Abstract
Background
Health care expenditure (HCE) is not distributed evenly over a person’s life course. How much is spent on the elderly is important as they are a population group that is increasing in size. However other factors, such as death-related costs that are known to be high, need be considered as well in any expenditure projections and budget planning decisions.
Objective
This article analyses, for the first time in Scotland, how expenditure projections for acute inpatient care are influenced when applying two different analytical approaches: (1) accounting for healthcare (HC) spending at the end of life and (2) accounting for demographic changes only. The association between socioeconomic status and HC utilisation and costs at the end of life is also estimated.
Methods
A representative, longitudinal data set is used. Survival analysis is employed to allow inclusion of surviving sample members. Cost estimates are derived from a two-part regression model. Future population estimates were obtained for both methods and multiplied separately by cost estimates.
Results
Time to death (TTD), age at death and the interaction between these two have a significant effect on HC costs. As individuals approach death, those living in more deprived areas are less likely to be hospitalised than those individuals living in the more affluent areas, although this does not translate into incurring statistically significant higher costs. Projected HCE for acute inpatient care for the year 2028 was approximately 7 % higher under the demographic approach as compared to a TTD approach.
Conclusion
The analysis showed that if death is postponed into older ages, HCE (and HC budgets) would not increase to the same extent if these factors were ignored. Such factors would be ignored if the population that is in their last year(s) of life were not taken into consideration when obtaining cost estimates.
Similar content being viewed by others
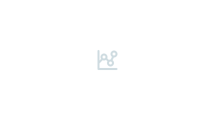
Explore related subjects
Discover the latest articles, news and stories from top researchers in related subjects.Avoid common mistakes on your manuscript.
Introduction
Population ageing is a major concern for developed countries and the expected increase in healthcare expenditure (HCE) is mainly based on the higher demand that is placed on healthcare (HC) services by older people. There is a growing body of literature that takes into account factors other than population ageing to explain and estimate future HC spending. Among the frequently researched factors is remaining time to death (TTD) [1–8]. As health deteriorates at the end of life patients might receive expensive and aggressive treatments in order to prolong life. This implies that older people are more expensive not because they are older but because they tend to be closer to death compared to younger people. If population ageing was the result of increased life expectancy, it would be expected that the age profiles of HCE move downwards for those age groups for which mortality is improving, i.e. HCE decreases for any given age. HC costs are not distributed evenly over an individual’s life and TTD research aims to attribute these costs correctly. In their seminal study that analysed data for individuals aged 65 and older, Zweifel et al. [2] found that the positive relationship between age and HCE can be completely attributed to the fact that mortality increases with increasing age. This conclusion is very strong and it has motivated a number of other national studies, some of which conclude that age is still an important factor associated with HCE, but accounting for TTD reduces the magnitude of the effect that age has on HC costs [4, 5, 7, 9].
A more recent study by Breyer et al. [6] examined whether population ageing alongside decreasing mortality rates at any given age will lead to increased HCE and results clearly showed that this is the case. The authors also raised the question of age-related rationing, where physicians will have to make decisions given scarce resources that might favour the younger age groups as they will have a greater opportunity to benefit from the treatment than older age groups [6]. Baal and Wong used macro-level data to forecast HCE and in contrast to other literature that used individual level data found that the inclusion of death-related costs does not alter HCE projections [10]. Moorin et al. [8] highlighted the importance of separating the different types of HC services when analysing the association between population ageing, HCE and TTD as they showed no association between changes in out-of-hospital services and the number of decedents. There is not unanimous agreement in the field and the use of various estimation techniques, cross-sectional versus longitudinal data and the focus on varying HC sectors or not separating between different HC sectors when analysing the association between population ageing and HCE make it difficult to draw final conclusions. It could be argued that there is a need for separate analyses for each country to account for different HC systems and population characteristics.
To date, there is no research in Scotland that quantifies the extent to which population ageing might have an impact on future HCE. This article therefore aims to provide further empirical evidence of the distribution of future HCEs under two different scenarios. It will quantify the extent to which projected costs might differ using (1) a ‘TTD approach’ that takes into account both age and proximity to death and (2) assuming constant age profiles for HCE over time, the ‘demographic approach’. This is particularly relevant for resource allocation and budget planning decisions, as these currently do not account for potentially lower costs for the oldest age groups at the end of life. In austere times it becomes increasingly important to make accurate projections for public expenditures.
A novel and large representative longitudinal sample of the Scottish population will be employed to estimate HCE under a TTD approach, which also allows for the inclusion of previously omitted variables, such as socioeconomic status and a baseline measure of self-reported health. Previous research seems to suggest that ‘the poor cost more’ [11]. However, potentially confounding this is that those with lower socioeconomic status are closer to death because of their shorter than average life expectancy. This is highly relevant particularly in Scotland, where there is a poor record of premature deaths in some areas with very high levels of deprivation [12]. No robust regression analysis has been undertaken to date that estimated the effect of socioeconomic status at the end of life. Preliminary, descriptive analysis from Scotland suggested a socioeconomic gradient in terms of costs incurred towards the end of life, with decedents from more deprived areas incurring lower costs in younger age groups with the effect reversing in the very old age groups over 75 [13]. The questions remain: is there evidence that individuals from more deprived areas cost more, and to what extent are costs influenced by HC utilisation?
The remainder of the article is structured as follows. A description of the data set is followed by a presentation of survival analysis and econometric regression methods that were employed in order to estimate HC utilisation and expenditure. Following this, the two approaches to project costs are presented. Results are presented in the “Results” section and the article concludes with a discussion of the main findings.
Methods
Data
The Scottish Longitudinal Study (SLS) is a truly anonymised data set of a representative sample of the Scottish population (5.3 %). It draws on data from a series of statistical and administrative sources and started with the 1991 census from which about 270,000 SLS members were identified. The novelty of the SLS is that it can be linked with data on hospital admissions [Scottish Morbidity Records 01 (SMR01Footnote 1)]. It also has very low attrition rates and very high rates for successful linkage of events [14–17]. Information on hospitalisations (and their associated costs) together with SLS data on socioeconomic status and demographic information provides a novel platform from which to analyse the cost of ageing and the cost of dying, which can be utilised for HCE projections.
Healthcare expenditure in the last 5 years (20 quartersFootnote 2) of life for individuals aged 45 and older is examined. This age limit has been applied because the ageing population is the focus of this research, although it is acknowledged that death occurs at younger ages. Importantly, the analysis also includes individuals without any recorded hospital episodes. These observations have been neglected in previous research [2, 13]. Quarters in which no hospitalisation was observed enter the model as zero cost observations.
While TTD for those SLS members who have died is known, the inclusion of surviving sample members requires the use of survival analysis to predict TTD. The costing method that is applied utilises Healthcare Resource Groups (HRGs) as the basis on which unit costs are assigned to a Continuous Inpatient Stay (CIS) [18].
Survival analysis
Regression analysis is undertaken in order to estimate the hazard of dying using a parametric modelling approach. A Gompertz distribution of the hazard of dying is assumed and has been used extensively by researchers to model mortality data [19]. Time until death is predicted for both survivors and decedents utilising the entire sample (N = 140,753). Results guided the decision to exclude those whose death was predicted to be beyond April 2015 (20 quarters beyond censoring), resulting in 60,808 observations. The following covariates are included to predict death: age at study entry (in either 1991 or 2001), gender and the socioeconomic status (measured in deprivation score quintiles and with quintile 1, the most affluent quintile, serving as the reference group). The coefficients obtained from the Gompertz regression are utilised to calculate the linear predictor of time until failure for each surviving participant using their respective covariate values. Based on these results the probability of surviving each year after study entry can be calculated using the respective survival function for a Gompertz distribution (Eq. 1). This is extended up to t = 100 with the probability of survival becoming infinitesimal.
where: \(\lambda_{j}\) = linear predictor; \(\gamma\) = ancillary or shape parameter; t = time period indicator.
In order to obtain a value for survivors’ additional predicted years of life, the area under the curve is calculated for that part of the curve that is beyond the censoring date using the trapezoid rule (Eq. 2). Adding up values for each of the segments beyond censoring provides a prediction of the number of additional years of life for survivors.
where T = trapezoid segment; H = hazard; Y = years after study entry.
Predicted additional years of life are transformed into quarters and the observed quarters before death are adjusted according to the number of additional quarters of life that were predicted. Adjustment of quarters is displayed in Fig. 1. In addition to adjusting observed quarters before death, the age at death is adjusted accordingly. Surviving individuals will not contribute any cost observations for the quarters closest to death, which had been predicted as additional quarters of life. As a result there will be missing cost observations (right censoring) stemming from the fact that individuals cannot be observed beyond the censoring date. Similarly, cost observations are missing (left censoring) for any hospitalisations that occurred before the start date of the study.
Explanatory variables
To represent TTD, a series of 20 quarter dummies are defined, where 1 represents the last quarter of life, 2 the penultimate quarter of life, etc. The quarter furthest away from death (20th quarter) serves as the reference category. Age at death is measured in seven categories (45–64, 65–69, 70–74, 75–79, 80–84, 85–89, 90 years and over) with the youngest age group serving as the reference category. Interaction terms between TTD in quarters and age at death are included to capture any combined effect of ageing and TTD on HC costs. Gender is included to account for differences in costs incurred by males and females. To account for differences in costs incurred by socioeconomic status a measure of deprivation is included using the Carstairs deprivation score quintiles, where the lowest quintile (1) serves as the reference category. Interaction terms between TTD and deprivation quintiles are included to control for any combined effects. The assumption is that TTD affects costs differently for different socioeconomic groups. An indicator to capture time trends (year of admission), especially to reflect advances of medical technology, is included. This variable is measured in five categories: 1986–1990, 1991–1995, 1996–2000, 2001–2005 and 2006–2010), with the most historic period serving as the reference group. A measure of individuals’ health status at baseline is included using information on self-reported health problems. In 1991 participants were asked whether they had a health problem or not. This question was worded differently in 2001, asking SLS sample members whether they would perceive themselves as having a limiting long-term illness (LTI), a health problem or disability that limits them in carrying out their daily activities and the work they are able to do. Therefore, a composite measure of health is used whereby an individual was categorised as having a health problem if they had replied ‘yes’ in either 1991 or 2001 or in both years.
The underlying assumption is that the expected value of HC expenditure is a function of these explanatory variables.
Econometric model
Costs for acute inpatient care are estimated using a two-part model with the first part estimating the probability of accessing HC and the second part estimating costs conditional on positive HC utilisation. The results of this two-part model are later utilised to project HC costs under the TTD approach. The first modelling part employs a probit link and a binomial distribution to estimate the probability of utilising hospital care in any given quarter before death conditional on regressors X (Eq. 3).
where A represents categories for age at death with the youngest age group serving as the reference category; S represents gender; H is a dummy variable representing poor health (LTI); Q is a dummy variable representing the remaining quarters of life (such that Q × A is the interaction of TTD and age) and the quarter furthest away from death (20th quarter) serves as the reference category; Y is a time period dummy, indicating in which time period hospital admissions fall; D is a dummy for deprivation score quintiles such that Q × D is the interaction of TTD and deprivation.
The second part of the model provides estimates of HC costs, conditional on HCE being greater than zero and conditional on the same set of regressors X (Eq. 4).
with xβ representing the linear predictor for HCE.
Quarterly HCE is estimated fitting a Generalised Linear Model (GLM) clustered on patient identifier. Following conventions for determining the appropriate distribution and link function, diagnostic tests are performed using the user written programme ‘glmdiagnostic’ within STATA [20–22]. Predicted probabilities of positive HC utilisation, obtained from the first part of the model, are multiplied by cost estimates from the second part of the model in order to derive average cost estimates (Eq. 5).
To mitigate problems arising from serial correlation a CIS was used as the basis for the cost variable, summarising single hospital episodes if more than one episode formed the entire hospital stay. Clustering on individual identifier was applied to account for any serial correlation that still existed because multiple observations (CISs) can come from the same individual.
HC expenditure projections: demographic approach
Healthcare expenditure projections are based on 2008 population estimates provided by National Records of Scotland (NRS) up until the year 2033. Population estimates for the year 2011 are utilised as the base year, representing an index of 100. Projected costs are obtained for four future time points (2016, 2021, 2026 and 2028Footnote 3) and for that part of the population aged 65 and older, stratified by age group and gender. Observed annual per capita HCE is obtained for the entire SLS sample. Per capita HCE is observed for 3 consecutive years (2006, 2007 and 2008) for which the average is calculated stratified by gender and age group. Per capita average annual HCE is multiplied by population estimates, stratified by age group and gender for the years 2011, 2016, 2021, 2026 and 2028 to obtain an estimate of the overall expected financial burden on a population level. Finally, costs are aggregated over age group and gender and the resulting cost provides a projection under a simplified approach, which accounts for demographic changes in the population.
HC expenditure projections: TTD approach
In addition to stratifying projected population numbers by age group and gender, the TTD approach also accounts for the number of people in each of these groups who are in their last 5 years of life. Each age is stratified by TTD in years, so that an estimate of the population in each calendar year that is in their last year of life, the penultimate year of life, etc., can be obtained. This is achieved by using projected number of deaths by age and gender for the years 2012–2016 (Number of Deathsage/sex 2012–2016), available from NRS. Equation 6 estimates the number of individuals projected to be in a particular year before death (year 1–5), using the base year 2011 as an example.
where TTD1–5 is the number of the population projected to be in a particular year before death year 1–5. Deathsage/sex 2012–2016 is the projected number of deaths by age and sex for the years 2012–2016.
Cost estimates per capita for the TTD approach are obtained from the econometric model (Eq. 5). Costs are stratified by age group, gender and remaining TTD. Quarterly cost estimates are aggregated to represent annual costs. Finally, per capita costs are multiplied by the respective population estimates for each age group, gender and TTD stratum, providing a population estimate of the future financial burden. Summarising individual group results provides an estimate of HCE projections for the years 2011, 2016, 2021, 2026 and 2028. As above, the year 2011 serves as the base year for HCE projections and costs for that year are indexed to 100. All subsequent analyses consider any deviation from this indexed level. A comparison of the growth rate of costs from 2011 onwards between the demographic approach and the TTD approach is undertaken to show whether a simplified approach overestimates future HC costs and, if that is the case, the magnitude of the overestimation.
Results
Characteristics for the sample utilised in the regression analyses (N = 60,808)—for the entire sample and by survivor status at the end of the study—are presented in Table 1. A total of 18,140 individuals (29.8 %) did not have a death record at the end of the study period on the 30 April 2010. A similar distribution of males and females can be found in both the survivor group and in the decedent group. Approximately 7 % of the entire sample population had never accessed hospital care, a proportion that differs significantly (p < 0.01) by survival status. A slightly higher proportion of survivors lives in postcode areas that belong to the most affluent deprivation quintile compared to the decedent group, while a slightly lower proportion of survivors can be found living in areas that belong to the most deprived quintile compared to the decedent group (p < 0.01). Overall, 53.2 % of all sample members reported an LTI. A significantly higher proportion of decedents reported having an LTI 56.3 %, while 45.8 % of survivors stated that they suffered from an LTI (p < 0.01).
Survival analysis
Regression results for the Gompertz survival analysis are presented in Table 2. Estimates are presented as hazard ratios and show the expected signs with the risk of failing (dying) increasing as age at study entry increases. Each additional year at study entry increases the risk of dying by about 10 %. Male SLS sample members also show a higher risk (47 %) of dying than their female counterparts and an overall significant effect of socioeconomic status on the risk of dying is observed. Individuals from the most deprived quintile show a risk of dying that is 65 % higher than that of individuals living in the most affluent quintile. The size of the effect increases as deprivation increases. The shape parameter ‘gamma’ shows a positive value, indicating an exponentially increasing risk of failure.
Probability of HC utilisation
Regression results for the first modelling part are presented in Table 3 (columns 1 and 2). Up to the 15th quarter before death, TTD has a highly statistically significant and positive association with the probability of being admitted to hospital (p < 0.01). The size of the effect is largest for the last quarter before death and generally increases as people approach death. Significant interaction effects can be found between age and TTD (Table 4). TTD estimates shown in Table 3 are compared with the quarter furthest away from death (20th quarter) and are for the youngest age group (45–65 years) due to the inclusion of interaction terms. Significant interaction effects can especially be found for the older age groups and up until the 12th quarter before death (Table 4). A steeper gradient, i.e., a larger effect of TTD, can be observed for the younger age groups and a slightly flatter gradient is found for the two oldest age groups. Regression results in Table 3 also show that individuals’ socioeconomic status does not have an impact on their probability of utilising hospital services. Similar to the effect that age has on the probability of utilising HC, these main effects cannot be interpreted directly and results for the interaction terms between TTD and deprivation score quintiles (Table 5) reveal that any effect that the socioeconomic status has on the probability of accessing HC services is also influenced by TTD. This can be observed especially for the last quarter of life, where the association is highly significant and negative. As individuals approach death, those living in more deprived areas are less likely to reach hospital than those individuals living in the most affluent areas. Individuals who had stated that they suffered from an LTI are shown to have a higher probability of being admitted to hospital (p < 0.01) compared with those who do not suffer from an LTI.
Cost estimation conditional on HCE being > zero
Regression results (cost ratios and corresponding standard errors) for the 2nd modelling part estimating costs given positive HC utilisation are also presented in Table 3 (columns 3 and 4). Costs increase as people approach death. Significant interaction effects between age and TTD can also be found for this second modelling part; however, the magnitude is not as pronounced as the one for the first modelling part (Table 6). Costs are estimated to be about 85 % higher in the last quarter of life compared to the 20th quarter before death and are again for those aged 45–64 years. Age at death is a significant predictor for mean quarterly costs for all but the second youngest age group. A statistically significant effect of the interaction between TTD and age is only observed for the last quarter of life and the two oldest age groups (Table 6). The socioeconomic status has a negative impact on costs for those individuals living in the 2nd, 3rd, 4th or 5th quintile compared to individuals living in the most affluent quintile; however, this effect is not statistically significant. In order to investigate whether the effect that the socioeconomic status has on costs is influenced by TTD, the interaction terms were included (Table 7) and results only show very small effects for some quarters without any recognisable pattern. Individuals who have reported an LTI incur significantly higher costs compared to those without any LTI (6 %).
Overall cost estimates
Average cost estimates by age and TTD are shown in Fig. 2. These were obtained from multiplying the first part of the model (probability of hospitalisation) and the second modelling part (costs incurred given positive utilisation), as outlined in Eq. 5. Costs in the last year of life seem to be highest for ages 70–84 at death and lowest for the two oldest age categories. Differences in costs between different times away from death are less pronounced for the two oldest age groups than they are for the younger ages. Figure 2 clearly shows the interaction effects between age and TTD.
Average cost estimates by deprivation score quintile vary between £1,960 (SD = 587) for the least deprived quintile and £2,054 (SD = 521) for the most deprived quintile in the last quarter of life. This shows that in the last quarter of life nearly £100 more is spent on individuals from the most deprived areas compared to people from the most affluent areas. Differences in costs between socioeconomic groups become less marked the further away from death an individual is.
HC expenditure projections
Results obtained from HCE projections for both the demographic approach and the TTD approach are shown in Fig. 3, showing an overestimation of future HCEs for the over 65s for the acute inpatient care sector under the demographic approach.
The magnitude of the overestimation is observed to be between 2 % in 2016 and 7 % in the year 2028 (Table 8). The growth rate observed for the TTD approach is projected to be lower than the growth rate that would be obtained when applying a simplified approach and only accounting for demographic changes in the population. Comparing the two approaches of projecting HCEs suggests that expenditure is not predicted to rise as quickly if factors such as TTD and increasing longevity are accounted for.
Discussion
Using a representative sample of the Scottish population, the analysis has shown that TTD, age at death and the interaction between these two have a significant effect on HC costs and so confirms findings from previous research [2, 4, 23]. This confirmation is significant because we were able to utilise data from a large, representative sample of the population, something that has not been possible in other studies. The analysis further showed that TTD influences HCE differently for different age groups and deprivation score quintiles, highlighting the importance of including interaction terms in any such analyses. Overall, costs increase towards the end of life; however these costs are lower for the older age groups. This suggests that HC costs are not distributed evenly over an individual’s life cycle, implying that physicians may treat older people less aggressively and may devote more resources to those that benefit most, i.e. younger people. This age-related rationing of HC had been described in the literature by Williams, who put forward his ‘fair innings’ argument [24].
As people approach death, those living in more deprived areas are less likely to be hospitalised compared to those living in the most affluent deprivation score quintile. These findings confirm previous research undertaken in Scotland that considered out-of-hospital cardiac deaths by socioeconomic status and found that mortality rates were much higher in deprived socioeconomic groups [25]. Although less likely to be admitted to hospital, ‘poorer’ people seem to be costing marginally more in their last 5 years of life compared with people from more affluent areas and results were only marginally statistically significant for some TTD and deprivation score combinations. Previous research had shown that the type of costing method can significantly influence estimated results [18], something that merits further investigation.
In light of the findings on the association between TTD, age and costs, two approaches to projecting HCE were presented. It was demonstrated that expenditure is not projected to rise as quickly if factors such as TTD, increasing longevity and the postponement of diseases into older ages were accounted for. The analysis showed that if death is postponed into older ages, HCE (and HC budgets) would not increase to the same extent should these factors be ignored. Such factors would be ignored if the population that is in their last year(s) of life were not taken into consideration when obtaining cost estimates.
Findings in this article are in line, although at the lower end of the scale, with other research that found that future HCE is at risk of being overestimated if TTD is not accounted for with estimates ranging from 3.4 to 18.5 % [5, 23, 26, 27]. If death is the main contributing factor to HC costs, then the fact that people live longer and death occurring at a later age can result in the costs of dying decreasing with age beyond the age of 75 (Fig. 2). It is therefore partially counteracting the effect that an increasing proportion of elderly people might have on HCE. However, as diagnostic techniques, management and treatment of diseases are changing and more is being spent on avoiding disease rather than curing it, a shift from expenditure on acute inpatient care towards HC sectors that are concerned with preventative care, i.e. primary care, might be observed in the future.
This article is the first to provide estimates of death-related costs in Scotland. As argued in the introduction, HC systems and organisation of HC delivery differ between countries, and it is thus important to undertake these analyses on a national level. In addition, this study estimated and compared future cost projections with and without taking account of costs at the end of life. This research has implications for both resource allocation, as it highlights the need to include a measure of TTD into resource allocation formulae, and HC planning and budgeting. It clearly shows a divergence in future costs should TTD be excluded from the modelling process. Especially in austere times it is important to correctly predict and project at least part of the future financial burden. Data are usually readily available for remaining life expectancy and future population structure. Over-budgeting for an elderly population would consequently mean that not enough financial resources are planned for other areas.
It is also pivotal to take account of the exact distribution of costs over a life cycle. Death-related costs constitute a very large part of these costs and as such should be included in any resource allocation formulae. If age alone was responsible for all HC spending estimates, then there would be considerable opportunity costs ignoring other aspects, such as treatment intensity at the end of life, age-related rationing and advances in medical technology over time.
Considerations and future research
The analysis utilised a large, representative sample of the Scottish population; however when interpreting results it should be noted that HC expenditure projections have been calculated for the acute inpatient care sector only. Although constituting a large proportion of the entire HCE, it does not provide a complete picture of the entire HC system and its associated costs. Another important factor that contributes to differences in findings will be each country’s demographic structure. Differences between projection approaches will be larger if demographic changes occur more rapidly, for example emigration of younger individuals. If the number of deaths in each age group were to remain constant over time then no differences would be found between approaches.
The analyses in this article assumed exogeneity between TTD and HCE, similar to many other studies. However, the possible endogeneity between these two variables is an issue that has not been solved entirely to date. Further methodological work should be concerned with the inclusion of measures of uncertainty around HCE, TTD and population estimates. In addition, other HC sectors would need to be analysed. It would be highly informative to be able to analyse a broader spectrum of HC services, in particular the long-term care sector, in order to gain an overall understanding of the financial impact of an ageing population.
Notes
SMR01 has episode-based patient records that relate to all acute inpatient and day cases. A record is generated when a patient completes an episode of inpatient or day case care and episodes are summarised into ‘Continuous Inpatient Stays’, CIS.
An initial exploration of the data showed that costs increased markedly in the last two quarters of life. Exploratory regression analysis determined when TTD became an insignificant predictor for costs. It was therefore decided to analyse the last 5 years of life, measured in quarters to provide variance for the analysis. Quarters have also been used in previous studies [4, 5].
Since population estimates are only available until 2033 and since it is required for the TTD approach to be able to calculate the proportion of the population in year 1 to 5 before death, the last estimate can be obtained for the year 2028.
References
Payne, G., Laporte, A., Deber, R., Coyte, P.C.: Counting backward to health care’s future: using time-to-death modelling to identify changes in end-of-life morbidity and the impact of aging on health care expenditures. Milbank Q. 85(2), 213–257 (2007)
Zweifel, P., Felder, S., Meiers, M.: Ageing of population and health care expenditure: a red herring? Health Econ. 8(6), 485–496 (1999)
Zweifel, P., Felder, S., Werblow, A.: Population ageing and health care expenditure: new evidence on the ‘red herring’. Geneva Pap. Risk Insur. 29(4), 652–666 (2004)
Seshamani, M., Gray, A.M.: Ageing and health-care expenditure: the red herring argument revisited. Health Econ. 13(4), 303–314 (2004)
Seshamani, M., Gray, A.: Time to death and health expenditure: an improved model for the impact of demographic change on health care costs. Age Ageing 33(6), 556–561 (2004)
Breyer, F., Lorenz, N., Niebel, T.: Health care expenditures and longevity: is there a Eubie Blake effect? Discussion Paper 1226, DIW Berlin (2012)
Moorin, R.E., Holman, C.D.: The cost of in-patient care in Western Australia in the last years of life: a population-based data linkage study. Health Policy 85(3), 380–390 (2008)
Moorin, R., Gibson, D., Holman, D., Hendrie, D.: The contribution of age and time-to-death on health care expenditure for out-of-hospital services. J. Health Serv. Res. Policy 17(4), 197–205 (2012)
McGrail, K., Green, B., Barer, M.L., Evans, R.G., Hertzman, C., Normand, C.: Age, costs of acute and long-term care and proximity to death: evidence for 1987–88 and 1994–95 in British Columbia. Age Ageing 29(3), 249–253 (2000)
Baal, P.H., Wong, A.: Time to death and the forecasting of macro-level healthcare expenditures: some further considerations. J. Health Econ. 31(2012), 876–887 (2012)
Cookson, R., Laudicella, M.: Do the poor cost much more? The relationship between small area income deprivation and length of stay for elective hip replacement in the English NHS from 2001 to 2008. Soc. Sci. Med. 72(2), 173–184 (2011)
WHO World Health Organisation: Health inequality, inequity and social determinants of health. http://www.who.int/social_determinants/resources/interim_statement/csdh_interim_statement_inequity_07.pdf (2007). Accessed Sept 2011
Graham, B., Normand, C.: Proximity to death and acute health care utilisation in Scotland. Final Report, Chief Scientist Office (2001)
Hattersley, L., Boyle, P: The Scottish longitudinal study. An introduction. LSCS working paper 1.0. Edinburgh/St Andrews, Longitudinal Studies Centre Scotland (2007)
Hattersley, L., Raab, G., Boyle, P.: The Scottish longitudinal study. Tracing rates and sample quality for the 1991 census SLS sample. LSCS Working Paper 2.0. Edinburgh/St Andrews: Longitudinal Studies Centre Scotland (2007)
Hattersley, L., Boyle, P.: The Scottish longitudinal study, a technical guide to the creation, quality and linkage of the 2001 census SLS sample. LSCS working paper 3.0. Edinburgh/St Andrews, Longitudinal Studies Centre Scotland (2009)
Hattersley, L., Boyle, P.: The Scottish longitudinal study, The 1991–2001 Scottish longitudinal study census link. LSCS Working paper 4.0. Edinburgh/St Andrews, Longitudinal Studies Centre Scotland (2009)
Geue, C., Lewsey, J., Lorgelly, P., Govan, L., Hart, C., Briggs, A.: Spoilt for choice: implications of using alternative methods of costing hospital episode statistics. Health Econ. 21(10), 1201–1216 (2012)
Cleves, M., Gould, W., Gutierrez, R., Marchenko, Y.: An introduction to survival analysis using STATA, 2nd edn. STATA Press, College Station (2008)
Glick, H.A.: ‘glmdiagnostic.do’. http://www.uphs.upenn.edu/dgimhsr/eeinct_multiv.htm (2008). Accessed Sept 2010
Pregibon, D.: Goodness of link tests for generalized linear models. Appl. Stat. 29, 15 (1980)
Pearson, E.S., Please, N.W.: Relation between the shape of population distribution and the robustness of four simple test statistics. Biometrika 62, 223–241 (1975)
Stearns, S.C., Norton, E.C.: Time to include time to death? The future of health care expenditure predictions. Health Econ. 13(4), 315–327 (2004)
Williams, A.: The rationing debate. Rationing health care by age: the case for. BMJ 314(7083), 820–825 (1997)
Capewell, S., MacIntyre, K., Stewart, S., Chalmers, J.W.T., Boyd, J., Finlayson, A., Redpath, A., Pell, J.P., McMurray, J.J.V.: Age, sex, and social trends in out-of-hospital cardiac deaths in Scotland 1986–95: a retrospective cohort study. Lancet 358, 1213–1217 (2001)
Hakkinen, U., Martikainen, P., Noro, A., Nihtila, E., Peltola, M.: Aging, health expenditure, proximity to death, and income in Finland. Health Econ. Policy Law 3(Pt 2), 165–195 (2008)
Serup-Hansen, N., Wickstroem, J., Kristiansen, I.S.: Future health care costs–do health care costs during the last year of life matter? Health Policy 62(2), 161–172 (2002)
Acknowledgments
The authors would like to thank Fiona Cox, Lee Williamson, Claire Boag and Joan Nolan of the Longitudinal Studies Centre-Scotland (LSCS) for their help provided. The LSCS is supported by the ESRC/JISC, the Scottish Funding Council, the Chief Scientist’s Office and the Scottish Executive. The authors alone are responsible for the interpretation of the data. Census output is Crown copyright and is reproduced with the permission of the Controller of HMSO and the Queen’s Printer for Scotland. This work was supported by a Medical Research Council (MRC) PhD studentship.
Conflict of interest
None.
Ethical standard
Permission was given by the Privacy Advisory Committee of ISD to use linked SMR data.
Author information
Authors and Affiliations
Corresponding author
Rights and permissions
About this article
Cite this article
Geue, C., Briggs, A., Lewsey, J. et al. Population ageing and healthcare expenditure projections: new evidence from a time to death approach. Eur J Health Econ 15, 885–896 (2014). https://doi.org/10.1007/s10198-013-0543-7
Received:
Accepted:
Published:
Issue Date:
DOI: https://doi.org/10.1007/s10198-013-0543-7