchanged polyvalent cations. Robust pedotransfer function for the estimation of glyphosate Kd was built from multiple regression analysis of the literature data. This Kd pedotransfer function enables prediction of glyphosate sorption for a wide range of soils and sediments with a limited number of properties and with reasonable accuracy for most environmental conditions. This method provides an efficient tool to parameterize models for the evaluation of glyphosate dispersion within a watershed (FOCUS 2007). The predicted Kd values may also be useful to calibrate simplified risk assessment methods, such as pesticide leaching indices (e.g., the GUS index) (Funari and Vighi 1995) or surface retention indices (e.g., the PRP index) (Lagacherie et al. 2006; Margoum et al. 2006).
References
Accinelli C, Koskinen WC, Seebinger JD, Vicari A, Sadowsky MJ (2005) Effects of incorporated corn residues on glyphosate mineralization and sorption in soil. J Agric Food Chem 53:4110–4117. doi:10.1021/jf050186r
Albers CN, Banta GT, Hansen PE, Jacobsen OS (2009) The influence of organic matter on sorption and fate of glyphosate in soil—comparing different soils and humic substances. Environ Pollut 157:2865–2870. doi:10.1016/j.envpol.2009.04.004
Al-Rajab AJ, Amellal S, Schiavon M (2008) Sorption and leaching of 14C-glyphosate in agricultural soils. Agron Sustain Dev 28:419–428. doi:10.1051/agro:2008014
ANSES (2015) Agritox database. http://www.agritox.anses.fr/
Aparicio VC, De Gerónimo E, Marino D, Primost J, Carriquiriborde P, Costa JL (2013) Environmental fate of glyphosate and aminomethylphosphonic acid in surface waters and soil of agricultural basins. Chemosphere 93:1866–1873. doi:10.1016/j.chemosphere.2013.06.041
Autio S, Siimes K, Laitinen P, Rämö S, Oinonen S, Eronen L (2004) Adsorption of sugar beet herbicides to finnish soils. Chemosphere 55:215–226. doi:10.1016/j.chemosphere.2003.10.015
Bailly JS, Dages C, Dollinger J, Lagacherie P, Voltz M (2015) Protocole de spatialisation et d’évolution d’états de surface de fossés. French Government Water and Aquatic Bodies Office, 60 p
Beltran J, Gerritse RG, Hernandez F (1998) Effect of flow rate on the adsorption and desorption of glyphosate, simazine and atrazine in columns of sandy soils. Eur J Soil Sci 49:149–156. doi:10.1046/j.1365-2389.1998.00132.x
Bergström L, Börjesson E, Stenström J (2011) Laboratory and lysimeter studies of glyphosate and aminomethylphosphonic acid in a sand and a clay soil. J Environ Qual 40:98. doi:10.2134/jeq2010.0179
Borggaard OK (2011) Does phosphate affect soil sorption and degradation of glyphosate? A Review. Trends Soil Sci Plant Nutr 2:17–27
Borggaard OK, Gimsing AL (2008) Fate of glyphosate in soil and the possibility of leaching to ground and surface waters: a review. Pest Manag Sci 64:441–456. doi:10.1002/ps.1512
Candela L, Álvarez-Benedí J, Condesso de Melo MT, Rao PSC (2007) Laboratory studies on glyphosate transport in soils of the Maresme area near Barcelona, Spain: transport model parameter estimation. Geoderma 140:8–16. doi:10.1016/j.geoderma.2007.02.013
Cheah U-B, Kirkwood RC, Lum K-Y (1997) Adsorption, desorption and mobility of four commonly used pesticides in malaysian agricultural soils. Pestic Sci 50:53–63. doi:10.1002/(SICI)1096-9063(199705)50:1<53:AID-PS558>3.0.CO;2-P
Cran R organization (2015) R. http://cran.r-project.org/
da Cruz LH, de Santana H, Zaia CTBV, Zaia DAM (2007) Adsorption of glyphosate on clays and soils from Paraná State: effect of pH and competitive adsorption of phosphate. Braz Arch Biol Technol 50:385–394. doi:10.1590/S1516-89132007000300004
De Jonge H, Wollesen de Jonge L (1999) Influence of pH and solution composition on the sorption of glyphosate and prochloraz to a sandy loam soil. Chemosphere 39:753–763. doi:10.1016/S0045-6535(99)00011-9
De Jonge H, de Jonge LW, Jacobsen OH, Yamaguchi T, Moldrup P (2001) Glyphosate sorption in soils of different pH and phosphorus content. Soil Sci 166:230–238. doi:10.1097/00010694-200104000-00002
Dideriksen K, Stipp SLS (2003) The adsorption of glyphosate and phosphate to goethite: a molecular-scale atomic force microscopy study. Geochim Cosmochim Acta 67:3313–3327. doi:10.1016/S0016-7037(02)01369-8
Dion HM, Harsh JB, Hill HHJ (2001) Competitive sorption between glyphosate and inorganic phosphate on clay minerals and low organic matter soils. J Radioanal Nucl Chem 249:385–390. doi:10.1023/A:1013222704311
Dousset S, Jacobson AR, Dessogne J-B, Guichard N, Baveye PC, Andreux F (2007) Facilitated transport of diuron and glyphosate in high copper vineyard soils. Environ Sci Technol 41:8056–8061. doi:10.1021/es071664c
Eberbach PL (1999) Influence of incubation temperature on the behavior of triethylamine-extractable glyphosate (N-phosphonomethylglycine) in four soils. J Agric Food Chem 47:2459–2467. doi:10.1021/jf980785g
FOCUS (2007) Landscape and mitigation factors in aquatic risk assessment. Volume 2: detailed technical reviews. European Commission
FOOTPRINT (2015) Footprint PPDB database. http://sitem.herts.ac.uk/aeru/ppdb/en/
Funari E, Vighi M (1995) Pesticide risk in groundwater. CRC Press, New York
Gevao B, Semple KT, Jones KC (2000) Bound pesticide residues in soils: a review. Environ Pollut 108:3–14. doi:10.1016/S0269-7491(99)00197-9
Gimsing AL, Borggaard OK (2002) Competitive adsorption and desorption of glyphosate and phosphate on clay silicates and oxides. Clay Miner 37:509–515. doi:10.1180/0009855023730049
Gimsing AL, Borggaard OK, Bang M (2004) Influence of soil composition on adsorption of glyphosate and phosphate by contrasting Danish surface soils. Eur J Soil Sci 55:183–191. doi:10.1046/j.1365-2389.2003.00585.x
Gimsing AL, Szilas C, Borggaard OK (2007) Sorption of glyphosate and phosphate by variable-charge tropical soils from Tanzania. Geoderma 138:127–132. doi:10.1016/j.geoderma.2006.11.001
IUSS W (2014) World reference base for soil resources 2014 (no. 106), World soil resources reports
Jacobsen CS, van der Keur P, Iversen BV, Rosenberg P, Barlebo HC, Torp S, Vosgerau H, Juhler RK, Ernstsen V, Rasmussen J, Brinch UC, Jacobsen OH (2008) Variation of MCPA, metribuzine, methyltriazine-amine and glyphosate degradation, sorption, mineralization and leaching in different soil horizons. Environ Pollut 156:794–802. doi:10.1016/j.envpol.2008.06.002
Kogan M, Metz A, Ortega R (2003) Adsorption of glyphosate in chilean soils and its relationship with unoccupied phosphate binding sites. Pesqui Agropecuária Bras 38:513–519. doi:10.1590/S0100-204X2003000400010
Lagacherie P, Diot O, Domange N, Gouy V, Floure C, Kao C, Moussa R, Robbez-Masson JM, Szleper V (2006) An indicator approach for describing the spatial variability of artificial stream networks with regard to herbicide pollution in cultivated watersheds. Ecol Indic 6:265–279. doi:10.1016/j.ecolind.2005.02.003
Laitinen P, Siimes K, Rämö S, Jauhiainen L, Eronen L, Oinonen S, Hartikainen H (2008) Effects of soil phosphorus status on environmental risk assessment of glyphosate and glufosinate-ammonium. J Environ Qual 37:830. doi:10.2134/jeq2007.0256
Litz NT, Weigert A, Krause B, Heise S, Grützmacher G (2011) Comparative studies on the retardation and reduction of glyphosate during subsurface passage. Water Res 45:3047–3054. doi:10.1016/j.watres.2011.02.015
Mamy L, Barriuso E (2005) Glyphosate adsorption in soils compared to herbicides replaced with the introduction of glyphosate resistant crops. Chemosphere 61:844–855. doi:10.1016/j.chemosphere.2005.04.051
Mamy L, Barriuso E (2007) Desorption and time-dependent sorption of herbicides in soils. Eur J Soil Sci 58:174–187. doi:10.1111/j.1365-2389.2006.00822.x
Maqueda C, Morillo E, Undabeytia T, Martín F (1998) Sorption of glyphosate and Cu(II) on a natural fulvic aced complex: mutual influence. Chemosphere 37:1063–1072. doi:10.1016/S0045-6535(98)00103-9
Margoum C, Malessard C, Gouy V (2006) Investigation of various physicochemical and environmental parameter influence on pesticide sorption to ditch bed substratum by means of experimental design. Chemosphere 63:1835–1841. doi:10.1016/j.chemosphere.2005.10.032
Mazzei P, Piccolo A (2012) Quantitative evaluation of noncovalent interactions between glyphosate and dissolved humic substances by NMR spectroscopy. Environ Sci Technol 46:5939–5946. doi:10.1021/es300265a
Mcconnell J, Hossner L (1985) Ph-dependent adsorption-isotherms of glyphosate. J Agric Food Chem 33:1075–1078. doi:10.1021/jf00066a014
Microsoft Office (2010) Excell 2010
Morillo E, Undabeytia T, Maqueda C (1997) Adsorption of glyphosate on the clay mineral montmorillonite: effect of Cu(II) in solution and adsorbed on the mineral. Environ Sci Technol 31:3588–3592. doi:10.1021/es970341l
Morillo E, Undabeytia T, Maqueda C, Ramos A (2000) Glyphosate adsorption on soils of different characteristics: influence of copper addition. Chemosphere 40:103–107. doi:10.1016/S0045-6535(99)00255-6
Nicholls PH, Evans AA (1991) Sorption of ionisable organic compounds by field soils. Part 2: cations, bases and zwitterions. Pestic Sci 33:331–345. doi:10.1002/ps.2780330307
OECD (2000) OECD guidelines for testing of chemicals
Ololade IA, Oladoja NA, Oloye FF, Alomaja F, Akerele DD, Iwaye J, Aikpokpodion P (2014) Sorption of glyphosate on soil components: the roles of metal oxides and organic materials. Soil Sediment Contam 23:571–585. doi:10.1080/15320383.2014.846900
Pessagno RC, Torres Sánchez RM, dos Santos Afonso M (2008) Glyphosate behavior at soil and mineral–water interfaces. Environ Pollut 153:53–59. doi:10.1016/j.envpol.2007.12.025
Piccolo A, Celano G, Pietramellara G (1992) Adsorption of the herbicide glyphosate on a metal-humic acid complex. Sci Total Environ Behav Pestic Soil Environ 123–124:77–82. doi:10.1016/0048-9697(92)90134-E
Piccolo A, Celano G, Arienzo M, Mirabella A (1994) Adsorption and desorption of glyphosate in some European soils. J Environ Sci Health B 29:1105–1115. doi:10.1080/03601239409372918
Piccolo A, Celano G, Conte P (1996) Adsorption of glyphosate by humic substances. J Agric Food Chem 44:2442–2446. doi:10.1021/jf950620x
Prata F, Cardinali VC, do B, Lavorenti A, Tornisielo VL, Regitano JB (2003) Glyphosate sorption and desorption in soils with distinct phosphorus levels. Sci Agric 60:175–180. doi:10.1590/S0103-90162003000100026
Rampazzo N, Rampazzo Todorovic G, Mentler A, Blum WEH (2013) Adsorption of glyphosate and aminomethylphosphonic acid in soils. Int Agrophys 27:203–209
Rampoldi EA, Hang S, Barriuso E (2011) The fate of glyphosate in crop residues. Soil Sci Soc Am J 75:553. doi:10.2136/sssaj2010.0105
Singh B, Farenhorst A, Gaultier J, Pennock D, Degenhardt D, McQueen R (2014) Soil characteristics and herbicide sorption coefficients in 140 soil profiles of two irregular undulating to hummocky terrains of western Canada. Geoderma 232–234:107–116. doi:10.1016/j.geoderma.2014.05.003
Strange-Hansen R, Holm PE, Jacobsen OS, Jacobsen CS (2004) Sorption, mineralization and mobility of N-(phosphonomethyl)glycine (glyphosate) in five different types of gravel. Pest Manag Sci 60:570–578. doi:10.1002/ps.842
Székacs A, Darvas B (2012) Forty years with glyphosate. Hungarian Academy of Sciences, Hungary
Vereecken H (2005) Mobility and leaching of glyphosate: a review. Pest Manag Sci 61:1139–1151. doi:10.1002/ps.1122
Villeneuve A, Larroudé S, Humbert J-F (2011) Herbicide contamination of freshwater ecosystems: impact on microbial communities. InTech open, pp 285–312
Wang Y, Zhou D, Sun R (2005) Effects of phosphate on the adsorption of glyphosate on three different types of Chinese soils. J Environ Sci 17:711–715
Wang Y-J, Zhou D-M, Sun R-J, Cang L, Hao X-Z (2006) Cosorption of zinc and glyphosate on two soils with different characteristics. J Hazard Mater 137:76–82. doi:10.1016/j.jhazmat.2006.02.032
Wauchope RD, Yeh S, Linders JBHJ, Kloskowski R, Tanaka K, Rubin B, Katayama A, Kördel W, Gerstl Z, Lane M, Unsworth JB (2002) Pesticide soil sorption parameters: theory, measurement, uses, limitations and reliability. Pest Manag Sci 58:419–445. doi:10.1002/ps.489
Weber JB, Wilkerson GG, Reinhardt CF (2004) Calculating pesticide sorption coefficients (Kd) using selected soil properties. Chemosphere 55:157–166. doi:10.1016/j.chemosphere.2003.10.049
Xu D, Meyer S, Gaultier J, Farenhorst A, Pennock D (2009) Land use and riparian effects on prairie wetland sediment properties and herbicide sorption coefficients. J Environ Qual 38:1757. doi:10.2134/jeq2008.0357
Yu Y, Zhou Q-X (2005) Adsorption characteristics of pesticides methamidophos and glyphosate by two soils. Chemosphere 58:811–816. doi:10.1016/j.chemosphere.2004.08.064
Zhou D-M, Wang Y-J, Cang L, Hao X-Z, Luo X-S (2004) Adsorption and cosorption of cadmium and glyphosate on two soils with different characteristics. Chemosphere 57:1237–1244. doi:10.1016/j.chemosphere.2004.08.043
Acknowledgments
This work was performed in the framework of a research and development project funded by the French Office for Water and Aquatic Bodies (ONEMA). The first author is grateful to the French National Institute for Agricultural Research (INRA) for providing her Ph.D. Grant.
Author information
Authors and Affiliations
Corresponding author
Rights and permissions
About this article
Cite this article
Dollinger, J., Dagès, C. & Voltz, M. Glyphosate sorption to soils and sediments predicted by pedotransfer functions. Environ Chem Lett 13, 293–307 (2015). https://doi.org/10.1007/s10311-015-0515-5
Received:
Accepted:
Published:
Issue Date:
DOI: https://doi.org/10.1007/s10311-015-0515-5