Abstract
Epigenetic modifications are considered to have an important role in evolution. DNA methylation is one of the best studied epigenetic mechanisms and methylation variability is crucial for promoting phenotypic diversification of organisms in response to environmental variation. A critical first step in the assessment of the potential role of epigenetic variation in evolution is the identification of DNA methylation polymorphisms and their relationship with genetic variations in natural populations. However, empirical data is scant in animals, and particularly so in wild mammals. Bats are considered as bioindicators because of their sensitivity to environmental perturbations and they may present an opportunity to explore epigenetic variance in wild mammalian populations. Our study is the first to explore these questions in the female great roundleaf bat (Hipposideros armiger) populations using the methylation-sensitive amplified polymorphism (MSAP) technique. We obtained 868 MSAP sites using 18 primer combinations and found (1) a low genomic methylation level (21.3 % on average), but extensive DNA methylation polymorphism (90.2 %) at 5′-CCGG-3′ sites; (2) epigenetic variation that is structured into distinct between- (29.8 %) and within- (71.2 %) population components, as does genetic variation; and (3) a significant correlation between epigenetic and genetic variations (P < 0.05). These results may also apply to other wild mammalian populations. The possible causes for the correlation between epigenetic and genetic variations are discussed.
Similar content being viewed by others
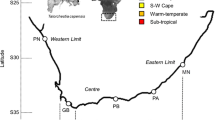
Avoid common mistakes on your manuscript.
Introduction
Biodiversity faces challenges from habitat destruction, fragmentation, over exploitation, and global climate change (Jablonski 2004). Organisms have to modify their phenotype in response to such abiotic stresses (Agrawal 2001). Studies conducted in animals (Dolinoy et al. 2007; Kucharski et al. 2008) and plants (Bossdorf et al. 2010; Lira-Medeiros et al. 2010) have suggested that epigenetic processes play a crucial role in mediating environmentally induced phenotypic variations (Vogt et al. 2008; Angers et al. 2010; Gao et al. 2010). Those epigenetic patterns may be stably inherited by future generations (Richards 2006; Jablonka and Raz 2009; Skinner and Guerrero-Bosagna 2009), implying that epigenetic processes have potential evolutionary effects (Jablonka et al. 2005; Bossdorf et al. 2008; Richards 2008).
Epigenetics is the study of heritable changes in gene expression that result from covalent modifications of DNA and/or histone proteins, which do not entail a change in the DNA sequence per se (Richards 2006; Bird 2007). The epigenetic information is encrypted in genetic sequences (Bonasio et al. 2010; Migicovsky and Kovalchuk 2011). Before assessing their potential role in evolution, investigating epigenetic variations and their relationship with genetic variations that are observed in a real-world context are the important steps (Richards 2006; Bossdorf et al. 2008). Significant correlation suggests that epigenetic differentiation may be interpreted by genetic variation (Li et al. 2008; Ochogavía et al. 2009) or a downstream consequency of DNA sequence variation (Herrera and Bazaga 2010). In contrast, the lack of correlation between the epigenetic and genetic variances would suggest that natural variation in epigenetic modifications may be autonomous from the strict control of genetic variance (Richards 2006), as an additional system in evolution (Cervera et al. 2002; Vaughn et al. 2007).
DNA methylation is one of the best studied epigenetic mechanisms and methylation variability is crucial for promoting phenotypic variance of organisms under different environmental conditions (Gao et al. 2010; Massicotte et al. 2011). Natural genomic methylation variations have been investigated in plant populations (Li et al. 2008; Gao et al. 2010; Herrera and Bazaga 2010, 2011; Lira-Medeiros et al. 2010); however, studies on the origin, stability, variation, and inheritance of DNA methylation polymorphisms of wild animal populations in the evolution and ecological adaptation are scarce. There have only been three studies on fish (Blouin et al. 2010; Massicotte et al. 2011; Morán and Pérez-Figueroa 2011) and one on birds (Schrey et al. 2012). Wild animals constantly experience environmental variations; therefore, it is necessary to expand our understanding of their genomic DNA methylation patterns.
Bats are considered as bioindicators because they can reflect wider-scale impacts on the biota of interest because of their sensitivity to environmental variation, e.g., global climate change or habitat deterioration (Jones et al. 2009). Varied environmental conditions could induce epigenetic variance (Gao et al. 2010; Lira-Medeiros et al. 2010), so bats may present an opportunity to investigate epigenetic variation in wild mammalian populations. We selected female great roundleaf bat (Hipposideros armiger, Hodgson, 1835) populations, because this species is widely distributed in South China and has the wing morphology of short-distance migrants (Norberg and Rayner 1987), implying a low level of population mixing. Using the methylation-sensitive amplified polymorphism (MSAP) technique and five female populations, we explored: (1) the extent and pattern of genome-wide DNA methylation of the female H. armiger populations. In mammals, about 70 % of all CpG sites in somatic tissues are methylated (Ehrlich et al. 1982; Allis et al. 2007) and we should find high level of methylation in bats; (2) whether the DNA methylation variation varied within- and between-populations, as does genetic variation; and (3) the correlation between epigenetic and genetic variances in bats; a key aspect that has not been previously explored for any wild animal.
Materials and methods
Samples and DNA extraction
All female H. armiger samples were from the deposited specimens in the Museum of Natural History of Northeast Normal University, Jilin Province, China. Five populations comprising 25 females in total were chosen, including 6 from Sichuan Province (SCWY), 6 from Jiangxi Province (JXJGS), 5 from Guizhou Province (GZAL), 4 from Guangdong Province (GDSG), and 4 from Yunnan Province (YNYJ). According to Zhang et al. (1997), these populations belong to four zoological regions in China: JXJGS and GDSG belong to the East region, GZAL and SCWY belong to the West region, and YNYJ is from the edge of the Southwest and South Yunnan regions. Information on their caves of origin, including latitude, longitude, elevation, and climate data (mean annual temperature, MAT; mean annual rainfall, MAR; mean annual atmospheric pressure, MAAP; and mean annual wind velocity, MAWV; data from China Meteorological Data Sharing Service System, http://cdc.cma.gov.cn/shuju) are shown in Table 1. Genomic DNA was extracted from muscle tissues using a UNIQ-10 Column Animal Genome DNA Extraction Kit (SK1205, Shanghai Sangon Biological Engineering Technology & Services Co., Ltd.).
MSAP technique
The MSAP method, based on the amplified fragment length polymorphism (AFLP) (Vos et al. 1995) technique, is well suited for non-model species with little genomic information and provides rapid epigenetic fingerprints for a large number of samples (Bossdorf et al. 2008). This technique uses the enzyme EcoRI (rare cutter) with either one of two methylation-sensitive isoschizomer restriction enzymes, HpaII and MspI (frequent cutters). The isoschizomer enzymes recognize the same restriction site (5′-CCGG-3′), but have different cytosine (Cs) methylation sensitivities. HpaII is sensitive to full methylation (both strands methylated) of either Cs, but cleaves the hemimethylated external Cs (Mann and Smith 1977), whereas MspI cleaves sites with fully methylated internal Cs (Waalwijk and Flavell 1978; Ehrlich and Wang 1981) (the restriction enzyme database: http://rebase.neb.com/rebase/rebase.html). Both enzymes cleave fully non-methylated 5′-CCGG-3′ (Ehrlich and Wang 1981; McClelland et al. 1994).
The adapters and primers for EcoRI and HpaII–MspI digest fragments were designed according to Vos et al. (1995) and Xu et al. (2000), respectively. Two copies of each individual genome were digested by EcoRI/HpaII and EcoRI/MspI, respectively. Eighteen selective amplification primer pairs were chosen for PCR (Eco + AGC − H/M + TAC/TAG/TCG/TTA/TGA/TGT, Eco + ACT − H/M + TAC/TCT/TGA/TGC, Eco + ACA − H/M + TAC/TAG/TCT/TGC, Eco + ACC − H/M +TCT/TGA/TGT, Eco + AAC − H/M + TTC). The PCR protocols were according to Vos et al. (1995). The products were electrophoresed for 3 h on 6 % denaturing polyacrylamide gels and visualized by silver staining (Bassam et al. 1991). To reduce the potential impact of size homoplasy, only unambiguous and intense bands, ranging in size from 150 to 500 bp, were scored (Caballero et al. 2008).
Statistical analysis
Methylation-sensitive amplified polymorphism bands were scored as present “+” and absent “−”, hence there were three patterns for comparing amplification products from EcoRI/HpaII and EcoRI/MspI (Table 2): (a) present in both (+/+), non-methylated; (b) present in only EcoRI/HpaII (+/−, CHG methylation) or EcoRI/MspI (−/+, CG methylation), methylated; (c) absent in both (−/−), uninformative because of the absence of a fragment or hypermethylation. MSAP fragments that differ in their presence/absence in the EcoRI/HpaII and EcoRI/MspI patterns in at least one individual were considered as methylated bands; a site was considered as “methylation-sensitive polymorphism” (MSP) band if there was at least one individual in which the site was methylated and at least one individual for which the site was non-methylated or missing. The MSP binary matrix was scored as 1 (+/− or −/+), 0 (+/+), and missing (−/−). The other non-methylated loci, which were identical in their presence/absence in the EcoRI/HpaII and EcoRI/MspI patterns, were considered as CCGG-genetic markers; a site was considered as “methylation-insensitive polymorphism” (MIP) band if the fragments were absent in both enzymes in at least one individual. The MIP binary matrix was scored as 1 (+/+) and 0 (−/−).
We calculated the gene flow among populations based on the MIPs matrix by the PopGen program (version 1.31) and used the Kruskal–Wallis H test to estimate the significance of the difference in the CG and CHG methylation patterns among populations. We calculated the Shannon diversity index based on the frequency of each band among the 25 individuals to estimate the within-population epigenetic diversity (H pop). Significant differences in the Shannon index among populations were assessed by the Kruskal–Wallis H test, and the significance of the test was adjusted by the sequential Bonferroni correction (Rice 1989). The coefficient of epigenetic differentiation was computed as G ST = (H sp − H pop)/H sp (Bussell 1999), where H sp is the within-species epigenetic diversity.
We used multivariate analyses to explore between-population epigenetic structure. Principal component analysis (PCA) on inter-profile covariance matrix based on MSP and MIP binary profiles were performed to provide a genome-wide variability point of view, summarized in a few synthetic variables. The between-group Eigen analysis [BPCA–PCA among groups based on PCA among individuals, (Parisod and Christin 2008)] was processed to group individual PCA profiles into populations maximizing the between-group variance. Statistical significance was assessed by the Romesburg randomization test (104 permutations). Multivariate analyses were performed by ADE-4 software (Thioulouse et al. 1997).
Between-group Eigen analysis divides the variance into within- and between-population components and it is based on Euclidean distances; therefore, it can be considered as analogous to F statistics (called β ST) (Parisod et al. 2005; Lira-Medeiros et al. 2010). The β ST value equals the ratio of the inertia of the between PCA to the total inertia. However, β ST are not equivalent to F statistics and β ST values may be overestimated because BPCA maximizes the between-group variance (Parisod and Christin 2008).
To further explore the contribution of both epigenetic and genetic profiles to the female H. armiger population structure, we used the symmetrical co-inertia analysis, which maximizes shared structures among multiple data sets drawn from the same samples (Lira-Medeiros et al. 2010). This analysis can be safely used for multivariables that are related because it does not rely on linear regressions (Dolédec and Chessel 1994). Statistical significance was assessed by 104 Monte Carlo permutations in the ADE-4 software (Thioulouse et al. 1997).
We used Mantel’s tests (Mantel 1967) to assess relationship of epigenetic (MSP) and genetic (MIP) profiles by two main indexes (104 permutations). First, according to Cervera et al. (2002), we compared Dice coefficients (Sneath and Sokal 1973), which were calculated independently from both MSP and MIP profiles in NTSYS 2.0 Software. Second, we compared pairwise β ST (BPCA) values of both profiles (Parisod et al. 2005; Parisod and Christin 2008; Lira-Medeiros et al. 2010), which were calculated by the ADE-4 software (Thioulouse et al. 1997).
To exclude the effects of confounding environmental factors, we performed partial Mantel’s tests while controlling environmental variables (geographical distance, climate variables) by PASSaGE v.2 (Rosenberg and Anderson 2011). This information was used to determine whether the residual variability in the epigenetic and genetic profiles was still significantly correlated after removing the effects of those environmental variables. The distance matrices of those environmental variables were calculated by a Euclidean method. The geographical distance was calculated from the latitude and longitude of each population, and was then logarithmically transformed (by loge). For climate variables, we implemented a PCA on a correlation matrix of the four climate variables (MAT, MAR, MAAP, and MAWV) and used the first two PC factor scores to obtain a matrix of Euclidean distances. All the Mantel’s tests were randomly permuted 104 times.
Results
Variation in methylation profiles
To characterize variation in methylation profiles, we analyzed genomic DNA methylation patterns of 25 female H. armiger from 5 populations. We scored 868 MSAP sites using 18 primer combinations, with an average of 48.2 bands per primer pair. By comparing the presence/absence of restricted fragments in HpaII and MspI assays for each individual, 499 loci showed similar digestibility, but varied among populations, suggesting a non-methylated 5′-CCGG-3′ restriction site, i.e., 5′-CCGG-genetic variation, and showed low gene flow among those populations (Nm ranged from 1.03 to 2.23). The other 369 loci showed differential digestibility suggesting MSPs. Thirty-six loci were methylated in all 25 animals and 333 loci (90.2 %) were polymorphic among those individuals. Of these 333 loci, 46 polymorphic sites were found with both isoschizomers, while 287 were detected with only one of the isoschizomers, including 104 CHG methylated and 183 CG methylated sites.
The numbers of various fragments attributed to non-methylated (+/+), CHG methylated (+/−), CG methylated (−/+), and uninformative (−/−), respectively, were calculated for each population based on MSAP profiles. The total 5′-CCGG-methylation level ranged from 19.6 % in YNYJ to 23.5 % in GZAL, with a mean of 21.3 % at the species level (Fig. 1). The difference in the genome-wide methylation level (CG and CHG methylation) among all populations was significant (Kruskal–Wallis χ2 = 13.33, df = 4, P = 0.010); however, a non-significant difference was found in the level of CG (Kruskal–Wallis χ2 = 0.566, df = 4, P = 0.967) and CHG methylation patterns (Kruskal–Wallis χ2 = 8.826, df = 4, P = 0.066). Populations showed more difference in CHG than in CG methylation patterns, because isoschizomer MspI is insensitive to methylation and shows similar CG pattern among populations (Blouin et al. 2010).
MSAP-based cytosine methylation levels of two major types (CG and CHG) of the randomly sampled 5′-CCGG sites in five female H. armiger populations. Methylation level (%) was calculated by dividing MSAP bands representing methylated 5′-CCGG sites (differential presence/absence of restricted fragments in HpaII and MspI assays) against the total number of scored bands
Epigenetic structure
We used frequency based and multivariate methods to assess the epigenetic structure of female H. armiger populations. The within-population epigenetic Shannon indices calculated for the five populations were 0.078 (SCWY), 0.071 (JXJGS), 0.084 (GZAL), 0.074 (GDSG), and 0.114 (YNYJ), respectively, and were significantly different (Kruskal–Wallis χ2 = 50.982, df = 4, P < 0.001). The YNYJ population showed a higher Shannon index than the others, implying higher variation. Based on the partition of Shannon diversity within and among populations, we calculated a G ST of 0.327.
For the between-population analysis, the first axes of the PCA based on covariance matrix (MSP) summarized only 12.2 % of total inertia, and the first 19 PCA axes, which together represented 91.0 % of the information contained in the epigenetic data set, would be used for further analyses. The multivariate BPCA based on the above PCA scores resulted in a significant β ST of 0.298 (P < 0.001), showing that epigenetic variance could be divided into distinct between- (29.8 %) and within- (71.2 %) population components. β ST are considered as analogous, but not equivalent, to F statistics, and were used to represent the epigenetic structure (Parisod et al. 2005; Parisod and Christin 2008). In the new subspace (Fig. 2), the five populations showed obvious clustering in four quadrants by the first two axes (summarizing 62.2 % of the total inertia), while GZAL and GDSG populations (represented by 3 and 4, respectively) were intertwined, and YNYJ (represented by 5) showed more variation than the other populations.
Between-group Eigen analysis of the five female H. armiger populations using PCA values based on the epigenetic covariance matrix (MSP). F1 and F2 values show the contribution of the two principal components summarizing the total variance of each data set. The first two axes summarize 62.2 % of the total variance. The numbers from 1 to 5 represent the five populations: SCWY, JXJGS, GZAL, GDSG, and YNYJ. β ST were calculated by BPCA for epigenetic profiles and tested with 104 Romesburg randomization permutations
Correlation between epigenetic and genetic profiles
Co-inertia analysis was used to evaluate the contribution of both genetic and epigenetic profiles to the H. armiger population structure. The first two axes explained 41.6 % of the total co-variation between the epigenetic and genetic profiles, and this association was significantly different from the value expected for random association (P < 0.001). Epigenetic and genetic profiles displayed similar distributions (Fig. 3). Epigenetic and genetic profiles were significantly correlated based on Dice coefficients (r = 0.913, P < 0.001) and pairwise β ST (r = 0.882, P = 0.009). To remove the effects of environmental variables, we performed partial Mantel’s tests while controlling geographic distance and climate variables, and the results still showed significant correlation (Dice coefficients: r = 0.967, P = 0.034; β ST: r = 0.943, P = 0.027). These results suggested a significant correlation between epigenetic variance and nucleotide sequence variation.
Co-inertia analysis of female H. armiger populations using PCA scores based on genetic (MIP) and epigenetic (MSP) covariance matrices. The co-inertia analysis maximized the covariance of PCAs. F1 and F2 values show the contribution of the two principal components summarizing the total variance of each data set. Circles correspond to the projection of genetic profiles (MIP) and arrowheads correspond to the projection of epigenetic profiles (MSP). The numbers from 1 to 5 represent the five populations: SCWY, JXJGS, GZAL, GDSG, and YNYJ. The significance test of this association was done with 104 permutations (Monte Carlo test)
Discussion
The MSAP technique only detects methylation at 5′-CCGG-3′ sites and cannot discriminate between methylation and fragment absence when both cytosines are hypermethylated; therefore, the level of genomic DNA methylation may be underestimated. Bearing in mind this inherent limitation of the technique, our study has explored the level and pattern of genome-wide 5′-CCGG-methylation in five female H. armiger populations. From our results, those populations have a low genomic methylation level (21.3 % on average), but extensive DNA methylation polymorphism (90.2 %) at 5′-CCGG-3′ sites. The difference in the genome-wide methylation level among populations was significant. Significant relative β ST values suggested that epigenetic variation was structured into distinct between- and within-population components in the female H. armiger populations, similar to plants (Keyte et al. 2006; Li et al. 2008), in a manner that could be considered analogous to genetic structure (Herrera and Bazaga 2010).
The BPCA plot based on methylation profile also showed that female H. armiger populations were obviously separated to four major groups, as if the environmental factors covarying across geographical locations exert a relatively greater impact on DNA methylation (Fig. 2). However, GZAL and GDSG populations, which came from different zoological regions, were intertwined. Additionally, individuals in the YNYJ population showed more variation than the other populations. YNYJ is located at the edge of the Southwest and South Yunnan regions, implying migration in this population. Moreover, epigenetic and genetic profiles displayed similar distributions in the co-inertia subspace (Fig. 3) and had a significant association (P < 0.001). The above findings raise an interesting question: is the differentiation of genome-wide DNA methylation among populations dependent on nucleotide sequence variation in bats?
To address this question, we estimated the correlation between epigenetic and genetic profiles. It is a matter of debate amongst ecologists as to whether epigenetic variance is independent (Cervera et al. 2002; Vaughn et al. 2007) and/or dependent from genetic variation (Li et al. 2008; Ochogavía et al. 2009; Herrera and Bazaga 2010) in natural populations. In our study, Dice coefficients and/or pairwise β ST (based on inter-individual distance matrices of MSP and MIP with control of environmental variables) were used to address this question by partial Mantel’s tests, respectively. Although we used genetic characterization of the populations by multilocus approaches based on the whole set of non-methylated MSAP fragments (Ashikawa 2001; Cervera et al. 2002), we found a clear and significant correlation between epigenetic and genetic variations in the female H. armiger populations. Similar result has been reported in humans (Zhang et al. 2010).
The significant correlation suggested that methylation-based epigenetic variance might be associated with the control of genetic instability. Bjornsson et al. (2008) found that DNA methylation varies over time with familial clustering in humans, which implies that epigenetic variance may be under genetic control. In addition, genetic variation in the form of a transposon, e.g., absence, would directly affect the epigenetic state of the retroelement, which could induce varied phenotype in the genetically identical offsprings (Morgan et al. 1999; Michaud et al. 1994). Those researches in mammals have found that the influence of some epigenetic effectors is sequence dependent or allele specific (Meaney and Ferguson-Smith 2010). Therefore, the significant correlation between epigenetic and genetic variances in the female H. armiger populations in our study is probably caused by methylation variation that depends on DNA sequence difference through interactions involving multiple genes (Liu et al. 2010).
However, neutral drift could also account for the significant correlation between epigenetic and genetic variations. If epigenetic differences are determined entirely by genetic control, strong divergent selection would be required to cause the epigenetic variations in a situation of high gene flow between populations. That means high gene flow might minimize the population differences caused by the drift. But in this study, we detected limited gene flow among female H. armiger populations studied (Nm ranged from 1.03 to 2.23), which implies that stochastic processes of drift might result in epigenetic population patterns that develop in parallel to genetic differences, causing a correlation without a functional link between the two (Richards et al. 2010).
Investigating DNA methylation polymorphisms in natural populations and evaluating the relevance of their epigenetic and genetic profiles are critical steps for assessing the potential role of epigenetic variation in wild mammalian microevolution. Using MSAP, we found extensive epigenetic polymorphism, distinct epigenetic structures, and a significant correlation between epigenetic and genetic variations in the female great roundleaf bat (H. armiger) populations, which may also apply to other wild mammalian populations. The latter observation suggests that the epigenetic variation is under genetic control and/or the correlation was caused by neutral drift.
References
Agrawal AA (2001) Phenotypic plasticity in the interactions and evolution of species. Science 294:321–326. doi:10.1126/science.1060701
Allis CD, Jenuwein T, Reinberg D, Caparros ML (2007) Epigenetics. CSHL Press, New York
Angers B, Castonguay E, Massicotte R (2010) Environmentally induced phenotypes and DNA methylation: how to deal with unpredictable conditions until the next generation and after. Mol Ecol 19:1283–1295. doi:10.1111/j.1365-294X.2010.04580.x
Ashikawa I (2001) Surveying CpG methylation at 5′-CCGG in the genomes of rice cultivars. Plant Mol Biol 45:31–39. doi:10.1023/A:1006457321781
Bassam BJ, Caetano-Anollés G, Gresshoff P (1991) Fast and sensitive silver staining of DNA in polyacrylamide gels. Anal Biochem 196:80–83. doi:10.1016/0003-2697(91)90120-I
Bird A (2007) Perceptions of epigenetics. Nature 447:396–398. doi:10.1038/nature05913
Bjornsson HT, Sigurdsson MI, Fallin MD, Irizarry RA, Aspelund T, Cui H, Yu W, Rongione MA, Ekström TJ, Harris TB (2008) Intra-individual change in DNA methylation over time with familial clustering. J Am Med Assoc 299:2877–2883. doi:10.1001/jama.299.24.2877
Blouin MS, Thuillier V, Cooper B, Amarasinghe V, Cluzel L, Araki H, Grunau C (2010) No evidence for large differences in genomic methylation between wild and hatchery steelhead (Oncorhynchus mykiss). Can J Fish Aquat Sci 67:217–224. doi:10.1139/F09-174
Bonasio R, Tu S, Reinberg D (2010) Molecular signals of epigenetic states. Science 330:612–616. doi:10.1126/science.1191078
Bossdorf O, Richards CL, Pigliucci M (2008) Epigenetics for ecologists. Ecol Lett 11:106–115. doi:10.1111/j.1461-0248.2007.01130.x
Bossdorf O, Arcuri D, Richards CL, Pigliucci M (2010) Experimental alteration of DNA methylation affects the phenotypic plasticity of ecologically relevant traits in Arabidopsis thaliana. Evol Ecol 24:541–553. doi:10.1007/s10682-010-9372-7
Bussell JD (1999) The distribution of random amplified polymorphic DNA (RAPD) diversity amongst populations of Isotoma petraea (Lobeliaceae). Mol Ecol 8:775–789. doi:10.1046/j.1365-294X.1999.00627.x
Caballero A, Quesada H, Rolan-Alvarez E (2008) Impact of amplified fragment length polymorphism size homoplasy on the estimation of population genetic diversity and the detection of selective loci. Genetics 179:539–554. doi:10.1534/genetics.107.083246
Cervera MT, Ruiz-García L, Martínez-Zapater J (2002) Analysis of DNA methylation in Arabidopsis thaliana based on methylation-sensitive AFLP markers. Mol Genet Genomics 268:543–552. doi:10.1007/s00438-002-0772-4
Dolédec S, Chessel D (1994) Co-inertia analysis: an alternative method for studying species–environment relationships. Freshw Biol 31:277–294. doi:10.1111/j.1365-2427.1994.tb01741.x
Dolinoy DC, Huang D, Jirtle RL (2007) Maternal nutrient supplementation counteracts bisphenol A-induced DNA hypomethylation in early development. Proc Natl Acad Sci USA 104:13056–13061. doi:10.1073/pnas.0703739104
Ehrlich M, Wang RY (1981) 5-Methylcytosine in eukaryotic DNA. Science 212:1350–1357. doi:10.1126/science.6262918
Ehrlich M, Gama-Sosa MA, Huang LH, Midgett RM, Kuo KC, McCune RA, Gehrke C (1982) Amount and distribution of 5-methylcytosine in human DNA from different types of tissues or cells. Nucleic Acids Res 10:2709–2721. doi:10.1093/nar/10.8.2709
Gao L, Geng Y, Li BO, Chen J, Yang JI (2010) Genome-wide DNA methylation alterations of Alternanthera philoxeroides in natural and manipulated habitats: implications for epigenetic regulation of rapid responses to environmental fluctuation and phenotypic variation. Plant Cell Environ 33:1820–1827. doi:10.1111/j.1365-3040.2010.02186.x
Herrera CM, Bazaga P (2010) Epigenetic differentiation and relationship to adaptive genetic divergence in discrete populations of the violet Viola cazorlensis. New Phytol 187:867–876. doi:10.1111/j.1469-8137.2010.03298.x
Herrera CM, Bazaga P (2011) Untangling individual variation in natural populations: ecological, genetic and epigenetic correlates of long term inequality in herbivory. Mol Ecol 20:1675–1688. doi:10.1111/j.1365-294X.2011.05026.x
Jablonka E, Raz G (2009) Transgenerational epigenetic inheritance: prevalence, mechanisms, and implications for the study of heredity and evolution. Q Rev Biol 84:131–176. doi:10.1086/598822
Jablonka E, Lamb MJ, Zeligowski A (2005) Evolution in four dimensions. The MIT Press, London
Jablonski D (2004) Extinction: past and present. Nature 427:589. doi:10.1038/427589a
Jones G, Jacobs DS, Kunz TH, Willig MR, Racey PA (2009) Carpe noctem: the importance of bats as bioindicators. Endanger Species Res 8:93–115. doi:10.3354/esr00182
Keyte AL, Percifield R, Liu B, Wendel JF (2006) Infraspecific DNA methylation polymorphism in cotton (Gossypium hirsutum L.). J Hered 97:444–450. doi:10.1093/jhered/esl023
Kucharski R, Maleszka J, Foret S, Maleszka R (2008) Nutritional control of reproductive status in honeybees via DNA methylation. Science 319:1827–1830. doi:10.1126/science.1153069
Li Y, Shan X, Liu X, Hu L, Guo W, Liu B (2008) Utility of the methylation-sensitive amplified polymorphism (MSAP) marker for detection of DNA methylation polymorphism and epigenetic population structure in a wild barley species (Hordeum brevisubulatum). Ecol Res 23:927–930. doi:10.1007/s11284-007-0459-8
Lira-Medeiros CF, Parisod C, Fernandes RA, Mata CS, Cardoso MA, Meyer P (2010) Epigenetic variation in mangrove plants occurring in contrasting natural environment. PLoS One 5:e10326. doi:10.1371/journal.pone.0010326
Liu J, Hutchison K, Perrone-Bizzozero N, Morgan M, Sui J, Calhoun V (2010) Identification of genetic and epigenetic marks involved in population structure. PLoS One 5:e13209. doi:10.1371/journal.pone.0013209
Mann MB, Smith HO (1977) Specificity of Hpa II and Hae II DNA methylases. Nucleic Acids Res 4:4211–4222. doi:10.1093/nar/4.12.4211
Mantel N (1967) The detection of disease clustering and a generalized regression approach. Cancer Res 27:209–220
Massicotte R, Whitelaw E, Angers B (2011) DNA methylation: a source of random variation in natural populations. Epigenetics 6:421–427. doi:10.4161/epi.6.4.14532
McClelland M, Nelson M, Raschke E (1994) Effect of site-specific modification on restriction endonucleases and DNA modification methyltransferases. Nucleic Acids Res 22:3640–3659. doi:10.1093/nar/22.17.3640
Meaney MJ, Ferguson-Smith AC (2010) Epigenetic regulation of the neural transcriptome: the meaning of the marks. Nat Neurosci 13:1313–1318. doi:10.1038/nn1110-1313
Michaud EJ, Van Vugt M, Bultman SJ, Sweet HO, Davisson MT, Woychik RP (1994) Differential expression of a new dominant agouti allele (Aiapy) is correlated with methylation state and is influenced by parental lineage. Genes Dev 8:1463–1472. doi:10.1101/gad.8.12.1463
Migicovsky Z, Kovalchuk I (2011) Frontiers: epigenetic memory in mammals. Front Epigenomics. doi:10.3389/fgene.2011.00028
Morán P, Pérez-Figueroa A (2011) Methylation changes associated with early maturation stages in the Atlantic salmon. BMC Genet 12:86. doi:10.1186/1471-2156-12-86
Morgan HD, Sutherland HGE, Martin DIK, Whitelaw E (1999) Epigenetic inheritance at the agouti locus in the mouse. Nat Genet 23:314–318. doi:10.1038/15490
Norberg UM, Rayner JMV (1987) Ecological morphology and flight in bats (Mammalia; Chiroptera): wing adaptations, flight performance, foraging strategy and echolocation. Philos Trans R Soc B 316:335–427. doi:10.1098/rstb.1987.0030
Ochogavía AC, Cervigni G, Selva JP, Echenique VC, Pessino SC (2009) Variation in cytosine methylation patterns during ploidy level conversions in Eragrostis curvula. Plant Mol Biol 70:17–29. doi:10.1007/s11103-009-9454-5
Parisod C, Christin PA (2008) Genome wide association to fine scale ecological heterogeneity within a continuous population of Biscutella laevigata (Brassicaceae). New Phytol 178:436–447. doi:10.1111/j.1469-8137.2007.02361.x
Parisod C, Trippi C, Galland N (2005) Genetic variability and founder effect in the pitcher plant Sarracenia purpurea (Sarraceniaceae) in populations introduced into Switzerland: from inbreeding to invasion. Ann Bot Lond 95:277–286. doi:10.1093/aob/mci023
Rice WR (1989) Analyzing tables of statistical tests. Evolution 43:223–225. doi:10.2307/2409177
Richards EJ (2006) Inherited epigenetic variation—revisiting soft inheritance. Nat Rev Genet 7:395–401. doi:10.1038/nrg1834
Richards EJ (2008) Population epigenetics. Curr Opin Genet Dev 18:221–226. doi:10.1016/j.gde.2008.01.014
Richards CL, Bossdorf O, Verhoeven KJF (2010) Understanding natural epigenetic variation. New Phytol 187:562–564. doi:10.1111/j.1469-8137.2010.03369.x
Rosenberg MS, Anderson CD (2011) PASSaGE: pattern analysis, spatial statistics and geographic exegesis. Version 2. Method Ecol Evol 2:229–232. doi:10.1111/j.2041-210X.2010.00081.x
Schrey AW, Coon CAC, Grispo MT, Awad M, Imboma T, McCoy ED, Mushinsky HR, Richards CL, Martin LB (2012) Epigenetic variation may compensate for decreased genetic variation with introductions: a case study using house sparrows (Passer domesticus) on two continents. Genet Res Int. doi:10.1155/2012/979751
Skinner MK, Guerrero-Bosagna C (2009) Environmental signals and transgenerational epigenetics. Epigenomics 1:111–117. doi:10.2217/EPI.09.11
Sneath PHA, Sokal RR (1973) Numerical taxonomy. The principles and practice of numerical classification. WH Freeman, San Francisco
Thioulouse J, Chessel D, Dolédec S, Olivier JM (1997) ADE-4: a multivariate analysis and graphical display software. Stat Comput 7:75–83. doi:10.1023/A:1018513530268
Vaughn MW, Tanurdži M, Lippman Z, Jiang H, Carrasquillo R, Rabinowicz PD, Dedhia N, McCombie WR, Agier N, Bulski A (2007) Epigenetic natural variation in Arabidopsis thaliana. PLoS Biol 5:e174. doi:10.1371/journal.pbio.0050174
Vogt G, Huber M, Thiemann M, Van Den Boogaart G, Schmitz OJ, Schubart CD (2008) Production of different phenotypes from the same genotype in the same environment by developmental variation. J Exp Biol 211:510–523. doi:10.1242/jeb.008755
Vos P, Hogers R, Bleeker M, Reijans M, Lee T, Hornes M, Friters A, Pot J, Paleman J, Kuiper M (1995) AFLP: a new technique for DNA fingerprinting. Nucleic Acids Res 23:4407–4414. doi:10.1093/nar/23.21.4407
Waalwijk C, Flavell RA (1978) Msp I, an isoschizomer of Hpa II which cleaves both unmethylated and methylated Hpa II sites. Nucleic Acids Res 5:3231–3236. doi:10.1093/nar/5.9.3231
Xu M, Li XQ, Korban S (2000) AFLP-based detection of DNA methylation. Plant Mol Biol Rep 18:361–368. doi:10.1007/BF02825064
Zhang RZ, Jin SK, Quan GQ, Li SH, Ye Z, Wang FG, Zhang ML (1997) Distribution of mammalian species in China. China Forestry Publishing House, Beijing
Zhang D, Cheng L, Badner JA, Chen C, Chen Q, Luo W, Craig DW, Redman M, Gershon ES, Liu C (2010) Genetic control of individual differences in genes-pecific methylation in human brain. Am J Hum Genet 86:411–419. doi:10.1016/j.ajhg.2010.02.005
Acknowledgments
Our study was supported by the National Natural Science Foundation of China (grant no. 30870371, 30900132 and 31030011), new teacher fund of doctorial program sponsored by Ministry of Education of China (grant no. 20090043120002), the Campus youth fund (grant no. 10QNJJ015) and the Programme of Introducing Talents of Discipline to Universities (grant no. B07017). We thank Dr. Oliver Bossdorf for guidance in questions about ecological epigenetics, and Drs. Xiaofang Zhong, Bao Qi and Linfeng Li for the help in experiments and data analysis. We thank the language editing service “Edanz Group Ltd., China” for the correction and improvement of the article’s English usage.
Author information
Authors and Affiliations
Corresponding author
Additional information
Communicated by S. Hohmann.
Rights and permissions
About this article
Cite this article
Liu, S., Sun, K., Jiang, T. et al. Natural epigenetic variation in the female great roundleaf bat (Hipposideros armiger) populations. Mol Genet Genomics 287, 643–650 (2012). https://doi.org/10.1007/s00438-012-0704-x
Received:
Accepted:
Published:
Issue Date:
DOI: https://doi.org/10.1007/s00438-012-0704-x