Abstract
Purpose
Brain imaging of FDG uptake and cerebrospinal fluid (CSF) concentration of amyloid-beta 1–42 (Aβ1-42) or tau proteins are promising biomarkers in the diagnosis of Alzheimer’s disease (AD). There is still uncertainty regarding any association between decreased FDG uptake and alterations in CSF markers.
Methods
The relationship between FDG uptake, CSF Aβ1-42 and total tau (T-tau), as well as the Mini-Mental State Examination (MMSE) score was investigated in 34 subjects with probable AD using step-wise linear regression. FDG uptake was scaled to the pons.
Results
Scaled FDG uptake was significantly reduced in the probable AD subjects compared to 17 controls bilaterally in the precuneus/posterior cingulate area, angular gyrus/inferior parietal cortex, inferior temporal/midtemporal cortex, midfrontal cortex, and left caudate. Voxel-based single-subject analysis of the probable AD subjects at p < 0.001 (uncorrected) revealed a total volume of significant hypometabolism ranging from 0 to 452 ml (median 70 ml). The total hypometabolic volume was negatively correlated with the MMSE score, but it was not correlated with the CSF measures. VOI-based step-wise linear regression revealed that scaled FDG uptake in the precuneus/posterior cingulate was negatively correlated with CSF Aβ1-42. Scaled FDG uptake in the caudate was positively correlated with CSF T-tau.
Conclusion
The extent and local severity of the reduction in FDG uptake in probable AD subjects are associated with cognitive impairment. In addition, there appears to be a relationship between local FDG uptake and CSF biomarkers which differs between different brain regions.
Similar content being viewed by others
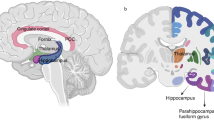
Explore related subjects
Discover the latest articles, news and stories from top researchers in related subjects.Avoid common mistakes on your manuscript.
Introduction
Specific biomarkers of Alzheimer’s disease (AD) in the cerebrospinal fluid (CSF) and functional imaging using positron emission tomography (PET) with the glucose analogue 18F-fluorodeoxyglucose (FDG) have been investigated in recent years in order to improve the accuracy of diagnosing AD (see references [1] and [2] for review).
Changes in CSF biomarkers in AD comprise an increase in total tau protein (T-tau) and tau phosphorylated at threonine 181 (P-tau181P) as well as a decrease in beta-amyloid peptide (Aβ1-42) [2–4]. These changes are thought to reflect the defining pathology of AD, i.e. extracellular accumulation of soluble oligomers and nonsoluble polymers of misfolded Aβ, and intracellular accumulation of hyperphosphorylated tau into neurofibrillary tangles [5, 6]. It has been hypothesized that the decrease in Aβ1-42 in CSF is due to Aβ1-42 accumulation in amyloid plaques which thereby act as an Aβ1-42 “sink”, and/or reduced clearance of Aβ1-42 to the CSF [5, 7], while the increase in tau in the CSF is thought to be related to the degeneration of neurofibrillary tangle-loaded neurons [6]. However, the exact mechanisms relating changes in CSF biomarkers to pathophysiological processes in AD are still unknown.
Changes in FDG uptake indicate AD-related alterations in the local metabolic rate of glucose in the brain. At early stages, prior to the occurrence of significant cell loss, reduced glucose metabolism most likely reflects dysfunction and/or loss of presynaptic terminals [8]. Therefore, in early AD stages the spatial pattern of hypometabolism (posterior cingulate/precuneus, temporo-parietal cortex) is not identical with the spatial pattern of Aβ or tau accumulation (medial temporal lobe), but rather tends to overlap with brain regions most strongly innervated by the Aβ- or tau-affected brain regions [9].
CSF biomarkers and FDG PET both provide good sensitivity for the detection of AD, in the ≥90% range, but specificity for discrimination between AD and non-AD neurodegenerative disorders is limited, in the 70–80% range [10, 11]. Combination of CSF biomarkers and FDG PET might, in principle, improve specificity, although there are only a few studies dealing with this issue.
To our best knowledge, there are presently only four studies which investigated the relationship between CSF Aβ and tau and local brain activity as measured by FDG PET [12–15]. Only two of these studies included subjects with clinical AD. Okamura et al. performed FDG PET and CSF analysis in 15 subjects with probable AD. Whole-brain and local metabolism in the inferior temporal cortex showed a positive correlation with CSF Aβ1-42 [12]. There was no significant correlation between global or local brain metabolism and CSF T-tau. In a very recent study, Ceravolo et al. investigated the relationship between CSF T-tau and P-tau181P and local FDG uptake scaled to the cerebellum in a cohort of 28 subjects with probable AD [15]. They found a negative correlation between both T-tau and P-tau181P and scaled FDG uptake bilaterally in the temporal lobe, the parietal lobe, and the entorhinal/hippocampal region. In addition, T-tau was negatively correlated with scaled FDG uptake in the occipital lobe. Because of the rather small sample size of the first study and the restriction to CSF T-tau and P-tau181P of the second study, validation/replication of these findings appears important.
The aim of the present retrospective study, therefore, was to investigate the relationship between the CSF markers Aβ1-42, T-tau, P-tau181P and local FDG uptake in an independent sample of subjects with probable AD. Cognitive performance as measured by the Mini-Mental State Examination (MMSE) and apolipoprotein E (apoE) genotype were also taken into account. Included in the study were 34 subjects in whom probable AD according to the NINDS-ADRDA criteria had been confirmed after clinical follow-up of at least 24 months after PET. The hypothesis put to test was that, within brain regions with AD-related reduction of FDG uptake, the magnitude of the reduction is correlated with CSF biomarkers of AD. Voxel-by-voxel statistical parametric mapping [16] and analysis based on volumes of interest (VOI) were used to address this hypothesis.
Materials and methods
Subjects with probable AD
Subjects with probable AD were recruited retrospectively from all subjects who had been referred for brain FDG PET to the Department of Nuclear Medicine of the University Medical Center Hamburg-Eppendorf (UKE) by the UKE Memory Clinics between February 1998 and October 2004 using the following (and only the following) inclusion and exclusion criteria. Inclusion criteria were:
-
I1
The subject was diagnosed with possible or probable AD according to the NINDS-ADRDA criteria [17] prior to PET imaging.
-
I2
CSF markers Aβ1-42, T-tau, and P-tau181P had been obtained no longer than 2 weeks before or after the PET scan.
-
I3
Visual evaluation of the FDG PET images had resulted in grading scores of 0 (definitely normal; n = 2 subjects), 1 (probably normal; n = 5), 2 (definitely abnormal with varying degree of bilateral temporoparietal hypometabolism; n = 4), or 3 (classic bilateral temporoparietal hypometabolism; n = 23) as described and validated by Hoffman et al. [18] for the classification of brain FDG PET images in subjects with suspected AD.
-
I4
During clinical follow-up of at least 2 years a previous diagnosis of possible AD was strengthened to probable AD according to the NINDS-ADRDA criteria, or the previous diagnosis of probable AD was confirmed.
Exclusion criteria were
-
E1
Age below 50 years.
-
E2
Brain lesions (tumour, insult, multiinfarction) reported from conventional CT or MRI.
-
E3
Frontally accentuated atrophy reported from conventional CT or MRI.
-
E4
Visual evaluation of the PET images had resulted in a grading score of 4 (abnormal, but no AD pattern, such as frontal, focal, or only unilateral hypometabolism) as described by Hoffman et al. [18].
These criteria were fulfilled by 34 subjects, 15 women and 19 men, aged between 50.1 and 73.8 years (mean±1 standard deviation 63.4±6.2 years). MMSE scores at the time of the PET/CSF examinations ranged from 7 to 28 (20.5±5.9). Subjects with possible AD but not yet probable AD at the time of PET and CSF examinations were included to cover a large range of stages of AD.
Control subjects
A group of control subjects was included to identify AD-related brain regions of hypometabolism. Subsequent correlation analyses were then restricted to these brain regions.
A group of 19 consecutive subjects from routine clinical practice were recruited retrospectively. In these subjects whole-body FDG PET including the brain had been performed for of a non-CNS oncological indication. Subject preparation and brain scanning, however, had been performed according to the same standard protocols as in the subjects with probable AD. Exclusion criteria were:
-
E1
Psychiatric or neurological disorders including memory complaints.
-
E2
Psychotropic medication at the time of PET.
-
E3
Abnormal findings in the brain based on visual evaluation of the PET images by an experienced reader.
Exclusion criteria E1 and E2 were based on the subject’s self-report during the documentation of the anamnesis for the PET scanning.
PET scans of controls were tested voxel-by-voxel using the leave-one-out strategy. The Statistical Parametric Mapping (SPM) software version 2 (SPM2) was used for this purpose [16]. Subjects who showed any significant cluster of either hypo- or hypermetabolism at p < 0.05 corrected for multiple comparisons were excluded. This applied to two subjects. The remaining 17 subjects were included as controls for the present study, 8 women and 9 me, aged between 47 and 69 years (58.9±6.4 years). The controls did not differ from probable AD subjects with respect to gender (p = 1.000, two-sided chi-squared test). However, the control subjects were on average 4.5 years younger than the probable AD subjects. This effect was significant (p = 0.019, two-sided unpaired t-test for equal variances). Therefore, age was included as nuisance variable in the between-groups analyses.
CSF sampling and analysis
CSF samples of the probable AD subjects were taken by lumbar puncture between L3 and L4. CSF samples were aliquoted at the bed-side and directly frozen on dry ice within 10 min of collection. The samples were stored at −80°C and thawed immediately before analysis. CSF biomarkers Aβ1-42, T-tau and P-tau181P were determined by ELISA using commercially available kits (Innotest, Innogenetics, Ghent, Belgium). CSF sampling was not performed in the control subjects.
ApoE
The apoE genotype was determined using the restriction isotyping method as described by Hixson and Vernier [19]. ApoE status was determined in 31 of the 34 subjects with probable AD. The remaining three subjects did not consent to apoE genotyping.
PET imaging
PET imaging was performed on a full-ring PET system ECAT EXACT 921/47 (Siemens/CTI, Knoxville, TN, USA) in 2-D mode [20]. Subjects fasted for at least 4 h prior to injection of about 370 MBq FDG. A static emission scan of 20 min duration was acquired about 45 min after injection. The sinograms were corrected for random coincidences, radioactive decay, dead time, and varying detector efficiency. Calculated attenuation correction was applied as provided by the scanner software (ECAT 6.5 B). Transaxial slices with 128×128 voxels were reconstructed using an iterative ordered subsets expectation maximization method. Subsequently, images were smoothed by application of a 5×5×3 binomial kernel. The voxel size was 1.7×1.7×3.4 mm3, spatial resolution was about 7 mm full-width at half-maximum (FWHM). No scatter correction was performed.
Image preprocessing
Individual PET images were stereotactically normalized (including warping) using the Normalize Tool of SPM2. A custom-made brain FDG PET template in Montreal Neurological Institute (MNI) space was used as reference image for stereotactic normalization. In preparation for voxel-based statistical analysis, the normalized images were smoothed with an isotropic 3-D gaussian kernel with 10 mm FWHM resulting in a final spatial resolution of about 12 mm FWHM.
The smoothed and normalized images were scaled to a common mean voxel value within the pons [21]. The pons VOI as proposed by the International Consortium for Brain Mapping (ICBM) and implemented in the PickAtlas software package version 1.04 was used to delineate the pons (volume 20.9 ml).
Statistical analysis
First, scaled FDG uptake was compared between the group of probable AD subjects and the control group on a voxel-by-voxel basis using ANCOVA as implemented in SPM2 with age as nuisance variable. Proportional scaling was turned off, since the images had been scaled during preprocessing. A p-value of 0.05 corrected for multiple comparisons was employed for definition of statistical significance (probability of family-wise type I error <0.05). Only clusters of at least 125 voxels (= 1 ml) were considered. Anatomical labelling of significant clusters was performed using the SPM toolbox Automated Anatomic Labeling (AAL) made available by Tzourio-Mazoyer et al. [22].
Second, voxel-by-voxel single-subject analysis was performed, i.e. scaled FDG uptake in each individual subject with probable AD was compared to the uptake in the control group using the two-sample t-test implemented as PET model in SPM2 (no proportional scaling, no nonsphericity correction). A significance level of p < 0.001 uncorrected for multiple comparison was used, which is commonly accepted as appropriate in single-subject analysis of brain FDG PET images. The lower threshold on the cluster volume was set to 125 voxels (= 1 ml). The brain volume with significant reduction of scaled FDG uptake, in the following also denoted as ’hypometabolic volume’, was determined in each probable AD subject from the total number of non-zero voxels in the thresholded t-map.
Step-wise linear regression was performed to test for potential relationships between the (size of the) hypometabolic volume in the probable AD subjects (dependent variable) and age, MMSE score, Aβ1-42, or T-tau (independent variables). F-probabilities of 0.05 and 0.10 were set for inclusion and exclusion, respectively. A constant term was included. The step-wise linear regression analysis was repeated with the number of E4 alleles as additional regressor (regression analysis was first performed without the number of E4 alleles, because this variable was not available in three probable AD subjects). SPSS 15.0.1 for Windows (SPSS, Chicago, IL) was used for the regression analyses.
Finally, step-wise linear regression was performed to test for relationships between VOI-based scaled FDG uptake in the probable AD subjects and age, MMSE score, Aβ1-42, and T-tau. F-probabilities of 0.05 and 0.10 were set for inclusion and exclusion, respectively. A constant term was included. The regression analysis was performed separately for each AAL VOI (or union of AAL VOIs) in which the voxel-by-voxel group comparison had detected a cluster of significant reduction in scaled FDG uptake in the probable AD subjects compared to the controls. Again, the VOI-based regression analyses were repeated with the number of E4 alleles as additional regressor. Bonferroni correction for the number of VOIs was not performed, following the recommendation of Perneger [23].
Results
CSF markers in the subjects with probable AD were Aβ1-42 419±107 pg/ml (range 202–596 pg/ml), T-tau 485±64 pg/ml (203–1136 pg/ml), and P-tau181P 97.8±56.0 pg/ml (0–286 pg/ml). Analysis of bivariate correlation between each pair of variables including Aβ1-42, T-tau, and P-tau181P, as well as age and MMSE score revealed a strong correlation between T-tau and P-tau181P which was also highly significant statistically (Pearson’s correlation coefficient 0.852, p = 0.000; Table 1). Because of this high redundancy of T-tau and P-tau181P, only the results for T-tau are presented in the following. T-tau was chosen instead of P-tau181P because it was available in all 34 probable AD subjects, while P-tau181P was missing in one subject. There was no significant correlation between any pair of the variables Aβ1-42, T-tau, age, and MMSE score (Table 1).
The number of E4 alleles was positively correlated with T-tau (Pearson’s correlation coefficient 0.374, p = 0.038), but there was no significant correlation between the number of E4 alleles and Aβ1-42, age, or MMSE score (Table 1).
Voxel-by-voxel ANCOVA of scaled FDG uptake with age as nuisance variable revealed significantly reduced FDG uptake in the probable AD subjects bilaterally in the precuneus/posterior cingulate area, the angular gyrus/inferior parietal cortex, the inferior temporal/midtemporal cortex, the midfrontal cortex, and the left caudate (Fig. 1). There were no clusters of significantly increased scaled FDG uptake in the probable AD subjects.
Voxel-by-voxel ANCOVA of the scaled FDG uptake with age as nuisance variable. Shown are areas of significantly reduced scaled FDG uptake in the probable AD group (n = 34) compared to the controls (n = 17). The t-map corresponding to 48 degrees of freedom was thresholded at p = 0.05 corrected for multiple comparisons (family-wise error rate <0.05), restricted to clusters >1.0 ml, converted to a binary mask (white), and then overlaid to mean scaled FDG uptake in the probable AD group. There were no clusters of significantly increased scaled FDG uptake in the probable AD subjects
Transverse slices of the voxel-by-voxel coefficient of variance (COV), defined as COV (%) = 100×standard deviation/mean, in the group of probable AD subjects and in the control group are given in Fig. 2. The COV in the control group was rather uniform in cortical brain regions suggesting that the power of the ANCOVA to detect alterations in scaled FDG uptake in the probable AD subjects was more or less uniform over the whole brain. In the probable AD group, the COV was increased particularly in those brain regions in which scaled FDG uptake was significantly decreased compared to the controls (cf. Fig. 1). The results of an investigation concerning whether this additional variability of scaled FDG uptake in the probable AD subjects could be (in part) explained by age, MMSE score, Aβ1-42, and/or T-tau are presented below.
The voxel-based single-subject analysis of the probable AD subjects revealed a total volume of significant hypometabolism ranging from 0 to 452 ml (median 70 ml). The two subjects with a hypometabolic volume larger than 300 ml showed significant enlargement of the ventricular space in addition to the typical AD pattern of hypometabolism.
Step-wise linear regression of the hypometabolic volume with age, MMSE score, Aβ1-42, and T-tau as independent variables revealed a significant explanation of variance only by the MMSE score (Table 2, Fig. 3). Age, Aβ1-42, and T-tau were not included in the regression model (p≥0.314). Step-wise linear regression with the number of E4 alleles as additional regressor confirmed these results. The number of E4 alleles was not included in the model (p = 0.367).
Total brain volume with significant reduction of scaled FDG uptake (hypometabolic volume) in the probable AD patients versus MMSE score. The Hoffman scores [18] for visual evaluation of FDG PET images in AD subjects are explained in the subsection “Subjects with probable AD” in Materials and methods
The VOI-based regression analysis included the following VOIs reflecting the areas of significantly reduced scaled FDG uptake in the probable AD subjects according to the voxel-by-voxel ANCOVA (Fig. 1):
-
Precuneus/posterior cingulate (defined as the union of the following standard VOIs predefined in the AAL atlas: precuneus_L + precuneus_R + cingulum_post_L + cingulum_post_R).
-
Angular gyrus/inferior parietal cortex (angular_L + angular_R + parietal_inf_L + parietal_inf_R).
-
Inferior temporal/midtemporal cortex (temporal_inf_L + temporal_inf_R + temporal_mid_L + temporal_mid_R).
-
Midfrontal cortex (frontal_mid_L + frontal_mid_R).
-
Caudate (caudate_L + caudate_R).
-
Cerebellum (cerebelum_crus1_L + cerebelum_crus1_R + cerebelum_crus2_L + cerebelum_crus2_R + cerebelum_3_L + cerebelum_3_R + … + cerebelum_10_L + cerebelum_10_R).
The cerebellum was included as control VOI in which no effect was expected. Univariate analysis of variance with age as covariate confirmed the results of the voxel-by-voxel ANCOVA, i.e. the reduction of scaled FDG uptake in the probable AD subjects compared to the controls was highly significant (p < 0.005) in all VOIs except the cerebellum VOI (p = 0.272; Fig. 4). Age had a highly significant effect in the caudate VOI (p = 0.002), but no significant effect in the other VOIs.
The results of the VOI-based step-wise linear regression analysis are given in Table 2. Scaled FDG uptake showed a significant positive partial correlation with MMSE score in the precuneus/posterior cingulate (Fig. 5a), the angular gyrus/inferior parietal cortex, and in the inferior temporal/midtemporal cortex. Scaled FDG uptake in the precuneus/posterior cingulate in addition showed a negative partial correlation with Aβ1-42 (Fig. 5b). Scaled FDG uptake in the caudate was negatively correlated with age and positively with T-tau (Fig. 5c). There was no significant effect in the midfrontal cortex or in the cerebellar control VOI. These results were confirmed by the repeat regression analyses with the number of E4 alleles as additional regressor. The number of E4 alleles was not included in the model in any of the VOIs.
Scatter plots of (a) scaled FDG uptake in precuneus/posterior cingulate versus MMSE score (inset with FDG uptake corrected for Aβ1-42 using the regression coefficient of the linear regression analysis), (b) scaled FDG uptake in the precuneus/posterior cingulate versus Aβ1-42 (inset with FDG uptake corrected for MMSE score), and (c) scaled FDG uptake in the caudate versus T-tau (inset with FDG uptake corrected for age)
Discussion
Scaled FDG uptake was significantly reduced in the probable AD subjects included in the present study bilaterally in the precuneus/posterior cingulate area, the angular gyrus/inferior parietal cortex, the inferior temporal/midtemporal cortex, the midfrontal cortex, and the left caudate, in very good agreement with previous findings [11, 24, 25]. There was a medium to strong positive correlation between the spatial extent of hypometabolism and cognitive impairment (MMSE scores), supporting the hypothesis that a progressive decrease in brain metabolism is paralleled by cognitive decline during the course of AD [26]. Regression analyses identified a significant positive correlation between MMSE scores and scaled FDG in the precuneus/posterior cingulate, the angular gyrus/inferior parietal cortex, and the inferior temporal/midtemporal cortex. There was no significant correlation between the tested CSF biomarkers and the global extent of hypometabolism. However, VOI-based regression analyses revealed a negative partial correlation between CSF Aβ1-42 and scaled FDG uptake in the precuneus/posterior cingulate and a positive partial correlation between CSF T-tau and scaled FDG uptake in the caudate.
The direction of both of these correlations appears unexpected at first glance [5–7]. However, it has recently been reported that – although CSF Aβ1-42 is reduced compared to controls – it might increase during the course of the clinical phase of AD. Bouwman et al. observed a significant 14% increase in CSF Aβ1-42 at follow-up 21 months after baseline in 50 patients with probable AD [27]. This increase in CSF Aβ1-42 during the course of AD is expected to parallel a decrease in glucose metabolism in brain regions commonly affected in AD, such as the precuneus/posterior cingulate, resulting in a negative correlation coefficient.
The observed positive partial correlation between CSF T-tau and scaled FDG uptake in the caudate was unexpected, because CSF T-tau increases over time in clinical AD [27]. Glucose metabolism in the caudate is generally assumed to be more or less unaffected by AD [28]. However, stable glucose metabolism in the caudate during the course of AD might be the net effect of two competing processes, an age-related decline and a tau pathology-related increase in glucose metabolism. Possible explanations for a tau-related increase in glucose metabolism in the caudate include (1) increased rate of compulsive behaviour and (2) upregulation of implicit memory relative to explicit memory. In relation to explanation (1), compulsive behaviour, which according to molecular psychiatry is associated with tau pathology [29], is observed in about 10% of AD subjects [30]. The link to glucose metabolism in the caudate might be provided by the finding of increased local glucose metabolism in the caudate of subjects with obsessive compulsive disorder, first described by Baxter et al. [31]. In relation to explanation (2), the caudate is thought to be part of the implicit memory system, in which unconscious skills, e.g. motor patterns, are learned, while the medial temporal lobe is part of the explicit memory system, which is used for more conscious memory content, e.g. episodic or verbal. Both memory systems are related and compete with each other [32]. In a functional MRI study a negative correlation was observed between the activity in the caudate and the activity in the medial temporal lobe during a weather prediction task [33]. Klimkowicz-Mrowiec et al. recently assessed explicit and implicit memory in AD patients and controls [34]. Despite the more severely impaired explicit memory, patients with moderate dementia showed significantly better implicit memory performance (weather prediction task) compared to mildly demented patients or normal controls.
There are also a number of technical aspects related to imaging and data analysis which might have affected the correlation analyses in the present study: (a) intensity scaling of FDG uptake images, (b) lack of correction for photon scatter, and (c) nonlinear spatial normalization of atrophic brains in combination with the use of predefined standard VOIs. In relation to point (a), the pons was used as reference region for intensity scaling of the FDG uptake images, since Minoshima et al. have shown that the pons is particularly reliable for this purpose in AD subjects [21]. However, a subtle reduction in pontine glucose metabolism at advanced stages of AD cannot be ruled out [21]. This would cause an AD stage-dependent overestimation of scaled FDG uptake, which might correlate with AD severity with the ‘wrong’ sign. Clarification of this point requires comparison between scaled FDG uptake and CMRGlc, preferably in the same subjects. However, absolute CMRGlc was not available in the present retrospective study, because blood sampling for measurement of the input function was not performed.
In relation to point (b), PET images were not corrected for scattered photons, because (subtraction/convolution-based) scatter correction increases statistical noise and, thus, might reduce statistical power for the detection of AD-related effects. Within the SPM community, scatter correction is not recommended for PET activation studies for this reason [35]. However, omitting scatter correction assumes that the distribution of scatter is not significantly different between subjects with probable AD and controls. More precisely, it assumes that the reduction of local scatter fraction in AD subjects by the reduction of the number of photons scattered in from hypometabolic brain regions can be neglected in all brain regions (including the pons, the reference region). In the present study, PET imaging was performed with an ECAT EXACT 921/47 system in 2-D mode so that the scatter fraction was <20% in all brain regions [20, 36]. From this limit and the mean difference in total scaled tracer uptake between the AD subjects and the controls, scatter-related difference in local scaled tracer uptake between AD subjects and controls is estimated to be <5%. The observed variation of scaled FDG uptake in both the precuneus/posterior cingulate and the caudate that was accounted for by variation of CSF Aβ1-42 and T-tau, respectively, was significantly larger (standardized regression coefficients −0.372 and 0.380, respectively; see Table 2; compare also Fig. 5b,c).
In relation to point (c), although (elastic) stereotactic normalization (i.e. with warping) was used in preparation for voxel-based statistical testing, this might not fully account for the shift in brain structures such as the caudate in the atrophic brain of AD subjects [37]. Atrophy-related imperfection of stereotactic normalization affects not only voxel-based analysis but also the use of a standard VOI atlas in template space. However, systematic errors are expected to be smaller at the level of VOIs than at the voxel level, provided that the anatomical mismatch between the structure of interest and the corresponding standard VOI is small. In the present study, the VOI analysis of the caudate might have been most affected, since atrophy-related anatomical mismatch is largest at ventricular edges [37]. Figure 6 shows the AAL caudate VOI overlaid on the mean scaled FDG uptake in the probable AD subjects and in the controls, respectively. Visual inspection does not reveal gross anatomical mismatch between the caudate and the caudate VOI either in the controls or in the probable AD subjects. The impression that the caudate is slightly shifted in a lateral posterior direction relative to the caudate VOI is mainly due to partial volume effects at the caudate-to-ventricle edge. Furthermore, atrophy-related systematic errors of stereotactic normalization would be expected to cause a negative correlation between CSF T-tau and scaled FDG uptake in the caudate VOI: increased CSF T-tau → increased atrophy → increased atrophy-related errors of stereotactic normalization → increased mismatch of caudate and caudate VOI → reduced scaled FDG uptake in caudate VOI. The observed positive partial correlation between CSF T-tau and scaled FDG uptake in the caudate, therefore, most likely was not secondary to atrophy-related errors in stereotactic normalization.
To our best knowledge, there are only four studies which have investigated the relationship between CSF Aβ and tau with brain activity as measured by FDG PET. Only two of these included subjects with clinical AD. Okamura and colleagues performed FDG PET and CSF analysis in 15 subjects with probable AD [12]. Voxel-by-voxel CMRGlc was computed by the approach of Sokoloff et al. [38]. However, prior to the correlation analyses, local CMRGlc was scaled to the mean CMRGlc in the cerebellum, resulting in ‘metabolic ratios’. The whole-brain metabolic ratio was obtained by averaging the local metabolic ratio over all grey and white matter structures of the brain. This ratio showed a significant positive correlation with CSF Aβ1-42, but it did not correlate with CSF T-tau. Partial correlation analysis with correction for age was performed at the level of VOIs. There was a significant positive correlation between CSF Aβ1-42 and the metabolic ratio in the inferior temporal cortex. Ceravolo et al. recently investigated the relationship between CSF T-tau and P-tau181P and local FDG uptake scaled to the cerebellum in a cohort of 28 subjects with probable AD [15]. Pearson’s correlation analysis revealed a significant negative correlation between both T-tau and P-tau181P and scaled FDG uptake bilaterally in the temporal lobe, the parietal lobe, and the entorhinal/hippocampal region. In addition, T-tau showed a significant negative correlation with scaled FDG uptake in the occipital lobe. There was no significant effect in the frontal lobe nor in the striatum. Fellgiebel et al. examined 16 subjects with mild cognitive impairment and found that the occurrence of at least one cluster of significantly reduced scaled FDG uptake within the typical AD regions was significantly related to elevated CSF P-tau181P levels, but not to elevated T-tau levels [13]. Mosconi et al. performed FDG PET imaging and CSF analysis in 28 middle-aged normal subjects [14]. CMRGlc was obtained voxel-by-voxel using the approach of Sokoloff et al. [38]. Voxel-based correlation analysis revealed a negative correlation between CMRGlc and CSF P-tau231P in the middle occipital gyrus, parahippocampal gyrus, superior frontal gyrus, and the thalamus. There was no significant relationship between CMRGlc and Aβ1-42.
The detection of a positive correlation between CSF Aβ1-42 and the whole-brain metabolic ratio in the study of Okamura et al. [12], of negative correlations between CSF T-tau and P-tau181P and scaled FDG uptake in some brain regions in the study of Ceravolo et al. [15], and of negative correlations between CMRGlc and CSF P-tau231P in some brain regions in the study of Mosconi et al. [14] appears to contradict the present findings (‘sign flip’). Possible explanations include differences between the studies with respect to included subjects, brain regions, and intensity scaling of the PET images. For example, the 34 subjects with probable AD included in the present study covered a particularly wide range of cognitive performance (MMSE score 7 to 28), improving the detectability of longitudinal effects related to disease stage (compared to cross-sectional effects in a group of patients with a more similar stage of the disease). The study by Mosconi et al. [14] examined healthy subjects with and without subjective memory complaints, and, therefore, analysed correlations between CSF markers and FDG PET scans in normal aging or in preclinical stages of AD which might differ from the correlations in clinical AD stages.
The following limitations of the present study should be mentioned. First, the inclusion/exclusion criteria for subjects with probable AD with respect to the FDG PET pattern might have introduced some selection bias, although a standard AD pattern was not required. Exclusion criterion E4 excluded subjects with abnormal FDG PET patterns suggestive of other diseases than AD such as hypometabolism restricted to frontal brain areas, focal hypometabolism, or extended but strictly unilateral hypometabolism. This might have excluded subjects with some (but not all) rare variants of AD. Second, in the control subjects of the present study whole-body FDG PET including the brain had been performed for a non-CNS oncological indication. Seven of the 17 controls had been treated with chemotherapy prior to PET which might have affected FDG uptake [39]. The time period between the last administration of chemotherapy and PET ranged between 1 and 50 weeks. Voxel-based t-test comparison of controls with chemotherapy versus controls without chemotherapy did not reveal a significant effect (p = 0.001, cluster ≥1 ml; results not shown). This might be explained by the fact that chemotherapy tends to affect all brain regions similarly [40] so that potential effects on local FDG uptake cancel to a large extent during intensity scaling. It is worth noting that the analyses of associations between CSF markers and local FDG uptake in the group of probable AD subjects were not directly affected by any limitation of the control group. Finally, the lack of CSF measures, apoE genotype, and MMSE score in the control subjects prevented the computation of sensitivity and specificity of these measures for discrimination of the probable AD subjects and the controls as well as statistical testing of more sophisticated models including both probable AD subjects and controls simultaneously.
In conclusion, the present results indicate a rather strong association between the extent and local severity of the reduction in scaled FDG uptake and cognitive impairment in probable AD subjects. In addition, the results suggest that there is a relationship between scaled FDG uptake and the CSF biomarkers T-tau and Aβ1-42 which varies between brain regions.
References
Silverman DHS. Brain 18F-FDG PET in the diagnosis of neurodegenerative dementias: comparison with perfusion SPECT and with clinical evaluations lacking nuclear imaging. J Nucl Med 2004;45:594–607.
Sunderland T, Linker G, Mirza N, Putnam KT, Friedman DL, Kimmel LH, et al. Decreased beta-amyloid1-42 and increased tau levels in cerebrospinal fluid of patients with Alzheimer disease. JAMA 2003;289:2094–103.
Blennow K, Hampel H. CSF markers for incipient Alzheimer’s disease. Lancet Neurol 2003;2:605–13.
Engelborghs S, De Vreese K, Van de Casteele T, Vanderstichele H, Van Everbroeck B, Cras P, et al. Diagnostic performance of a CSF-biomarker panel in autopsy-confirmed dementia. Neurobiol Aging 2008;29:1143–59.
Strozyk D, Blennow K, White LR, Launer LJ. CSF Abeta 42 levels correlate with amyloid-neuropathology in a population-based autopsy study. Neurology 2003;60:652–6.
Clark CM, Xie S, Chittams J, Ewbank D, Peskind E, Galasko D, et al. Cerebrospinal fluid tau and beta-amyloid: how well do these biomarkers reflect autopsy-confirmed dementia diagnoses? Arch Neurol 2003;60:1696–702.
Fagan AM, Mintun MA, Mach RH, Lee SY, Dence CS, Shah AR, et al. Inverse relation between in vivo amyloid imaging load and cerebrospinal fluid Abeta42 in humans. Ann Neurol 2006;59:512–9.
Kadekaro M, Crane AM, Sokoloff L. Differential effects of electrical stimulation of sciatic nerve on metabolic activity in spinal cord and dorsal root ganglion in the rat. Proc Natl Acad Sci U S A 1985;82:6010–3.
Mielke R, Schroder R, Fink GR, Kessler J, Herholz K, Heiss WD. Regional cerebral glucose metabolism and postmortem pathology in Alzheimer’s disease. Acta Neuropathol 1996;91:174–9.
Buerger K, Zinkowski R, Teipel SJ, Tapiola T, Arai H, Blennow K, et al. Differential diagnosis of Alzheimer disease with cerebrospinal fluid levels of tau protein phosphorylated at threonine 231. Arch Neurol 2002;59:1267–72.
Silverman DH, Small GW, Chang CY, Lu CS, Kung De Aburto MA, Chen W, et al. Positron emission tomography in evaluation of dementia: regional brain metabolism and long-term outcome. JAMA 2001;286:2120–7.
Okamura N, Arai H, Higuchi M, Tashiro M, Matsui T, Itoh M, et al. Cerebrospinal fluid levels of amyloid beta-peptide1-42, but not tau have positive correlation with brain glucose metabolism in humans. Neurosci Lett 1999;273:203–7.
Fellgiebel A, Siessmeier T, Scheurich A, Winterer G, Bartenstein P, Schmidt LG, et al. Association of elevated phospho-tau levels with Alzheimer-typical 18F-fluoro-2-deoxy-D-glucose positron emission tomography findings in patients with mild cognitive impairment. Biol Psychiatry 2004;56:279–83.
Mosconi L, De Santi S, Brys M, Tsui WH, Pirraglia E, Glodzik-Sobanska L, et al. Hypometabolism and altered cerebrospinal fluid markers in normal apolipoprotein E E4 carriers with subjective memory complaints. Biol Psychiatry 2008;63:609–18.
Ceravolo R, Borghetti D, Kiferle L, Tognoni G, Giorgetti A, Neglia D, et al. CSF phosphorylated TAU protein levels correlate with cerebral glucose metabolism assessed with PET in Alzheimer’s disease. Brain Res Bull 2008;76:80–4.
Friston KJ, Holmes AP, Worsley KJ, Poline JP, Frith CD, Frackowiak RSJ. Statistical parametric maps in functional Imaging: a general linear approach. Hum Brain Mapp 1995;2:189–210.
McKhann G, Drachman D, Folstein M, Katzman R, Price D, Stadlan EM. Clinical diagnosis of Alzheimer’s disease: report of the NINCDS-ADRDA Work Group under the auspices of Department of Health and Human Services Task Force on Alzheimer’s Disease. Neurology 1984;34:939–44.
Hoffman JM, Welsh-Bohmer KA, Hanson M, Crain B, Hulette C, Earl N, et al. FDG PET imaging in patients with pathologically verified dementia. J Nucl Med 2000;41:1920–8.
Hixson JE, Vernier DT. Restriction isotyping of human apolipoprotein E by gene amplification and cleavage with HhaI. J Lipid Res 1990;31:545–8.
Wienhard K, Eriksson L, Grootoonk S, Casey M, Pietrzyk U, Heiss WD. Performance evaluation of the positron scanner ECAT EXACT. J Comput Assist Tomogr 1992;16:804–13.
Minoshima S, Frey KA, Foster NL, Kuhl DE. Preserved pontine glucose metabolism in Alzheimer disease: a reference region for functional brain image (PET) analysis. J Comput Assist Tomogr 1995;19:541–7.
Tzourio-Mazoyer N, Landeau B, Papathanassiou D, Crivello F, Etard O, Delcroix N, et al. Automated anatomical labeling of activations in SPM using a macroscopic anatomical parcellation of the MNI MRI single-subject brain. Neuroimage 2002;15:273–89.
Perneger TV. What’s wrong with Bonferroni adjustments. BMJ 1998;316:1236–8.
Herholz K, Salmon E, Perani D, Baron JC, Holthoff V, Frolich L, et al. Discrimination between Alzheimer dementia and controls by automated analysis of multicenter FDG PET. Neuroimage 2002;17:302–16.
Minoshima S, Frey KA, Koeppe RA, Foster NL, Kuhl DE. A diagnostic approach in Alzheimer’s disease using three-dimensional stereotactic surface projections of fluorine-18-FDG PET. J Nucl Med 1995;36:1238–48.
Mielke R, Herholz K, Grond M, Kessler J, Heiss WD. Clinical deterioration in probable Alzheimer’s disease correlates with progressive metabolic impairment of association areas. Dementia 1994;5:36–41.
Bouwman FH, van der Flier WM, Schoonenboom NS, van Elk EJ, Kok A, Rijmen F, et al. Longitudinal changes of CSF biomarkers in memory clinic patients. Neurology 2007;69:1006–11.
Piert M, Koeppe RA, Giordani B, Berent S, Kuhl DE. Diminished glucose transport and phosphorylation in Alzheimer’s disease determined by dynamic FDG-PET. J Nucl Med 1996;37:201–8.
Cummings JL. Toward a molecular neuropsychiatry of neurodegenerative diseases. Ann Neurol 2003;54:147–54.
Mendez MF, Perryman KM, Miller BL, Swartz JR, Cummings JL. Compulsive behaviors as presenting symptoms of frontotemporal dementia. J Geriatr Psychiatry Neurol 1997;10:154–7.
Baxter LR Jr, Phelps ME, Mazziotta JC, Guze BH, Schwartz JM, Selin CE. Local cerebral glucose metabolic rates in obsessive-compulsive disorder. A comparison with rates in unipolar depression and in normal controls. Arch Gen Psychiatry 1987;44:211–8.
Poldrack RA, Packard MG. Competition among multiple memory systems: converging evidence from animal and human brain studies. Neuropsychologia 2003;41:245–51.
Poldrack RA, Clark J, Pare-Blagoev EJ, Shohamy D, Creso Moyano J, Myers C, et al. Interactive memory systems in the human brain. Nature 2001;414:546–50.
Klimkowicz-Mrowiec A, Slowik A, Krzywoszanski L, Herzog-Krzywoszanska R, Szczudlik A. Severity of explicit memory impairment due to Alzheimer’s disease improves effectiveness of implicit learning. J Neurol 2008;255:502–9.
Andersson J. Re: PET scan reconstructions (item 009521 in SPM mail archive). 2002. https://www.jiscmail.ac.uk/cgi-bin/wa.exe?A2=ind02&L=SPM&P=R174130. Accessed 4 Feb 2009.
Hoffman EJ, Cutler PD, Guerrero TM, Digby WM, Mazziotta JC. Assessment of accuracy of PET utilizing a 3-D phantom to simulate the activity distribution of [18F]fluorodeoxyglucose uptake in the human brain. J Cereb Blood Flow Metab 1991;11:A17–25.
Ishii K, Willoch F, Minoshima S, Drzezga A, Ficaro EP, Cross DJ, et al. Statistical brain mapping of 18F-FDG PET in Alzheimer’s disease: validation of anatomic standardization for atrophied brains. J Nucl Med 2001;42:548–57.
Sokoloff L, Reivich M, Kennedy C, Des Rosiers MH, Patlak CS, Pettigrew KD, et al. The [14C]deoxyglucose method for the measurement of local cerebral glucose utilization: theory, procedure, and normal values in the conscious and anesthetized albino rat. J Neurochem 1977;28:897–916.
Okada J, Yoshikawa K, Imazeki K, Uno K, Minoshima S, Itami J, et al. Change of cerebral glucose metabolism by antineoplastic drug. Am J Physiol Imaging 1991;6:162–6.
Phillips PC, Dhawan V, Strother SC, Sidtis JJ, Evans AC, Allen JC, et al. Reduced cerebral glucose metabolism and increased brain capillary permeability following high-dose methotrexate chemotherapy: a positron emission tomographic study. Ann Neurol 1987;21:59–63.
Author information
Authors and Affiliations
Corresponding author
Rights and permissions
About this article
Cite this article
Arlt, S., Brassen, S., Jahn, H. et al. Association between FDG uptake, CSF biomarkers and cognitive performance in patients with probable Alzheimer’s disease. Eur J Nucl Med Mol Imaging 36, 1090–1100 (2009). https://doi.org/10.1007/s00259-009-1063-7
Received:
Accepted:
Published:
Issue Date:
DOI: https://doi.org/10.1007/s00259-009-1063-7