Abstract
Gene co-expression network analysis has been a research method widely used in systematically exploring gene function and interaction. Using the Weighted Gene Co-expression Network Analysis (WGCNA) approach to construct a gene co-expression network using data from a customized 44K microarray transcriptome of chicken epidermal embryogenesis, we have identified two distinct modules that are highly correlated with scale or feather development traits. Signaling pathways related to feather development were enriched in the traditional KEGG pathway analysis and functional terms relating specifically to embryonic epidermal development were also enriched in the Gene Ontology analysis. Significant enrichment annotations were discovered from customized enrichment tools such as Modular Single-Set Enrichment Test (MSET) and Medical Subject Headings (MeSH). Hub genes in both trait-correlated modules showed strong specific functional enrichment toward epidermal development. Also, regulatory elements, such as transcription factors and miRNAs, were targeted in the significant enrichment result. This work highlights the advantage of this methodology for functional prediction of genes not previously associated with scale- and feather trait-related modules.
Similar content being viewed by others
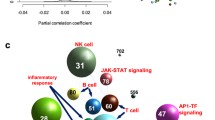
Avoid common mistakes on your manuscript.
Introduction
Avian feathers and scutate scales are intricate structures of birds and perform multiple functions such as flight, thermal regulation, and protection (Gill 1995). Hundreds of genes (i.e., signaling pathway genes, structural genes, cell adhesion genes, etc.) have been associated with the morphogenesis of these two structures (Harris et al. 2002; Widelitz et al. 1999; Andl et al. 2002; Bell and Thathachari 1963; Baden and Maderson 1970; Haake et al. 1984; Rogers 1985; Fischer et al. 1997, Shames et al. 1994; Tucker 1991; Maderson et al. 2009), and gene co-expression network analysis is a new approach to discover gene interactions in a large gene expression datasets (Van Noort et al. 2003; Stuart et al. 2003; Horvath and Dong 2008; Ng et al. 2014; Chang et al. 2015). It is based on the quantitative pairwise relationships among thousands of gene transcript profiles (Zhou et al. 2002; Steffen et al. 2002; Stuart et al. 2003; Zhang and Horvath 2005; Carey et al. 2005; Schäfer and Strimmer 2005; Chuang et al. 2008; Langfelder and Horvath 2008). Gene network construction has been applied to identify gene pathways and the genes underlying complex traits (Barabasi and Oltvai 2004; Featherstone and Broadie 2002; Thieffry et al. 1998).
Here we performed a Weighted Gene Co-expression Network Analysis (WGCNA) using a customized 44K chicken microarray dataset of total RNA extracted from feather and scutate scale tissue during chicken embryonic development (Li et al. 2008). A WGCNA network is defined as sets of cluster nodes with each node representing a single transcript (Langfelder and Horvath 2008). The edges, which are the connections between nodes, are calculated by the Pearson’s correlation coefficient based on the expression profile of the transcripts (Langfelder and Horvath 2008). WGCNA can identify gene modules which are the clusters of highly interconnected genes (Langfelder and Horvath 2008) associated with a trait. This method has been successfully applied in the diverse field of genomic research (Xue et al. 2013; Wang et al. 2014; Hickner et al. 2015). There are two types of co-expression networks: condition free and condition-dependent networks. The condition free co-expression network normally utilizes large datasets from different conditions including tissue types, biological traits, and experimental conditions to construct the basis for genetic architecture of gene expression profiles. The traditional (condition free or dependent) gene co-expression network analysis is limited by the small group of differential gene expression data which are chosen using arbitrary thresholds. In order to study the specificity of epidermal development in the chicken embryo, we defined and applied the trait conditions (condition dependent network analysis) during epidermal embryogenesis and built modules showing the trait relatedness with all annotated genes in the customized microarray.
Material and methods
Tissue samples and microarray experiment
Chicken dorsal feather (DF) and scutate scale (SC) tissues were taken at days 8, 17, and 19 of chicken embryonic development, while wing feather (WF) tissue was taken at days 17 and 19. A customized version of the chicken 44K Agilent microarray was used to analyze mRNA extracted from these tissue samples with a total of 31 individual microarray expression samples analyzed. The details of experiment can be found in Bao et al. (2016) and Greenwold et al. (2014).
Data set pre-processing
The raw gene expression Feature Extraction (FE) files produced by Agilent High-Resolution Microarray Scanner (Agilent Technologies; Palo Alto, CA) were imported into Agilent GeneSpring software (13.0 GX version: Agilent Technologies; Palo Alto, CA). The customized probes were 639 miRNA genes, 27 α-keratin genes, 102 β-keratins, and 2 Histidine-Rich Protein (HRP) genes. All the probes in the microarray were normalized with the percentile shift algorithm internally and the percentile target was set as the default 75% and the preprocess baseline for all samples was set to the median option. All the probes with the same Entrez Gene ID and Gene Symbol ID were grouped on average as the same gene and this also applied to the probes having the same customized annotation.
Network construction and module detection
After the normalization and grouping steps of all probe expressions, there were 12,601 genes with Entrez Gene IDs or customized annotations, while 13,118 genes did not have any type of Primary Accession annotations. We used the hclust function in WGCNA to achieve standard hierarchical clustering for the identification of outlier microarray samples and there is no obvious outlier sample (Supplemental Fig. 1) (Langfelder and Horvath 2008). The clustering result also complies with previous clustering results (Bao et al. 2016). We define the biological traits (scale, feather, day, stages, etc.) as a binary system (1 or 0 represents positive or negative; Supplemental Table 1) to relate each detected module. The adjacency of the unsigned network matrix was calculated based on the absolute Pearson correlation coefficient raised to the power β. Soft-thresholding power 12 was determined by the optimum scale-free topology which target the balance between scale-free fit index and the mean connectivity (Supplemental Fig. 2). The modules were detected by the calculation of Topological Overlap Measure (TOM) and the static tree was cut by merging height as 0.25 with the minimum module size as 30 genes. All modules were named by a different color (i.e., steelblue, brown, darkred, etc.; see also Fig. 1).
The heatmap of Module-Trait relationships for the co-expression network. Each row, identified by different colors, represents modules of highly correlated genes and each column corresponds to a defined external trait. Each cell contains the corresponding correlation and p value for each respective module and trait. The brown module and black module are highlighted with a gold rectangle. The module-scale trait correlation is 0.95 for the brown module and the module-feather trait correlation is −0.97 for the black module
Hub gene detection
The hub genes are defined as the ones having multiple internode connections based on the weight calculation (Langfelder and Horvath 2008). The intra-modular hub genes within the particular trait-related module are more important than the whole network hub genes (Zhang and Horvath 2005; Horvath et al. 2006; Langfelder et al. 2013). The weight threshold was set at default value (0.05) for the Medical Subject Headings (MeSH) enrichment analysis of module hub genes.
Traditional functional annotation and analysis of module genes
KOBAS (KEGG Orthology Based Annotation System, V3.0; http://kobas.cbi.pku.edu.cn/; Xie et al. 2011) was performed to identify biological pathways and Gene Ontology terms for the genes in 11 modules having both moderate Module-Trait and Module Membership correlations (Supplemental Table 2; see below in “Results”). The foreground genes are the ones having Entrez Gene ID annotations and the background was set as the whole genome of the chicken. The KEGG pathway database (Kanehisa and Goto 2000) were loaded to perform the hypergeometric/Fisher’s exact test and the FDR correction method (Benjamini and Hochberg 1995). GO classification count of all nonduplicate enriched GO annotations were performed by CateGOrizer (Hu et al. 2008).
Gene enrichment analysis using the Modular Single-Set Enrichment Test
Enrichment for chicken epidermal development-related genes (EDRGs) of the most significant microarray results, based on the ranking of Gene Significance (GS) value, was assessed using the Modular Single-Set Enrichment Test (MSET) algorithm (Eisinger et al. 2013). GS value is the correlation measurement based on the gene expression profile and the external traits (equation: GSi = |cor (Genei, Trait)|; Langfelder and Horvath 2008). Therefore, each gene has its own GS value corresponding to the specific trait, and the higher the absolute value of the GS, the more important the gene is to the trait (Langfelder and Horvath 2008). The gene set of interest, relating to chicken epidermal development, was established from a customized gene set having 302 genes as recently reported by Bao et al. (2016). This customized gene set essentially combines the Lowe et al. (2015) curated feather development-related genes and the chicken α- and β-keratins annotated by Greenwold et al. (2014). All background genes were ranked by the descending absolute value of gene significance (GS) for feather and scale traits. The GS value of each gene corresponding to a trait was calculated by WGCNA and the details can be found at Supplemental File 1. The number of genes for each MSET randomization step was determined by the number of genes with GS ≥ |0.85|. Each analysis used 5000 simulations for the randomization.
Medical Subject Headings-informed enrichment analysis
We downloaded the enrichment tool package meshr, MeSH database package MeSH.db, and the chicken-specific annotation package MeSH.Gga.eg.db from Bioconductor (http://bioconductor.org/packages/release/data/annotation/html/MeSH.Gga.eg.db.html) (Tsuyuzaki et al. 2015; Morota et al. 2016). We screened all genes having an Entrez Gene ID for the enrichment analysis since the MeSH terms are widely associated with this particular annotation. After loading all three packages, the hypergeometric test was performed on the background genes (all microarray genes with Entrez Gene IDs). We performed MeSH enrichment analyses on selected module hub genes and the selection of the hub genes in each module was based on the weight (w ≥ 0.05) and the connectivity (n ≥ 2) threshold calculated by WGCNA. Significantly enriched (p value ≤0.05) MeSH terms can be detected as three categories: A (Anatomy), D (Chemicals and Drugs), and G (Phenomena and Processes).
Results
Network structure
We used 26,359 expressed genes from the customized 44K chicken microarray to construct a scale-free co-expression network using the WGCNA algorithm. We identified 33 modules which included most of the genes in the customized microarray. There are 338 genes categorized as non-module genes that are not co-expressed with other module genes. Figure 1 is the heatmap of the Module-Trait relationship for the co-expression network. Each row is identified by different colors, representing modules of highly correlated genes and each column corresponds to a defined external trait. Each cell contains the corresponding eigengene (E) significance which is the correlation between each module eigengene and the defined external trait (scale, feather, etc.) of the samples. In this study, the eigengene is the virtual gene of the module that represents the expression profile in the whole module. We found that 9 out of the 33 modules are moderately correlated (r ≥ 0.5, p ≤ 0.01) with scale traits (Fig. 1). Six of the 33 modules are moderately correlated (r ≥ 0.5, p ≤ 0.01) with the feather traits (Fig. 1). It is notable that the brown module (r = 0.98, p = 2e−22) and the black module (r = −0.97, p = 1e−18) have very strong correlation values with scale and feather traits, respectively.
In contrast to the eigengene significance, each gene in the whole network has its own GS which is a measure of the correlation between a single gene and the external traits. In other words, the higher the absolute value of the GS, the more important the gene is to the trait. To further investigate the relationship between the traits and the genes, we plotted the correlation of module membership (MM) and the GS for each module (Fig. 2). Since the module membership is defined as the correlation between each gene and the modular eigengene, it is reasonable that the modules having high correlation of module membership and gene significance with the external trait tend to be more related to the trait because the eigengene is a more reliable representative gene for the whole module. Based on this intra-modular analysis, we further identified seven modules with the scale trait and five modules with the feather trait that have both moderate correlations (r > 0.5, p < 0.01) for Module-Trait correlation and MM-GS correlation (Supplemental Table 2). One of these modules (greenyellow) correlates with both the scale and feather traits. As Fig. 2 shows, the brown module with the scale trait and the black module with the feather trait have strong correlations (r > 0.9, p < 0.01) in the scatter of the MM-GS correlation plot and they are the only modules that also have strong Module-Trait correlations (r > 0.9, p < 0.01). Therefore, these two modules have great potential to influence epidermal development during chicken embryogenesis. Below we will present the analyses of these two important modules (brown and black) using novel enrichment methods (MSET and MeST) to understand the chicken epidermal development.
The gene scatter plot of correlation for Module Membership (MM) and Gene significance (GS). Each spot represents a single gene. The horizontal axis is the module membership value which represents the connectivity of a single gene and the virtual module representative gene (eigengene). The vertical axis is the gene significance (GS) value which represents the correlation of a single gene and the external trait. Figure 1 shows that brown module has a high module-scale trait correlation and black module also has a high module-feather trait correlation. The top figure shows that the brown module has high correlation of MM-scale GS (r = 0.98, p = 2e−22), while the bottom figure shows that the black module (r = −0.97, p = 1e-18) has high correlation of MM-feather GS. All the EDRG genes in each module
KEGG pathway and Gene Ontology enrichment
We used an integrative functional enrichment tool (KOBAS 3.0) on the 11 unique modules having both moderately correlated Module-Trait and MM-GS relationships to identify the biological pathways and Gene Ontology (GO) terms. We recorded all the significantly enriched KEGG pathways, GO annotations, corrected p values, and correlated traits for each of the 11 modules (Supplemental File 2). All 11 modules have enriched KEGG pathways and GO annotations. It is notable that some well-known signaling pathways (Wnt and TGF-beta) known to be involved in feather skin differentiation (Chang et al. 2004; Jiang et al. 2004; Chuong et al. 1991) were enriched. There are 1582 unique enriched GO terms across the 11 modules and 1123 (71%) of them are classified as Biological Process root terms.
Enrichment for chicken epidermal development genes using MSET analysis
A set of genes relating to chicken epidermal development (EDRGs) was compiled as stated in the “Material and methods” section. We ran four sets of MSET analyses using the background genes: all microarray genes using their feather GS value, all microarray genes using their scale GS value, brown module genes using their scale GS value, and black module genes using their feather GS value. For the MSET analysis using the whole microarray background (feather and scale GS values), we preprocessed the gene module dataset and obtained 13,241 genes having either Gene Symbol annotations or our customized α- and β-keratin annotations. The enrichment analyses of scale and feather traits are based on the rankings of the GS values of scale and feather traits obtained with WGCNA. MSET analyses found significant enrichment in both top (genes with GS ≥ |0.85|) microarray results (scale and feather traits) indicating that chicken epidermal development genes appear in the significant microarray data at a rate higher than what would be due to random chance (Fig. 3). Alpha (α-) and beta (β-) keratins were enriched in both of the top list of genes in the analysis based on ranked feather/scale GS value as the background. By applying the same threshold for the top gene selection under the MSET algorithm, we also performed a MSET analysis on the background genes of the brown and black modules ranked by scale GS (brown module) and feather GS (black module) for each module. Both of these analyses found significant enrichment for the “top genes” based on the trait GS threshold (genes with GS ≥ |0.85|; see “Material and methods” section) and α- and β-keratins are still enriched in the brown module with scale GS (Fig. 3).
The output of MSET enrichment analysis for the customized chicken epidermal development-related gene database within the chicken embryonic epidermal development expression data. The background genes for window a and b are the whole microarray genes and the importance ranking are based on scale gene significance (GS) and feather gene significance (GS) for each gene, respectively. Likewise, the background genes for window c and d are the black module and brown module, respectively. The gene importance order of black and brown modules is based on the value of the feather gene significance (GS) and scale gene significance (GS) for each gene. The vertical axis represents the probability of X number of matches to the customized database found in a randomly generated set of simulated results from the background
Hub gene enrichment analysis for the highly correlated trait modules
The MeSH database provides 16 different categories of MeSH terms (Tsuyuzaki et al. 2015) derived from the National Library of Medicine (NLM). We chose three major categories (A, Anatomy; D, Chemicals and Drugs; and G, Phenomena and Processes) as they related directly to this study and ignored other unrelated categories such as “Psychiatry and Psychology,” “Geographical Locations,” “Humanities,” etc. Table 1 lists the statistically significant MeSH terms enriched for the hub genes in the brown and black modules. The MeSH term “Chicken Embryo” in the Anatomy (A) category (MeSH: D002642) was enriched in the brown module and this term has the highest number of unique curated literature sources (4836) relating to the background genes of the brown module. Another MeSH term “Gene Expression Regulation, Developmental” (MeSH: D018507) was also significantly enriched in both the brown and black modules. These results depict the scenario that the module hub genes are involved in gene regulation of chicken embryogenic development. The “Feathers” MeSH term (MeSH: D005241) of the Anatomy (A) category was enriched in the black (feather trait) module hub genes while it is not enriched in brown (scale trait) module genes. These findings validate the WGCNA module detection approach based on trait correlation and the effectiveness of our strategy indicating that the black module hub genes specifically contribute to feather structure during chicken embryogenesis. It is notable that the “MicroRNAs” term (MeSH: D035683) was found to be significant and has the most (1700) unique curated literature sources relating to the background genes in the Chemicals and Drugs (D) category for the black module hub genes.
We selected all edges (gene connections) with nodes (genes) having Gene Symbol annotations, then collected all unique nodes with the frequency calculation. There are 785 and 400 unique genes having at least two edges for the brown and black modules, respectively. The highest number of edges for the hub genes in the brown module is 653, while the highest number of edges for the hub genes in the black module is 328. We also found that there are 20 and 10 EDRGs in the brown and black modules, respectively (Supplemental Table 3). We ranked these EDRGs based on the number of edges (edge connectivity) in the modules. For each module, more than half of the EDRGs are among the top 50% of all nodes with the most connectivity in each module. Furthermore, three claw β-keratin EDRGs in the brown module and the retinoic acid receptor beta (RARB) gene in the black module ranked among the top 5% of edge connectivity among all the other nodes. Most of the EDRGs in brown or black modules have at least 20 connections and only one EDRG in each module has the minimum two connections. Therefore, EDRGs in these highly correlated epidermal traits-related modules have high connectivity and likely play an important role in chicken epidermal embryogenesis (Supplemental Table 3).
Discussion
In this study, we did not employ the traditional method of using differentially expressed genes to construct the co-expression network. Typically, researchers will screen their datasets to remove genes that are not differentially expressed or lack annotation (Miller et al. 2014; Maschietto et al. 2015; Wong et al. 2015). In contrast, Hudson et al. (2009) used their entire dataset and found that modifications of transcription factors (TF) such as reversible phosphorylation can act independently of the TF expression levels. Similarly, we took advantage of this method and used a large number of microarray datasets (31 arrays) and we were able to include most of the available expression data on the 44K microarray chips and build a more robust co-expression network related to epidermal developmental traits. The detection of co-expressed modules related to phenotypic traits can facilitate the identification of grouped genes and their regulatory mechanisms. The workflow of this study is summarized in Fig. 4.
Unlike quantitative traits such as body weight, length, etc., the qualitative traits are difficult to measure and to build a Module-Trait relationship. Based on previous work defining the embryonic stages of the chicken (Hamburger and Hamilton 1951), we used the strategy of 1 for positive and 0 for negative of Böhne et al. (2014) to discover the modules related to the scale or feather traits. Figure 1 not only shows the correlation of the scale and feather traits to the clustered module genes, it also shows correlation with other non-quantitative traits, such as stages (day 8, day 17, and day 19), which will be studied in the future.
Although 11 modules meet the basic correlation threshold (r > 0.5) for Module-Trait and MM-GS relationships, only the brown module for the scale trait and the black module for the feather trait have very strong correlations (r > 0.9). Therefore, we focused on analyzing these two EDRG modules, which are highly correlated to avian epidermal development. Chang et al. (2015) applied a cosine similarity algorithm to identify the differentially expressed/coregulated genes in chicken developing epidermal tissue and found five significantly enriched KEGG pathways: ECM-receptor interaction, Focal adhesion, Melanogenesis, Calcium signaling pathway, and Vascular smooth muscle contraction. Their further verification work found that voltage-gated calcium channel subunits were expressed spatio-temporally in the epithelium and this strongly suggests that the calcium signaling pathway is involved in early skin development as a novel pathway. Besides the ECM-receptor interaction pathway, all the other four KEGG pathways were also enriched in our analysis. Our first KEGG pathway analysis, using an earlier version of KOBAS (2.0), failed to identify any enriched pathways for the black module because of the missing Entrez Gene ID annotations. This may also explain the missing ECM-receptor interaction pathway enrichment. Although the enriched GO terms are numerous and complicated, we are able to identify informative terms such “skin development,” “embryo development ending in birth or egg hatching,” and “morphogenesis of embryonic epithelium” that legitimizes tissue type in our analysis. Interestingly, we found that Tenascin C (TNC) is involved in an enriched Gene Ontology term (GO:0070161). Previous studies have shown that TNC is dynamically expressed and alters the cell adhesiveness during feather and scutate scale development (Fischer et al. 1997; Shames et al. 1994; Tucker 1991). Furthermore, TNC was also discovered to be a possible miRNA target gene during epidermal development in the chicken embryo (Bao et al. 2016).
The traditional KEGG pathway and Gene Ontology analysis is based on the established and curated pathways and gene database. However, there are no miRNAs or beta-keratins involved in any chicken KEGG pathways. Considering our WGCNA modules contain a large number of these genes, we did not find it appropriate to only perform the KEGG pathway and Gene Ontology analyses and we therefore additionally utilized performed MSET analysis. MSET employs a simple randomization test (Eisinger et al. 2013) allowing comparison of the microarray results with independently curated gene sets that are not commonly associated or categorized in other enrichment tools. In other words, the background genes for the enrichment are customized based on the research interests. In our case, the customized gene set is the EDRGs. It is interesting that the brown module for the scale trait has five β-keratins enriched as the top microarray genes. Considering β-keratins are the major structural genes for avian scales and the MSET analysis is sensitive to the gene significance result, this indicates the trait-specific modules heavily utilize this specific type of β-keratin.
Topologically, central genes in the co-expression network, the hub genes, have more internode connections. It is reasonable that hub genes are more likely to be required for network integrity and play more important regulatory functions. Therefore, the identification and characterization of hub genes in the network is helpful to understanding the basis of the epidermal development network. However, we cannot simply input the hub genes of a module to perform the KEGG pathway enrichment analysis as the results may not be fruitful because the lack of other non-hub genes associated with pathways may heavily influence the enrichment results. Therefore, we ran the KEGG pathway analysis using KOBAS 3.0 on all the hub genes in black module and brown module and compared it to the analysis with all of the module genes. The enrichment results for all genes in black and brown modules produced a total of 19 enriched pathways while there was no enriched pathway for the hub genes in black module and only one “Ribosome” KEGG pathway enriched for the hub genes in the brown module. The traditional enrichment methods (KEGG pathway and GO enrichment) were not useful in identifying the functional role of modular hub genes.
In contrast to the enriched MeSH Anatomy term “Feathers” (D005241) for the black module with the feather trait, we did not find the “Scale” MeSH term enriched in the brown module based on the scale trait, because there is no anatomical or epidermal term “Scale” deposited in the NLM. However, the brown module hub genes may still be important for the formation of the scale, but are not detectable. Datasets such as the brown module hub genes may be candidates to be deposited in the NLM to improve its number of MeSH terms.
Besides the “Feathers” term enriched in the black module, there are hub genes in the black and brown modules that are enriched for the MeSH Anatomy (A) category, which are related to epidermal development, such as “Wing” (D014921), “Hindlimb” (D006614), and “Limb Buds” (D018878). During embryonic days 9 to 16, the feather buds undergo a complicated process to eventually become the feather follicles, which involves the connection to nerve fibers and muscles to provide physiological functions (Yu et al. 2002, 2004). Interestingly, we found that many muscle or nerve-related MeSH terms were enriched either for the Chemicals and Drugs (D) category or Phenomena and Processes (G) category such as “Muscle Contraction” (D009119) and “Nerve Tissue Proteins” (D009419). In addition, we found that the MeSH term “Thyroxine” (D012269) is enriched with a high p value in the brown module D category. Thyroxine initiates epidermal thickening, secondary periderm formation and even contributes to the cornification of embryonic chicken skin in culture (Tammi and Maibach 1987). The classic “Wnt Signaling Pathway” (D060449) is enriched as the top ranked G term in the black module, and some other enriched MeSH terms such as “Signal Transduction” (D015398) indicate that the signaling transduction pathways are involved, but the data are too general for annotation.
We noticed that there are two genes of the SMADs protein family present in our customized feather-related gene set, and that the SMAD5 protein was another gene family member enriched as MeSH D category in the brown module. Ng et al. (2015) conducted a transcriptome analysis of regenerating adult feathers of the chicken and found that SMAD5 was significantly enriched as one of the differentially expressed genes in multiple functional enrichment annotations. Considering our MeSH enrichment results, we suggest that SMAD5 is involved in signaling pathway regulation as early as the embryonic developmental stages examined here. Lowe et al. (2015) curated and collected the feather development-related genes with specific standards such as feather phenotype mutation or defined region of the feather, thus it is inevitable that they missed some genes contributing to epidermal development, such as those found by Ng et al. (2015) in their transcriptome analysis. Therefore, our MeSH enrichment results based on module hub genes may provide a new approach for selection of candidate genes involved in avian epidermal development.
We also found that two TF genes (specific protein 1 [Sp1], specific protein 3 [Sp3]) are enriched by MeSH. Ng et al. (2015) found that Sp3 was significantly upregulated in the late body feather development. Sp1 was predicted by Ingenuity Pathway Analysis (IPA) canonical pathway analysis to be an upstream regulator gene contributing to the gene expression differences between distal and proximal body feathers in the chicken. These results together with other pathway enriched terms suggest that module hub genes play important roles in TF regulation to coordinate with the biological signaling cascade process of chicken epidermal embryogenesis. This research has utilized the co-expression network and various enrichment strategies to characterize the most highly featured gene modules instead of exhaustively listing every single ontology. The entire gene regulatory network is very complicated when we consider the thousands of target gene possibilities produced by TF and miRNA genes. Hopefully, this study will shed light on our understanding of the co-expression networks and the important role of hub genes in the featured modules.
Conclusions
The detection of chicken co-expression network modules during embryonic epidermal development in the chicken is useful for understanding the relatedness between modules and the traits via customized enrichment methods. Specifically, for the modules with strong correlations of high Module-Trait and MM-GS values, the MSET enrichment results demonstrate the important functional role of epidermal-related genes in both whole genome and modular datasets. The novel application of MeSH enrichment for identifying the hub genes, which have more gene connections, allows for the discovery of their functional roles in the formation of chicken epidermal structures.
References
Andl T, Reddy ST, Gaddapara T, Millar SE (2002) WNT signals are required for the initiation of hair follicle development. Dev Cell 2:643–653
Baden HP, Maderson PF (1970) Morphological and biophysical identification of fibrous proteins in the amniote epidermis. J Exp Zool A Ecol Genet Physiol 174:225–232
Bao W, Greenwold MJ, Sawyer RH (2016) Expressed miRNAs target feather related mRNAs involved in cell signaling, cell adhesion and structure during chicken epidermal development. Gene 591:393–402
Barabasi AL, Oltvai ZN (2004) Network biology: understanding the cell’s functional organization. Nat Rev Genet 5:101–113
Bell E, Thathachari YT (1963) Development of feather keratin during embryogenesis of the chick. J Cell Biol 16:215–223
Benjamini Y, Hochberg Y (1995) Controlling the false discovery rate: a practical and powerful approach to multiple testing. J R Stat Soc Ser B Methodol 57:289–300
Böhne A, Sengstag T, Salzburger W (2014) Comparative transcriptomics in East African cichlids reveals sex-and species-specific expression and new candidates for sex differentiation in fishes. Genome Biology and Evolution 6:2567–2585
Carey VJ, Gentry J, Whalen E, Gentleman R (2005) Network structures and algorithms in Bioconductor. Bioinformatics 21:135–136
Chang CH, Jiang TX, Lin CM, Burrus LW, Chuong CM, Widelitz R (2004) Distinct Wnt members regulate the hierarchical morphogenesis of skin regions (spinal tract) and individual feathers. Mech Dev 121:157–171
Chang KW, Huang NA, Liu IH, Wang YH, Wu P, Tseng YT, Hughes MW, Jiang TX, Tsai MH, Chen CY, Oyang YJ (2015) Emergence of differentially regulated pathways associated with the development of regional specificity in chicken skin. BMC Genomics 16:22
Chuang CL, Jen CH, Chen CM, Shieh GS (2008) A pattern recognition approach to infer time-lagged genetic interactions. Bioinformatics 24:1183–1190
Chuong CM, Chen HM, Jiang TX, Chia J (1991) Adhesion molecules in skin development: morphogenesis of feather and hair. Ann N Y Acad Sci 642:263–280
Eisinger BE, Saul MC, Driessen TM, Gammie SC (2013) Development of a versatile enrichment analysis tool reveals associations between the maternal brain and mental health disorders, including autism. BMC Neurosci 14:147
Featherstone DE, Broadie K (2002) Wrestling with pleiotropy: genomic and topological analysis of the yeast gene expression network. BioEssays 24:267–274
Fischer D, Tucker RP, Chiquet-Ehrismann R, Adams JC (1997) Cell-adhesive responses to tenascin-C splice variants involve formation of fascin microspikes. Mol Biol Cell 8:2055–2075
Gill FB (1995) Ornithology. Macmillan, London: W. H Freeman and Company New York. https://books.google.com/books?hl=en&lr=&id=jFfs1jsPfwgC&oi=fnd&pg=PR15&dq=Gill+FB+(1995)+Ornithology&ots=TaF-F3DS8N&sig=LLQo3GG3jXeLAchnRIyMr9V-y_U#v=onepage&q&f=false
Greenwold MJ, Bao W, Jarvis ED, Hu H, Li C, Gilbert MT, Zhang G, Sawyer RH (2014) Dynamic evolution of the alpha (α) and beta (β) keratins has accompanied integument diversification and the adaptation of birds into novel lifestyles. BMC Evol Biol 14:249
Haake AR, König G, Sawyer RH (1984) Avian feather development: relationships between morphogenesis and keratinization. Dev Biol 106:406–413
Hamburger V, Hamilton HL (1951) A series of normal stages in the development of the chick embryo. J Morphol 88:49–92
Harris MP, Fallon JF, Prum RO (2002) Shh-Bmp2 signaling module and the evolutionary origin and diversification of feathers. J Exp Zool 294:160–176
Hickner PV et al (2015) Whole transcriptome responses among females of the filariasis and arbovirus vector mosquito Culex pipiens implicate TGF-β signaling and chromatin modification as key drivers of diapause induction. Functional & Integrative Genomics 15:439–447
Horvath S, Zhang B, Carlson M, Lu KV, Zhu S, Felciano RM, Laurance MF, Zhao W, Qi S, Chen Z, Lee Y (2006) Analysis of oncogenic signaling networks in glioblastoma identifies ASPM as a molecular target. Proc Natl Acad Sci 103:17402–17407
Horvath S, Dong J (2008) Geometric interpretation of gene coexpression network analysis. PLoS Comput Biol 4:e1000117
Hu ZL, Bao J, Reecy JM (2008) CateGOrizer: a web-based program to batch analyze Gene Ontology classification categories. Online Journal of Bioinformatics 9:108–112
Hudson NJ, Reverter A, Wang Y, Greenwood PL, Dalrymple BP (2009) Inferring the transcriptional landscape of bovine skeletal muscle by integrating co-expression networks. PLoS One 4:e7249
Jiang TX et al (2004) Integument pattern formation involves genetic and epigenetic controls: feather arrays simulated by digital hormone models. The International Journal of Developmental Biology 48:117
Kanehisa M, Goto S (2000) KEGG: Kyoto encyclopedia of genes and genomes. Nucleic Acids Res 28:27–30
Langfelder P, Horvath S (2008) WGCNA: an R package for weighted correlation network analysis. BMC Bioinformatics 9:559
Langfelder P, Mischel PS, Horvath S (2013) When is hub gene selection better than standard meta-analysis? PLoS One 8:e61505
Li X, Chiang HI, Zhu J, Dowd SE, Zhou H (2008) Characterization of a newly developed chicken 44K Agilent microarray. BMC Genomics 9:60
Lowe CB, Clarke JA, Baker AJ, Haussler D, Edwards SV (2015) Feather development genes and associated regulatory innovation predate the origin of Dinosauria. Mol Biol Evol 32:23–28
Maderson PF, Hillenius WJ, Hiller U, Dove CC (2009) Towards a comprehensive model of feather regeneration. J Morphol 270(10):1166–1208
Maschietto M et al (2015) Co-expression network of neural-differentiation genes shows specific pattern in schizophrenia. BMC Med Genet 8:23
Miller JA et al (2014) Transcriptional landscape of the prenatal human brain. Nature 508:199–206
Morota G, Beissinger TM, Peñagaricano F (2016) MeSH-informed enrichment analysis and MeSH-guided semantic similarity among functional terms and gene products in chicken. G3: Genes| Genomes| Genetics 6:2447–2453
Ng CS, Wu P, Fan WL, Yan J, Chen CK, Lai YT, Wu SM, Mao CT, Chen JJ, Lu MY, Ho MR (2014) Genomic organization, transcriptomic analysis, and functional characterization of avian α-and β-keratins in diverse feather forms. Genome Biology and Evolution 6:2258–2273
Ng CS, Chen CK, Fan WL, Wu P, Wu SM, Chen JJ, Lai YT, Mao CT, Lu MY, Chen DR, Lin ZS (2015) Transcriptomic analyses of regenerating adult feathers in chicken. BMC Genomics 16:756
Rogers GE (1985) Genes for hair and avian keratins. Ann N Y Acad Sci 455:403–425
Schäfer J, Strimmer K (2005) An empirical Bayes approach to inferring large-scale gene association networks. Bioinformatics 21:754–764
Shames RB, Bade BC, Sawyer RH (1994) Role of epidermal–dermal tissue interactions in regulating tenascin expression during development of the chick scutate scale. J Exp Zool A Ecol Genet Physiol 269:349–366
Steffen M, Petti A, Aach J, D’haeseleer P, Church G (2002) Automated modelling of signal transduction networks. BMC Bioinformatics 3:34
Stuart JM, Segal E, Koller D, Kim SK (2003) A gene-coexpression network for global discovery of conserved genetic modules. Science 302:249–255
Tammi R, Maibach H (1987) Skin organ culture: why? Int J Dermatol 26:150–160
Thieffry D, Huerta AM, Pérez-Rueda E, Collado-Vides J (1998) From specific gene regulation to genomic networks: a global analysis of transcriptional regulation in Escherichia coli. BioEssays 20:433–440
Tsuyuzaki K, Morota G, Ishii M, Nakazato T, Miyazaki S, Nikaido I, MeSH ORA framework (2015) R/Bioconductor packages to support MeSH over-representation analysis. BMC Bioinformatics 16:45
Tucker RP (1991) The sequential expression of tenascin mRNA in epithelium and mesenchyme during feather morphogenesis. Roux’s Archives of Developmental Biology 200:108–112
Van Noort V, Snel B, Huynen MA (2003) Predicting gene function by conserved co- expression. Trends Genet 19:238–242
Wang J et al (2014) Metabolomic analysis of the salt-sensitive mutants reveals changes in amino acid and fatty acid composition important to long-term salt stress in Synechocystis sp. PCC 6803. Functional & Integrative Genomics 14:431–440
Widelitz RB, Jiang TX, Chen CW, Stott NS, Chuong CM (1999) Wnt-7a in feather morphogenesis: involvement of anterior-posterior asymmetry and proximal-distal elongation demonstrated with an in vitro reconstitution model. Development 126:2577–2587
Wong RY, Melissa SL, John G (2015) Characterizing the neurotranscriptomic states in alternative stress coping styles. BMC Genomics 16:425
Xie C, Mao X, Huang J, Ding Y, Wu J, Dong S, Kong L, Gao G, Li CY, Wei L (2011) KOBAS 2.0: a web server for annotation and identification of enriched pathways and diseases. Nucleic Acids Res 39:W316–W322
Xue Z et al (2013) Genetic programs in human and mouse early embryos revealed by single-cell RNA sequencing. Nature 500:593–597
Yu M, Wu P, Widelitz RB, Chuong CM (2002) The morphogenesis of feathers. Nature 420:308–312
Yu M, Yue Z, Wu P, Wu DY, Mayer JA, Medina M, Widelitz RB, Jiang TX, Chuong CM (2004) The developmental biology of feather follicles. The International Journal of Developmental Biology 48:181
Zhang B, Horvath S (2005) A general framework for weighted gene co-expression network analysis. Stat Appl Genet Mol Biol 4:1128
Zhou X, Kao MC, Wong WH (2002) Transitive functional annotation by shortest-path analysis of gene expression data. Proc Natl Acad Sci 99:12783–12788
Acknowledgements
Authors are thankful to Dr. Richard L. Goodwin (University of South Carolina, School of Medicine) for kindly providing chicken embryos and Dr. Diego Altomare for performing microarray experiments. The microarray work was supported by the National Institute of General Medical Sciences (grant number 8 P20 GM103499) and the National Center for Research Resources (grant number 5 P20 RR016461).
Author information
Authors and Affiliations
Corresponding author
Electronic supplementary material
Supplemental Table 1
We apply a binary system (1 for positive and 0 for negative) to define and quantify the qualitative traits to measure the external trait based on the microarray expression profiles. This is the spreadsheet of this particular trait-defining strategy for 31 samples (XLSX 10 kb)
Supplemental Table 2
For the scale and feather traits, we list all modules having moderate Module-Trait correlations (r > 0.5, p < 0.01) individually, we also highlighted the modules also having moderate MM-GS correlations (r > 0.5, p < 0.01) (XLSX 10 kb)
Supplemental Table 3
Demonstrates all epidermal development-related genes (EDRGs) in brown and black modules. We also list the number of the edges (connectivity) for these genes. The rank of these EDRGs based on the connectivity number among all genes having gene symbol annotation and the top percentage were listed (XLSX 10 kb)
Supplemental Fig. 1
Illustrates the sample clustering based on individual sample expression profiles. Each array sample was named as the tissue type with the days of embryonic stages. The y-axis (height) is the distance metric calculated by average linkage hierarchical clustering method. There are no outliers and most of the samples with the same tissue type and embryonic stage cluster together (PNG 72 kb)
Supplemental Fig. 2
We performed the analysis of network topology to facilitate choosing soft threshold power β for constructing the WGCNA network. The left panel demonstrates the scale-free topology fit index (vertical axis) as the soft threshold power β (horizontal axis) varies. The right panel displays the mean connectivity (degree, vertical axis) as the soft threshold power β (horizontal axis) varies. The soft threshold power was selected based on the criterion of approximate scale-free topology which has high scale-free topology fit index (normally above 0.8) and the saturation of the mean connectivity was reached by the lowest power β (PNG 66 kb)
Supplemental File 1
To incorporate the external traits, gene significance (GS) of the genes having Entrez Gene ID annotations for Feather (“Feather trim” worksheet) and Scale (Scale trim worksheet) traits were sorted based on the absolute GS value. We also list the corresponding module color and the p value of GS (XLSX 4447 kb)
Supplemental File 2
We performed the KEGG pathway and Gene Ontology enrichment analysis individually for 11 modules having both correlated Module-Trait and MM-GS relationships (red color modules in Supplemental Table 2). The 2 worksheets listed all significantly enriched KEGG pathways and GO annotations with details based on the corrected p value (XLSX 10 kb) (XLSX 337 kb)
Rights and permissions
About this article
Cite this article
Bao, W., Greenwold, M.J. & Sawyer, R.H. Using scale and feather traits for module construction provides a functional approach to chicken epidermal development. Funct Integr Genomics 17, 641–651 (2017). https://doi.org/10.1007/s10142-017-0561-0
Received:
Revised:
Accepted:
Published:
Issue Date:
DOI: https://doi.org/10.1007/s10142-017-0561-0