Abstract
In this work, authors examine the variabilities of precipitation and surface air temperature (T2m) in Northeast China during 1948–2012, and their global connection, as well as the predictability. It is noted that both the precipitation and T2m variations in Northeast China are dominated by interannual and higher frequency variations. However, on interdecadal time scales, T2m is shifted significantly from below normal to above normal around 1987/1988. Statistically, the seasonal mean precipitation and T2m are largely driven by local internal atmospheric variability rather than remote forcing. For the precipitation variation, circulation anomalies in the low latitudes play a more important role in spring and summer than in autumn and winter. For T2m variations, the associated sea surface pressure (SLP) and 850-hPa wind (uv850) anomalies are similar for all seasons in high latitudes with significantly negative correlations for SLP and westerly wind anomaly for uv850, suggesting that a strong zonal circulation in the high latitudes favors warming in Northeast China. The predictability of precipitation and T2m in Northeast China is assessed by using the Atmospheric Model Inter-comparison Project type experiments which are forced by observed sea surface temperature (SST) and time-evolving greenhouse gas (GHG) concentrations. Results suggest that T2m has slightly higher predictability than precipitation in Northeast China. To some extent, the model simulates the interdecadal shift of T2m around 1987/1988, implying a possible connection between SST (and/or GHG forcing) and surface air temperature variation in Northeast China on interdecadal time scales. Nevertheless, the precipitation and T2m variations are mainly determined by the unpredictable components which are caused by the atmospheric internal dynamic processes, suggesting low predictability for the climate variation in Northeast China.
Similar content being viewed by others
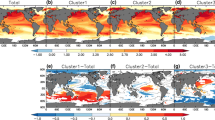
Avoid common mistakes on your manuscript.
1 Introduction
Northeast China (42.5°–50°N, 118°–130°E) is located in the mid-high latitudes of East Asia and in the northern side of East Asian jet stream. It is affected by the general circulation in both the extratropics and tropics. In the mid-high latitudes, blocking and associated cold vortex occur very often. In early summer, with a northward jump of the jet stream, cold vortex and blocking over the Lake Baikal and the Sea of Okhotsk become active (Sun et al. 1994), associated with Rossby wave activity in the mid-high latitudes (Bueh et al. 2008). In summer, both the northward migration of subtropical high over the northwestern Pacific and East Asian summer monsoon as well as the blocking and low vortex in the mid-high latitudes affect the climate in Northeast China (Shen et al. 2011). For winter, the atmospheric circulation in the mid-high latitudes plays a dominated role. On average, summer is short but there is a lot of sunshine, whereas winter is long and cold with a lot of snows (Liu et al. 2010). The temperature range between summer and winter in the region is large and precipitation is largely concentrated in summer (Zhu et al. 2007).
Northeast China is a major industrial and agriculture base of China. Regional climate variability is an important factor affecting people’s daily life and the productions of industrial and agriculture in the region. Previous works have suggested that multiple factors play roles in the climate variability in the region. The first one is the variation of the East Asian monsoon system (e.g., Tao and Chen 1987; Ding 1994; Lian and An 1998; Zhang et al. 2003; Zhu et al. 2007). For example, strong winter monsoon may result in cold and snowy winter, and strong summer monsoon may cause flood in Northeast China (e.g., Ding 1994; Lian and An 1998; Zhu et al. 2007).
Another possible factor is El Niño and Southern Oscillation (ENSO) (Wang and Zhu 1985; Lian and An 1998). However, the connection between ESNO and the climate variation in Northeast China seems lack of robustness. For example, Wu et al. (2003) did not find significant correlations between ENSO and seasonal mean precipitation in Northeast China during 1951–2000. Later, Wu et al. (2010) argued that an interdecadal change occurred in the relationship between ENSO and summer air temperature variability in Northeast China. The summer temperature tends to be warmer (cooler) than normal in El Niño (La Niña) developing years during 1950s through mid-1970s. The relationship is weakened or even becomes opposite in 1980 and 1990s.
In addition to the influence from the eastern tropical Pacific linked with ENSO, the convective activity over the warm pool region in the western Pacific may also affect the climate in East China, Korea, and Japan, through exciting a Pacific-Japan pattern (Nitta 1987; Nitta and Hu 1996). Furthermore, sea surface temperature (SST) in the North Atlantic might also influence the climate variations in Northeast China. For example, Wu et al. (2010, 2011) noted a close relationship between the interannual variations of Northeast China summer temperature and a tripole SST anomaly pattern in the North Atlantic in the preceding spring.
In this work, we further examine the variability of precipitation and surface air temperature in Northeast China, and its connection with atmospheric circulations and global SSTs based on long observational data and model simulations. The predictability is also assessed by using 12 members of AMIP (Atmospheric Model Inter-comparison Project) type experiments which are forced by observed SST and time-evolving greenhouse gas (GHG) concentrations.
The paper is organized as follows. Observational datasets and AMIP experiments are described in Sect. 2. In Sect. 3, the variability of monthly mean precipitation and surface air temperature anomalies and their global connections are presented. The connections of seasonal mean precipitation and surface air temperature anomalies in Northeast China with atmospheric circulation and SST are discussed in Sect. 4. In Sect. 5, the predictability of precipitation and surface air temperature anomalies in Northeast China is assessed. Finally, conclusions with some discussion are given in Sect. 6.
2 Observational datasets and model simulations
We use following monthly mean data in this study: (1) analyzed precipitation from gauge observations (PREC; Chen et al. 2002); (2) analyzed air temperature at 2 m (T2m; GHCN_CAMS; Fan and van den Dool 2008); (3) sea level pressure (SLP), wind at 850 hPa (uv850), and 200-hPa meridional wind (v200) from the National Centers for Environmental Prediction (NCEP) and the National Center for Atmospheric Research (NCAR) reanalysis (Kalnay et al. 1996); and (4) NOAA reconstructed SST (ERSSTv3b; Smith et al. 2008). Here, v200 is used to track the propagation of tropical forced stationary waves (Jin and Hoskins 1995). The precipitation and T2m data used in this work span from January 1948 to February 2013, and the NCEP/NCAR reanalysis data and SST cover January 1949—February 2013. All these observation-based data are called observations in the following.
The AMIP-type runs used in this study come from the atmospheric component (GFS) of the NCEP Climate Forecast System version 2 model (Kumar et al. 2012, Saha et al. 2013). The sub-grid physical process includes simplified Arakawa Schubert deep convection and shallow scheme convection with new mass flux scheme. The model has a horizontal resolution of T126 and 64 vertical levels extending from the surface to 0.26 hPa. The detailed description of model was documented in Saha et al. (2010). The GFS is forced by observed monthly SST, sea ice, and observed time-evolving GHG concentrations. For each simulation, the same observed evolution of SST, sea ice extent, and GHG concentrations were specified as external forcing. There are 12 integrations with slightly different atmosphere initial conditions. Each run is integrated from January 1950—December 2010.
3 Climate variability in Northeast China and its global connection
In this section, we examine the variability of monthly and seasonal precipitation and T2m in Northeast China, and their global connections. Figures 1a and 2a show the monthly precipitation and T2m anomalies averaged in Northeast China (42.5°–50°N, 118°–130°E) from January 1948 to December 2012, respectively. The region used to represent Northeast China was chosen following Wu et al. (2010, 2011). This regional mean represents the coherent variation portion of precipitation and T2m in Northeastern China, which is also the leading mode of empirical orthogonal function analysis for at least summer precipitation (Sun et al. 2000). The representatives of the regional means for both precipitation and T2m are also confirmed by the spatial correlation patterns with same sign in a broad region around Northeast China shown in Figs. 1b and 2b.
a Observed monthly mean air temperature anomaly at 2 m (T2m) averaged in Northeast China (42.5°–50°N, 118°–130°E); b correlation between the time series in (a) and observed T2m anomaly. The T2m data are from GHCN_CAMS in January 1948-Decemebr 2012. The shift around 1987/88 in (a) is significant at confidence level of 99.9 % using T test. The unit is °C in (a)
It is seen from Fig. 1a that precipitation in Northeast China is dominated by interannual and higher frequency variations and there is no obvious trend or decadal variation. The pronounced correlation of precipitation in Northeast China with global precipitation is mainly confined in Northeast Asia and East Siberia (Fig. 1b), suggesting that precipitation variation in Northeast China has a coherent variation within a broad region over Northeast Asia and Mongolia. Meanwhile, there are some weak negative correlations of precipitation variations between Northeast China and Southwest China as well as the high-latitudes of East Siberia. The correlations between Northeast China monthly mean precipitation and global SLP anomalies (not shown) suggest that Northeast China precipitation has negative correlations with SLP anomaly in East Asian land. Above (below) normal precipitation in the Northeast China is associated with negative (positive) SLP anomaly in the East Asian land. Meanwhile, Southwest China is located in the southwest side of the negative (positive) SLP anomaly, favoring dry (wet) in Southwest China and resulting in the opposite variations of precipitation between Northeast and Southwest China.
Different from the precipitation variations shown in Fig. 1a, T2m in Northeast China displays a significant shift from below normal (cold) to above normal (warm) around 1987/1988, in addition to large monthly and interannual variability (Fig. 2a). Compared with the correlation pattern for precipitation (Fig. 1b), T2m variations in Northeast China have a much broader spatial distribution with coherent variations (Fig. 2b). Pronounced positive correlations are seen in the mid-high latitudes of Asia and East Siberia, implying that surface air temperature variation in Northeast China is associated with broad scale circulation anomaly in the mid-high latitudes of Asia and East Siberia. On the other hand, the pronounced positive correlations in the broad region for surface air temperature may be largely caused by the overall and pronounced warming trend in the region (Hu et al. 2003). In our next step work, we will examine the spatial patterns of the climate variability associated with the shift around 1987/1988 in Northeast China and its global connections.
Consistent with monthly anomaly, seasonal mean precipitation anomaly in Northeast China does not show obvious long-term trends (Fig. 3). However, it is noted that variability seems enhanced in spring (March, April, and May: MAM) since about 2003, a dry tendency presents in summer (June, July, and August: JJA) and autumn (September, October, and November: SON) since 1999, and a wet tendency presents in winter (December, January and February: DJF) since 1999.
Same as Fig. 1a, but for seasonal mean precipitation anomalies in spring (March, April, May: MAM), summer (June, July, August: JJA), autumn (September, October, November: SON), and winter (December, January, February, DJF) in 1948–2012/2013. The unit is mm/day
For seasonal mean T2m (Fig. 4), positive anomaly becomes dominant since 1989 in MAM, since 1994 in JJA, since 1988 in SON, and since 1987 in DJF, suggesting a slightly seasonal dependence of the interdecadal variation of T2m in late 1980s. These interdecadal variations for seasonal mean T2m anomalies are generally consistent with the shift around 1987/1988 for monthly mean anomaly shown in Fig. 2a and as well as with a strong warming trend in North and Northeast China, which implies potential impact of increased GHG concentrations on the surface air temperature variations in Northeast China (Hu et al. 2003).
Same as Fig. 3, but for seasonal mean T2m anomalies. The unit is °C
4 Link with general circulation and SST
We now discuss the connection of the seasonal mean precipitation and T2m variations in Northeast China with atmospheric general circulation (SLP, uv850, and v200) and SST. To eliminate the impacts of trend and low-frequency variation and to focus on the relationship at interannual time scales, all the correlations in this section are calculated using high-pass filtered data with time scales shorter than 9 years.
Figure 5 shows the simultaneous correlations between the seasonal mean precipitation anomaly in Northeast China shown in Fig. 3 and SLP and uv850 anomalies of the NCEP/NCAR reanalysis. There are both similarities and differences in the correlation patterns for both SLP and uv850 anomalies in different seasons. For example, all correlations with SLP anomaly (shading in Fig. 5) are negative in Northeast China and it is cyclonic anomaly pattern for uv850 (vectors in Fig. 5). However, the correlations with SLP anomaly in the mid-high latitudes are much weaker and less significant in spring and winter than in summer and autumn (shading in Fig. 5). On the other hand, the correlations with SLP anomaly in the low latitudes are much stronger and more significant in spring and summer than in autumn and winter (shading in Fig. 5), suggesting that circulation anomaly in the low latitudes plays a more important role in spring and summer than in autumn and winter in the precipitation variation in Northeast China. For summer precipitation in Northeast China (Fig. 5b), the circulation anomalies in both low and high latitudes play a role, consistent with the correlation with uv850 anomaly (vectors in Fig. 5). For example, in spring and summer (vectors of Fig. 5a, b), there are clear southerly anomalies along eastern China, implying a moisture transport from the Indian and Northwest Pacific Oceans to Northeast China (Gao 2007). On the contrary, the transportations are less evident for autumn and winter (vectors in Fig. 5c, d). This result is consistent with Sun and Wang (2013) who noted that during widespread snowfall events in northeastern China winter, in addition to the southerly water vapor transport that transports moisture from eastern China, its adjacent seas, and the Sea of Japan, local atmospheric moisture over Northeast China likely makes a considerable contribution to the widespread snowfall.
Simultaneous correlation of observed seasonal mean precipitation anomaly shown in Fig. 3 with anomalies of SLP and wind at 850 hPa in the NCEP/NCAR reanalysis in 1948–2012/2013. The data are high-pass filtered for time scales shorter than 9 years. The hatched regions show the significant correlation with SLP at 95 % confidence level using T-test
For the seasonal mean T2m anomaly in Northeast China, the correlations with SLP and uv850 anomalies over Northeast Asia depict an overall analogue pattern for all four seasons in the high latitudes with significantly negative correlations for SLP and westerly wind anomaly for uv850 (Fig. 6), suggesting that a strong zonal circulation in the high latitudes favors warming in Northeast China. Nevertheless, the correlations change with seasons in the mid-low latitudes, including over Eastern China and the Indian and Northwest Pacific Oceans. For instance, an anticyclone-like correlation for uv850 anomaly and positive correlation for SLP anomaly present in Northeast China in spring and summer (Fig. 6a and b), suggesting that blocking-like anomaly over Northeast China favors warm spring and hot summer. However, in autumn, the correlations with SLP anomaly in Northeast China are small, although there are anticyclone-like correlations for uv850. The blocking-like circulation anomaly over Northeast China is associated with enhanced downward net shortwave radiation at the surface (not shown), that results in positive T2m anomaly. In winter, in addition to positive correlation with SLP anomaly in the regions from India to the Northwest Pacific, positive correlations in the middle latitudes of Northwest Pacific are also noticeable, which may be associated with weakened Aleutian low or blocking-like anomaly in the region that favors transportation of warm air from oceans to Northeast China (see vectors in Fig. 6d).
For global connection, the correlations between seasonal mean precipitation and SST anomalies (contours in Fig. 7) indicate some marginally significant connections with 0.2-0.4 correlation coefficients. For example, there are some significant correlations over the tropical North Atlantic and Indian Oceans in spring (contours in Fig. 7a). Nevertheless, the overall correlations in the equatorial Pacific are not significant for all four seasons, suggesting the precipitation variation in Northeast China seems not significantly affected by ENSO, consistent with weak correlation between ENSO and Northeastern China shown in Wu et al. (2003). Furthermore, from the correlations between seasonal mean precipitation and v200 anomalies (shading in Fig. 7), no significant connections are observed between the precipitation in Northeast China and stationary wave activity, further confirming the insignificant impact of tropical oceans on the precipitation in Northeast China.
Simultaneous correlation of observed seasonal mean precipitation anomaly shown in Fig. 3 with SST (contour) and v200 (shading) anomalies. Hatched and shading regions represent significance correlation at 95 % confidence level using T-test for SST and v200 anomalies, respectively. Contour interval is 0.2 with zero line eliminated. The data are high-pass filtered for time scales shorter than 9 years
On the other hand, there are some significant correlations between seasonal mean T2m and SST anomalies (contours in Fig. 8). The significant negative correlations in the tropical eastern and central Pacific in summer and autumn (Fig. 8b, c) may suggest a possible impact from ENSO. For example, Wu et al. (2010) suggested that Northeast China summer temperature tended to be lower (higher) than normal in El Niño (La Niña) developing years during 1950s through mid-1970s. The relationship between the Northeast China summer temperature and ENSO was weakened or even became opposite in the 1980s and the 1990s. They argued that ENSO affected the Northeast China summer temperature through modulating the South Asian heating and consequently the mid-latitude Asian circulation. After the late 1970s, the connection between ENSO and the Indian summer monsoon and that between the South Asian heating and the mid-latitude Asian circulation have been weakened. This leads to a weakening of ENSO impact on Northeast China summer temperature. Except in spring (Fig. 8a), the correlations with SST anomaly in the tropical North Atlantic are not significant in the other three seasons, consistent with Wu et al. (2011) that no significant connection between North Atlantic SSTA and summer temperature variations in Northeast China could be found in the whole data period. In addition, the significant positive correlations in the Northwestern Pacific in all seasons are probably due to the forcing from the atmospheric circulation variations associated with T2m anomaly in Northeast China.
Interestingly, different from the insignificant stationary wave propagation patterns associated with seasonal precipitation anomalies (shading in Fig. 7), we note some significant stationary wave patterns associated with the seasonal T2m anomalies (shading in Fig. 8). For example, we see a wave pattern propagating from the extratropical North Atlantic via the Eurasian continent to northern Northeast Asia in spring, autumn, and winter (Fig. 8a, c, d), generally consistent with Wu et al. (2011) who argued that a tripole SST anomaly pattern in the North Atlantic had a weak correlation with the Northeast China summer temperature during the 1950s through the mid-1970s, in contrast to the strong negative correlations in the 1980s and the 1990s. This change is related to the difference in the persistence of the tripole SST pattern. Before the late 1970s, the tripole SST pattern weakened from spring to summer, and thus, the spring North Atlantic tripole SST pattern had a weak connection with summer temperature in Northeast China. On the contrary, after the late 1970s, the tripole SST pattern displayed a tendency of persistence from spring to summer, contributing to circulation changes that affected the Northeast China summer temperature. On the other hand, in summer (Fig. 8b), the wave activity is mainly in the central and eastern China and propagates from the northern Indian Ocean to Northeast China via the central eastern China (Hu et al. 2003). The overall results seem to suggest that in addition to the influence of the internal atmospheric dynamical processes, remote forcing may also play a role to some extent on the seasonal mean T2m variations in Northeast China.
Overall, the seasonal climate (precipitation and T2m) variability in Northeast China is mainly affected by atmospheric circulation anomaly in the local and nearby regions, implying that the climate variations in Northeast China, particularly for seasonal mean precipitation, are largely driven by local internal atmospheric variability, and remote forcings (such as ENSO and Atlantic and Indian Ocean SSTs) may not play a dominant role. Nevertheless, the surface air temperature variations in Northeast China show some connections with the remote forcings. This may also be due to the fact that surface air temperature variations in Northeast China are associated with a broader scale pattern than precipitation variations as shown in Figs. 1b and 2b. The differences in the association with the remote forcings between the surface air temperature and precipitation in Northeast China are further supported by the predictability assessment presented below.
5 Predictability
Using the AMIP runs of GFS model, we assess the predictability of precipitation and T2m in Northeast China. Here, the ensemble mean of monthly mean anomalies of 12 members of the AMIP runs is referred to as signal, and the departure of individual member from the ensemble mean is called noise.
Figure 9a is the standard deviation (STDV) of precipitation signal and Fig. 9b is the averaged STDV of precipitation noise. Both STDV values of the signal and the noise decrease from southeast to northwest in East Asia, consistent with the spatial distribution pattern of total precipitation with decreasing from low to high latitudes and from coastal regions to the inner lands. The ratio of the signal to noise STDV (Fig. 9c) is mostly smaller than 0.4 in Northeast China, suggesting small predictability of the regional precipitation. The low signal to noise ratio is also confirmed by the similarity between the STDV of noise (Fig. 9b) and observed STDV (Fig. 9d). This similarity implies that the STDV of observed precipitation is mainly determined by the unpredictable component caused by the atmospheric internal dynamic processes, which is also consistent with analyses shown in Sect. 4.
Precipitation in January 1950-December 2010, a Standard deviation (STDV) of GFS AMIP run of 12 member ensemble mean; b same as (a), but for individual member departure from the ensemble mean; c ratio of signal to noise ((a) divided by (b)); d STDV of the observed precipitation. The unit is mm/day in (a), (b), and (d)
The conclusion of low predictability Northeast China precipitation driven from the signal to noise ratio of the STDV is further verified through a perfect model approach. Figure 10a is the GFS perfect prediction skill, which is computed as the average of correlation between any individual member of the AMIP run and the ensemble mean of the remaining 11 members. If the model was perfect, the perfect prediction skill would be the maximum skill that the model could achieve. We note that the perfect prediction skill shows an overall decrease from the coast to the inner lands and from the low latitudes to higher latitudes. In Northeast China, perfect prediction skill is mainly around 0.1–0.2, consistent with the low signal to noise ratio of the STDV. The differences between the perfect prediction skill and the real prediction skill (the correlation between the observed precipitation and the model simulated ensemble mean precipitation) are also around 0.1–0.2 in Northeast China, suggesting that the model has some potential to improve its prediction skill for precipitation in Northeast China.
Similar to precipitation, low predictability is also seen for T2m over Northeast China. Both STDV values of T2m signal and noise decrease from southeast to northwest in East Asia (Fig. 11a, b). The lower STDV of the signal (Fig. 11a) compared with the STDV of the noise (Fig. 11b) is consistent with the fact that both the spatial distribution pattern and the amplitude of the STDV of noise (Fig. 11b) and observed STDV (Fig. 11d) are comparable, implying that a small fraction of T2m variation in Northeast China may be predictable. This is consistent with the small ratio of signal to noise STDV (Fig. 11c) with amplitude mostly smaller than 0.6 in Northeast China, and with analysis in Sect. 4.
Same as Fig. 9, but for T2m. The unit is °C in (a), (b), and (d)
The perfect model skill for T2m (Fig. 12a) is slightly better than that of precipitation (Fig. 10a), probably because to some extent the model simulates the interdecadal shift around 1987/1988 (Fig. 13), suggesting a possible connection between SST (and/or also GHG forcing) and surface air temperature variation in Northeast China on interdecadal time scales. Nevertheless, compared with observations (Fig. 13a), the amplitude of interdecadal shift is smaller in the model simulations. Meanwhile, the spread among individual ensemble members is large, suggesting that the atmospheric internal dynamical processes play an overwhelm role in the surface temperature variation in Northeast China. Furthermore, the shift in the model simulations is more pronounced around 1994/1995 than around 1987/1988 (Fig. 13b). The change in the model simulation around 1987/1988 is temporary and the temperature returns to negative in just a few years. The differences between the perfect prediction skill and the real prediction skill (the correlation between the observed T2m and the model simulated ensemble mean T2m) are around 0.1–0.2 in Northeast China (Fig. 12b), indicating the potential to improve GFS prediction skill of T2m in Northeast China.
Same as Fig. 10, but for T2m
T2m anomaly in January 1950-December 2010 averaged in Northeast China (42.5o-50oN, 118o-130oE): a observations (GHCN_CAMS); b ensemble mean (shading) and individual member (curves) of GFS AMIP runs. The blue lines represent the means in January 1950-December 1987, and January 1988-December 2010, respectively. The shifts around 1987/1988 in both (a) and (b) are significant at confidence level of 99.9 % using T-test. The unit is °C
Interestingly, we also note that although the overall prediction skills and the improvement potentials for both precipitation and T2m in Northeast China are low, the model performs well in capturing the spatial connection of their global connections of Northeast China precipitation and T2m (compare Figs. 14a with 1b, 14b with 2b). This feature documents the reliability of the model in simulating the large-scale features of climate variability in Northeast China and the broad nearby regions, and also provides confidence for the predictability assessment done in this section.
6 Summary
In this work, we have examined the variabilities of precipitation and surface air temperature (T2m) in Northeast China in 1948–2012 and their global connections, as well as the predictability. It is noted that both precipitation and T2m in Northeast China is dominated by interannual and higher frequency variations. However, T2m shifted significantly from below normal (cold) to above normal (warm) around 1987/1988.
The associated SLP and uv850 anomalies for the precipitation in Northeast China are a cyclone-like pattern in Northeast China and its nearby regions. The correlations with SLP anomaly in the mid-high latitudes are less significant in spring and winter than in summer and autumn, and the correlations with SLP anomaly in the low latitudes are more significant in spring and summer than in autumn and winter. That suggests that circulation anomaly in the low latitudes plays a more important role in spring and summer than in autumn and winter for the precipitation variation in Northeast China. In spring and summer, there are apparent southerly anomalies along the eastern China, implying to transport moisture from the Indian and Northwest Pacific Oceans to Northeast China. On the contrary, moisture transportation is less evident for autumn and winter. The associated SLP and uv850 anomalies for the T2m is similar for all seasons in the high latitudes with significantly negative correlations for SLP and westerly wind anomaly for uv850, suggesting that a strong zonal circulation in the high latitudes favors warming in Northeast China. On the other hand, the correlations vary with seasons in the mid-low latitudes. Anomalous blocking over Northeast China favors warm spring and hot summer. SLP anomaly in Northeast China is small in autumn and weakened Aleutian low or blocking-like anomaly in the middle latitudes of Northwest Pacific favors transportation of warm air from oceans to Northeast China and generates warm winter.
It should be indicated that the results for precipitation and T2m are the averages over the whole Northeast China without considering their spatial variations within the region, although Liu et al. (2010) have shown a large homogeneity of the regional climate. It is further shown that the seasonal mean precipitation and surface temperature anomalies in Northeast China are largely driven by local internal atmospheric variability rather than remote forcings, suggesting low predictability for the climate variability in Northeast China. The low predictability for the climate anomaly in Northeast China is in agreement with the fact that predictable signal in East Asia is mainly associated with ENSO (Liang et al. 2009; Yang et al. 2008), but the influence of ENSO on Northeast China is weak (Wu et al. 2003).
The predictability of precipitation and T2m in Northeast China is assessed by using the AMIP runs of GFS model forced by observed SST and time-evolving GHG concentrations. Overall results suggest that T2m has slightly higher predictability than precipitation in Northeast China. It is indicated that observed precipitation and T2m variabilities are mainly determined by the unpredictable component which is caused by the internal atmospheric dynamic processes. The perfect prediction skills for both precipitation and T2m are around 0.1–0.2 in Northeast China, suggesting low predictability for the climate variations in Northeast China. Nevertheless, to some extent, the model simulates the interdecadal shift of surface air temperature around 1987/1988, implying a possible connection between SST (and/or GHG forcings) and surface air temperature variation in Northeast China on interdecadal time scales.
It should be mentioned that the perfect model skill is achieved under the assumption that the model is perfect. Unfortunately, general circulation models (GCMs) still have obvious biases in the Northwest Pacific and Asian regions (e.g., Kang et al. 2002; Zhou et al. 2009; Xue et al. 2013). For example, the mean position and seasonal migration of the subtropical high over the western Pacific, which directly affects the location of rain belt of the East Asian summer monsoon, were unable to be simulated well by GCMs (Kang et al. 2002). Also, in historical runs, models cannot reproduce the zonal land-sea thermal contrast in summer across the East Asia (Zhou et al. 2009), which is a major driver for the East Asia summer monsoon. Furthermore, in the AMIP-type experiments used in this work, the atmospheric feedback to oceans is inhibited. This is particularly questionable for the monsoon in the Northwest Pacific and East Asian regions, where air-sea coupling plays an important role in the summer monsoon variation (Wang et al. 2005; Zhu and Shukla 2013). Therefore, the perfect model skill actually reflects the influence of global SST on model climate.
In fact, besides SST, other factors such as sea ice (Li and Wu 2012), soil moisture (Yang and Lau 1998; Yang et al. 2007), and stratospheric processes may also provide additional prediction skill (National Research Council 2010). It also should be pointed out that the prediction skill discussed in this work is the average of all seasons. It may be seasonal dependent, with some seasons higher (or lower).
Lastly, the northern part of East Asia, especially the area from North China to East Siberia via Mongolia and Northeast China, is a region sensitive to the increase in GHG concentrations (Hu et al. 2000, 2003; Qian and Leung 2007; Zhu et al. 2012). The impact of the increase in GHG concentrations on climate variability and predictability in Northeast China is an interesting future research topic. Specifically, what role does the increase in GHG concentrations play in the surface air temperature shift in Northeast China around 1987/1988?
References
Bueh CL, Shi N, Ji L et al (2008) Features of the EAP events on the medium-range evolution process and the mid- and high-latitude Rossby wave activities during then Meiyu period. Chin Sci Bull 53:111–121 (in Chinese with English abstract)
Chen M, Xie P, Janowiak JE, Arkin PA (2002) Global land precipitation: a 50-year monthly analysis based on gauge observations. J Hydrometeor 3:249–266
Ding YH (1994) Monsoons over China. Kluwer Academic Publisher, London 419 pp
Fan Y, van den Dool H (2008) A global monthly land surface air temperature analysis for 1948-present. J Geophys Res 113:D01103. doi:10.1029/2007JD008470
Gao ZT (2007) Moisture transportation and budget over Northeast China. Scientia Geographica Sinica. 27(supp.):28–38 (in Chinese with English abstract)
Hu Z-Z, Bengtsson L, Arpe K (2000) Impact of global warming on the Asian winter monsoon in a coupled GCM. J Geophys Res 105(D4):4607–4624
Hu Z–Z, Yang S, Wu R (2003) Long-term climate variations in China and global warming signals. J Geophys Res 108(19):4614. doi:10.1029/2003JD003651
Jin F–F, Hoskins BJ (1995) The direct response to tropical heating in a baroclinic atmosphere. J Atmos Sci 52:307–319
Kalnay E, Kanamitsu M, Kistler R, Collins W et al (1996) The NCEP/NCAR 40-year reanalysis project. Bull Amer Meteor Soc 77:437–471
Kang I-S, Jin K, Wang B et al (2002) Intercomparison of the climatological variations of Asian summer monsoon precipitation simulated by 10 GCMs. Clim Dyn 19:383–395
Kumar A, Chen M, Zhang L, Wang W, Yang Y, Wen C, Marx L, Huang B (2012) An analysis of the nonstationarity in the bias of sea surface temperature forecasts for the NCEP Climate Forecast System (CFS) version 2. Mon Wea Rev 140:3003–3016
Li J, Wu Z (2012) Importance of autumn Arctic sea ice to northern winter snowfall. Proc Natl Acad Sci USA 109(28):E1899–E1990. doi:10.1073/pnas.1205075109
Lian Y, An G (1998) The relationship among El Niño, East Asia summer monsoon, and low temperature in Songliao Plains of Northeast China. J App Meteor Sci 56(6):724–735 (in Chinese with English abstract)
Liang JY, Ang S, Hu Z–Z, Huang BH, Kumar A, Zhang ZQ (2009) Predictable patterns of the Asian and Indo-Pacific summer precipitation in the NCEP CFS. Clim Dyn 32:989–1001
Liu S, Yang S, Lian Y et al (2010) Time–frequency characteristics of regional climate over Northeast China and their relationships with atmospheric circulation patterns. J Clim 23:4956–4972
National Research Council (2010) Assessment of Intraseasonal to Interannual Climate Prediction and Predictability, 192 PP, ISBN-10: 0-309-15183-X, the National Academies Press. Washington, DC
Nitta T (1987) Convective activities in the tropical western Pacific and their impact on the Northern Hemisphere summer circulation. J Meteor Soc Japan 65:373–390
Nitta T, Hu Z–Z (1996) Summer climate variability in China and its association with 500 hPa height and tropical convection. J Meteor Soc Japan 74:425–445
Qian Y, Leung LR (2007) A long-term regional simulation and observations of the hydroclimate in China. J Geophys Res D112. doi:10.1029/2006JD008134
Saha S, Moorthi S, Pan H-L, Wu X et al (2010) The NCEP climate forecast system reanalysis. Bull Amer Meteor Soc 91:1015–1057. doi:10.1175/2010BAMS3001.1
Saha S, Moorthi S, Wu X, Wang J, Nadiga S, Coauthors (2013) The NCEP Climate Forecast System Version 2. J Clim (revised)
Shen BZ, Lin ZD, Lu RY et al (2011) Circulation anomalies associated with interannual variation of early- and late-summer precipitation in Northeast China. Sci China Earth Sci 54(7):1095–1104. doi:10.1007/s11430-011-4173-6
Smith TM, Reynolds RW, Peterson TC, Lawrimore J (2008) Improvements to NOAA’s historical merged land-ocean surface temperature analysis (1880-2006). J Clim 21:2283–2296
Sun B, Wang H (2013) Water vapor transport paths and accumulation during widespread snowfall events in Northeastern China. J Clim 26:4550–4566
Sun L, Zhen XY, Wang Q (1994) The climatological characteristics of Northeast cold vortex in China. Quart J Appl Meteor 5:297–303 (in Chinese with English abstract)
Sun L, An G, Ding L, Shen BZ (2000) A climatic analysis of summer precipitation features and anomaly in Northeast China. Acta Meteorologica Sinica 58(1):70–82 (in Chinese with English abstract)
Tao SY, Chen LX (1987) A review of recent research of the East Asian summer monsoon in China. CP Chang and TN Krishnamurti, Ed, Oxford Univer Press, London, Monsoon Meteorology, pp 60–92
Wang S-W, Zhu H (1985) Cool summer in East Asia and El Niño. Chinese Sci Bull 17:1323–1325 (in Chinese)
Wang B, Ding Q, Fu X, Kang I-S, Jin K, Shukla J, Doblas-Reyes F (2005) Fundamental challenges in simulation and prediction of summer monsoon rainfall. Geophys Res Lett 32(15):L15711. doi:10.1029/2005GL022734
Wu R, Hu Z–Z, Kirtman BP (2003) Evolution of ENSO-related rainfall anomalies in East Asia. J Clim 16(22):3742–3758
Wu R, Yang S, Liu S, Sun L, Lian Y, Gao ZT (2010) Changes in the relationship between Northeast China summer temperature and ENSO. J Geophys Res 115:D21107. doi:10.1029/2010JD014422
Wu R, Yang S, Liu S, Sun L, Lian Y, Gao ZT (2011) Northeast China summer temperature and North Atlantic SST. J Geophys Res 116:D16116. doi:10.1029/2011JD015779
Xue Y, Chen M, Kumar A, Hu Z-Z, Wang W (2013) Prediction skill and bias of tropical Pacific sea surface temperatures in the NCEP climate forecast system version 2. J Clim 26(15): 5358–5378. doi:10.1175/JCLI-D-12-00600.1
Yang S, Lau K-M (1998) Influence of SST and ground wetness on the Asian summer monsoon. J Clim 11:3230–3246
Yang S, Yoo S-H, Yang R, Mitchell KE, van den Dool H, Higgins W (2007) Response of seasonal simulations of a regional climate model to high-frequency variability of soil moisture during the summers of 1988 and 1993. J Hydrometeor 8: 738–757. doi:10.1175/JHM616.1
Yang S, Zhang ZQ, Kousky VE, Higgins RW, Yoo S-H, Liang JY, Fan Y (2008) Simulations and seasonal prediction of the Asian summer monsoon in the NCEP Climate Forecast System. J Clim 21:3755–3775
Zhang QY, Tao SY, Chen LT (2003) The interannual variability of East Asian summer monsoon indices and its association with the pattern of general circulation over East Asia. Acta Meteor Sinica 61:559–568
Zhou T et al (2009) The CLIVAR C20C Project: which components of the Asian-Australian Monsoon circulation variations are forced and reproducible? Clim Dyn 33:1051–1068. doi:10.1007/s00382-008-0501-8
Zhu J, Shukla J (2013) The role of air-sea coupling in seasonal prediction of Asian-Pacific summer monsoon rainfall. J Clim 26 (15): 5689–5697, doi:10.1175/JCLI-D-13-00190.1
Zhu Q, Lin J, Shou S, Tang D (2007) Synoptic Meteorology Principles and Methodologies, 1-649. China Meteor Press, Beijing
Zhu C, Wang B, Qian W, Zhang B (2012) Recent weakening of northern East Asian summer monsoon: a possible response to global warming. Geophys Res Lett 39:L09701. doi:10.1029/2012GL051155
Acknowledgments
We thank the constructive comments and suggestions from two reviewers. We would also like to thank Emily Becker for providing GHCN_CAMS T2m data, and Mingyue Chen for providing PREC precipitation data. This work was jointly supported by the R&D Special Fund for Public Welfare Industry (Meteorology; Grant GYHY201106015), National Basic Research Program of China (Grant 2012CB955303), the National Natural Science Foundation of China (Grant 41275096), and Zhongshan University “985 Project” Phase 3.
Author information
Authors and Affiliations
Corresponding author
Electronic supplementary material
Below is the link to the electronic supplementary material.
Rights and permissions
About this article
Cite this article
Gao, Z., Hu, ZZ., Jha, B. et al. Variability and predictability of Northeast China climate during 1948–2012. Clim Dyn 43, 787–804 (2014). https://doi.org/10.1007/s00382-013-1944-0
Received:
Accepted:
Published:
Issue Date:
DOI: https://doi.org/10.1007/s00382-013-1944-0