Abstract
Effective water quality management requires careful consideration of pollutant fate and transport, proper estimation of non-point source loadings, and maximum allowable allocation of point source discharges. A decision support system (DSS) that addresses all these issues is developed in this study by embedding mass-balance expressions, GIS, and a remote-sensing-based non-point source loading scheme into a hybrid goal-programming approach and is applied to the rapidly growing Arroyo Colorado River watershed along the US–Mexico border. The model components were favorably evaluated against field data and previous studies. The DSS was used to evaluate the carrying capacity of the river, defined based on the water quality standards for biochemical oxygen demand, dissolved oxygen, and minimum in-stream flow requirements. The results indicated that on a macro-scale, the current stresses utilize about 40 % of the maximum carrying capacity. However, the most upstream and downstream sub-watersheds are currently over stressed and need to reduce their loadings. The assimilative capacity of the river is not sufficient to carry current flows at their permitted discharge concentrations implying an inequity among discharges with regard to treatment cost burden. Sensitivity analyses indicated that the carrying capacity was more affected by policy choices made for water quality standards then where they were to be enforced (i.e., compliance locations). Urban areas currently cover 13 % of the watershed but contribute nearly 45 % of the total non-point source loadings. Therefore, the urbanization in this watershed must be carefully planned with emphasis on stormwater treatment and management to sustain this valuable resource for future generations.
Similar content being viewed by others
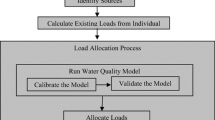
Avoid common mistakes on your manuscript.
Introduction
The promulgation of the North Atlantic Free Trade Agreement and associated policies has spurred significant economic development and population growth along the US–Mexico border region. The Arroyo Colorado River, depicted in Fig. 1, is a distributary of the Rio Grande River. The Arroyo Colorado runs along the economically important South Texas–Mexico border region (inset map) and is home to several rapidly developing cities such as Harlingen, McAllen, and Mission whose populations are expected to double in the next decade or so. The river drains into the ecologically sensitive region of the Laguna Madre, an estuarine wetland system along the Gulf of Mexico and a common stopping ground for migratory birds. The watershed is home to over 40 endangered and exotic species, and the near stream habitats provide unique eco-tourist attractions as well as diverse cultural experiences and contribute to the overall economy of the region.
Being in a fertile deltaic plain, the Arroyo Colorado River watershed traditionally has had a strong agricultural base and has been a leading producer of citrus, sugarcane, and cotton crops in the United States. Over 90 % of the nearly 333,000 acres (134,760 ha) of the watershed are irrigated croplands. Urbanization in recent years has, however, led to increased water transfers from agriculture to urban uses (RGRWPG 2011). These shifts are altering the nature, location, and extent of wastewater loadings into the river. Also, the watershed is currently experiencing increased loadings from urban runoff causing the cities in the region to begin developing the necessary policies and infrastructure to effectively manage their stormwater as they continue to grow in size.
Historically, the Arroyo Colorado River was used to divert and route floodwaters from the Rio Grande River to avoid flooding in downstream cities like Brownsville and Matamoras, Mexico. However, factors such as increased water use in both the US and Mexico, construction of reservoirs, and recent drought conditions have significantly curtailed downstream flows in the Rio Grande River (IBWC 2005). As a result, in recent times, the flows in the Arroyo Colorado River are mainly sustained by wastewater discharges from cities along its length and augmented by irrigation return flows and stormwater runoff.
Improper wastewater management practices in this under-served region have caused severe water quality problems, and sections of the river have experienced poor water quality with regard to dissolved oxygen, bacteria, and algae (TCEQ 2006a). The tidal segment of the river is included in the 303(d) list of the impaired water bodies for dissolved oxygen (DO) levels below the criteria specified in the Texas surface water quality standards (TCEQ 2008; TCEQ 2010). The increased anthropogenic activities in the upper non-tidal segment are hypothesized to be the cause for this impairment (Raines and Miranda 2002; Hernandez 2007).
The socio-economic, cultural, and environmental importance of the Arroyo Colorado River has been recognized in recent times. A multi-stakeholder “Arroyo Colorado Watershed Partnership” (ACWP) has been formed with the mission to reduce the pollutant additions to the river to the maximum extent possible to meet the state water quality standards and improve natural terrestrial, aquatic, and riparian habitats (ACWP 2007). The watershed partnership consists of representatives from local, regional, and state regulatory agencies as well as those representing municipal, industrial, agricultural, and environmental interests. In addition, the cities in the region have established a taskforce to address stormwater issues.
Sustainable development calls for reconciling the competing objectives of economic growth and environmental protection. From a stream water quality standpoint, this idea translates to balancing the increased wastewater discharges from urbanization and population growth against the water quality and quantity needs of in-stream and other ecological receptors. Identification of areas in the watershed where the river has available assimilative capacity and sections that have reached or are close to their critical limits is also useful to foster smart regional-scale growth (Pollard 2000; ICCMA and SGN 2006; Ewing et al. 2007) and evaluate policy and engineering options like effluent trading and construction and upgrading treatment plants to manage wastewater in a holistically efficient manner (Mujumdar and Saxena 2004; Malano and Davidson 2009). Watershed-scale decision support systems (DSS) that facilitate scientifically credible, risk-informed dialog among different stakeholders, and help them understand current conditions and evaluate policy and engineering options in a practical, easy-to-use, and understandable manner are vital to translate the vision of multi-stakeholder groups such as ACWP into reality and foster consensus-oriented and cost-effective solutions (Korfmacher 1998; Korfmacher 2001; Hernandez and Uddameri 2010; Webler et al. 2011).
The primary focus of this study was to develop a DSS to evaluate the impacts of point and non-point biochemical oxygen demand (BOD) loadings on the DO levels in the river. The DSS is developed by integrating pollutant mass-balances with a Geographic Information System (GIS) and remote-sensing-based non-point source (NPS) pollutant loading estimation scheme which are then linked to a hybrid goal-programming-based optimization model. The DSS can be used to simulate BOD and DO concentrations in the river and to identify maximum allowable loadings and wastewater load reductions in the system. The development of the DSS and its implementation to answer key sustainability-related questions are illustrated in this paper. In addition to addressing critical water quality questions specific to the Arroyo Colorado River watershed, the proposed DSS methodology is generic and can be adapted to other watersheds facing similar issues.
Methodology
Study area delineation and conceptual model
The study area of interest is the non-tidal segment of the Arroyo Colorado River watershed depicted in Fig. 1 along the US–Mexico border (Fig. 1, inset). The headwater of the river starts near the City of Mission and the non-tidal segment extends to the City of San Benito on the eastern side of the watershed. There are 34 permitted wastewater dischargers, also referred to as permit compliance systems (PCS), in the segment of interest of which 23 have direct outfalls into the river or a drainage ditch near the river. Of these, 14 are major dischargers that are currently active and account for over 95 % of all the point source discharges in the watershed and are listed in Table 1. Two cities, Donna and Weslaco, discharge into the river at the same confluence point and are treated as one aggregated discharge (Donna–Weslaco) in this study.
The wastewater treatment plants typically utilize activated sludge-type treatment systems, with the exception of the City of San Benito which, until recently, used a pond system (TCEQ 2006a). Most discharge permits currently require primary and secondary treatment and regulate carbonaceous biochemical oxygen demand releases into the river. However, as seen from Table 1, some dischargers are discharging above their permitted concentration levels. In addition, there are several small-scale domestic wastewater treatment facilities that treat less than 0.15 million gallons per day (MGD) or 568 m3/day using mechanisms such as on-site wastewater and low flow systems. These systems do not directly contribute to pollutant loadings into the river and any indirect inflows (via leaching into and subsequent discharge from groundwater) are minimal (TCEQ 2006a) and as such not included in this analysis.
The land use in the watershed is varied but nearly 75 % agricultural. Therefore, in addition to the point dischargers identified above, BOD in the river is also affected by the organic matter in irrigation return flows and stormwater runoff from both agricultural and urban areas. For the most part, the NPS loadings from both these sources drain into the river through a labyrinth of drainage ditches that crisscross the watershed (Stubbs et al. 2005) and as such, these two sources are lumped and treated as a single NPS contribution. On a watershed scale, the NPS contributions will tend to be non-uniform as the precipitation varies from nearly 20 inches per year in the western sections to over 30 inch (76.2 cm) per year in the east (NCDC 2006). In addition, the NPS pollutant loadings will also vary at the sub-watershed scale due to variations in land use (Charbeneau and Barrett 1998). To capture the variability in the NPS loadings, the watershed area of interest was divided into 12 sub-watersheds whose outlets coincided with the confluence points of the major point source discharges as depicted in Fig. 2. Details with regards to quantifying NPS loadings are presented in a subsequent section following the discussion on modeling point sources.
Modeling point source discharges
The confluence point where discharges from the point source meet the river is modeled as a zero-volume continuous flow-stirred tank reactor (Chapra 1997). A steady-state mass-balance with no reaction terms was used to estimate the BOD concentrations and DO deficit at the confluence point (e.g., Point B in Fig. 3). The total flow at the confluence point was obtained via a steady-state mass-balance
where Q is the flowrate (m3/day), BOD is the concentration of the BOD (g/m3), O is the oxygen concentration (g/m3), O s is the saturated DO concentration (g/m3), and D is the oxygen deficit (g/m3). The subscripts B and pcs refer to values at point B and from the PCS, respectively. Similarly, the subscripts A–B and B–C refer to the upstream and downstream reaches around confluence point B.
The transport of BOD and the oxygen deficit (D) in the river segment (e.g., B–C) between any two confluence points of interest (e.g., B and C) was modeled using a steady-state, one-dimensional transport equation considering advection and first-order decay
where U is the velocity (m/s), a and b are empirical coefficients, k d is the lumped first-order deoxygenation rate (1/day), k r is the lumped reaeration rate (1/day), and x is the distance measure in the segment with value equal to zero at the beginning of the segment. The subscripts B and B–C refer to the confluence point B and segment B–C. The solutions to the above differential equations can be obtained analytically and can be found in Chapra (1997) and therefore not repeated here. The mass-balance equations were sequentially applied starting with the headwaters of the river and were linked using the superposition principle.
While it is acknowledged that the simulation model does not include several processes such as sediment oxygen demand and influences of vegetation on DO, the formulation and complexity is consistent with the available data and recommendations of keeping the water quality management models simple to minimize uncertainty (NRC et al. 2001). Furthermore, this type of formulation has a long history of application starting with Streeter and Phelps (1925), and is considered a standard approach for waste load allocation and management studies (Arbabi and Elzinga 1975; Burn and McBean 1985; Burn 1989). In recent years, there has been a renewed interest in using BOD/DO models for water quality management (Lung and Sobeck 1999; Takyi and Lence 1999; Vasquez et al. 2000; Mujumdar and Sasikumar 2002; Lindenschmidt 2006; Nas and Nas 2009). Therefore, the use of the adopted modeling scheme is considered reasonable for the purposes of this application.
In the Arroyo Colorado River, discharges from PCSs are a significant component of the overall flow in many reaches. Non-uniform flow conditions are to be expected because variable point and NPS discharges are added to the river as it flows downstream. The importance of incorporating non-uniform flow conditions during water quality assessments has been emphasized in the literature (Cardwell and Ellis 1993; Murty et al. 2006). Therefore, non-uniform flows were simulated using the superposition principle. However, the flows were assumed to be quasi-uniform and held constant within a given stream reach for mathematical tractability. This assumption is commonly invoked in water quality models such as QUAL2E (Brown and Barnwell 1987) and as such deemed reasonable for the purposes of this study. To obtain velocities from quasi-uniform flows, Manning’s equation is commonly adopted (Chapra 1997). However, this study employed a different approach because the Arroyo Colorado River does not conform to a regularly shaped geometry. An empirical power-law expression (Leopold and Maddock 1953; Thomann and Mueller 1987) was used to calculate velocities in each segment required to model BOD/DO advection (Eq. 5). The coefficients of the power-law expressions were obtained by calibrating the model using synoptic flow and velocity measurements obtained as part of a total maximum daily load (TMDL) study that was carried out by the Texas Commission for Environmental Quality (TCEQ) during the years 2000–2002 (TCEQ 2006b).
Modeling NPS discharges
A variety of approaches are available to model NPS discharges including the use of databases, regression equations, unit load, and flow-concentration approaches (Marsalek 1991). For planning and assessment endeavors, it is generally recommended that parsimonious models be adopted in favor of more complex formulations (Reckhow et al. 1985; Mitchell 2005). Therefore, a semi-distributed modeling scheme was chosen to estimate NPS loadings. The NPS loadings are modeled as the product of discharge (runoff) and the event mean concentration (EMC) which is the average concentration of the pollutant in the runoff during a storm event. In addition to its simplicity, the reasonableness of the approach has been empirically verified for planning purposes (Mitchell 2005; Behera et al. 2006) and facilitated by the availability of EMC values for different land use types in different regions (Lin 2004).
The first step toward modeling NPS discharges using the EMC approach is to obtain reasonable estimates for runoff and BOD loadings at different locations within the watershed. As stated previously, the rainfall variability within the watershed could be considerable and must be properly accounted for to obtain realistic NPS loading estimates. In recent years, remotely sensed moisture measurements from the next-generation radar (NEXRAD) have become available within the watershed and were utilized in this study. Stage-II NEXRAD measurements were employed to correct for local biases as well as the mean field bias in rainfall measurements (Briendenbach et al. 1998). A Visual Basic for Applications code was written to calculate interstorm duration and storm intensities using hourly data (Hernandez 2007). The corresponding runoff for individual events were then computed using the modified rational method (Di Toro 1979). Again the selection of this method was based on factors including consistency with the application objectives, ease of implementation, prior use, acceptability, and reasonableness of estimates.
For each runoff producing storm event, the event mean runoff corresponding to each land use category within each sub-watershed was computed using the modified rational method (Di Toro 1979) and multiplied with the corresponding EMC to obtain the event mean loading for each land use category. These event mean loadings were then summed to obtain the total NPS loading for the sub-watershed corresponding to a storm event. The loadings from different storm events were used to obtain an average, steady-state NPS loading for each sub-watershed using the approach suggested by Di Toro (1979) and presented in Thomann and Mueller (1987).
Having estimated the spatially distributed NPS loadings, the next step was to integrate point and non-point loadings into a unified modeling framework. The integration was achieved by discretizing the non-point loads as a set of equivalent point loads. Following the group response unit concept (Kouwen 1988), each sub-watershed was treated as a discrete unit and the aggregated NPS loadings from the different land use types was assigned at the centroid of the sub-watershed and vertically projected on to the river. This “equivalent point load” approach was adopted because it provided a conceptually simple yet robust method to model NPS loadings without increasing the computational burden (León et al. 2001). Furthermore, as the point source loading model is linear, this equivalent point load model provided the same response as that of a more discretized version (i.e., where the non-point loading is divided into multiple equivalent point loads) as long as all these point loads are equally spaced along the segment of the river within each sub-watershed.
Goal-programming optimization model
Linear and nonlinear optimization models with deterministic or stochastic constraints are commonly used in waste load allocation studies (e.g., Revelle et al. 1968; Lohani and Thanh 1979; Burn and McBean 1985; Sasikumar and Mujumdar 1998; Vasquez et al. 2000; Murty et al. 2006; Qin et al. 2009). A hybrid goal-programming approach was adopted here. As the primary goal of this study was to identify how much additional point source loadings (due to urbanization) can be added into the river, the allowable discharge at each treatment plant was represented as the sum of current flowrates, Q PCS,j , and deviational variables (Z + and Z −)
The positive deviational variable Z + represents the additional wastewater flow that can be discharged from the wastewater treatment plant (j), while Z − (the negative deviational variable) is the amount of wastewater flow that must be curtailed at the wastewater treatment plant (j) to meet water quality objectives in the river. The objective, therefore, seeks to maximize the positive deviations at the treatment plants and minimize the negative deviations at the same time. Hence, the objective function was specified as the maximization of the differences between the positive and the negative deviational variables summed over all wastewater treatment plant locations. This maximization was, however, constrained to insure that the concentrations of BOD and DO at the checkpoints (cp) were less than prescribed regulatory limits. A cp was placed 500 m downstream of each point source release point in baseline runs. In addition, as the point sources contribute significantly to the flows in the river, a minimum wastewater release requirement, specified as 40 % of the minimum permitted discharge from the treatment plants, was also imposed to maintain sufficient in-stream flows for ecological purposes. All these constraints were stated as hard constraints (i.e., without deviational variables). Hence, the hybrid goal-programming model could result in an infeasible solution if one or more constraints are not rigorously met. The mathematical equations for the optimization model are presented below:
Subject to:
All the above variables have been described before and the subscripts std, PCS, PCSav refer to water quality standard, PCSs (point source discharger) and current average value at the PCS, respectively. The total number of PCSs is equal to N and the number of cp is equal to K. As a check point was placed 500 m downstream of each PCS, N = K = 12 in this study.
As the velocity is a nonlinear function of the flowrate, the estimated concentration also bears a nonlinear function with the flow rate. Consequently, the optimization problem described above is nonlinear and its convergence to a global maximum is not guaranteed. Therefore, the standard practice of carrying out several optimization runs, with various starting conditions was adhered to in order to insure that the model converged to a reasonable set of values (Edgar et al. 2001).
DSS software
The DSS consisting of the simulation and the optimization model was coded into a MS-EXCEL 2003 spreadsheet (Microsoft Corp, Redmond, WA). The spreadsheet platform was selected as it includes built-in optimization routines and allows for transparent model development. Also, MS-EXCEL is widely available on computers, so that the DSS could be widely disseminated to stakeholders. Most stakeholders have some familiarity with the software, and as such, there is no additional burden on the stakeholders to obtain and learn specialized software allowing them to focus on the decision-making process.
Results and discussion
Evaluation of the simulation model
The usefulness of the developed DSS hinges on its ability to provide reliable results. Therefore, the outputs from the simulation model were evaluated against available information obtained from field observations and other modeling studies. The simulation model was parameterized using literature derived values for rate constants as presented in Table 2. As no site-specific data were available, these rate constants were assumed to be constant over the stream length to maintain input parsimony and avoid introducing artificial variability which could not be evaluated independently with other data. However, site-specific discharge flowrates and outflow concentrations from the wastewater treatment plants presented in Table 1 (TCEQ 2006a) as well as NPS loadings estimated using the EMC approach, as discussed previously (Table 3), were used in the modeling as well. The model-predicted BOD and DO concentrations were then compared against synoptic field measurements collected by the Texas Commission of Environmental Quality as part of a TMDL study between December 2000 and October 2002. No calibration was attempted given the limited data availability and the non-uniqueness of the process (Oreskes et al. 1994).
A comparison of observed and simulated values is presented in Figs. 4 and 5. At most stations, only one BOD measurement was available, however, multiple BOD concentrations were available at station 4. Therefore, the percent variability at this station was used to establish error bars at other locations and depicted using dotted lines in Fig. 4. The comparison of observed and predicted values indicates that the model predictions are somewhat lower than the observed average values. This bias was expected as some processes such as sediment oxygen demand and the vegetation impacts of DO have not been considered. Nevertheless, the predictions are comparable to the observed average values and fall within the measured range of variability. Despite the acknowledged bias, the model captured the trends in the fate and transport of the tracked constituents. Furthermore, while the constructed model resulted in conservative estimates, it was considered reasonable for its intended use as a policy planning and management tool for point sources as it fell within the range of measured values.
Assessment of NPS loading estimates
The NPS loading estimates within each sub-watershed corresponding to current land use land cover conditions are summarized in Table 3. The results presented in Tables 1 and 3 indicate that 11 % of the BOD loadings into the river are from point sources and 89 % from NPS. Using a more comprehensive HSPF modeling approach, Raines and Miranda (2002) computed the BOD point and NPS contributions into the Arroyo Colorado River to be 23 and 77 %, respectively.
The heterogeneous nature of the NPS loadings within the watershed is clearly evident from Table 3. Four sub-watersheds—Harlingen 1, Harlingen 2, Mercedes, and San Juan contribute nearly 65 % of the total NPS loading into the river. The total NPS loading from major land use categories is summarized in Table 4. It is evident that the loading contributions from different land use categories exhibit heterogeneity among different sub-watersheds as well. The results presented in Table 4 also indicate that the urban land use classes (residential, industrial, and highway categories) contribute significantly (i.e., over 90 %) to the non-agricultural NPS loadings. These results are consistent with the findings from Raines and Miranda (2002). The average NPS BOD contributions among various land use categories in the watershed are summarized in Fig. 6 and indicate that NPS contributions are roughly equally divided among agricultural and urban (residential, industrial, and transportation) uses. As stated previously, currently the watershed is approximately 75 % agricultural and 15 % urban. Therefore, NPS modeling results underscore the need for managing urbanization efficiently in order to insure the sustainability of the river with regard to its water quality.
Evaluation of critical carrying capacity under current conditions
Roughly 35 % of the population in the Arroyo Colorado River Watershed are classified as living in poverty as compared to the State of Texas average of 15.4 % and the per-capita income of $9,337 compares poorly to the Texas average of $19,617 (Sethi and Arriola 2002). Hence, recent strides in economic development should not be curtailed so that economic growth may continue to bring this under-represented area on-par with other regions of the state. However, the increased stress on the river’s water quality due to increased growth has to be properly managed. A critical first step toward the selection of appropriate regulatory and management policies and programs lies in identifying the carrying capacity of the river taking into account its water quality.
In this study, the carrying capacity is defined as the maximum amount of BOD loading that can be added into the river without violating required water quality requirements. Water quality requirements were specified in terms of critical BOD and DO limits and assumed to be 4.75 and 5.0 mg/L, respectively and enforced at 500 m (one-half kilometer) downstream from each point source. These water quality standards stem from the need to maintain sufficient in-stream quality for aquatic organisms and also provide opportunities for downstream cities to dispose of their wastewater. Similarly, the minimum wastewater flow from the source was constrained to be 40 % of the overall lowest flow. This constraint meant that the dischargers could not reduce their discharges by more than 60 % based on their re-use options and to insure adequate in-stream flows.
The current and allowable loadings from different point sources are summarized in Table 5 and are based on ideal convergence from multiple optimization runs with different starting conditions. The results from the optimization runs (Table 5) indicate that the total loadings from all the point sources cannot exceed 765 kg/day of BOD and is in addition to 714 kg/day loading from NPSs (Table 3). Therefore, the aggregate critical limit of the river is estimated to be approximately 1,479 kg/day under existing conditions. Comparing this value to the current point and non-point loadings in Tables 1 and 3, suggests that on a macro-scale the watershed is, at a minimum of 50 % of its critical limit, and, still capable of assimilating additional growth. However, the results in Table 3 also highlight the heterogeneity between the dischargers in regards to increasing their loading into the river indicating that water quality considerations can severely limit the amount of possible growth in 7 of the 12 sub-watersheds under consideration.
The optimization results suggest reducing current BOD loadings at the most upstream (Mission) and the most downstream (San Benito) locations which indicates that these sub-watersheds have already reached their critical limits. There are currently no inflows into the river prior to the Mission wastewater treatment plant and as such, the current loadings from this plant do not undergo any mixing with cleaner water at the confluence point. Being the most downstream location, the loadings from the San Benito treatment plant mix with water from upstream sources that already have significant levels of BOD, especially caused by additions from nearby Harlingen metropolitan area. Furthermore, San Benito currently discharges a significant amount of BOD (i.e., 20 % of the total point source loading) into the river as it uses an antiquated pond system for its treatment (TCEQ 2006a) and infrastructure additions/enhancements are clearly warranted at this location. Given the proximity, re-routing some of the wastewater from San Benito to the Harlingen 1 treatment plant could be an option to promote water quality improvements in the short-term.
The difference between the maximum allowable loading and existing point source loadings (potential increase) generally exhibits an inverse relationship with the NPS loading generated within the corresponding sub-watershed (Fig. 7). Urbanization of sub-watersheds currently with significant NPS loadings critically hinges on how well NPS loadings are managed in the future. The locations having the greatest potential for increasing their point source discharges—McAllen, San Juan, Donna & Weslaco, Mercedes, and Harlingen 1 contribute approximately 40 % of the total NPS discharge into the system and are most amenable to growth. The potential to increase point source loadings should however not be construed as the ability to urbanize ad nauseam. Additional urbanization will likely increase the amount of NPS loadings into the system whose management and loading characteristics (magnitude and location) will eventually dictate how much additional loading can be added in these sub-watersheds. The current analysis however demonstrates areas where significant growth is possible (and not possible) if the NPS loadings can be capped at current levels.
Evaluation of parametric uncertainty and policy implications
The models used to simulate the transport of BOD and DO require several model parameters that were obtained from the literature and were not known with complete certainty. While the comparison of model results with field observations indicated their reasonableness, small-scale variations around these baseline values cannot be ruled out due to measurement limitations and variability induced by changes in temperature, pressure, and salinity (Hattis and Anderson 1999). Also, a range of input estimates would have yielded similar fits to the limited observed data—an aspect referred to as model non-uniqueness (Oreskes et al. 1994). Similarly, the EMCs used in the study were derived from the literature, and while comparisons with other modeling studies indicated the reasonableness of NPS loading estimates, considerable variability given the use of typical EMC values is also noted (Lin 2004). As the simulation model is used within the optimization framework to calculate maximum allowable loadings into the river, understanding the impacts of parametric uncertainty on estimated maximal loadings is critical to prioritize scientific experimentation and data collection activities.
Important policy choices have to be made during the specification of the optimization model, including the specification of cp locations where regulations will be enforced. Clearly, upstream cities will attempt to negotiate for a cp that is farthest from the effluent discharge point, while ecological interests will aim to seek cp locations as close to the PCS as possible. Similarly, the selection of regulatory standards entails some subjectivity, with dischargers preferring less stringent standards, while environmental and recreational interests valuing a cleaner river. Again, an understanding of how these policy choices affect the estimated critical loading limits is vital during collaborative decision making.
A comprehensive sensitivity analysis was carried out by varying the input parameters of the simulation model, the EMCs, and the policy choices in the optimization model by ±10 % around the baseline values. This range of variability, albeit smaller than the natural variability of most inputs, was selected to identify those inputs that have the greatest impact on the estimated maximum loadings. Clearly, if the estimated maximum loadings exhibit substantial variability for even a small (±10 %) change in the input, then every effort must be made to specify this critical input with the greatest possible precision. Also, using a uniform variability for all inputs instead of varying them according to their natural variability was preferred in this preliminary analysis to identify relative impacts when all the inputs are known with the same level of precision (Harr 1987).
The sensitivity analysis was carried out on the combined simulation–optimization model using the total maximum point source loading (the objective function) as the output. The inputs were changed one at a time (while all others were held at the baseline value) and the optimization model was run to identify the maximum allowable point source loadings into the system. However, for certain input values, the optimization model was rendered infeasible, indicating that one or more constraints (BOD, DO, or discharge limits) could not be satisfied. The infeasibility of the optimization model implies that the system, as a whole, has reached its critical limits (as defined using water quality and discharge limits) and cannot sustain increased loadings. Therefore, parameters causing infeasibility were considered to be the most sensitive and are referred to as primary inputs. Typically, infeasibility was caused either for an upper or lower limit of the input variable. Therefore, the absolute sensitivity coefficient was computed using either the forward or backward difference equation as follows:
Equation 14 is the forward difference form which was used when the lower limit of the input caused infeasibility and Eq. 15 is the backward difference form used when the upper limit of the input caused the infeasibility. Parameters whose variations did not cause any infeasibility were considered relatively less sensitive and termed as secondary inputs. The central-difference approximation was used, in this case, to estimate the absolute sensitivity coefficient (ASC) as follows:
where O refers to the output, I refers to the input and superscripts +, −, and b refer to upper, lower and baseline values, respectively.
The relative sensitivity coefficient (RSC) was computed from the knowledge of absolute sensitivity coefficient and baseline values as
The RSC are dimensionless and can be used to rank input parameters according to their importance. As the central-difference approximation is second-order accurate and the backward and the forward difference equations are only first-order accurate (Chapra and Canale 2002), it is best to compare the sensitivity rankings separately for primary and secondary inputs.
The sensitivity of the primary input parameters, as presented in Table 6, indicates that the water quality standard for BOD is the most sensitive parameter. The loading for this case was noted to increase to 829 kg/day from the baseline value of 633 kg/day if the standard were to be changed from 4.75 to 5.225 mg/L. However, a less stringent standard implies lesser ecological services and should therefore be accepted only after a careful ecological risk assessment and valuation. The standard for DO is also a sensitive primary parameter, but not as important as the BOD standard. This result is to be expected because the Arroyo Colorado River is characterized by relatively high wind speeds and shallower depths which do not pose sizable resistance to oxygen mass-transfer (i.e., reaeration). The results from Table 6 also identify the need for site-specific EMC data, particularly for agricultural, urban, and industrial loadings. The higher impact of the agriculture EMCs are to be expected because a large area of the watershed is used for agricultural purposes. Nevertheless, the sensitivity of urban and industrial EMCs emphasize that improper management of urban NPSs could easily render the river unsustainable from a water quality standpoint. The results from the sensitivity analyses also highlight the important role of NPS loadings on estimating critical limits of the river.
The results presented in Table 7 indicate that the flow coefficient and exponent and the BOD removal rates are important at the secondary level, as the assumed fluctuations in these parameters did not render the model infeasible. The estimated total allowable loadings were fairly insensitive to secondary input parameters and a 10 % change in the input values caused less than 3 % change in the estimated output. Again, several EMCs were seen to exhibit secondary sensitivity, the impacts of these parameters was less because the corresponding land use types occupied relatively smaller areas in the watershed. The distance at which the check point was placed from the discharge location had a minimal impact on the estimated loadings. This result along with the sensitivities of the BOD and DO standards suggests that discussions pertaining to acceptable water quality standards must take precedence over where these standards should be enforced.
In addition to the sensitivity analysis, an optimization run was performed assuming all cities discharged at their permitted levels. This run resulted in an infeasible solution indicating that the river cannot sustain current permitted loadings. The result, therefore, indicates that cities with better infrastructure are compensating for the inefficient systems in others, and, as such, costs of treatment are currently inequitably distributed and must be corrected. For example, while the City of McAllen is permitted for a BOD concentration of 20 mg/L, the current effluent has an average BOD concentration of 2.5 mg/L. Conversely, the next city is permitted for a BOD concentration of 30 mg/L with a current effluent average BOD concentration of 62.27 mg/L. Given the relative flows (City of McAllen has larger flows), the large loadings from the second city are being diluted by the relatively cleaner flows from McAllen. Therefore, the amount of wastewater treatment that is being subsidized by cities with better infrastructure needs to be quantified and economic instruments like effluent trading must be adopted to make the sharing of wastewater treatment costs more equitable.
Final remarks
Balancing the competing demands of economic development and environmental protection is vital to insure continued ecological services. Toward this end, waste assimilation and water purification provided by rivers and streams should be available for future generations at the same level as today. Identification of whether the river has reached its critical limits in regards to its waste assimilation characteristic is fundamental to fostering smart growth and implementing sustainability-oriented watershed stewardship. However, an assessment of critical limits considering waste load allocations hinges upon how the river assimilates and transports pollutants, the amount of NPSs that enter the system and stakeholders preferences with regards to water quality management. A DSS that addresses all these issues is developed in this study by embedding mass-balances and a GIS-based NPS loading scheme into a hybrid goal-programming-based optimization approach and applied to the Arroyo Colorado River watershed along the US-Mexico border. The DSS was evaluated against available field data and other information and found to be useful as a general guide for water quality planning and management purposes. However, the underlying assumptions of the model must be fully understood during the policy-planning process.
The application of the model indicates that while the watershed may not have reached its overall critical limit, certain sections may have to curtail their effluent discharge loadings. The model results were sensitive to policy choices concerning water quality standards. Furthermore, an assessment of NPS loadings indicated a major influence on the estimated waste load allocations. Therefore, infrastructure improvements and non-structural practices to properly treat stormwater loadings, especially in the urban areas of the watershed is vital for meeting the competing objectives of economic development and protection of water quality in this internationally important region.
References
ACWP (2007) A watershed protection plan for the arroyo Colorado—Phase I. Arroyo Colorado Watershed Partnership, http://www.arroyocolorado.org/watershed-protection-plan/. Accessed Dec 2011
Arbabi M, Elzinga J (1975) A general linear approach to stream water quality modeling. Water Resour Res 11(2):191–196
Behera PK, Adams BJ, Li JY (2006) Runoff quality analysis of urban catchments with analytical probabilistic models. J Water Resour Plan Manag 132(1):4–14
Bitton G (1998) Formula handbook for environmental engineers and scientists. Wiley, New York
Briendenbach J, Seo DJ, Fulton R (1998) Stage II and stage III post processing of NEXRAD precipitation estimates in the modernized weather service. In: AMS 78th annual meeting, Phoenix
Brown LC, Barnwell TO (1987) The enhanced stream water quality models Qual2e and Qual2e-Uncas: documentation and user manual. Environmental Research Laboratory, U.S. EPA, Athens
Burn DH (1989) Water quality management through combined simulation–optimization approach. J Environ Eng 115:1011–1024
Burn DH, McBean EA (1985) Optimization modeling of water quality in an uncertain environment. Water Resour Res 21(7):934–940
Cardwell H, Ellis H (1993) Stochastic dynamic programming models for water quality management. Water Resour Res 29(4):803–817
Chapra SC (1997) Surface water quality modeling. McGraw-Hill, New York
Chapra SC, Canale RP (2002) Numerical methods for engineers: with software and programming applications. McGraw-Hill, New York
Charbeneau RJ, Barrett ME (1998) Evaluation of methods for estimating stormwater pollutant loads. Water Environ Res 70(7):1295–1302
Di Toro DM (1979) Statistics of receiving water response to runoff. In: Urban stormwater and combined sewer overflow impact on receiving water bodies, national conference, University of Central Florida, Orlando
Edgar TF, Himmelblau DM, Lasdon LS (2001) Optimization of chemical processes. McGraw-Hill, New York
Ewing R, Bartholomew K, Winkelman S, Walters J, Chen D (2007) Growing cooler: evidence on urban development and climate change. Urban Land Institute, Washington
Harr ME (1987) Reliability-based design in civil engineering. McGraw-Hill, New York
Hattis D, Anderson EL (1999) What should be the implications of uncertainty, variability, and inherent “biases”/“conservatism” for risk management decision-making? Risk Anal 19(1):95–107
Hernandez EA (2007) Risk based methodologies for watershed management: integrated simulation and optimization techniques. Dissertation, Texas A&M University—Kingsville, Kingsville
Hernandez E, Uddameri V (2010) Selecting agricultural best management practices for water conservation and quality improvements using Atanassov’s intuitionistic fuzzy sets. Water Resour Manag 24(15):4589–4612
IBWC (2005) “2005 annual report.” International Boundary and Water Commission, www.ibwc.gov/Organization/Public_Affairs.html. Accessed 11 Dec 2011
ICCMA, SGN (2006) This is smart growth [Brochure]. EPA 231-K-06-002, International City/County Management Association, and Smart Growth Network
Korfmacher KS (1998) Water quality modeling for environmental management: lessons from the policy sciences. Policy Sci 31:35–54
Korfmacher KS (2001) The politics of participation in watershed modeling. Environ Manag 27(2):161–176
Kouwen N (1988) Watflood: a micro-computer based flood forecasting system based on real-time weather radar. Can Water Resour J 13(1):62–77
Stubbs MJ, Rister ME, Sturdivant AW, Lacewell, RD (2005) An overview of operational characteristics of selected irrigation districts in the Texas Lower Rio Grande Valley: Hidalgo County Irrigation District No. 2 (San Juan). Texas A&M University, College Station
León LF, Soulis ED, Kouwen N, Farquhar GJ (2001) Nonpoint source pollution: a distributed water quality modeling approach. Water Res 35(4):997–1007
Leopold LB, Maddock T (1953) The hydraulic geometry of stream channels and some physiographic implications. U. S. Geological Survey, Washington, DC
Lin JP (2004) Review of published export coefficient and event mean concentration (Emc) data. U.S. Army Corps of Engineers, Wetlands Regulatory Assistance Program
Lindenschmidt K-E (2006) The effect of complexity on parameter sensitivity and model uncertainty in river water quality modelling. Ecol Model 190:72–86
Lohani BN, Thanh NC (1979) Probabilistic water quality control policies. J Environ Eng 105(4):713–725
Lung W-S, Sobeck RG Jr (1999) Renewed use of Bod/Do models in water quality management. J Water Resour Plan Manag 125(4): 222–227
Malano HM, Davidson B (2009) A framework for assessing the trade-offs between economic and environmental uses of water in a river basin. Irrig Drain 58(S1):S133–S147
Marsalek J (1991) Pollutant loads in urban stormwater: review of methods for planning level estimates. Water Resour Bull 27(2):283–291
Mitchell G (2005) Mapping hazard from urban non-point pollution: a screening model to support sustainable urban drainage planning. J Environ Manag 74:1–9
Mujumdar PP, Sasikumar K (2002) A fuzzy risk approach for seasonal water quality management of a river system. Water Resour Res 38:51–59
Mujumdar P, Saxena P (2004) A stochastic dynamic programming model for stream water quality management. Sadhana 29(5):477–497
Murty YSR, Bhallamudi SM, Srinivasan K (2006) Non-uniform flow effect on optimal waste load allocation in rivers. Water Resour Manag 20:509–530
Nas SS, Nas E (2009) Water quality modeling and dissolved oxygen balance in streams: a point source Streeter–Phelps application in the case of the Harsit stream. Clean Soil Air Water 37(1):67–74
NCDC (2006) NCDC—image and publications system. National Climatic Data Center. http://www7.ncdc.noaa.gov/IPS/. Accessed June 2006
NRC, CASB, WSTB (2001) Assessing the Tmdl approach to water quality management. National Research Council, Committee to Assess the Scientific Basis of the Total Maximum Daily Load Approach to Water Pollution Reduction, and Water Science and Technology Board. National Academy Press, Washington, DC
Oreskes N, Shrader-Frechette K, Belitz K (1994) Verification, validation, and confirmation of numerical models in the earth sciences. Science 263:641–646
Orlob GT (1983) Mathematical modeling of water quality: streams, lakes, and reservoirs. Wiley, New York
Pollard OA (2000) Smart growth: the promise, politics, and potential pitfalls of emerging growth management strategies. Copyright (c) 2000 Virginia Environmental Law Journal Association Virginia Environmental Law Journal
Qin X, Huang G, Chen B, Zhang B (2009) An interval-parameter waste-load-allocation model for river water quality management under uncertainty. Environ Manag 43(6):999–1012
Raines TH, Miranda RM (2002) Simulation of flow and water quality of the arroyo Colorado, Texas, 1989–1999. Water-Resources Investigations Report 02-4110, U.S. Geological Survey, Austin, Texas
Reckhow KH, Butcher JB, Marin CM (1985) Pollutant runoff models: selection and use in decision making. Water Resour Bull 21(2):185–195
Revelle CS, Loucks DP, Lynn WP (1968) Linear programming applied to water quality management. Water Resour Res 4(1):1–9
RGRWPG (2011) Region M 2011 Water Plan. Rio Grande Regional Water Planning Group. riograndewaterplan.org/waterplan.php. Accessed 28 Dec 2011
Sasikumar K, Mujumdar PP (1998) Fuzzy optimization model for water quality management of a river system. J Water Resour Plan Manag 124:79–88
Sethi SJ, Arriola RS (2002) Targeting the future: a report about the evolving labor market in Texas’ Rio Grande Valley. Tech Prep of the Rio Grande Valley, Inc. for CBIRD-Texas Regional Action Council
Streeter HW, Phelps EB (1925) A study of the pollution and natural purification of the Ohio River. Public Health Bulletin 146, U.S. Public Health Service, Washington, DC, pp 127–146
Takyi AK, Lence BJ (1999) Surface water quality management using a multiple-realization chance constraint method. Water Resour Res 35:1657–1670
TCEQ (2006a) Pollutant reduction plan for the arroyo colorado: segments 2201 and 2202, Hidalgo, Cameron, and Willacy Counties. Texas Commission on Environmental Quality. www.tceq.state.tx.us/assets/public/implementation/water/tmdl/13arroyo/13-arroyo_prp_july2006.pdf. Accessed Aug 2006
TCEQ (2006b) Sampling data query, surface water quality monitoring. Texas Commission on Environmental Quality. http://www.tceq.state.tx.us/compliance/monitoring/crp/data/samplequery.html. Accessed June 2006
TCEQ (2008) 2008 Texas 303(D) List (March 19, 2008). Texas Commission on Environmental Quality. http://www.tceq.texas.gov/waterquality/assessment/08twqi/twqi08.html. Accessed Dec 2011
TCEQ (2010) Draft 2010 Texas 303(D) List (February 5, 2010). Texas Commission on Environmental Quality. http://www.tceq.texas.gov/waterquality/assessment/10twqi/10twqi. Accessed Dec 2011
Thomann RV, Mueller JA (1987) Principles of water quality modeling and control. Harper and Row Publishers, New York
Vasquez JA, Maier HR, Lence BJ, Tolson BA, Foschi RO (2000) Achieving water quality system reliability using genetic algorithms. J Environ Eng 126(10):954–962
Webler T, Tuler S, Dietz T (2011) Modellers’ and outreach professionals’ views on the role of models in watershed management. Environ Policy Gov 21(6):472–486
Acknowledgments
This material is based upon work supported by NSF under Cooperative Agreement No. HRD-0206259. Any opinions, findings, and conclusions or recommendations expressed in this material are those of the author and do not necessarily reflect the views of the National Science Foundation. The authors would like to thank Javier Davila for his data collection efforts. In addition, the authors would like to thank Theodore G. Cleveland for his editorial review and the anonymous reviewers for the constructive criticism, both of which enhanced the clarity and readability of this paper.
Author information
Authors and Affiliations
Corresponding author
Rights and permissions
About this article
Cite this article
Hernandez, E.A., Uddameri, V. An assessment of optimal waste load allocation and assimilation characteristics in the Arroyo Colorado River watershed, TX along the US–Mexico border. Clean Techn Environ Policy 15, 617–631 (2013). https://doi.org/10.1007/s10098-012-0546-6
Received:
Accepted:
Published:
Issue Date:
DOI: https://doi.org/10.1007/s10098-012-0546-6