Abstract
Lethal carnivore management is a prevailing strategy to reduce livestock predation. Intensity of lethal management varies according to land-use, where carnivores are more intensively hunted on farms relative to reserves. Variations in hunting intensity may result in the formation of a source–sink system where carnivores disperse from high-density to low-density areas. Few studies quantify dispersal between supposed sources and sinks—a fundamental requirement for source–sink systems. We used the black-backed jackal (Canis mesomelas) as a model to determine if heterogeneous anthropogenic mortality induces a source–sink system. We analysed 12 microsatellite loci from 554 individuals from lightly hunted and previously unhunted reserves, as well as heavily hunted livestock- and game farms. Bayesian genotype assignment showed that jackal populations displayed a hierarchical population structure. We identified two genetically distinct populations at the regional level and nine distinct subpopulations at the local level, with each cluster corresponding to distinct land-use types separated by various dispersal barriers. Migration, estimated using Bayesian multilocus genotyping, between reserves and farms was asymmetric and heterogeneous anthropogenic mortality induced source–sink dynamics via compensatory immigration. Additionally some heavily hunted populations also acted as source populations, exporting individuals to other heavily hunted populations. This indicates that heterogeneous anthropogenic mortality results in the formation of a complex series of interconnected sources and sinks. Thus, lethal management of mesopredators may not be an effective long-term strategy in reducing livestock predation, as dispersal and, more importantly, compensatory immigration may continue to affect population reduction efforts as long as dispersal from other areas persists.
Similar content being viewed by others
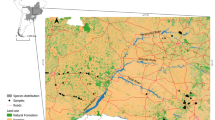
Avoid common mistakes on your manuscript.
Introduction
Carnivores are extensively hunted for several reasons, including the protection of human lives, livestock, or preferred ungulate populations; or for disease control, recreation, or fur and body parts. Besides the obvious and immediate effects on population size, such anthropogenic mortality will also affect the behaviour, social structure (e.g. lions, Panthera leo, Snyman et al. 2015), demography (black-backed jackal, Canis mesomelas, Minnie et al. 2016b), reproduction (e.g. black-backed jackal, Minnie et al. 2016b; coyotes, Canis latrans, Sacks et al. 2005) and dispersal (e.g. cougar, Puma concolor, Stoner et al. 2013) of these carnivores. Anthropogenic mortality often varies considerably across the landscape because of variation in accessibility to humans, land-use and tenure (Novaro et al. 2005). Additionally, anthropogenic mortality may also vary according to local hunting legislation where a species is hunted on one side of a socio-political boundary and protected on the other side (e.g. Gervasi et al. 2015). These unevenly distributed hunting pressures create habitats of varying quality and localised mortality, which play a major role in shaping animal populations.
Local variation in mortality may induce a source–sink population structure (Pulliam 1988). Source–sink dynamics emphasise the effects of dispersal on local population dynamics which result from differences in habitat quality (Elmhagen and Angerbjörn 2001). High quality habitats support populations with growth rates that are either positive or balanced by emigration (source populations; Hanski and Gilpin 1991), whereas populations occupying low quality habitats may become extinct if individuals do not immigrate from elsewhere (sink populations; Hanski and Gilpin 1991). Therefore, source and sink populations can be identified based on net dispersal, with source populations exporting individuals to sink populations (Andreasen et al. 2012; Pulliam 1988).
In many cases, carnivore dispersal is driven by disparities in localised anthropogenic mortality, where individuals disperse from low mortality areas to the high mortality areas (i.e. compensatory immigration, Pulliam 1988). This compensatory immigration has been documented for several carnivores, where unevenly distributed hunting pressures induce source–sink dynamics (e.g. wolverines, Gulo gulo, Gervasi et al. 2015; culpeo foxes, Pseudalopex culpaeus, Novaro et al. 2005; lynx, Lynx lynx, Slough and Mowat 1996). Additionally, spatial variation in anthropogenic mortality and compensatory immigration often play a fundamental role in the sustainable harvest of carnivore populations (e.g. culpeo foxes, Novaro et al. 2005), but may also counter management actions aimed at reducing carnivore densities (e.g. coyote, Knowlton et al. 1999). According to habitat selection theory (Rosenzweig 1981), compensatory immigration should be driven by intraspecific competition which facilitates the dispersal of subordinates from their natal ranges. This process is magnified in highly territorial animals, where density-dependent intraspecific competition (i.e. ideal despotic model, Fretwell 1972) will decrease the fitness of subordinates in high density source habitats, thereby increasing emigration into low density sink habitats, where dispersing individuals may experience increased fitness (Pulliam 1988).
In addition to the effects of localised mortality on dispersal, anthropogenic (e.g. fences and roads) and natural (e.g. mountains and rivers) landscape features have been shown to constrain the inter-habitat dispersal of carnivores, ranging from American mink (Neovison vison, Zalewski et al. 2009) to brown bears (Ursus arctos, Mateo-Sánchez et al. 2014). These dispersal barriers may constrict or direct dispersal (e.g. cougar, Stoner et al. 2013), influencing the net movement of individuals from the source to the sink population. Thus, the formation of a source–sink system is dependent not only on variations in local mortality (e.g. heterogeneous hunting pressure), but also on landscape connectivity and the permeability of potential dispersal barriers.
Black-backed jackals (hereafter jackal) are ubiquitous across southern Africa, occurring in all habitat types and most land-use types except forests and urban areas (Minnie et al. 2016a), suggesting that jackals may have few barriers to dispersal (Ferguson et al. 1983). Additionally, jackals are the dominant predators on livestock farms (van Niekerk 2010) and play a large role in ungulate predation on game farms. Consequently, jackals are aggressively hunted. For example, van Niekerk (2010) surveyed 350 livestock farmers within the study region (7.9% of small livestock farmers in the region), and 73% of the sample used lethal management, killing 8582 jackals between 2006 and 2007. These farmers believe that lethal management is effective in reducing population sizes and associated livestock and ungulate losses on these farms (van Niekerk 2010). Conversely, jackals on reserves are infrequently hunted—when they are hunted it is at a much lower intensity than surrounding farms—thereby creating a landscape with local variations in anthropogenic mortality (see “Methods”). A recent study investigating the demographics of jackals on farms and reserves suggests that this variation in anthropogenic mortality leads to the formation of a source–sink system, which may counter management actions aimed at reducing jackal densities and associated livestock losses on farms (Minnie et al. 2016b). A large number of demographic studies on hunted carnivores draw similar conclusions (e.g. cougar, Stoner et al. 2006; coyote, Tremblay et al. 1998). However, few studies quantify the dispersal of individuals between supposed sources and sinks—a fundamental requirement for the formation of a source–sink system (Elmhagen and Angerbjörn 2001). This is due to the logistical constraints on estimating dispersal at an appropriate scale (Storfer et al. 2010). The advent of modern molecular techniques makes estimating dispersal feasible (e.g. jackals, James et al. 2017), allowing us to effectively address metapopulation processes operating at larger spatial scales (Oliver et al. 2016).
Here, we use multilocus Bayesian genetic techniques to investigate the influence of variation in anthropogenic mortality and potential dispersal barriers on the genetic structure and dispersal of jackal populations associated with distinct land-uses (James et al. 2017). Dispersal of jackals from reserves into habitats with high levels of anthropogenic mortality (farms) may negate population reduction efforts and contribute to the persistence of jackals (Minnie et al. 2016b; van Niekerk 2010). However, empirical evidence in support of this hypothesis is non-existent. Therefore, we hypothesise that lightly- or unhunted areas (reserves) will act as source populations, exporting individuals (net emigration) to heavily hunted areas (livestock- and game farms) that act as sink populations (net immigration), resulting in the formation of genetically distinct subpopulations coinciding with these land-use types. Alternatively, owing to the ubiquitous distribution of this generalist mesopredator, we hypothesise that the highly vagile nature of jackals (Ferguson et al. 1983; Humphries et al. 2016; James et al. 2017) will result in the formation of genetically indistinct non-equilibrium metapopulations. Second, we hypothesise that dispersal will occur between sources and sinks irrespective of potential barriers, as suggested by Ferguson et al. (1983).
Methods
Study area
The study area is located in a semi-arid (mean annual precipitation: Karoo National Park = 274 mm, Mountain Zebra National Park = 403 mm, Addo Elephant National Park = 401 mm) region of the Eastern- and Western Cape Provinces, South Africa (Fig. 1). The vegetation is dominated by the Nama-Karoo biome which transitions into Grassland in the north-east and Albany Thicket in the south-east (Mucina and Rutherford 2006). Three distinct land-use types occur in the study area. Small livestock farming (sheep, Ovis aries, and goats, Capra aegagrus hircus) is the dominant land-use, with game farms and reserves scattered throughout. Game farms stock indigenous and extralimital wild ungulates, whereas reserves stock indigenous animals only. Several potential barriers that may limit dispersal between land-use types are scattered throughout the study area (Fig. 1). All reserves and some game farms have “predator-proof” electrified fencing designed to prevent the dispersal of animals onto neighbouring areas, which may present a major barrier to jackal dispersal. However, the physical structure of these predator-proof fences may fail due to floods and animals (e.g. warthogs, Phacochoerus africanus) moving through or under the fences, thereby allowing jackals to move across these potential barriers. In contrast, the fencing on livestock farms varies greatly, but is typically not electrified and is, therefore, more permeable to animal movements. Other potential barriers include major tarred roads and rivers (Fig. 1), but Ferguson et al. (1983) documented that an individual jackal dispersed over tarred roads and a major river. However, the effects of these potential dispersal barriers at the population level are unknown.
Study area indicating jackal sample locations in relation to reserves. The white dots represent individuals assigned to the KrNP Cluster and the grey dots represent individuals assigned to the Eastern Cluster (k = 2 from Global structure analysis). Population codes: KrNP Karoo National Park, NWF North-West Farm, NEF North-East Farm, NWG North-West Game Farm, MZNP Mountain Zebra National Park, SWF South-West Farm, SEF South-East Farm, SEG South-East Game Farm, K-AENP Kuzuko section of the Addo Elephant National Park, N-AENP Nyathi section of the Addo Elephant National Park
No quantitative information on the variation in hunting intensity between land-uses and the effects on population size exists (Minnie et al. 2016a). However, we base the differentiation in hunting intensity between land-uses on the fundamental difference in management regime. On livestock- and game farms jackals cause substantial losses in livestock and game production (Kerley et al. 2017; Minnie et al. 2016a; van Niekerk 2010). Thus, there is extreme intolerance of jackals and they are aggressively and continuously hunted to reduce population size and the associated livestock losses (Minnie et al. 2016a). In fact, the intolerance is so severe that the use of aerial gunning and professional hunters to reduce jackal densities are becoming commonplace (du Plessis 2013; van Niekerk 2010). Thus, hunting pressure on farms is very high. In contrast, the reserves represent conservation areas in which jackals are infrequently managed. It is important to note that jackal hunting on these reserves is not a standard practise, and hunting is not intended to eradicate jackal from the reserves, unlike on livestock- and game farms. Thus, hunting pressure on reserves is relatively low.
Reserve samples, represented by National Parks, were collected from three conservation areas: Karoo National Park (KrNP, size 884 km2), Mountain Zebra National Park (MZNP, 284 km2) and two isolated sections of the Addo Elephant National Park (AENP)—Kuzuko (K-AENP, 160 km2) and Nyathi (N-AENP, 140 km2)—separated by the Zuurberg and Kabouga Sections and predator-proof electrified fencing. Jackal populations in these reserves are generally unmanaged, but samples became available during experimental population reductions conducted by South African National Parks (South African National Parks project number: GAYA1167), aimed at investigating the impacts of jackal predation on small antelope species. MZNP and N-AENP have never been hunted before, whereas jackals in KrNP and K-AENP were hunted 3 years prior to sample collection. Accordingly, samples collected from these reserves may thus not represent unmanaged populations. However, the demographic structure of KrNP and K-AENP populations is similar to those of the other previously unmanaged reserve populations (Minnie et al. 2016b), suggesting that these populations may have recovered from the initial hunting perturbation or that the scale of previous hunting may have been insufficient to influence jackal populations. Similar patterns have been documented for intensively hunted (80–100 individuals per year) red foxes (Vulpes vulpes), where individuals collected 5–6 years apart were genetically similar, suggesting that reproduction instead of immigration maintained the population (Sacks et al. 2016). However, sustaining such high levels of hunting over a longer time frame may substantially reduce local population densities, and consequently favour immigration from neighbouring populations (Sacks et al. 2016). Therefore, we refer to reserves as lightly hunted and livestock- and game farms as heavily hunted.
Sample collection and microsatellite analysis
Jackals, killed as part of predator control programmes, were collected from managers of reserves (n = 238 jackals), livestock farms (n = 227) and game farms (n = 89; Fig. 1). Reserve samples were collected September–October 2013 and livestock- and game farm samples were collected August 2011–August 2013. The jackals on farms and reserves were killed for management reasons, typically in attempts to reduce predation on livestock (most notably sheep and goats) and wild ungulates (e.g. springbok, Antidorcas marsupialis). Thus, all the samples used for this study are a by-product of a predator management activities on reserves, livestock- and game farms, and the use of these samples was approved by the Nelson Mandela University Animal Ethics Committee (A14-SCI-ZOO-005). The majority of jackals used in this study were hunted using the same approach on reserves and farms (i.e. call-and-shoot), thereby reducing any potential biases in sample collection. We attempted to collect muscle tissue samples from jackal carcases within 8 h of death. If this was not possible, the carcasses were stored at 2 °C for no longer than 3 days prior to tissue extraction. Tissue samples were collected from the thigh muscle and preserved in absolute ethanol (99%) in sterilised DNA tubes at room temperature until analysis.
DNA was extracted using Qiagen DNeasy® Blood & Tissue extraction kits according to manufacturer’s protocol. Fourteen microsatellite loci, selected from the domestic dog (Canis lupus familiaris) library, were used to genotype individuals: CXX.130, CXX.204, CXX.213, CXX.225, CXX.250, CXX.253 (Ostrander et al. 1993), CXX.466 (Ostrander et al. 1995), FH2001, FH2010, FH2017, FH2054, FH2088, FH2096 (Francisco et al. 1996), vWF (Shibuya et al. 1994). Microsatellites were amplified via polymerase chain reactions (PCR) in three multiplex reactions (set 1—FH2096, FH2054, CXX130, FH2010, FH2017; set 2—CXX253, FH2001, CXX250, CXX225; set 3—CXX288, CXX213, vWF, CXX204, CXX466) using a Qiagen Multiplex PCR Kit following the manufacturer’s protocol. PCR amplification was performed using forward primers labelled with 6-FAM, VIC, PET, NED fluorescent dyes. Reaction mixtures contained approximately 1.0 μl (20–40 ng) of template DNA in a total volume of 5.0 μl. The thermal cycle, performed in a DNA Engine Dyad Peltier Thermal Cycler (BIO-RAD), consisted of an initial denaturalisation step at 95 °C for 15 min, followed by 30 cycles at 94 °C for 30 s, 60 °C (for set 1) and 58 °C (for sets 2 and 3) for 1.5 min, and 72 °C for 1 min, and then a final extension period of 30 min at 60 °C. The amplified fragments were resolved by electrophoresis using the ABI 3130XL Genetic Analyser (Applied Biosystems) and allele size scored against a GeneScan 500 LIZ size standard (Applied Biosystems) using genemarker v1.85. The programme micro-checker v2.2.3 (van Oosterhout et al. 2004) was used to test for stuttering, large allele dropout, or the presence of null alleles.
Genetic clustering analyses
Bayesian genotype assignment methods were used to assess genetic population structure. The programme structure (Pritchard et al. 2000) was used to assign individuals (q) to populations (k, Pritchard et al. 2000). We did not use a priori geographic information to assign individuals to specific clusters, which allowed for the unbiased assessment of population structure. We ran an admixture model for uncorrelated alleles with a burn-in of 104 Markov chain Monte Carlo (MCMC) iterations, followed by 204 iteration for k 1–10 with 10 replicates for each k (Andreasen et al. 2012). The most likely number of genetic clusters was determined by evaluating Evanno’s K (Evanno et al. 2005) via the programme structure harvester (Earl and von Holdt 2012). To test for the highest level of structure within the sampled population, we ran a Global model incorporating all the individuals. This resulted in the delimitation of two genetic clusters (k = 2; KrNP vs. Eastern Cluster; see “Results”). Thereafter, we removed KrNP, and following the Δk hierarchical procedure of Coulon et al. (2008), re-ran structure using the same parameter set as above (except for k 1–14) to detect any hierarchical population structure in the Eastern Cluster (EC). Individuals assigned to a group other than the sampled location were considered to be potential migrants (posterior probability, q ≥ 0.75; Sacks et al. 2016).
According to Puechmaille (2016), structure does not provide reliable estimates of k when sampling is uneven, as was the case here (Table 1). Therefore, we estimated true k by randomly subsampling 20 individuals from each sampling location. Thereafter, we re-ran structure using the same parameter set as the global model above, except for k 1–12, in addition to using a priori sampling locations to assign individuals to clusters (Puechmaille 2016). The most likely k was determined in the R-package kestimator v1.12 (Puechmaille 2015) by estimating the median of medians (MedMedK) and maximum of medians (MaxMedK) over 20 replicates for each k value (Puechmaille 2016). MedMedK and MaxMedK were used because these estimators are less sensitive to the presence of migrants (Puechmaille 2016). Sampling locations were assigned to clusters when their median membership coefficient was greater than 0.5, and the maximum of these values represented the most likely k (Puechmaille 2016).
Genetic diversity
We assessed deviations from Hardy–Weinberg equilibrium (HWE) for each cluster by using Fisher’s exact tests implemented in genepop v4.1 (Raymond and Rousset 1995; Rousset 2008) and P values were adjusted for multiple comparisons using Bonferroni corrections. Linkage disequilibrium (LD) between locus pairs, within and across clusters, was assessed using genepop v4.1. Population level genetic variability, as calculated in genalex v6.5 (Peakall and Smouse 2012) and fstat v2.9.3.2 (Goudet 1995), was quantified by the number of alleles (A), allelic richness (Ar), observed (HO) and expected (HE) heterozygosities and inbreeding coefficients (FIS). Pairwise population FST comparisons and associated P values were estimated via permutation in fstat v2.9.3.2 (Goudet 1995).
Dispersal and source–sink structure
We used the overlay approach of landscape genetics to visually inspect for spatial coincidences of genetic cluster boundaries with obvious landscape structures (Storfer et al. 2010). To assess whether these landscape features represent putative barriers to dispersal and to identify potential source–sink populations, bimr v1.0 (Faubet and Gaggiotti 2008) was used. bimr estimates the recent rates of movement between clusters by measuring the genetic disequilibrium generated by migration (Faubet and Gaggiotti 2008). Here, bimr was run twice (1: KrNP vs. EC; 2: EC only) using the same parameter set to compare dispersal between clusters. Initially, 20 pilot runs with 1000 iterations were run to allow the programme to obtain acceptance rates of 25–45%. Thereafter, 20 replicates were run with a burn-in of 106 iterations, which were discarded. Following Andreasen et al. (2012), 20,000 samples from each of the 20 replicates were collected using a thinning interval of 100 iterations. Parameter estimates were extracted from the run that produced the lowest Bayesian deviance (Dassign, Faubet and Gaggiotti 2008). We used 95% HDPIs to assess the significance of asymmetrical migration rates for each pairwise migration rate estimate. In addition, the significance of asymmetrical migration rates was assessed, for each step in the MCMC, by the proportion of times an estimate of migration was less than or greater than the corresponding estimated migration rate in each pairwise comparison (Fordyce et al. 2011). The migrants identified by bimr were compared to those of identified by structure to assess concordance.
Results
Descriptive statistics
A total of 554 jackals across 10 sampling sites (Table 1) were genotyped at 14 microsatellite loci. According to micro-checker, two loci (CXX.130 and CXX.225) had null alleles and were removed from all analyses. All remaining loci were polymorphic, with a mean of 5.8 alleles per locus (range 2–10; Table A1). Allelic diversity (A, Ar), and heterozygosity (HO, HE) were similar between sites (Table 1), falling within one standard error of each other. The inbreeding coefficient (FIS) was fairly similar between sites (range − 0.013 to 0.044; Table 1). None of the FIS estimates were significantly (P < 0.0004) different from zero in any of the sampling locations, and an average FIS of 0.02 ± 0.01 across all populations indicates that inbreeding is not apparent. Six sites showed significant (P < 0.004) deviations from Hardy–Weinberg equilibrium (Table 1). Significant LD (P < 0.05) was detected for 137 and 640 of the 660 locus pairs within sites and across all sites, respectively. The extensive LD detected across all sites suggests substantial population structuring, whereas the LD documented within sites suggests immigration of individuals from genetically differentiated sites (Nei and Li 1973).
Genetic clustering
The global structure model (n = 554) grouped individuals into two regional clusters (k = 2) at the highest level of genetic structuring (Fig. 2a). There was strong separation between KrNP and EC, with a small amount of admixture in both clusters (Fig. 3a). Evanno’s K (Δk) indicated that nine genetic clusters were the most likely k for EC (Fig. 2b). These nine clusters (Reserves: MZNP, K-AENP, and N-AENP; Farms: NWF = North-West Farms, NEF = North-East Farms, SWF = South-West Farms, and SEF = South-East Farms; Game Farms: NWG = North-West Game Farm and SEG = South-East Game Farm) correspond to areas with distinct land-uses, and potential dispersal barriers (fences, roads and rivers) between them, as predicted. Although nine genetic clusters were identified in EC, substantial admixing occurred between these clusters, with several individuals being assigned to clusters other than the cluster where they were sampled (Fig. 3c), suggesting a high level of dispersal between these clusters.
Bar plots from structure showing a k = 2 for the global analysis. Thereafter, structure was rerun excluding KrNP, resulting in b k = 3 and c k = 9. Population codes: KrNP Karoo National Park, NWF North-West Farm, NEF North-East Farm, NWG North-West Game Farm, MZNP Mountain Zebra National Park, SWF South-West Farm, SEF South-East Farm, SEG South-East Game Farm, K-AENP Kuzuko section of the Addo Elephant National Park, N-AENP Nyathi section of the Addo Elephant National Park
MedMedK and MaxMedK indicated that structure overestimated the number of clusters, with seven clusters being the most likely k for the global model. KrNP still represented a distinct cluster with the EC cluster including six subpopulations. Notably NWF and NWG clustered together even though, according to FST comparisons, these populations are differentiated (FST = 0.017; P < 0.001; Table 2). Additionally, individuals from NEF and SEF could not be assigned to a specific cluster. The non-assignment of individuals may be ascribed to individuals of mixed ancestry or individuals that immigrated from other differentiated subpopulations (as suggested by the within-population LD results), making it difficult to assign them to a specific cluster (Puechmaille 2016). To test for migration across potential dispersal barriers and for the formation of a hunting-induced source–sink system, we consider k = 10 the most likely number of clusters (supported by Δk and FST results), as grouping different land-uses would detract from this experimental approach.
All pairwise FST comparisons between clusters were significantly greater than zero (P < 0.001), suggesting significant differentiation among sampling sites, as predicted (Table 2). Larger FST values (0.067–0.121) were observed when comparing between regions (KrNP vs. EC) than within EC (0.017–0.087), indicating that genetic differentiation was much higher between regions than between subpopulations in EC. The largest FST values in the EC were observed when comparing K-AENP (range: 0.032–0.081) and N-AENP (range 0.062–0.087) to all the other sites in the EC. This indicates that considerably less dispersal occurs between these reserves and all the other sites, relative to the dispersal between the other sites in the EC.
Source–sink dynamics
The run with the lowest Dassign indicated that mean migration rates (proportion of the population that migrated within the last generation) between KrNP and EC were low, with asymmetrical migration (P = 0.02) from KrNP to EC (4%; 95% HDPI 0.7–4.1) and almost no back migration (0.6%; 95% HDPI 0.08–3.8). This was in concordance with structure results where one individual (1% of the sampled population) sampled in the KrNP was assigned to the Eastern cluster (EC; posterior probability, q ≥ 0.75) and three individuals (0.7%) sampled in the EC were assigned to KrNP.
bimr was subsequently rerun incorporating only the subpopulations of EC. Mean migration rates were similar across all ten replicate bimr runs. The run with the lowest Dassign indicated that there was substantial dispersal between the nine subpopulations, with significant asymmetrical migration rates (no overlap in 95% HDPIs) identified between many of the subpopulations (Table 3; Fig. 4). None of the lightly hunted reserve subpopulations (MZNP, K-AENP and N-AENP) received any immigrants, but all exported individuals to neighbouring subpopulations (Table 3). Consequently, all the lightly hunted reserve subpopulations were identified as sources, as hypothesised (net emigration; Figs. 4, 5). Additionally, three heavily hunted subpopulations—two farm subpopulations (NEF and SWF) and one game farm subpopulation (SEG)—did not receive any immigrants (Table 3), but exported individuals to other heavily hunted subpopulations. Thus, these subpopulations were identified as source populations (Figs. 4, 5), contrary to our predictions for these land-uses. SEF had the highest immigration rate (70%) followed by NWF (63%) and NWG (38%, Table 3), and although emigration from these subpopulations occurred, these heavily hunted populations were identified as dispersal sinks (net immigration; Figs. 4, 5), as predicted.
Migration asymmetry (mean emigration rate − mean immigration rate) for the Eastern Cluster, estimated using bimr for k = 9. White bars indicate reserves and grey bars indicate livestock- and game farms. Population codes: NWF North-West Farm; NEF North-East Farm, NWG North-West Game Farm, MZNP Mountain Zebra National Park, SWF South-West Farm, SEF South-East Farm, SEG South-East Game Farm, K-AENP Kuzuko section of the Addo Elephant National Park, N-AENP Nyathi section of the Addo Elephant National Park
Genetic clusters (k = 9) and significant asymmetrical migration rates (estimated using bimr; only showing migration > 4%) between subpopulations in the Eastern Region. Polygons represent coarsely outlined subpopulations, and arrows indicate migration direction and rate (width). Light grey polygons represent sink populations, dark grey polygons represent reserve source populations, and dashed polygons represent livestock- and game farm source populations. Population codes: NWF North-West Farm, NEF North-East Farm, NWG North-West Game Farm, MZNP Mountain Zebra National Park, SWF South-West Farm, SEF South-East Farm, SEG South-East Game Farm, K-AENP Kuzuko section of the Addo Elephant National Park, N-AENP Nyathi section of the Addo Elephant National Park
Discussion
Genetic structure
Jackal populations in the Karoo, South Africa display a hierarchical population structure. At the regional level, multiple analyses indicated significant genetic separation and restriction in gene flow between KrNP and the Eastern Cluster populations (EC—incorporating all subpopulations except KrNP; Fig. 3a). This was confirmed by (1) the comparatively high FST values (average = 0.1) between KrNP and all the EC sites, (2) structure analyses which revealed two clusters at the upper level of hierarchical structure and (3) the low proportion of last-generation migrants (Dassign) between KrNP and the EC. The area (154 km between closest sampled individuals, Fig. 1) separating KrNP and EC is dominated by small livestock farming and these farmers hunt jackals to reduce population size and livestock predation on an on-going basis. Therefore, this area represents an extremely hostile environment to jackals, and the chances of jackals eluding farmers in this area is extremely low, thereby limiting gene flow between KrNP and EC. However, as was evident from our results, jackals can disperse over such long distances (in excess of 100 km, Ferguson et al. 1983), thereby maintaining a limited amount of gene flow between regions.
bimr identifies F1 descendants of migrants and estimates the probability that an individual migrated during the previous generation (i.e. recent migration rates, Faubet and Gaggiotti 2008). Thus, regional populations may be connected via step-wise dispersal, where jackals disperse relatively short distances to establish a territory and then reproduce. Thereafter, offspring can repeat the process which may eventually lead to gene flow between these two populations, as reflected in the individuals displaying some level of admixture (Fig. 3a). Accordingly, we hypothesise that the regional source–sink system can operate on a stepping stone basis between generations.
The nine genetically distinct EC subpopulations display significant genetic structuring according to Bayesian Assignment analyses, extensive LD across all sites and pairwise F ST comparisons, this supporting our hypothesis that jackal populations exhibit genetic structure. As predicted, genetically distinct subpopulations are structured according to dispersal barriers (Fig. 5). A large permanent river (separating NEF from the other subpopulations) appears to be the least permeable to jackal dispersal (mean dispersal 2%; range 3–5%) followed by the electrified “predator-proof” fencing of reserves (mean 6%; range 1–19%), livestock fences (mean 8%; range 3–24%) and tarred roads with government-built fences (mean 9%; range 3–24%). Ferguson et al. (1983) radio-tracked jackals and showed that dispersing individuals may disperse over long distances, traversing tarred roads, railway tracks and rivers in the process. Thus, even though these barriers constrained dispersal between subpopulations, none of the barriers were absolute, as predicted. The presence of absolute dispersal barriers would result in the formation of non-equilibrium metapopulations, where dispersal is absent or insufficient to counter local extinctions, due to the deterioration, reduction or fragmentation of habitats (Harrison 1991). This may result in the local extinction of jackal populations due to on-going lethal management, as is the case for many larger carnivores which historically occurred throughout the study area, but are now confined to reserves (e.g. lion). However, the jackal’s ability to traverse these potential dispersal barriers likely contributes to maintaining dispersal between lightly hunted and heavily hunted areas.
Source–sink dynamics
Jackal subpopulations in the Karroo, South Africa, are characterised by intricate dispersal patterns resulting in the formation of a complex series of interconnected sources and sinks (Fig. 5). Even though we identified significant asymmetric dispersal between subpopulations, there was mixed support for our hypothesis that heterogeneous hunting pressure will induce source–sink dynamics. All reserves (both lightly hunted and unhunted) were identified as source populations exporting individuals into heavily hunted sink populations, as expected. Additionally, three heavily hunted subpopulations—two livestock farm and one game farm subpopulations—were also identified as potential sources. We posit that this might be due to an inadequate spatial coverage in sampling locations, as we were not able to sample all the properties/populations in the study area. Similar patterns have been documented for red foxes where a portion of the sampled population was assigned to another unsampled population (Sacks et al. 2016). Further, this hypothesis is supported by the within-population migration rates (m = 1; Table 3) which indicates that none of the sampled individuals immigrated into these populations. Therefore, the heavily hunted populations that were identified as sources (NEF, SWF and SEG) may represent both a source and a sink depending on the population it is connected to (i.e. relative source/sink, Watkinson and Sutherland 1995). Thus, NEF, SWF and SEG may receive individuals from neighbouring unsampled areas, which may lead to net immigration (i.e. relative sink). Demographic and reproductive data indicate that these subpopulations are in fact sink populations (Minnie Unpublished data; Minnie et al. 2016b). Similarly, we did not collect samples from the farming areas surrounding K-AENP and N-AENP, which were identified as source populations. However, these two subpopulations display the demographic and reproductive characteristics of source populations (Minnie unpublished data; Minnie et al. 2016b), providing further support for their identification as sources (net emigration).
Relying solely on dispersal data would have limited our ability to identify source–sink dynamics. The only population that would have been identified as a source would have been MZNP, owing to adequate spatial coverage in data collection (Fig. 1). However, using a combination of dispersal, demographic and reproductive data allowed us to describe source–sink dynamics operating at larger spatial scales, which provided support for our hypothesis that the spatial variation in hunting intensity induces source–sink dynamics. This highlights the importance of using a combination of dispersal, demographic and reproductive data to adequately describe metapopulation processes operating at larger scales, as well as the identification of source–sink dynamics in larger mammals (Elmhagen and Angerbjörn 2001).
Most source–sink models assume that dispersing animals are able to recognise poor quality habitats and avoid them (ideal free distribution, Fretwell and Lucas 1970), thereby selecting good quality habitats first (optimal habitat selection theory, Rosenzweig 1981). Thus, for a source–sink system to function, there needs to be an underlying mechanism driving dispersal into low quality habitats where mortality exceeds natality (i.e. sinks), as there is no benefit of selecting for low quality habitats. Here, dispersal is likely driven by a combination of two mechanisms.
First, territoriality has been identified as a one of the major drivers of source–sink systems (i.e. ideal despotic model, Fretwell 1972). In a stable social system, such as is assumed to occur on reserves, jackals are highly territorial with dominant pairs defending mutually exclusive territories (Loveridge and Nel 2004). Here, jackal populations are assumed to be close to or at social carrying capacity (i.e. the landscape is saturated with territories). Therefore, density-dependent interference competition reduces habitat quality and forces subordinates onto neighbouring farms (i.e. compensatory immigration, Pulliam 1988). This is facilitated by a disruption in the mutually exclusive social structure via anthropogenic mortality, resulting in a low density of conspecifics on farms. Therefore, similar to other small- to medium sized canids (e.g. red fox, Lieury et al. 2015; Sacks et al. 2016), compensatory immigration is driven by the disparity in densities of conspecifics between heavily hunted and lightly hunted areas, with jackals dispersing from reserves to neighbouring heavily hunted farms.
Second, even though hunting reduces local densities thereby relaxing density-dependent interference competition, there are still resident breeding individuals in these populations (Minnie et al. 2016b). The offspring of these resident pairs may disperse from their natal ranges in search of mates and territories, or remain with their parents to assist with rearing the next litter (Moehlman 1983). Dispersal is thought to be regulated by social intolerances which are mediated by resource availability (Loveridge and Macdonald 2001), but high levels of mortality reduce social ties and dispersal into vacant territories will be favoured (Loveridge and Macdonald 2001). Thus, according to the ideal free distribution (Fretwell and Lucas 1970) and habitat selection theory (Rosenzweig 1981), dispersing individuals should select the closest vacant territory with abundant resources (i.e. as available on heavily hunted farms). Therefore, dispersing jackals in farm populations will remain within or move between heavily hunted farm populations owing to the large number of vacant territories, which results in the relatively high levels of dispersal between heavily hunted areas presented here. Conversely, individuals on reserves will select for farm habitats as farms represent attractive habitats with a low density of conspecifics (i.e. vacant territories) and abundant resources. The selection of habitats perceived to be of good quality, but where high mortality rates are difficult to detect (e.g. anthropogenic mortality) has been described by Delibes et al. (2001b) as “maladaptive habitat selection” leading to the formation of attractive sinks or ecological traps (Delibes et al. 2001a). Here, heavily hunted farms likely represent ecological traps with attractive habitat cues, which facilitate the recruitment of jackals onto heavily hunted farms.
Conclusion
Here, we highlight the applicability of molecular techniques in elucidating prevailing dispersal patterns in response to anthropogenic mortality and barriers, thereby assessing metapopulation processes operating at regional and local spatial scales. The complex intersection of various land-uses (reserves, livestock- and game farms), hunting intensity (heavy, light or none) and dispersal barriers (fences, roads and rivers) resulted in the formation of genetically distinct subpopulations of jackal. Additionally, the spatial variation in anthropogenic mortality induced source–sink dynamics through compensatory immigration from lightly hunted and unhunted reserves into heavily hunted farms, as well as a high level of dispersal between heavily hunted areas. This highlights the importance of taking cognisance of the processes structuring populations when developing conservation and management plans. Many mesopredators, like jackals, are considered pests and are lethally managed to reduce predation on livestock, but are also protected in conservation areas. Therefore, similar to other hunted mesopredators (e.g. dingo, Canis dingo, Allen 2015; coyote, Knowlton et al. 1999; red fox, Lieury et al. 2015; Sacks et al. 2016), compensatory immigration will continue to affect population reduction efforts as long as dispersal from other areas persists.
References
Allen LR (2015) Demographic and functional responses of wild dogs to poison baiting. Ecol Manag Restor 16:58–66. https://doi.org/10.1111/emr.12138
Andreasen AM, Stewart KM, Longland WS, Beckmann JP, Forister ML (2012) Identification of source–sink dynamics in mountain lions of the Great Basin. Mol Ecol 21:5689–5701. https://doi.org/10.1111/j.1365-294X.2012.05740.x
Coulon A et al (2008) Congruent population structure inferred from dispersal behaviour and intensive genetic surveys of the threatened Florida scrub-jay (Aphelocoma coerulescens). Mol Ecol 17:1685–1701. https://doi.org/10.1111/j.1365-294X.2008.03705.x
Delibes M, Ferreras P, Gaona P (2001a) Attractive sinks, or how individual behavioural decisions determine source–sink dynamics. Ecol Lett 4:401–403. https://doi.org/10.1046/j.1461-0248.2001.00254.x
Delibes M, Gaona P, Ferreras P (2001b) Effects of an attractive sink leading into maladaptive habitat selection. Am Nat 158:277–285. https://doi.org/10.1086/321319
du Plessis JJ (2013) Towards the development of a sustainable management strategy for Canis mesomelas and Caracal caracal on rangeland. PhD, University of the Free State, Bloemfontein, South Africa
Earl DA, von Holdt BM (2012) STRUCTURE HARVESTER: a website and program for visualizing STRUCTURE output and implementing the Evanno method. Conserv Genet Resour 4:359–361. https://doi.org/10.1007/s12686-011-9548-7
Elmhagen B, Angerbjörn A (2001) The applicability of metapopulation theory to large mammals. Oikos 94:89–100. https://doi.org/10.1034/j.1600-0706.2001.11316.x
Evanno G, Regnaut S, Goudet J (2005) Detecting the number of clusters of individuals using the software structure: a simulation study. Mol Ecol 14:2611–2620. https://doi.org/10.1111/j.1365-294X.2005.02553.x
Faubet P, Gaggiotti OE (2008) A new bayesian method to identify the environmental factors that influence recent migration. Genetics 178:1491–1504. https://doi.org/10.1534/genetics.107.082560
Ferguson JWH, Nel JAJ, de Wet MJ (1983) Social organization and movement patterns of Black-backed jackals Canis mesomelas in South Africa. J Zool 199:487–502. https://doi.org/10.1111/j.1469-7998.1983.tb05101.x
Fordyce JA, Gompert Z, Forister ML, Nice CC (2011) A hierarchical bayesian approach to ecological count data: a flexible tool for ecologists. PLoS One 6:e26785. https://doi.org/10.1371/journal.pone.0026785
Francisco LV, Langsten AA, Mellersh CS, Neal CL, Ostrander EA (1996) A class of highly polymorphic tetranucleotide repeats for canine genetic mapping. Mamm Genome 7:359–362. https://doi.org/10.1007/s003359900104
Fretwell SD (1972) Populations in a seasonal environment. Princeton University Press, Princeton
Fretwell SD, Lucas HL (1970) On territorial behaviour and other factors influencing habitat distribution in birds. Acta Biotheor 19:16–36. https://doi.org/10.1007/BF01601954
Gervasi V, Brøseth H, Nilsen EB, Ellegren H, Flagstad Ø, Linnell JDC (2015) Compensatory immigration counteracts contrasting conservation strategies of wolverines (Gulo gulo) within Scandinavia. Biol Conserv 191:632–639. https://doi.org/10.1016/j.biocon.2015.07.024
Goudet J (1995) FSTAT (Version 1.2): a computer program to calculate F-statistics. J Hered 86:485–486
Hanski I, Gilpin M (1991) Metapopulation dynamics: brief history and conceptual domain. Biol J Linn Soc 42:3–16. https://doi.org/10.1111/j.1095-8312.1991.tb00548.x
Harrison S (1991) Local extinction in a metapopulation context: an empirical evaluation. Biol J Linn Soc 42:73–88. https://doi.org/10.1111/j.1095-8312.1991.tb00552.x
Humphries BD, Ramesh T, Hill TR, Downs CT (2016) Habitat use and home range of black-backed jackals (Canis mesomelas) on farmlands in the Midlands of KwaZulu-Natal, South Africa. Afr Zool 51:37–45. https://doi.org/10.1080/15627020.2015.1128356
James RS, Scott DM, Yarnell RW, Overall ADJ (2017) Food availability and population structure: how do clumped and abundant sources of carrion affect the genetic diversity of the black-backed jackal? J Zool 301:184–192. https://doi.org/10.1111/jzo.12407
Kerley GIH et al (2017) Livestock predation in South Africa: the need for and value of a scientific assessment. S Afr J Sci. https://doi.org/10.17159/sajs.2017/a0198 (Art. #a0198)
Knowlton FF, Gese EM, Jaeger MM (1999) Coyote depredation control: an interface between biology and management. J Range Manag 52:398–412. https://doi.org/10.2307/4003765
Lieury N et al (2015) Compensatory immigration challenges predator control: an experimental evidence-based approach improves management. J Wildl Manag 79:425–434. https://doi.org/10.1002/jwmg.850
Loveridge AJ, Macdonald DW (2001) Seasonality in spatial organization and dispersal of sympatric jackals (Canis mesomelas and C. adustus): implications for rabies management. J Zool 253:101–111. https://doi.org/10.1017/S0952836901000097
Loveridge AJ, Nel JAJ (2004) Black-backed jackal Canis mesomelas Schreber, 1775 Least Concern (2004). In: Sillero-Zubiri C, Hoffman M, Macdonald DW (eds) Canids: foxes, wolves, jackals and dogs. Status Survey and Conservation Action Plan. IUCN/SSC Canid Specialist Group, Gland, pp 161–166
Mateo-Sánchez MC, Cushman SA, Saura S (2014) Connecting endangered brown bear subpopulations in the Cantabrian Range (north-western Spain). Anim Conserv 17:430–440. https://doi.org/10.1111/acv.12109
Minnie L et al (2016a) A conservation assessment of Canis mesomelas. In: Child MF, Roxburgh L, Do Linh San E, Raimondo D, Davies-Mostert HT (eds) The red list of mammals of South Africa, Swaziland and Lesotho. South African National Biodiversity Institute, Endangered Wildlife Trust, Pretoria
Minnie L, Gaylard A, Kerley GIH (2016b) Compensatory life history responses of a mesopredator may undermine carnivore management efforts. J Appl Ecol 53:379–387. https://doi.org/10.1111/1365-2664.12581
Moehlman PD (1983) Socioecology of silverbacked and golden jackals (Canis mesomelas and Canis aureus). Spec Publ Am Soc Mammal 7:423–453
Mucina L, Rutherford MC (eds) (2006) The vegetation of South Africa, Lesotho and Swaziland. Strelitzia 19. South African National Biodiversity Institute, Pretoria
Nei M, Li W-H (1973) Linkage disequilibrium in subdivided populations. Genetics 75:213–219
Novaro AJ, Funes MC, Walker RS (2005) An empirical test of source–sink dynamics induced by hunting. J Appl Ecol 42:910–920. https://doi.org/10.1111/j.1365-2664.2005.01067.x
Oliver MK, Piertney SB, Zalewski A, Melero Y, Lambin X (2016) The compensatory potential of increased immigration following intensive American mink population control is diluted by male-biased dispersal. Biol Invasions 18:3047–3061. https://doi.org/10.1007/s10530-016-1199-x
Ostrander EA, Sprague GF, Rine J (1993) Identification and characterization of dinucleotide repeat (CA) n markers for genetic mapping in dog. Genomics 16:207–213. https://doi.org/10.1006/geno.1993.1160
Ostrander EA, Mapa FA, Yee M, Rine J (1995) One hundred and one new simple sequence repeat-based markers for the canine genome. Mamm Genome 6:192–195. https://doi.org/10.1007/bf00293011
Peakall R, Smouse PE (2012) GenAlEx 6.5: genetic analysis in Excel. Population genetic software for teaching and research—an update. Bioinformatics 28:2537–2539. https://doi.org/10.1093/bioinformatics/bts460
Pritchard JK, Stephens M, Donnelly P (2000) Inference of population structure using multilocus genotype data. Genetics 155:945–959
Puechmaille SJ (2015) Manual for Kestimator version: 1.12
Puechmaille SJ (2016) The program structure does not reliably recover the correct population structure when sampling is uneven: subsampling and new estimators alleviate the problem. Mol Ecol Resour 16:608–627. https://doi.org/10.1111/1755-0998.12512
Pulliam HR (1988) Sources, sinks, and population regulation. Am Nat 132:652–661. https://doi.org/10.1086/284880
Raymond M, Rousset F (1995) GENEPOP (version 1.2): population genetics software for exact tests and ecumenicism. J Hered 86:248–249. https://doi.org/10.1093/oxfordjournals.jhered.a111573
Rosenzweig ML (1981) A theory of habitat selection. Ecology 62:327–335. https://doi.org/10.2307/1936707
Rousset F (2008) genepop’007: a complete re-implementation of the genepop software for Windows and Linux. Mol Ecol Resour 8:103–106. https://doi.org/10.1111/j.1471-8286.2007.01931.x
Sacks BN, Mitchell BR, Williams CL, Ernest HB (2005) Coyote movements and social structure along a cryptic population genetic subdivision. Mol Ecol 14:1241–1249. https://doi.org/10.1111/j.1365-294X.2005.02473.x
Sacks BN, Brazeal JL, Lewis JC (2016) Landscape genetics of the nonnative red fox of California. Ecol Evol 6:4775–4791. https://doi.org/10.1002/ece3.2229
Shibuya H, Collins BK, Huang TH, Johnson GS (1994) A polymorphic (AGGAAT)n tandem repeat in an intron of the canine von Willebrand factor gene. Anim Genet 25:122. https://doi.org/10.1111/j.1365-2052.1994.tb00094.x
Slough BG, Mowat G (1996) Lynx population dynamics in an untrapped refugium. J Wildl Manag 60:946–961. https://doi.org/10.2307/3802397
Snyman A, Jackson CR, Funston PJ (2015) The effect of alternative forms of hunting on the social organization of two small populations of lions Panthera leo in southern Africa. Oryx 49:604–610. https://doi.org/10.1017/S0030605313001336
Stoner DC, Wolfe ML, Choate DM (2006) Cougar exploitation levels in Utah: implications for demographic structure, population recovery, and metapopulation dynamics. J Wildl Manag 70:1588–1600. https://doi.org/10.2193/0022-541X(2006)70[1588:CELIUI]2.0.CO;2
Stoner DC, Wolfe ML, Mecham C, Mecham MB, Durham SL, Choate DM (2013) Dispersal behaviour of a polygynous carnivore: do cougars Puma concolor follow source–sink predictions? Wildl Biol 19:289–301. https://doi.org/10.2981/12-124
Storfer A, Murphy MA, Spear SF, Holderegger R, Waits LP (2010) Landscape genetics: where are we now? Mol Ecol 19:3496–3514. https://doi.org/10.1111/j.1365-294X.2010.04691.x
Tremblay JP, Crête M, Huot J (1998) Summer foraging behaviour of eastern coyotes in rural versus forest landscape: a possible mechanism of source–sink dynamics. Ecoscience 5:172–182. https://doi.org/10.1080/11956860.1998.11682456
van Niekerk HN (2010) The cost of predation on small livestock in South Africa by medium sized predators. MSc, University of the Free State, Bloemfontein, South Africa
van Oosterhout C, Hutchinson WF, Wills DPM, Shipley P (2004) micro-checker: software for identifying and correcting genotyping errors in microsatellite data. Mol Ecol Notes 4:535–538. https://doi.org/10.1111/j.1471-8286.2004.00684.x
Watkinson AR, Sutherland WJ (1995) Sources, sinks and pseudo-sinks. J Anim Ecol 64:126–130. https://doi.org/10.2307/5833
Zalewski A, Piertney SB, Zalewska H, Lambin X (2009) Landscape barriers reduce gene flow in an invasive carnivore: geographical and local genetic structure of American mink in Scotland. Mol Ecol 18:1601–1615. https://doi.org/10.1111/j.1365-294X.2009.04131.x
Acknowledgements
This project was funded by the International Foundation for Science, the National Wool Growers Association, Red Meat Research and Development South Africa, the Koos and Rona Rupert Education Trust, the Nelson Mandela University (NMU), and the National Research Foundation (NRF) Knowledge, Interchange and Collaboration (KIC). Field transport was provided by the Mazda Wildlife Fund and Bidvest rent-a-car. LM was supported by the German Academic Exchange Service (DAAD) and the NRF joint scholarship programme, NMU postgraduate scholarship, and the Dormehl-Cunningham Scholarship. We would like to thank the following organisations and people that were instrumental in sample collection: South African National Parks, Mohair South Africa, all the farm and reserve managers involved in the project, and all the problem animal control specialists.
Author information
Authors and Affiliations
Contributions
LM and GIHK conceptualised and designed the research. LM collected the data. HZ conducted the laboratory analyses. LM and AZ analysed the data. All authors contributed substantially to the preparation of the manuscript.
Corresponding author
Additional information
Communicated by Janne Sundell.
Electronic supplementary material
Below is the link to the electronic supplementary material.
Rights and permissions
About this article
Cite this article
Minnie, L., Zalewski, A., Zalewska, H. et al. Spatial variation in anthropogenic mortality induces a source–sink system in a hunted mesopredator. Oecologia 186, 939–951 (2018). https://doi.org/10.1007/s00442-018-4072-z
Received:
Accepted:
Published:
Issue Date:
DOI: https://doi.org/10.1007/s00442-018-4072-z