Abstract
This study examines the performance of the regional climate model, PRECIS, in reproducing the historical seasonal mean climatology over the Malaysian region. The performance of the model in simulating the seasonal climate pattern of the temperature, precipitation and large-scale circulation was reasonably good. The biases of temperature are less than 2 °C in general, while the seasonal cycles match the observed pattern despite some differences in certain regions. However, the biases for precipitation were greater, particularly over the mountainous areas. These biases could be associated with the deficiencies of the model physics, related to the misrepresentation of the land–surface interaction and convective scheme. Furthermore, the model fails to simulate the mean sea-level pressure over the interior part of Borneo with a significant low-pressure centre. A higher magnitude of the moisture convergence and divergence simulated by the model also contributed to the biases of precipitation over Malaysia.
Similar content being viewed by others
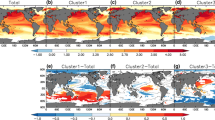
Avoid common mistakes on your manuscript.
1 Introduction
Future climate information associated with anthropogenic warming is essential for deciding adaptation measures to minimize the impact of climate change on the environment and human systems in a particular region. Global climate models (GCMs) are often used to project future climate conditions, by forcing the models with future greenhouse gas concentration scenarios. GCMs, used by the Intergovernmental Panel on Climate Change (IPCC) in its Fourth Assessment Report, are examples of such models (IPCC 2007). However, owing to their coarse resolution (∼100–300 km), most of these GCMs are unable to resolve climate features at regional scales (Christensen et al. 1997; Gao et al. 2008; Seguí et al. 2010). This is a result of the models’ inability to resolve complex topography, coastlines and islands, as well as processes that are too small of scale to be represented by large grids (Hudson and Jones 2002). Therefore, these models usually have difficulty in simulating regional as well as local climate features, such as cyclones, extreme precipitation events, orographic rainfall, etc. (Hudson and Jones 2002). Consequently, a finer resolution model is required to capture the regional as well as local climate features of a particular region. This is usually implemented by downscaling the GCMs’ outputs, using a regional climate model (RCM) that has much higher grid resolution compared to GCM. Malaysia lies in the western part of the Maritime Continent, a region known to have a very complex topography and land mass distributions, owing to the existence of many large and small islands within the region. GCM with low grid resolution is known to have difficulty in simulating the climate processes over this region (Hudson and Jones 2002). For climate change impact assessment over this region, a high-resolution regional climate model is required.
For the past few years, there have been a large number of studies dealing with the downscaling of GCM using RCM, both for simulation of regional current climate and for projections of future climate up to the end of the 21st century (Giorgi et al. 2004a; Giorgi et al. 2004b; Moberg and Jones 2004; Raisenan et al. 2004). There are several RCMs available, such as Hadley Centre, UK Met Office’s Providing Regional Climate for Impacts Studies (PRECIS), Abdus Salam International Centre for Theoretical Physics’ Regional Climate Model (RegCM3), Max Planck Institute for Meteorology’s Regional Model, etc., and these RCMs are known to have differences in climate simulation capability. Several studies on the evaluation of the accuracy and skill of the RCM have been carried out in various regions (Alves and Marengo 2010; Christensen et al. 1997; Giorgi et al. 2004a; Im et al. 2006; Jacob et al. 2007; Kotroni et al. 2008; Sylla et al. 2010). For a particular RCM, it is crucial that it has the capability to simulate the present climate before it can be used for future climate projection. The performance of a particular RCM in simulating the current climate can be evaluated by forcing the RCM with reanalysis observations and, later, comparing the simulated climate with those of the observed values (Giorgi and Mearns 1999). In the present study, the ability of PRECIS to simulate historical climate, driven by the second generation of the European Centre for Medium Range Weather Forecast (ECMWF) reanalysis (ERA40), was evaluated. We focus on different statistics (mean climatology, annual cycle and inter-annual variation) for the monthly total precipitation rate (mm/month) and surface air temperature over different areas in the Malaysian region.
2 Data and method
2.1 Model description
The PRECIS modelling system was developed by the Hadley Centre, UK Met Office (Hudson and Jones 2002). PRECIS includes a regional model component (HadRM3P) that can be used to simulate regional climate over any region of the globe. The HadRM3P is a land–atmosphere coupled model, which is similar to the HadRM3H (Hudson and Jones 2002). The model is configured with 19 levels of hybrid vertical coordinates, and the atmospheric model is based on hydrostatic primitive equations (Simmons and Burridge 1981; Simon et al. 2009). The mass flux penetrative scheme with an explicit downdraught is used as the convective scheme (Gregory and Allen 1991; Gregory and Rowntree 1990), while the Met Office Surface Exchange Scheme is employed as the land surface model component (Cox et al. 1999). The soil and land surface dataset is derived from the global land use and vegetation dataset of Wilson and Henderson-Seller (1985). Detailed descriptions of the model physical parameterization are explained by Jones et al. (2004). The model was configured with a relatively high horizontal resolution of 0.22° × 0.22° (∼25 km × 25 km), which allowed it to be run at reasonable computational cost over a domain covering the western Maritime Continent (95°E to 123°E and 7.5°S to 14°N). The domain included Peninsular Malaysia, Sumatera and Borneo Island which are large enough to drive the regionally important synoptic monsoon circulation such as the north-easterly cold surge winds (Holland 1984) and Borneo vortex (Chang et al. 2005). The combined interactions between Borneo vortex with the north-easterly cold surge wind may lead to severe hydrological disaster over the Peninsular Malaysia as well as the western part of the Borneo Island (Juneng et al. 2007; Salimun et al. 2010; Tangang et al. 2008). This study focuses mainly over the area of Malaysia region.
The initial and lateral boundary conditions for the PRECIS simulation were obtained from the ERA40 gridded reanalysis data, covering a period from September 1957 to August 2002. The ERA40 is the second generation of the product produced by the European Centre for Medium-Range Weather Forecasts (ECMWF), in collaboration with many institutions (Uppala et al. 2005). Surface and lateral boundary conditions were updated every 24 h and every 6 h, respectively. The PRECIS simulation was carried out for the period 1970–1999. The validation period was from 1971 to 1999 where the model simulation output was compared to the Climate Research Unit (CRU) data. The 1 year of spin-up integration is necessary to allow the atmosphere and the land–surface to adjust to a mutual equilibrium state (Simon et al. 2009).
2.2 Data and analysis method
The reliability of climate experiment results is largely based on the ability of the climate models in reproducing the observed states of present-day climate. The model simulated surface temperature and precipitation was compared to the established and widely used observed data from the Climate Research Unit (CRU) of the University of East Anglia (Mitchell and Jones 2005). The CRU gridded product extends over global land surface and was constructed from a dense network of global station observations obtained from various sources. Since CRU data have a resolution of 0.5° × 0.5°, PRECIS output was regridded to match this resolution using bilinear interpolation. The simulated patterns of seasonal precipitation and near-surface temperature were compared with the CRU values, and the biases were plotted. In addition to grid-to-grid comparison, the model ability to simulate seasonal precipitation and near-surface temperature for 11 subregions was also considered (Fig. 1). The annual cycles and standard deviations (coefficients of variance for precipitation) for both the simulated and the observed values were compared visually for each of the defined subregions. Correlation coefficient values between observed and simulated seasonal precipitation and temperature were calculated for each of the 0.5° × 0.5° grids, and correlation maps were prepared. The PRECIS ability to simulate the atmospheric circulation was also assessed by visually comparing the simulated 850 hPa wind, mean sea-level pressure (MSLP), moisture flux and moisture divergence, with the reanalysis values of the National Centre Environment Prediction/National Centre for Atmospheric Research (NCEP/NCAR) (Kalnay et al. 1996) and the ERA40.
3 Results and discussion
3.1 Surface temperature
3.1.1 Spatial pattern
The ERA40/PRECIS simulated near-surface temperature values, and the corresponding observed CRU data and their biases are shown in Fig. 2. During December–January–February (DJF) and September–October–November (SON) seasons, the north-eastern region of Peninsular Malaysia experiences cooler temperature as a result of the advection of air masses from the north by north-easterly winds (Fig. 2a, d). In contrast, during the March–April–May (MAM) and June–July–August (JJA) seasons, the temperature over Peninsular Malaysia and Borneo are higher, owing to the south-west monsoon dry wind (Fig. 2b, c; Ramage 1971). The PRECIS model was able to reproduce these spatial patterns, although the area of minimum temperature extends slightly southward for Peninsular Malaysia and westward for Borneo. Overall, the model underestimated temperature in the interior part of both Peninsular Malaysia and Borneo by 1 to 2 °C. For the coastal areas, the model overestimated the observed values by about the same magnitude. The biases along the coastline could be a result of the interpolation of PRECIS values onto the CRU land grid. Similar biases are observed by Giorgi et al. (2004a). In the mountainous area, the biases can exceed 2 °C. The underestimation of the temperature in the mountainous area could be due to the coarse model resolution that was unable to resolve the topography over the studied region. In addition, misrepresentation in the land–surface processes and interaction could also be contributing factors (Alves and Marengo 2010; Marengo et al. 2003). This misrepresentation may lead to changes in surface energy and water balance, resulting in surface cooling through a reduction in the amount of long-wave radiation (Alves and Marengo 2010). However, it is difficult to verify the causes of the model temperature biases, as the simulated temperature is a result of interaction of various model variables, such as cloud coverage, solar incoming radiation, surface albedo, energy fluxes, etc. (Konare et al. 2008; Sylla et al. 2010). On the other hand, the CRU dataset may also introduce biases owing to the fact that fewer air temperature stations were available in the mountainous area (Alves and Marengo 2010; Marengo et al. 2009; New et al. 2002). Overall, the temperature biases shown in this study are consistent with other investigations. Islam et al. (2007) used PRECIS reported temperature biases of around 2 °C during DJF and SON, and 1 °C during MAM and JJA, over Bangladesh. Giorgi et al. (2004a) and Sylla et al. (2010) found temperature biases around 2 °C, using RegCM regional climate model over the European and African continents, respectively. The multiple models used in the project Prediction of Regional scenario and Uncertainties for Defining European Climate change risks and Effects (PRUDENCE) also showed approximately ±2 °C of temperature biases over the European region (Jacob et al., 2007). Hence, the PRECIS temperature biases over this region are consistent with other regional downscaling studies.
Spatial distributions of seasonal averaged near-surface temperature (unit: °C) of the observation (CRU) (left panels), downscaled ERA40/PRECIS interpolated to the CRU grid resolution (centre panels) and the mean bias between the downscaled ERA40/PRECIS and observed CRU data (downscaled minus observation) (right panels) (unit: °C)
3.1.2 Area-averaged temperature
The mean annual cycles of surface temperature for all subregions (Fig. 1) are shown in Fig. 3. Generally, the modelled seasonal cycles match the observed pattern, despite notable differences in some regions (e.g. R2, R3, R4, R8, R9 and R10). In all subregions, the observed maximum temperature occurs during April–May–June, with the secondary peak during SON in some areas. In most subregions, these two peaks are simulated by the model, except in R3, R4, R8, R9 and R10. During the DJF season, both observed and modelled results show a temperature decrease of 1 to 2 °C from the maximum value. The major deficiency of the model in several subregions (R2, R3, R4, R8, R9 and R10) was the consistent underestimation of temperature by as much as 2 °C in all months. The underestimation is most visible in the mountainous areas. The CRU datasets may not adequately represent the observed values over the mountainous area, owing to few meteorological stations in this region (Alves and Marengo 2010; Kotroni et al. 2008; New et al. 2002). Large biases over mountainous areas were also described in other regional climate downscaling studies (Cavalcanti et al. 2002; Marengo et al. 2003).
Comparison between monthly temperature climatology (left ordinates) of the observation (CRU) (black bars) and the ERA40/PRECIS downscaling simulation (white bars), averaged over the 11 regions (refer to Fig. 1). The observed (dashed line) and simulated (solid line) inter-annual variability, as indicated by the year-to-year standard deviations (right ordinates), are also plotted
Figure 3 also provides the estimates of the inter-annual variability of both modelled and observed temperature. In most subregions, the inter-annual variability peaks during late DJF, and the JJA season was consistent with Tangang et al. (2007). Generally, there exists a general agreement between the simulated and observed inter-annual variability, suggesting that the model captured the inter-annual variations, especially those associated with El Niño Southern Oscillation (Tangang et al. 2007). The seasonal correlation coefficient map, between the model simulation and the CRU datasets for the near-surface temperature, is shown in Fig. 4. High correlation values (∼0.8) were found over the eastern part of Peninsular Malaysia for all seasons. However, the value decreases to about 0.4 over the north-western part of Peninsular Malaysia. Over Borneo, high correlation values that exceeded 0.8 were noted, but the value decreases to about 0.6 over the north-east Borneo during the DJF and SON seasons (Fig. 4a, d). The generally high correlation values between the model and observed temperature reflect the model ability and simulation of the surface temperature over Malaysia.
3.2 Precipitation
3.2.1 Spatial pattern
Figure 5 compares the seasonal distribution of modelled and observed precipitation over Malaysia, with the biases shown in percentages. In general, the PRECIS simulated precipitation features mesoscale patterns in comparison to much smoother CRU data. Overall, ERA40/PRECIS was able to simulate the general precipitation pattern over the Malaysian region during the MAM and SON seasons (Fig. 5b, d). During the MAM season, the model was able to reproduce the maximum peak of rainfall over the north-western part of Peninsular Malaysia and central Borneo (Fig. 5f). However, the model tended to produce more rainfall than was observed over the mountainous area of Peninsular Malaysia. During the SON season, the model simulated a higher amount of rainfall over the northern part of Peninsular Malaysia and the interior part of Borneo (Fig. 5h). The biases range from −40 to 60 %, with a tendency for overestimation over mountainous areas and underestimation over coastal regions.
Spatial distributions of seasonal averaged rainfall (unit: mm/month) of the observation (CRU) (left panels), downscaled ERA40/PRECIS interpolated to the CRU grid resolution (centre panels) and the percentage different between the downscaled ERA40/PRECIS and observed CRU data (downscaled minus observation) (right panels) (unit: %)
During the DJF and JJA seasons, the performance of the model was relatively low. The model was able to simulate the maximum rainfall over eastern Peninsular Malaysia and western Sarawak during the DJF season. However, the location of the maximum rainfall over Peninsular Malaysia shifted northward (Fig. 5a, e). Over Borneo, a higher amount of rainfall was simulated over the mountainous area and also the western tip of Sarawak. During the JJA season, the simulated pattern was inconsistent with the observed. Over north-eastern Peninsular Malaysia, the model overestimated the precipitation (Fig. 5c, g). The model also overestimated the precipitation over the mountainous area in the interior part of Borneo, with the biases exceeding 100 % (Fig. 5j, l). These discrepancies may be a result of the poor representation of the hydrological cycle and the convective parameterization (Salimun et al. 2010). Nevertheless, the precipitation biases shown here are consistent with other studies using the PRECIS model (Kumar et al. 2006; Alves and Marengo 2010). Similarly, several studies using the RegCM3 regional climate model found a noticeable overestimation over eastern Africa (Segele et al. 2008) and western Africa (Sylla et al. 2009). Moreover, overestimation of the precipitation over the mountainous area in regional simulations was considered a common behaviour (Giorgi et al. 2004a; Solman et al. 2008).
3.2.2 Area-averaged precipitation
Figure 6 shows the annual cycles of the total precipitation rate and inter-annual variation. Overall, the model was able to simulate the precipitation annual cycles reasonably well over several subregions (e.g. R1, R4, R5 and R9). On the eastern coast of Peninsular Malaysia (R1 and R5), the model simulated the increase in rainfall during the north-east monsoon rather well. However, in north-eastern Peninsular Malaysia (R1), the model overestimated rainfall during September and October, indicating the earlier onset of the north-east monsoon. Moreover, an apparent underestimation was also found over north-western Peninsular Malaysia (R2 and R3) and western Sarawak (R11). The model also underestimated precipitation during the DJF season over R6, R7 and R10. The deficiencies in these subregions could be a result of the model’s inability to resolve the orography. The biases may also be introduced by the possible shortcomings of CRU data in this region (Alves and Marengo 2010; Kotroni et al. 2008; Marengo et al. 2009; New et al. 2002).
Comparison between monthly rainfall climatology (left ordinates) of the observation (CRU) (black bars) and the ERA40/PRECIS downscaling simulation (white bars), averaged over the 11 regions (see Fig. 1). The observed (dashed line) and simulated (solid line) inter-annual variability, as indicated by the year-to-year standard deviations (right ordinates), are also plotted
The inter-annual variation of the total precipitation rate, represented by the coefficients of variance, was simulated well by the model, except for the northern part of Borneo. High rainfall variance was found during the DJF season and decreases during JJA seasons. This indicates that the rainfall rate varies the most during the north-east monsoon and vice versa for the dry season (Neale and Slingo 2003; Tangang and Juneng 2004). Over the northern part of Borneo Island (R6, R7 and R8), the model shows a significant underestimation of the inter-annual variations. Overall, ERA40/PRECIS performs satisfactorily in simulating rainfall climatology over the Malaysian regions. Although the model produces significantly weaker inter-annual variations over some of the area (e.g. R6, R7, R8), the patterns of the inter-annual variations were reasonably reproduced by the model. On the other hand, the correlation coefficients of the precipitation in Fig. 7 suggest that the model’s skill in simulating the precipitation is rather weak compared to the temperature. The correlation values are lower than 0.6 over the Malaysian region with a small area showing negative values. The negative value of the correlation indicates the inability of the model to simulate the precipitation for the respective area. These areas include the interior part of Borneo during DJF, and the interior part of Peninsular Malaysia during the SON season. A low correlation (<0.2) was also found in other areas throughout the season.
3.3 Large-scale circulation over the Maritime Continent
The regional precipitation distributions are very much influenced by the large-scale flow pattern. This section discusses the model performances in simulating the large-scale circulation over the Maritime Continent. Figures 8 and 9 show the comparison between simulated 850 hPa winds and mean sea-level pressure (MSLP) with the NCEP/NCAR reanalysis and the ERA40 reanalysis, respectively. Generally, the model was able to simulate the low-level wind flow pattern and wind magnitudes of all seasons reasonably well (Fig. 8). The bending of the wind direction, as a result of blocking and deflection by the mountain areas over Peninsular Malaysia and Sumatera Island, was well represented by the model. Seasonal variations of the MSLP over the Maritime Continent were also well simulated by the model, except for the low-pressure centre over the interior part of Borneo (Fig. 9). However, the model simulated a cyclonic wind circulation over the western part of Sarawak and significant low MSLP over the interior part of Borneo during the DJF season (Fig. 9i) where these features were absent in both the reanalysis data. Furthermore, a higher magnitude of moisture convergence and moisture flux over the same regions was simulated by the model, and this may contribute to excessive precipitation (Fig. 10a, e, i). Nevertheless, the underestimation of the precipitation over Peninsular Malaysia and Sabah might be due to the large magnitude of moisture divergence simulated by the model over northern Peninsular Malaysia and north-eastern Sabah.
Spatial distributions of seasonal averaged moisture divergence (shaded, 10−5 s−1) and moisture flux (vector, g m−1s−1) of the NCEP/NCAR reanalysis (left panels), the ERA40 reanalysis (centre panels) and the downscaled ERA40/PRECIS interpolated to the NCEP/NCAR reanalysis grid resolution (right panels)
Although the modelled JJA wind circulation matches both the observed data (Fig. 8c, g, k), MSLP again showed a low-pressure centre over the interior part of Borneo (Fig. 9k). A low-pressure system is normally associated with wind convergence and thus contributed to the increased rainfall. However, higher moisture divergence and fluxes was found over that area (Fig. 10k), thus lowering the moisture content in the atmosphere. Therefore, the biases of the rainfall amount over the interior of Borneo are relatively lower during the JJA season compared to the DJF season. Over Peninsular Malaysia, the model overestimated the moisture fluxes and convergence over the entire country (Fig. 10k) and slightly underestimated the MSLP (Fig. 9k), which could lead to a higher rainfall simulation. A similar bias of MSLP and moisture convergence was also noted over the entire domain during the SON season, with the moisture convergence extending towards Sumatera Island, suppressing the moisture divergence during the JJA season. Generally, ERA40 reanalysis is in good agreement with the NCEP reanalysis over the Maritime Continent. However, the regional model result appears to be different from the reanalyses over the interior part of Borneo with large discrepancies for MSLP and associated moisture convergence. This discrepancy was not inherited from the driving reanalysis data but mainly due to PRECIS’ inability to correctly reproduce the regional circulation over the area.
4 Conclusion
In this paper, the performance of the PRECIS model, in simulating the seasonal climatology, annual cycle and inter-annual variation for near-surface temperature and total precipitation rate over the Malaysian region, was investigated. The lateral boundary condition used was the second generation of the ECMWF ERA40 reanalysis data. The analysis consists of the spatial distribution over the whole Malaysia, region, and was later subdivided into 11 regions for more detailed climatology analyses. Later, the large-scale circulation of wind field, mean sea-level pressure (MSLP) and moisture flux and convergence were examined in order to investigate the biases in the precipitation field.
Overall, the seasonal mean pattern of the modelled near-surface temperature matches that of the observed data reasonably well with biases of 1–2°C. However, an underestimation of the temperature was found in the mountainous area throughout all the seasons. Along the coastline of both Peninsular Malaysia and Borneo Island, warm biases were noted. This is believed to be associated with the deficiencies of the model dynamics in clearly resolving the orography or misrepresentation of the land–surface interaction and convective scheme. Furthermore, the observed data used in the near-surface temperature field may also be doubtful, particularly over the mountainous area where the available air temperature stations were inadequate, leading to an interpolation error in the CRU dataset.
The model is able to simulate the precipitation seasonal patterns and mesoscale features rather well. Some of the maximum precipitation peaks over Peninsular Malaysia and Borneo Island were reproduced consistently by the model. However, the model simulated a persistent overestimation over the interior part of Borneo throughout all the seasons. Biases over this region may be a result of the modelled MSLP that showed a low-pressure centre over the interior part of Borneo instead of the high-pressure centre as evidenced in the observed data, leading to intense wind convergence. Furthermore, a higher moisture convergence was found over the same place, which contributed to the overestimation of precipitation, particularly during the DJF season. High moisture convergence over the upper part of the whole domain was simulated by the model during the JJA and SON seasons, which influences the magnitude of the total precipitation.
The analysis results show that PRECIS is considered capable of simulating the present climate variation and inter-annual variations over the Malaysian region. It is generally applicable for various climate simulation experiments over western maritime continent. However, in order to generate a more reliable future climate projection in the Malaysian region, an adjustment to the settings of the model dynamics are required to correct the different systematic errors presented. On the other hand, analyses of the model uncertainties are equally important with regard to understanding further the natural and the model physics behaviour. Also, better simulation analyses are needed for a better and more sustainable adaptation and mitigation strategy design.
References
Alves LM, Marengo J (2010) Assessment of regional seasonal predictability using the PRECIS regional climate modelling system over South America. Theor Appl Climatol 100:337–350. doi:10.1007/s00704-009-0165-2
Cavalcanti IFA, Marengo JA, Satyamurty P, Trosnikov I, Bonatti JP, Nobre CA, D’Almeida C, Sampaio G, Cunningham CAC, Camargo H, Sanches MB (2002) Global climatological features in a simulation using CPTEC/COLA AGCM. J Climate 15:2965–2988
Chang CP, Harr PA, Chen HJ (2005) Synoptic disturbances over the equatorial South China Sea and western Maritime Continent during boreal winter. Mon Wea Rev 113:489–503
Christensen JH, Machenhauer B, Jones RG, Schär C, Ruti PM, Castro M, Visconti G (1997) Validation of present-day regional climate simulations over Europe: lam simulations with observed boundary conditions. Clim Dyn 13:489–506
Cox PM, Betts RA, Bunton CB, Essery RLH, Rowntree PR, Smith J (1999) The impact of new land surface physics on the GCM simulation of climate and climate sensitivity. Clim Dyn 15:189–203
Gao X, Shi Y, Song R, Giorgi F, Wang Y, Zhang D (2008) Reduction of future monsoon precipitation over China: comparison between a high resolution RCM simulation and the driving GCM. Meteorol Atmos Phys 100:73–86. doi:10.1007/s00703-008-0296-5
Giorgi F, Mearns LO (1999) Introduction to special section: regional climate modelling revisited. J Geophys Res 104:6335–6352. doi:10.1029/98JD02072
Giorgi F, Bi X, Pal JS (2004a) Mean, interannual variability and trends in a regional climate change experiment over Europe. I. Present-day climate (1961–1990). Clim Dyn 22:733–756. doi:10.1007/s00382-004-0409-x
Giorgi F, Bi X, Pal JS (2004b) Mean, interannual variability and trends in a regional climate change experiment over Europe. II: climate change scenarios (2071–2100). Clim Dyn 23:839–858. doi:10.1007/s00382-004-0467-0
Gregory D, Allen S (1991) The effect of convective scale downdrafts upon NWP and climate simulations. Preprints, Ninth Conference on Numerical Weather Prediction, Denver, CO, Amer. Meteor. Soc. pp 122–123
Gregory D, Rowntree PR (1990) A mass flux convection scheme with representation of cloud ensemble characteristics and stability-dependent closure. Mon Weather Rev 118:1483–1506
Holland GJ (1984) On the climatology and structure of tropical cyclones in the Australian southwest Pacific region. Aust Meteor Mag 32:17–31
Hudson DA, Jones RG (2002) Regional climate model simulations of present-day and future climates of Southern Africa, (Note 39). Met Office, Bracknell
Im ES, Park EH, Kwon WT, Giorgi F (2006) Present climate simulation over Korea with a regional climate model using a one-way double-nested system. Theor Appl Climatol 86:187–200. doi:10.1007/s00704-005-0215-3
IPCC (2007) In: Solomon S, Qin D, Manning M, Chen Z, Marquis M, Averyt KB, Tignor M, Miller HL (eds) Climate Change 2007: The Physical Science Basis. Contribution of Working Group I to the Fourth Assessment Report of the Intergovernmental panel on Climate Change. Cambridge University Press, Cambridge, UK and New York, USA, pp 881–996
Islam MN, Rafiuddin M, Ahmed AU, Kolli RK (2007) Calibration of PRECIS in employing future scenarios in Bangladesh. Int J Climatol 28:617–628. doi:10.1002/joc.1559
Jacob D, Bärring L, Christensen OB, Christensen JH, Castro M, Déqué M, Giorgi F, Hagemann S, Hirschi M, Jones R, Kjellström E, Lenderink G, Rockel B, Sánchez E, Schär C, Seneviratne SI, Somot S, Ulden A, Hurk B (2007) An inter-comparison of regional climate models for Europe: model performance in present-day climate. Clim Chang 81:31–52. doi:10.1007/s10584-006-9213-4
Jones RG, Noguer M, Hassell DC, Hudson D, Wilson SS, Jenkins GJ, Mitchell JFB (2004) Generating high resolution climate change scenarios using PRECIS. Met Office Hadley Centre, Exeter
Juneng L, Tangang FT, Reason CJC (2007) Numerical case study of an extreme rainfall event during 9–11 December 2004 over the east coast of Peninsular Malaysia. Meteorol Atmos Phys 98:81–98
Kalnay E, Kanamitsu M, Kistler R, Collins W, Deaven D, Gandin L, Iredell M, Saha S, White G, Woollen J, Zhu Y, Leetmaa A, Reynolds R, Chelliah M, Ebisuzaki W, Higgins W, Janowiak J, Mo KC, Ropelewski C, Wang J, Jenne R, Joseph D (1996) The NCEP/NCAR 40 year reanalysis project. Bull Am Meteorol Soc 77:437–471
Konare A, Zakey AS, Solomon F, Giorgi F, Rauscher S, Ibrah S, Bi X (2008) A regional climate modelling study of the effect of desert dust on the west African monsoon. J Geophys Res 113:D12206. doi:10.1029/2007JD009322
Kotroni V, Lykoudis S, Lagouvardos K, Lalas D (2008) A fine resolution regional climate change experiment for the eastern Mediterranean: analysis of the present climate simulations. Glob Planet Chang 64:93–104. doi:10.1016/j.gloplacha.2008.10.0003
Kumar RK, Sahai AK, Kumar KK, Patwardhan SK, Mishra PK, Revadekar JV, Kamala K, Pant GB (2006) High-resolution climate change scenarios for India for the 21st century. Curr Sci 90:334–345
Marengo J, Cavalcanti IFA, Satyamurty P, Trosnikov I, Nobre CA, Bonatti JP, Camargo H, Sampaio G, Sanches MB, Manzi AO, Castro CAC, D’almeida C, Pezzi LP, Candido L (2003) Assessment of regional seasonal rainfall predictability using the CPTEC/COLA atmospheric GCM. Clim Dyn 21:459–475
Marengo JA, Jones R, Alves LM, Valverde MC (2009) Future change of temperature and precipitation extremes in South America as derived from the PRECIS regional climate modelling system. Int J Climatol 29:2241–2255. doi:10.1002/joc.1863
Mitchell TD, Jones (2005) An improved method of constructing a database of monthly climate observations and associated high-resolution grids. Int J Climatol 25:693–712
Moberg A, Jones PD (2004) Regional climate model simulations of daily maximum and minimum near-surface temperature across Europe compared with observed station data 1961–1990. Clim Dyn 23:695–715. doi:10.1007/s00382-004-0464-3
Neale R, Slingo J (2003) The Maritime Continent and its role in the global climate: a GCM study. J Clim 16:834–848
New M, Lister D, Hulme M, Makin I (2002) A high-resolution data set of surface climate over global land areas. Clim Res 21:1–25
Raisanan J, Hansson U, Ullerstig A, Doscher R, Graham LP, Jones C, Meier HEM, Samuelsson P, Willen U (2004) European climate in the late twenty first century regional simulations with two driving global models and two forcing scenarios. Clim Dyn 22:13–31. doi:10.1007/s00382-003-0365-x
Ramage CS (1971) Monsoon meteorology. International Geophysics Series, vol 15. Academic Press, San Diego, CA
Salimun E, Tangang FT, Juneng L (2010) Simulation of heavy precipitation episode over eastern Peninsular Malaysia using MM5: sensitivity to cumulus parameterization schemes. Meteorog Atmos Phys 107:33–49
Segele ZT, Leslie LM, Lamb PJ (2008) Evaluation and adaptation of a regional climate model for the Horn of Africa: rainfall climatology and interannual variability. Int J Climatol 29:47–65. doi:10.1002/joc.1681
Seguí PQ, Ribes A, Martin E, Habets F, Boé J (2010) Comparison of three downscaling methods in simulating the impact of climate change on the hydrology of Mediterranean basins. J Hydrol 383:111–124. doi:10.1016/j.jhydrol.2009.09.050
Simmons AJ, Burridge DM (1981) An energy and angular-momentum conserving finite-difference scheme and hybrid coordinates. Mon Wea Rev 109:758–766
Simon W, Hassel D, Hein D, Jones R, Taylor R (2009) Installing and using the Hadley Centre Regional Climate modelling system, PRECIS, Version 1.8.2, Met Office Hadley Centre, Exeter
Solman SA, Nuñez MN, Cabré MF (2008) Regional climate change experiments over southern South America. I: present climate. Clim Dyn 30:533–552
Sylla MB, Gaye AT, Pal JS, Jenkins GS, Bi XQ (2009) High-resolution simulations of west African climate using regional climate model (RegCM3) with different lateral boundary conditions. Theor Appl Climatol 98:293–314
Sylla MB, Coppola E, Mariotti L, Giorgi F, Ruti PM, Aquila AD, Bi X (2010) Multiyear simulation of the African climate using a regional climate model (RegCM3) with the high resolution ERA40-interim reanalyses. Clim Dyn 35:231–247. doi:10.1007/s00382-009-0613-9
Tangang FT, Juneng L (2004) Mechanisms of Malaysia rainfall anomalies. J Climate 17(18):3615–3621
Tangang FT, Juneng L, Ahmad S (2007) Trend and interannual variability of temperature in Malaysia: 1961–2002. Theor Appl Climatol 89:127–141
Tangang FT, Juneng L, Salimun E, Vinayachandran PN, Seng YK, Reason CJC, Behera SK, Yasunari T (2008) On the role of the northeast cold surge, the Borneo vortex, the Madden-Jullian Oscillation, and the Indian Ocean Dipole during the extreme 2006/2007 flood in the southern Peninsular Malaysia. Gephys Res Lett 35(14):1–6. doi:10.1029.2008GL033429
Uppala SM, Kållberg PW, Simmons AJ, Andrae U, Costa Bechtold V, Fiorino M, Gibson JK, Haseler J, Hernandez A, Kelly GA, Li X, Onogi K, Saarinen S, Sokka N, Allan RP, Andersson E, Arpe K, Balmaseda MA, Beljaars ACM, van de Berg L, Bidlot J, Bormann N, Caires S, Chevallier F, Dethof A, Dragosavac M, Fisher M, Fuentes M, Hagemann S, Hólm E, Hoskins BJ, Isaksen L, Janssen PAEM, Jenne R, McNally AP, Mahfouf JF, Morcrette JJ, Rayner NA, Saunders RW, Simon P, Sterl A, Trenberth KE, Untch A, Vasiljevic D, Viterbo P, Woollen J (2005) The ERA40 re-analysis. Q J R Meteorol Soc 131:2961–3012. doi:10.1256/qj.04.176
Wilson M, Henderson-Sellers A (1985) A global archive of land cover and soils data for use in general circulation climate models. J Clim 5:119–143
Acknowledgments
This research is funded by UKM Research Grants UKM-GUP-ASPL-08-05-218, UKM-AP-PI-18-2009/2 and LRGS/TD/2011/UKM/PG/01. The authors wish to thank the Hadley Centre for proving the PRECIS modelling system for the Research Centre for Tropical Climate Change System, Universiti Kebangsaan Malaysia. Also, the availability of the CRU gridded precipitation data from the Climate Research Unit, University of East Anglia is acknowledged.
Author information
Authors and Affiliations
Corresponding author
Rights and permissions
About this article
Cite this article
Kwan, M.S., Tangang, F.T. & Juneng, L. Present-day regional climate simulation over Malaysia and western Maritime Continent region using PRECIS forced with ERA40 reanalysis. Theor Appl Climatol 115, 1–14 (2014). https://doi.org/10.1007/s00704-013-0873-5
Received:
Accepted:
Published:
Issue Date:
DOI: https://doi.org/10.1007/s00704-013-0873-5