Abstract
Resource selection is a fundamental ecological process impacting population dynamics and ecosystem structure. Understanding which factors drive selection is vital for effective species- and landscape-level management. We used resource selection probability functions (RSPFs) to study the influence of two forms of wolf (Canis lupus) predation risk, snow conditions and habitat variables on white-tailed deer (Odocoileus virginianus), elk (Cervus elaphus) and moose (Alces alces) resource selection in central Ontario’s mixed forest French River-Burwash ecosystem. Direct predation risk was defined as the frequency of a predator’s occurrence across the landscape and indirect predation risk as landscape features associated with a higher risk of predation. Models were developed for two winters, each at two spatial scales, using a combination of GIS-derived and ground-measured data. Ungulate presence was determined from snow track transects in 64 16- and 128 1-km2 resource units, and direct predation risk from GPS radio collar locations of four adjacent wolf packs. Ungulates did not select resources based on the avoidance of areas of direct predation risk at any scale, and instead exhibited selection patterns that tradeoff predation risk minimization with forage and/or mobility requirements. Elk did not avoid indirect predation risk, while both deer and moose exhibited inconsistent responses to this risk. Direct predation risk was more important to models than indirect predation risk but overall, abiotic topographical factors were most influential. These results indicate that wolf predation risk does not limit ungulate habitat use at the scales investigated and that responses to spatial sources of predation risk are complex, incorporating a variety of anti-predator behaviours. Moose resource selection was influenced less by snow conditions than cover type, particularly selection for dense forest, whereas deer showed the opposite pattern. Temporal and spatial scale influenced resource selection by all ungulate species, underlining the importance of incorporating scale into resource selection studies.
Similar content being viewed by others
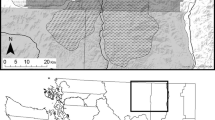
Avoid common mistakes on your manuscript.
Introduction
What drives a species’ resource selection decisions at different spatial scales is essential to understanding its ecology and planning relevant management strategies (Boyce and McDonald 1999). Factors that strongly influence ungulate resource selection include predation (Mech 1977), forage distribution (Fryxell et al. 2004), climatic conditions (Dussault et al. 2004), terrain features (Boyce et al. 2003) and competition (Fretwell and Lucas 1970), all mediated by inter-specific differences in body size and life history (Telfer and Kelsall 1984).
Behavioural responses to the non-lethal risk of predation are important factors shaping the movement patterns and spatial distribution of prey (Lima and Dill 1990). This “ecology of fear” (Brown et al. 1999) may even be more important to herbivore foraging patterns, and thus the spatial distribution of prey, than direct mortality events (Schmitz et al. 1997). Numerous studies have investigated ungulate resource selection (e.g. Telfer 1978; Forbes and Theberge 1993; Johnson et al. 2000; Boyce et al. 2003; Dussault et al. 2005) but relatively few have explicitly taken predator presence into account (e.g. Nelson and Mech 1991; Kunkel and Pletscher 2000; Anderson et al. 2005b) and only recently have researchers used a direct measure of risk in modelling resource selection (e.g. Fortin et al. 2005; Friar et al. 2005; Mao et al. 2005; McLoughlin et al. 2005).
Direct predation risk is measured by the frequency of a predator’s occurrence across the landscape (Fortin et al. 2005; Mao et al. 2005). The predator’s presence, unlinked to any predefined location, creates the necessary initial condition (an encounter) for a predation event. Indirect predation risks arise from landscape features associated with an increased probability of predator presence or prey vulnerability (Hebblewhite et al. 2005). We can then make a theoretical link between these landscape features and the perception of predation risk, independent of the spatio-temporal presence of the predator. For example, gray wolves (Canis lupus) use certain roads, railways and trails for travel (Whittington et al. 2005) because they offer easy routes across the landscape with low associated probabilities of encountering people (Musiani et al. 1998). This can result in a perception of increased predation risk in proximity to these features no matter the actual location of wolves (Bowyer et al. 2003). Similarly, dense coniferous forest is used by both moose (Alces alces) (Kunkel and Pletscher 2000) and elk (Cervus elaphus) (Fortin et al. 2005) to avoid winter wolf predation (lower risk), whereas white-tailed deer (Odocoileus virginianus) are more vulnerable to wolf predation (higher risk) in this habitat (Kunkel and Pletscher 2001). Direct and indirect predation risk measures have recently been incorporated into resource selection studies; however, their differences have not been acknowledged (see Kristan and Boarman 2003; Hebblewhite and Merrill 2007) and it is unclear to which ungulates more readily respond.
In addition to predation risk, ungulates react to a complex suite of climatic, physiographic and vegetative attributes when making habitat decisions (Johnson et al. 2000). For example, due to increased mortality risk for white-tailed deer in deep snow (Nelson and Mech 1986) it has been hypothesized that their northern distribution is strongly affected by winter severity (especially snow depth) whereas moose in the same range, being better able to cope with deep snow, are more affected by browse availability (Telfer 1970).
Heterogeneous resource distribution across the landscape differentially influences the resource selection of both predators and prey at different spatial scales (Orians and Wittenberger 1991; Johnson et al. 2002; Boyce 2006). The confounding effect of spatial scale is an impediment in the development of foundational theory that would allow for the application of conventional behavioural ecology to landscape-level ecological issues (Lima and Zollner 1996). Resource selection is a hierarchical process under which the most limiting factors for a species should be avoided at the largest scale (McLoughlin et al. 2004; Dussault et al. 2005), and continue to dominate selection across finer scales until the next most important limiting factor emerges (Rettie and Messier 2000). Wolf predation can be a limiting factor for white-tailed deer (Messier 1991) and moose (Messier 1991; Van Ballenberghe and Ballard 1998) and is the dominant cause of mortality among reintroduced elk in our study area (Rosatte et al. 2007). Alternately, abiotic environmental factors have been shown to primarily determine broad-scale ungulate distribution and be most strongly selected at the larger scale (Bailey et al. 1996; Boyce et al. 2003). Resource selection is also dependent on temporal scale (Orians and Wittenberger 1991) with factors such as varying winter severity resulting in different selection patterns in the same season over different years (Boyce 2006).
Resource selection probability functions (RSPFs) are functions scaled to determine the probability of use of a resource unit and can be used to investigate scale-dependent resource selection (Manly et al. 2002). We used RSPFs to model white-tailed deer, elk and moose winter resource selection at two scales in 2005 and 2006. The study was conducted in a mixed-forest ecosystem in central Ontario occupied by a single top predator, the eastern Canadian wolf (Canis lupus lycaon).
The primary objective of this study was to determine the importance of wolf predation risk to ungulate resource selection patterns. If wolf predation is the dominant factor limiting ungulate resource selection in this system, we predicted that the best resource selection models for deer, elk and moose would include direct predation risk. Because the most limiting factor should be dominant at the larger scale, this risk should be the most influential selection variable for all species at this scale. At the smaller scale, where secondary limiting factors emerge, we predicted snow depth would feature most prominently in deer models since study area snow depths routinely exceed those considered critical to deer movement (>50 cm; Ungulate Winter Range Technical Advisory Team 2005). These snow depths are rarely sufficient to inhibit moose (60–90 cm), which instead may be limited at fine scales by forage abundance (Jenkins et al. 2007). We therefore predicted cover type, which represents forage availability, would be most important in smaller scale moose models. We predicted direct predation risk would remain the dominant variable in small-scale elk models due to the strong mortality effects of wolf predation on elk (Rosatte et al. 2007). Alternately, if abiotic factors are the primary determinants of ungulate distribution, we would expect to see them more strongly selected at the larger scale (Bailey et al. 1996). Secondly, we wanted to determine whether ungulates actively attempt to minimize the detrimental effects of wolf predation. If so, their occurrence should be negatively associated with direct predation risk as they attempt to avoid the presence of predators. They should also be negatively associated with the study area’s roads, railways and trails, which are low-use features of the type preferred as wolf winter travel corridors, and upon which we routinely found evidence of wolf use. Dense coniferous forest cover should be avoided by deer but have a positive effect on elk and moose presence. Thirdly, we wanted to know whether ungulates respond more strongly to direct or indirect predation risk. If the former, direct predation risk should be ranked more highly in models, particularly at the larger scale, whereas if the latter, the avoidance of linear human infrastructure should be more highly ranked. A final aim was to determine whether species responses to environmental conditions and forage availability depend on species-specific morphology. Due to different morphological characteristics (Telfer and Kelsall 1984) we predicted current snow conditions would be more important than cover type in deer models and the reverse true in moose models. We further predicted deer would be negatively associated with snow depth whereas moose would not, based on typical snowfall levels in the region.
Materials and methods
Study area
The 1,024-km2 study area is located in central Ontario’s French River region, between Lake Nippissing and Georgian Bay (Electronic supplementary material, S1), based on the established home range extents of four adjacent wolf packs. It includes the southern extremity of Killarney Provincial Park and is in the transition zone between the eastern Great Lakes lowland and the boreal forest terrestrial eco-regions (Ricketts et al. 1999), characterized by temperate, mixed deciduous–conifer forest of birch (Betula sp.), aspen (Populus sp.), oak (Quercus sp.), maple (Acer sp.), pine (Pinus sp.), fir (Abies balsamea) and spruce (Picea sp.). Pockets of eastern hemlock (Tsuga canadensis) and eastern white cedar (Thuja occidentalis) are interspersed. Lakes, streams, bogs and marshes are abundant, as are hollows of gravel and sandy till created by retreating glaciers (Vankat 1979). The topography is flat to rolling with rugged, rounded granite outcrops rising to 300 m (Jost et al. 1999). In the southwest are the La Cloche Mountains, rugged white orthoquartzite and granite outcrop ridges with peaks of almost 450 m. Average winter (December–April) snowfall is 52 cm/month with a snow cover of 40 cm through January and February.
The study area is at the southern range extent of the eastern moose (Alces alces americana) (Bowyer et al. 2003). Regional moose density estimates range from 0.18 to 0.6/km2 [McKenney et al. 1998; Ontario Ministry of Natural Resources (OMNR) 2005]. White-tailed deer are common in the area, their densities unknown but fluctuating seasonally (OMNR 2005). Eastern elk (Cervus elaphus canadensis) were extirpated in Ontario in the 1880s, probably due to habitat destruction and hunting (Bryant and Maser 1982). Since 1998, one hundred and seventy-two elk (Cervus elaphus nelsonii) from Alberta have been released in the French River–Burwash area to restore viable provincial populations (Rosatte et al. 2007). The area encompassing the study site has an elk population of ∼120, estimated by annual helicopter calf-recruitment surveys (J. Hamr, personal communication). Elk are protected from hunting throughout the province whereas deer and moose are subject to seasonal hunting pressure. The wolf population is estimated at 19–27 individuals/1,000 km2, based on pack sightings during trapping and aerial monitoring (G. Desy, unpublished data).
Ungulate surveys
The study area was divided into 64 4 × 4-km quadrats and within each a 3-km, U-shaped line transect was established (Electronic supplementary material, S2). Transect arms were 1-km long at right angles to each other. Surveys were conducted an average of 3.2 days (n = 128; range 1–14 days) after a minimum 5-cm snowfall. Most surveys (91.4%) were conducted within 7 days of snowfall with consecutive surveys spatially separated across the study area. When track identification was uncertain, we followed the animal’s trail until scat was located and positively identified.
Between-winter and spatial scale comparisons
To compare selection between years we surveyed all transects once each in the winter of 2005 (20 January–18 March 2005) and 2006 (29 December 2005–28 March 2006). To investigate the impact of spatial scale we used GIS (ArcView 3.2; ESRI) to delineate 128 1 × 1-km quadrats, centred on the mid-point of each of the two parallel arms of every U-shaped transect (Electronic supplementary material, S2). By reducing the size of resource units to 1/16th that of the larger units we changed the extent at which available resources could be characterized, thus narrowing the spatial domain of selection. This offers the potential to identify selection patterns resulting from small-scale landscape heterogeneity that might be obscured at a larger extent (Boyce 2006).
We used a type 1, design A resource selection study design (Manly et al. 2002) with ungulate presence or absence determined by recording intersecting tracks. An ungulate species was considered present in the 16-km2 quadrat if its tracks were identified anywhere on the 3-km transect, whereas its presence in the 1-km2 quadrat required tracks be identified on the associated 1-km transect arm. No tracks equated to a classification of absent from the given quadrat. An undetected species is not necessarily absent and may be in an un-surveyed part of the resource unit (MacKenzie 2005); however, the transect length combined with the study ungulate’s size and mobility should result in a high detection probability, reducing this bias.
Ungulates exhibit high variation with respect to winter home range size both within and across species. White-tailed deer home ranges in northern New York State average 1.4 km2 (Tierson et al. 1985) and elk ranges in forested areas 28.4 km2 (Anderson et al. 2005a). Average moose home ranges are 5.7 km2 in northwestern Ontario’s boreal forest (Addison et al. 1980) and 7.5 km2 in New York’s Adirondack Mountains (Garner and Porter 1980). Our exact resource unit sizes were arbitrary, but at 16 and 1 km2, the quadrats differ by an order of magnitude and represent two distinct spatial scales. Johnson’s (1980) levels of hierarchical habitat selection include the selection of a species’ range (first order), individual home ranges within the landscape (second order), sites within a home range (third order) and items within a site (fourth order). The 16-km2 quadrats therefore represent second-order selection for elk and moose, but are closer to first-order selection for deer, whereas the 1-km2 quadrats represent third-order selection for elk and moose and second-order selection for deer.
Direct predation risk
This is a measure of the frequency of a predator’s physical occurrence across the landscape, dependent on definitive spatial locations. To get these locations, wolves were captured using rubber-padded leg-hold traps (Livestock Protection; Alpine, Tex., USDA) from August to October of 2003 and 2004, and by helicopter net gunning in February 2004 and 2005, and 15 adults (five male, ten female) from four adjacent packs fitted with a total of 19 GPS collars (Lotek 3300 and 4400S). Collars were identically programmed to record locations every 4 h and the data downloaded during aerial telemetry flights or directly from the collar upon retrieval. To ensure equal pack representation we used the winter data from one collar per pack. Direct predation risk was determined by plotting all locations on a map of the study area (ArcView; ESRI) and determining the proportion of a pack’s total locations in each quadrat. This was multiplied by 1,000 to mimic the true magnitude of observed location numbers:
where Pr(n) is the predation risk for the nth pixel, w i , n is the number of wolf locations from pack i in quadrat n and W i is the total number of wolf locations from pack i. Wolf pack size ranged from two to seven individuals. Since individual wolves frequently separate from and re-join their packs over short temporal periods (Mech 1970) and the ability of ungulates to differentiate group sizes in a forest setting is unknown, we did not weigh risk by pack size. The presence of un-collared wolf packs adjacent to the study area might influence direct predation risk measures in peripheral quadrats; however, there tends to be an unoccupied “no-man’s land” between neighbouring packs which should minimize this potential impact (Mech 1977).
Indirect predation risk
This is represented by landscape features associated with an increased probability of predator presence or prey vulnerability to predators and, unlike its direct form, does not rely on actual predator presence. As wolves used the study area’s low-intensity linear human infrastructure as winter travel corridors, we used the density/distance to roads, railways and trails as sources of indirect predation risk (Anderson et al. 2005b; Whittington et al. 2005). Densities (km/km2) were determined using the Feature Density and Spatial Analyst extensions of ArcView 3.2. To avoid an overabundance of zeros due to a lack of roads/rails and trails in the 1-km2 quadrats, we recorded the distance from the quadrat centre to the nearest road or railway line and trail (see DeCesare and Pletscher 2006). Dense coniferous forest cover proportions were determined with GIS [ArcView 3.2 (ESRI) with Landsat 5, zone 17 map (OMNR) at a 25-m2 pixel resolution].
Other variables
Based on a review of published literature we identified several important additional landscape predictors for white-tailed deer, elk and moose resource selection. These were cover type (Jost et al. 1999; Johnson et al. 2000; Boyce et al. 2003), snow depth, density and crust (Mech et al. 1987; Bowyer et al. 2003), terrain ruggedness (Julander and Jeffrey 1968; Johnson et al. 2000), elevation (D’Eon 2001; Bowyer et al. 2003; Boyce et al. 2003) and availability/proximity of water (Johnson et al. 2000).
Cover type and open water proportions were determined as above for dense coniferous forest. All land cover categories covering <1.5% of the study area were discarded and open and treed fen and bog categories combined into a single wetland category. Linear water and contour (ruggedness) densities (km/km2) were determined in the same way as human infrastructure densities above.
We recorded elevation (m) and UTM coordinates with GPS at seven equally spaced (500 m) points along each transect. Snow characteristics were measured at point 1, 4 and 7 in the winter of 2005 and at all points in the winter of 2006 (Electronic supplementary material, S2). In the 1-km2 analysis only those snow points on the relevant 1-km transects were used for calculations. Snow depth was measured using a 3-inch diameter PVC tube, inserted vertically into the snow until contacting the ground. Quarter-inch gradations marked on the tube exterior allowed snow depths to be read. Upon excavation, the tube’s contents were transferred to a plastic bag of known weight and measured using a hand-held Pesola scale. Measurements were converted to metric and snow density calculated by dividing the snow mass (grams) by its volume (square centimetres) and converting to kilograms per cubic metre. At each location we took five separate samples within a 3-m radius. There is debate as to whether moose and white-tailed deer populations respond to previous years’ and cumulative snow conditions (Mech et al. 1987; Messier 1991, 1995; McRoberts et al. 1995). To account for any possible lags manifest in resource selection behaviour, 2006 model sets included snow measurements from the current winter, previous winter and both winters.
We explicitly included the number of days since snow that surveys were conducted to account for sampling effects. We conducted a correlation analysis using Pearson’s correlation coefficient (r) with all parameters to detect any that were highly correlated. Logistic regression is sensitive to covariate collinearity so when two parameters exhibited r ≥ 0.7, the one deemed least informative, most difficult to accurately measure, or with the most missing data, was eliminated (Hosmer and Lemeshow 2000). The 17 remaining parameters were all continuous (Table 1).
Model development and comparison
We determined four sets of a priori models for each species based on the literature: one for each individual winter (2005 and 2006) at two scales (16 and 1 km2) (Electronic supplementary material, S3). Factors with the potential to influence resource selection for deer, elk and moose overlapped to a degree sufficient to justify the use of the same model sets for all species. To keep model and parameter numbers manageable, we did not include interaction terms a priori. If results indicated possible interactions we conducted post hoc analysis to determine their existence (Hosmer and Lemeshow 2000).
We used binary logistic regression (SPSS version 12.0) to estimate 12 RSPFs, one for each species at both scales in both winters (Manly et al. 2002), as this analysis is appropriate for presence/absence data (Hosmer and Lemeshow 2000; Keating and Cherry 2004). We used the Hosmer and Lemeshow goodness-of-fit test and Nagelkerke’s pseudo R 2 to evaluate general model fit and Akaike’s information criterion for small sample sizes (AICc) to rank models (Burnham and Anderson 2002). To further appraise model fit the sensitivity and specificity of top models were evaluated with receiver operating characteristics, where area-under-the-curve (AUC) values close to 1 indicate excellent predictability whereas those close to 0.5 are no better than random (Boyce et al. 2002).
Comparisons between models for all species at both spatial scales were based on differences in AICc values (Δ i ) (Burnham and Anderson 2002). The best model is not always clearly defined (Δ i < 2), so we determined Akaike weights (w i ) for each model (Burnham and Anderson 2002). To determine the relative importance of variables and further reduce model ambiguity, we summed w i of all models containing a common factor for each analysis. The higher the combined weights for an explanatory variable, the more important it is for the analysis (Burnham and Anderson 2002). For this measure to be meaningful it is necessary to have the same number of models containing each variable (Burnham and Anderson 2002), so we divided the cumulative model weights for a particular variable by the number of models containing that variable to get an average variable weight (w i ) per model.
To discriminate more thoroughly between parameters we used multi-model inference to determine the model averaged parameter estimate \( ({\hat {\bar \theta}}) \) and unconditional SE \( (S\bar E) \) (Burnham and Anderson 2002). Estimates obtained in this way show increased precision and less associated bias than those estimated from a single model (Anderson et al. 2000). To determine the importance of individual variables we calculated the 90% \( ({\hat {\bar \theta}} \pm (1.645) S{\bar E}) \) and 95% \( ({\hat{\bar \theta}} \pm (1.96)S{\bar E}) \) confidence intervals for the model averaged parameter estimates (Mazerolle 2004). When these confidence intervals do not include 0 we can conclude that the given parameter has an effect on the dependent variable (i.e. the estimate is different from 0).
Results
Only models with a substantial level of empirical support (Δ i ≤ 2; Burnham and Anderson 2002) are presented (Table 2). See Electronic supplementary material for complete model set results.
Does wolf predation risk limit ungulate resource use?
Direct predation risk was in 67% (n = 12) of top model sets across species but was never the top weighted variable for an individual analysis (Tables 2, 3). It was influential at the large scale for elk and moose selection, second to elevation, whereas in deer models, was mid-ranked at both scales (Table 3). Ruggedness was the most influential variable in large-scale deer models. At the smaller scale, direct predation risk was never highly ranked (Table 3). The apparent influence of human infrastructure on small-scale deer selection models was due to the 2005 analysis (w i = 0.245), as it was unimportant at this scale in 2006 (w i = 0.000). Current snow condition was second ranked at the larger scale and third ranked at the smaller scale in deer models. Ruggedness was the most important small-scale variable for elk and moose.
Predation risk avoidance
Ungulates did not select resources based on the avoidance of direct predation risk, being negatively associated with this risk in 25% of analyses, never strongly so (Table 4). Deer and elk presence were predominantly positively associated with direct predation risk, and in the larger scale 2006 deer analysis there was a strong positive effect. This risk was weakly and inconsistently associated with moose presence.
Ungulates did not consistently avoid linear human infrastructure (Table 4). Deer and elk were mostly positively associated with these variables, and only moose showed limited signs of avoidance. The response by ungulates to dense coniferous forest was mixed (Table 4). Deer exhibited varying degrees of negative association with this cover type, moose were positively associated with it in both years and at both scales, with association pronounced in 2006, and elk showed no strong selection pattern.
Direct versus indirect predation risk
Direct predation risk was more influential than indirect predation risk (human infrastructure) for all species at all scales, except deer at the small scale, which was due to selection in 2005 (Table 3). Furthermore, human infrastructure was in only 8% (n = 12) of top model sets, compared to 67% for direct predation risk.
Climatic conditions versus habitat
Current snow condition was more important to deer models than cover type whereas the opposite was true of moose models, with cover type appearing in three-quarters of top moose model sets (Tables 2, 3). In both species snow condition was more influential than cover type in 2006, with the reverse observed in 2005. Irrespective of scale, deer consistently selected against habitats with deep, dense snow, and in 2006 this avoidance was pronounced at both scales, consistent with model weighting (Table 4). Moose generally avoided deep, dense snow, and avoidance was small but notable in 2006 at the smaller scale despite a positive association at the larger scale. Current winter snow condition was weighted more heavily than cover type in most elk resource selection analyses but neither was heavily weighted overall. Elk always selected away from areas of dense snow and in 2006 also strongly avoided deep snow.
There was an interaction effect between current snow condition and elevation in the top small-scale 2006 deer model (SNW06, ELE, SD06; Table 2) indicating that these covariates depend upon each other for context (Hosmer and Lemeshow 2000).
Discussion
Does wolf predation risk limit ungulate resource use?
Direct predation risk does not appear a limiting factor for ungulates at the spatial scales investigated despite it being in most top model sets across analyses. Instead, consistent with the theory that abiotic factors primarily determine ungulate distribution at broad scales, topographical features—ruggedness and elevation—were most influential (Bailey et al. 1996; Boyce et al. 2003). These features were also most important to ungulate models at the smaller scale.
Deer selection of rugged terrain at the large scale is consistent with the use of deer yards on the sheltered southern slopes of the La Cloche Mountains. That it was not influential at the smaller scale indicates that features associated with rugged terrain were being selected, not rugged terrain itself (although selected features are dependent on it). Elk showed the opposite pattern, selecting for rugged areas of higher elevation at the smaller scale, consistent with observations of elk resident in the region prior to reintroduction selectively foraging in ridge habitats (Jost et al. 1999) and is perhaps due to a preference for the lower snow cover and greater forage availability on south-facing slopes (Irwin and Peek 1983). Greater variability in 2006 snow conditions probably exacerbated this effect, leading to a stronger positive association between elk and elevation in this year. Similarly, climatic variation was probably responsible for deer’s stronger selection of low elevation areas in 2006, when greater snow depths led to them selecting low elevation deer yards. These yards offer particular factors (i.e. dense cover) that effectively reduce snow depths as well as providing thermal protection and shelter (Morrison et al. 2003).
Habitat selection varies as a consequence of population density (Rosenzweig 1989; McLoughlin et al. 2006). With ∼120 individuals in the study area, elk should be free to utilize the highest quality resources available (Fretwell and Lucas 1970). However, much depends on their ability to disperse and detect new habitats (Fahrig and Paloheimo 1988) which may be hampered when low population density decreases the pressure on individual elk to disperse from initial reintroduction release sites (Matthysen 2005). The gregarious nature of elk and isolation of the study area population may further inhibit dispersal, reducing their range of habitat choices. The opposite effect may influence moose, whereby high population density results in a wider niche breadth, the use of secondary habitats and diluted resource selection (Fretwell and Lucas 1970). We were unable to test the importance of ungulate density on resource selection patterns as the limited spatial distribution of ungulates resulted in models unable to converge at a solution. The relatively low moose model R 2 and AUC values (Table 2) may be a reflection of density-dependent selection deterioration or may be due to different predation risk responses by males and females, confounding selection patterns (Bowyer et al. 2003).
Predation risk avoidance
No species effectively avoided areas of high direct predation risk despite its wide variability across the study area (Table 1), as 75% (n = 12) of analyses resulted in positive associations between this risk and ungulate presence (Table 4). The strong association between deer and direct predation risk in 2006 compared to 2005 (Table 4) probably stems from this winter’s denser snow accumulations (t = 2.843, P < 0.01) and greater variability in snow depth (s2006 = 18.873 vs. s2005 = 9.459) and density (s2006 = 46.369 vs. s2005 = 27.378). This likely inhibited mobility and foraging (Mech et al.1987), forcing deer to congregate in areas of shallower, lighter snow (D’Eon 2001), where wolves typically concentrate hunting activities (Kunkel and Pletscher 2001; Mech and Boitani 2003). Furthermore, in multi-prey systems, wolves select the prey which are smallest or easiest to capture (Mech 1970) and have been observed targeting white-tailed deer in the winter, even when scarce in comparison to moose (Potvin et al. 1988). This indicates a possible tradeoff, whereby deer congregate in areas of heightened predation risk because other factors are more limiting (i.e. forage, shelter) and eclipse the need to minimize this spatial risk (Lima and Dill 1990; Kie 1999). Our direct predation risk measure (frequency of wolf occurrence) represents the probability of a predator encounter and not of being killed, so alternately, congregation in deer yards may be an anti-predator strategy with better mobility reducing an individual’s probability of being killed given a predator encounter (Mech et al. 1987). This is supported by observations that predation is significantly higher for yearlings and adult females outside deer yards (Nelson and Mech 1991). Deer may also be employing other anti-predator strategies such as predation risk dilution or increased overall vigilance in these high-risk areas (Dehn 1990). We encourage further investigation into how the probability of being killed changes relative to snow conditions and the probability of a predator encounter in this study area (see Hebblewhite et al. 2005).
Elk resource selection also resulted in an increased risk of wolf encounters, again signifying a possible tradeoff between forage and/or mobility maximization and predation risk avoidance (Fryxell and Lundberg 1997; Dussault et al. 2005). From 1998 to 2004 22.5% of reintroduced elk were killed by wolves, with wolf predation accounting for 40.6% of total elk mortality (Rosatte et al. 2007). As ∼80% of the region’s elk were recently reintroduced from a predator-free environment, it is possible that they represent a naïve prey source being exploited by the resident wolf packs (Friar et al. 2007).
That moose lack strong or consistent associations with direct predation risk may be because deer and elk are more profitable prey (Weaver 1984). The greater biomass per kill provided by moose may be offset by the increased energy expenditure required per predation event (Weaver 1984), the greater danger posed by their persistent defensive behaviour (Huggard 1993), and the difficulty that relatively small eastern Canadian wolves have capturing them (Mech 1970). The Algonquin Provincial Park wolf population responds through prey utilization and density to fluctuating deer availability despite moose being more consistently available and a major food item (Forbes and Theberge 1996). Detailed wolf diet analysis is needed for further evaluation.
The positive association between deer and human infrastructure may be an artifact of the Killarney Provincial Park trail system, which is close to known deer yards. However, these ski trails are perhaps the most heavily utilized in the study area and, as wolves avoid areas of high human activity (Whittington et al. 2005), deer might be exploiting this avoidance by congregating in proximity to trails to reduce predation risk (Hebblewhite and Merrill 2007). In 2005, when winter conditions were milder, deer were strongly associated with trail density and inconsequentially or negatively associated with direct predation risk (Table 4). Perhaps deer preferentially select these areas to reduce predation risk when snow conditions allow but are forced into higher risk areas when snow depths increase. Deer’s negative association with dense coniferous forest indicates an avoidance of indirect predation risk, probably resulting from the differential predation vulnerability in different habitats (Kunkel and Pletscher 2001; Hebblewhite et al. 2005).
Elk did not avoid indirect sources of predation risk, with human infrastructure associations positive due to the dense road, rail and trail network around Burwash where elk were originally released and still congregate. This variable was the least important to elk resource selection models (Table 3). Instead of dense coniferous forest, elk selected for partially cut and sparse forest (Table 4), where better visibility and more accessible escape routes may offset the increased risk of an encounter (Geist 1982). Alternately, sparse forest was correlated with exposed bedrock (Pearson r = 0.73 at 16-km2 scale and r = 0.55 at 1-km2 scale) which rapidly warms, reflecting solar radiation and quickening snow melt to allow increased access to browse (Gelfan et al. 2004).
Moose did not consistently avoid human infrastructure, and weak model weights indicate that any avoidance observed was not a primary concern (Table 3). They were positively associated with dense coniferous forest, which moose select to avoid predators (Kunkel and Pletscher 2001; Dussault et al. 2005) but also provides shelter and contains winter forage—primarily balsam fir—highly utilized in eastern North America (Ludewig and Bowyer 1985). Deciduous forest offers moose better quality forage (Renecker and Schwartz 1998), so selecting dense coniferous forest indicates a tradeoff with abundant but low-quality forage selected in exchange for a reduced risk of predation. Determining the importance of moose as wolf prey in this system might affect the interpretation of these observed associations.
Direct versus indirect predation risk
Direct predation risk was clearly more influential to ungulate resource selection than indirect predation risk. This despite the fact that an ungulate’s response to the former is reactive since, uncoupled from any spatial reference point it cannot easily be anticipated, whereas sources of indirect predation risk are spatially predictable and can be proactively incorporated into resource-selection decisions. However, direct predation risk exclusively represents an increased mortality risk whereas indirect sources of risk are more ambiguous and may even represent potential fitness gains (i.e. higher forage availability) to offset the risk. These tradeoffs should temper avoidance of many indirect sources of risk. Furthermore, some apparent indirect risks might act to reduce risk in certain contexts (i.e. trails used by wolves when human presence is low might be selected by ungulates to avoid wolves when human presence is high) (Hebblewhite and Merrill 2007).
Climatic conditions versus habitat
Our results support previous observations that winter severity affects deer resource selection more than forage availability, whereas the opposite is true of moose (Telfer 1970; Kearney and Gilbert 1976). The more severe snow conditions in 2006 impacted more on deer selection due to their increased vulnerability to predation in deep snow (DelGiudice et al. 2002), but also influenced moose resource selection. In 2006, twenty-five percent of 16-km2 quadrats were characterized by snow depths inhibitory to moose movement (>60 cm), compared to 4.7% in 2005, and may have forced moose to select areas close to dense cover, which intercepts snow and is preferred by moose across much of their winter range (Forbes and Theberge 1993). At the 1 km2 scale 23.4% of quadrats had snow depths inhibiting to moose in 2006, when their avoidance of deep snow at this scale was marked. In 2005, when snow depth was inhibiting in 9.4% of quadrats, selection was based more on forage requirements with dense deciduous forest selected. That moose were positively associated with snow depth in large-scale 2006 models (Table 4) seems to contradict the selection for dense coniferous forest; however, Dussault et al. (2005) found the best landscape level predictor of moose habitat selection were areas of high quality forage (deciduous) interspersed with areas providing shelter from snow (coniferous).
References
Addison RB, Williamson JC, Saunders BP, Fraser D (1980) Radio-tracking of moose in the boreal forest of northwestern Ontario. Can Field Nat 94:269–276
Anderson DP, Forester JD, Turner MG, Frair JL, Merrill EH, Fortin D, Mao JS, Boyce MS (2005a) Factors influencing female home range sizes in elk (Cervus elaphus) in North American landscapes. Landsc Ecol 20:257–271
Anderson DP, Turner MG, Forester JD, Zhu J, Boyce MS, Beyer H, Stowell L (2005b) Scale dependent summer resource selection by reintroduced elk in Wisconsin, USA. J Wildl Manage 69:298–310
Anderson DR, Burnham KP, Thompson WL (2000) Null hypothesis testing: problems, prevalence, and an alternative. J Wildl Manage 64:912–923
Bailey DW, Gross JE, Laca EA, Rittenhouse LR, Coughenour MB, Swift DM, Sims PL (1996) Mechanisms that result in large herbivore grazing distribution patterns. J Ran Manage 49:386–400
Bowyer RT, Van Ballenberghe V, Kie JG (2003) Moose. In: Feldhamer GA, Thompson BC, Chapman JA (eds) Wild mammals of North America: biology, management, and conservation, 2nd edn. Johns Hopkins University Press, Baltimore, pp 931–964
Boyce MS (2006) Scale for resource selection functions. Divers Distr 12:269–276
Boyce MS, McDonald LL (1999) Relating populations to habitats using resource selection functions. Trends Ecol Evol 14:268–272
Boyce MS, Vernier PR, Nielsen SE, Schmiegelow FKA (2002) Evaluating resource selection functions. Ecol Model 157:281–300
Boyce MS, Mao JS, Merrill EH, Fortin D, Turner MG, Fryxell J, Turchin P (2003) Scale and heterogeneity in habitat selection by elk in Yellowstone National Park. EcoScience 10:421–431
Brown JS, Laundré JW, Gurung M (1999) The ecology of fear: optimal foraging, game theory, and trophic interactions. J Mammal 80:385–399
Bryant LD, Maser C (1982) Classification and distribution. In: Thomas JW, Toweill DE (eds) Elk of North America—ecology and management. Stackpole Books, Harrisburg, pp 1–59
Burnham KP, Anderson DR (2002) Model selection and inference: a practical information-theoretic approach, 2nd edn. Springer, New York
DeCesare NJ, Pletscher DH (2006) Movements, connectivity, and resource selection of Rocky Mountain bighorn sheep. J Mammal 87:531–538
Dehn MM (1990) Vigilance for predators: detection and dilution effects. Behav Ecol Sociobiol 26:337–342
DelGiudice GD, Riggs MR, Joly P, Pan W (2002) Winter severity, survival, and cause-specific mortality of female white-tailed deer in north-central Minnesota. J Wildl Manage 66:698–717
D’Eon RG (2001) Using snow-track surveys to determine deer winter distribution and habitat. Wildl Soc Bull 29:879–887
Dussault C, Ouellet JP, Courtois R, Huot J, Breton L, Larochelle J (2004) Behavioural responses of moose to thermal conditions in the boreal forest. EcoScience 11:321–328
Dussault C, Ouellet JP, Courtois R, Huot J, Breton L, Jolicoeur H (2005) Linking moose habitat selection to limiting factors. Ecography 28:619–628
Fahrig L, Paloheimo J (1988) Determinants of local population size in patchy habitats. Theor Pop Biol 34:194–312
Forbes GJ, Theberge JB (1993) Multiple landscape scales and winter distribution of moose, Alces alces, in a forest ecotone. Can Field Nat 107:201–207
Forbes GJ, Theberge JB (1996) Response of wolves to prey variation in central Ontario. Can J Zool 74:1511–1520
Fortin D, Beyer HL, Boyce MS, Smith DW, Dushesne T, Mao JS (2005) Wolves influence elk movements: behaviour shapes a trophic cascade in Yellowstone National Park. Ecology 86:1320–1330
Fretwell SD, Lucas HL Jr (1970) On territorial behaviour and other factors influencing habitat distribution in birds. I. Theoretical development. Acta Biol 19:16–36
Friar JL, Merrill EH, Visscher DR, Fortin D, Beyer HL, Morales JM (2005) Scales of movement by elk (Cervus elaphus) in response to heterogeneity in forest resources and predation risk. Landsc Ecol 20:273–287
Friar JL, Merrill EH, Allen JR, Boyce MS (2007) Know thy enemy: experience affects translocation success in risky landscapes. J Wildl Manage 71:541–554
Fryxell JM, Lundberg P (1997) Individual behaviour and community dynamics. Chapman and Hall, New York
Fryxell JM, Wilmshurst JF, Sinclair ARE (2004) Predictive models of movement by Serengeti grazers. Ecology 85:2429–2435
Garner DL, Porter WF (1980) Movements and seasonal home ranges of bull moose in a pioneering Adirondack population. Alces 26:80–85
Geist V (1982) Adaptive behavioural strategies. In: Thomas JW, Toweill DE (eds) Elk of North America: ecology and management. Stackpole Books, Harrisburg, pp 219–278
Gelfan AN, Pomeroy JW, Kuchment LS (2004) Modeling forest cover influences on snow accumulation, sublimation, and melt. J Hydrom 5:785–803
Hebblewhite M, Merrill EH (2007) Multiscale wolf predation risk for elk: Does migration reduce risk? Oecologia. doi:10.1007/s00442-007-0661-y
Hebblewhite M, Merrill EH, McDonald T (2005) Spatial decomposition of predation risk components using resource selection functions. Oikos 111:101–111
Hosmer DW, Lemeshow S (2000) Applied logistic regression analysis, 2nd edn. Wiley, New York
Huggard DJ (1993) Prey selectivity of wolves in Banff National Park. I. Prey species. Can J Zool 71:130–139
Irwin LL, Peek JM (1983) Elk habitat use relative to forest succession in Idaho. J Wildl Manage 47:664–672
Jenkins DA, Schaefer JA, Rosatte R, Bellhouse T, Hamr J, Mallory FF (2007) Winter resource selection of reintroduced elk and sympatric white-tailed deer at multiple spatial scales. J Mammal 88:614–624
Johnson BK, Kern JW, Wisdom MJ, Findholt SL, Kie JG (2000) Resource selection and spatial separation of mule deer and elk during spring. J Wildl Manage 64:685–697
Johnson CJ, Parker KL, Heard DC, Gillingham MP (2002) Movement parameters of ungulates and scale-specific responses to the environment. J Anim Ecol 71:225–235
Johnson DH (1980) The comparison of usage and availability measurements for evaluating resource preference. Ecology 61:65–71
Jost MA, Hamr J, Filion I, Mallory FF (1999) Forage selection by elk in habitats common to the French River—Burwash region of Ontario. Can J Zool 77:1429–1438
Julander O, Jeffrey DE (1968) Deer, elk, and cattle range relations on summer range in Utah. Trans North Am Wildl Nat Resour Conf 29:404–414
Kearney SR, Gilbert FF (1976) Habitat use by white-tailed deer and moose on sympatric range. J Wildl Manage 40:645–657
Keating KA, Cherry S (2004) Use and interpretation of logistic regression in habitat-selection studies. J Wildl Manage 68:774–789
Kie JG (1999) Optimal foraging and risk of predation: effects on behaviour and social structures in ungulates. J Mammal 80:1114–1129
Kristan WB, Boarman WI (2003) Spatial pattern of risk of common raven predation on desert tortoises. Ecology 84:2432–2443
Kunkel KE, Pletscher DH (2000) Habitat factors affecting vulnerability of moose to predation by wolves in southeastern British Columbia. Can J Zool 78:150–157
Kunkel KE, Pletscher DH (2001) Winter hunting patterns of wolves in and near Glacier National Park, Montana. J Wildl Manage 65:520–530
Lima SL, Zollner PA (1996) Towards a behavioral ecology of ecological landscapes. Trends Ecol Evol 11:131–135
Lima SL, Dill LM (1990) Behavioral decisions made under the risk of predation: a review and prospectus. Can J Zool 68:619–640
Ludewig HA, Bowyer RT (1985) Overlap in winter diets of sympatric moose and white-tailed deer in Maine. J Mammal 66:390–392
MacKenzie DI (2005) What are the issues with presence-absence data for wildlife managers? J Wildl Manage 69:849–860
Manly BFJ, McDonald LL, Thomas DL, McDonald TL, Erickson WP (2002) Resource selection by animals: statistical design and analysis for field studies, 2nd edn. Kluwer, Dordrecht
Mao JS, Boyce MS, Smith DW, Singer FJ, Vales DJ, Vore JM, Merrill EH (2005) Habitat selection by elk before and after wolf reintroduction in Yellowstone National Park. J Wildl Manage 69:1691–1707
Matthysen E (2005) Density-dependent dispersal in birds and mammals. Ecography 28:403–416
Mazerolle MJ (2004) Mouvements et reproduction des amphibians en tourbières perturbées. PhD thesis, Université Laval, Laval
McKenney DW, Rempel RS, Venier LA, Wang Y, Bisset AR (1998) Development and application of a spatially explicit moose population model. Can J Zool 76:1922–1931
McLoughlin PD, Walton LR, Cluff HD, Paquet PC, Ramsay MA (2004) Hierarchical habitat selection by tundra wolves. J Mammal 85:576–580
McLoughlin PD, Dunford JS, Boutin S (2005) Relating predation mortality to broad-scale habitat selection. J Anim Ecol 74:701–707
McLoughlin PD, Boyce MS, Coulson T, Clutton-Brock T (2006) Lifetime reproductive success and density-dependent, multi-variable resource selection. Proc R Soc B 273:1449–1454
McRoberts RE, Mech LD, Peterson RO (1995) The cumulative effect of consecutive winter’s snow depth on moose and deer populations: a defense. J Anim Ecol 64:131–135
Mech LD (1970) The wolf: the ecology and behaviour of an endangered species. Natural History Press, Garden City
Mech LD (1977) Wolf-pack buffer zones as prey reservoirs. Science 198:320–321
Mech LD, Boitani L (2003) Wolves: Behavior, ecology and conservation. University of Chicago Press, Chicago
Mech LD, McRoberts RE, Peterson RO, Page RE (1987) Relationship of deer and moose populations to previous winters’ snow. J Anim Ecol 56:615–627
Messier F (1991) The significance of limiting and regulating factors on the demography of moose and white tailed deer. J Anim Ecol 60:377–393
Messier F (1995) Is there evidence for a cumulative effect of snow on moose and deer populations. J Anim Ecol 64:136–140
Morrison SF, Forbes GJ, Young SJ, Lusk S (2003) Within yard habitat use by white-tailed deer at varying winter severity. For Ecol Manage 172:173–182
Musiani M, Okarma H, Jedrzejewski W (1998) Speed and actual distance traveled by radiocollared wolves in Bialowieza Primeval Forest (Poland). Acta Theriol 43:409–416
Nelson ME, Mech LD (1986) Relationship between snow depth and gray wolf predation on white-tailed deer. J Wildl Manage 50:471–474
Nelson ME, Mech LD (1991) Wolf predation risk associated with white-tailed deer movements. Can J Zool 69:2696–2699
Orians GH, Wittenberger JF (1991) Spatial and temporal scales in habitat selection. Am Nat 137:S29–S49
Ontario Ministry of Natural Resources (OMNR) (2005) Backgrounder on wolf conservation in Ontario. OMNR. ON
Potvin F, Jolicoeur H, Huot J (1988) Wolf diet and prey selectivity during two periods for deer in Quebec: decline versus expansion. Can J Zool 66:1274–1279
Renecker LA, Schwartz CC (1998) Food habits and feeding behaviour. In: Franzmann AW, Schwartz CC (eds) Ecology and management of the North American moose. Smithsonian Institution Press, Washington, DC, pp 403–439
Rettie WJ, Messier F (2000) Hierarchical habitat selection by woodland caribou: its relationship to limiting factors. Ecography 23:466–478
Ricketts TH, Dinerstein E, Olson DM, Loucks CJ, Eichbaum W, DellaSala D, Kavanagh K, Hedao P, Hurley PT, Carney KM et al (1999) Terrestrial ecoregions of North America: a conservation assessment. Island Press, Washington, DC
Rosatte R, Hamr J, Ranta B, Young J, Cool N (2007) Elk restoration in Ontario, Canada: infectious disease management strategy 1998–2001. Ann NY Acad Sci 969:358–363
Rosenzweig ML (1989) Habitat selection, community organization, and small mammal studies. In: Morris DW, Abramsky Z, Fox BJ, Willig MR (eds) Patterns in the structures of mammalian communities. Texas Tech University Press, Lubbock, pp 5–21
Schmitz OJ, Beckerman AP, O’Brien KM (1997) Behaviorally mediated trophic cascades: effects of predation risk on food web interactions. Ecology 78:1388–1399
Telfer ES (1970) Winter habitat selection by moose and white-tailed deer. J Wildl Manage 34:553–559
Telfer ES (1978) Cervid distribution, browse and snow cover in Alberta. J Wildl Manage 42:352–361
Telfer ES, Kelsall JP (1984) Adaptations of some large North American mammals for survival in snow. Ecology 65:1828–1834
Tierson WC, Mattfeld GF, Sage RW, Behrend DF (1985) Seasonal movements and home ranges of white-tailed deer in the Adirondacks. J Wildl Manage 49:760–769
Ungulate Winter Range Technical Advisory Team (UWRTAT) (2005) Desired conditions for mule deer, elk and moose winter range in the southern interior of British Columbia. BC Ministry of Water, Land and Air protection, Biodiversity branch, Victoria BC, Wildlife Bulletin B-120
Van Ballenberghe V, Ballard WB (1998) Population dynamics. In: Franzmann AW, Schwartz CC (eds) Ecology and management of the North American moose. Smithsonian Institution Press, Washington, DC, pp 223–246
Vankat JL (1979) The natural vegetation of North America: an introduction. Wiley, Toronto
Weaver JL (1984) Ecology of wolf predation amidst high ungulate diversity in Jasper National Park, Alberta. PhD thesis, University of Montana
Whittington J, St Clair CC, Mercer G (2005) Spatial responses of wolves to roads and trails in mountain valleys. Ecol Appl 15:543–553
Acknowledgements
Funding for the project was provided by Natural Sciences and Engineering Research Council of Canada Collaborative Research Opportunities grant, Discovery grant and PGS–Master’s scholarship (A. M. K.), as well as by the National Science Foundation (IRCEB grant 0078130). Crucial field assistance was provided by M. Hartmann, B. Cox, A. Watson, S. Wyshinski and P. Kirzan. We thank Bighorn Helicopters for animal capture services and the pilots and staff of Central North Airways for fixed wing telemetry flights. Finally, we thank L. Borger, B. Dalziel, M. Drever, C. Johnson, K. McCann, B. Patterson, J. Shuter and two anonymous reviewers for helpful discussion and reviews of past manuscript drafts. All research was conducted with authorization from, and in accordance with, appropriate University and Provincial legislation and policies.
Author information
Authors and Affiliations
Corresponding author
Additional information
Communicated by Steven Kohler.
Electronic supplementary material
Below is the link to the electronic supplementary material.
Rights and permissions
About this article
Cite this article
Kittle, A.M., Fryxell, J.M., Desy, G.E. et al. The scale-dependent impact of wolf predation risk on resource selection by three sympatric ungulates. Oecologia 157, 163–175 (2008). https://doi.org/10.1007/s00442-008-1051-9
Received:
Accepted:
Published:
Issue Date:
DOI: https://doi.org/10.1007/s00442-008-1051-9