Abstract
Farming practices affect the soil microbial community, which in turn impacts crop growth and crop-weed interactions. This study assessed the modification of soil bacterial community structure by organic or conventional cropping systems, weed species identity [Amaranthus retroflexus L. (redroot pigweed) or Avena fatua L. (wild oat)], and living or sterilized inoculum. Soil from eight paired USDA-certified organic and conventional farms in north-central Montana was used as living or autoclave-sterilized inoculant into steam-pasteurized potting soil, planted with Am. retroflexus or Av. fatua and grown for two consecutive 8-week periods to condition soil nutrients and biota. Subsequently, the V3-V4 regions of the microbial 16S rRNA gene were sequenced by Illumina MiSeq. Treatments clustered significantly, with living or sterilized inoculum being the strongest delineating factor, followed by organic or conventional cropping system, then individual farm. Living inoculum-treated soil had greater species richness and was more diverse than sterile inoculum-treated soil (observed OTUs, Chao, inverse Simpson, Shannon, P < 0.001) and had more discriminant taxa delineating groups (linear discriminant analysis). Living inoculum soil contained more Chloroflexi and Acidobacteria, while the sterile inoculum soil had more Bacteroidetes, Firmicutes, Gemmatimonadetes, and Verrucomicrobia. Organically farmed inoculum-treated soil had greater species richness, more diversity (observed OTUs, Chao, Shannon, P < 0.05), and more discriminant taxa than conventionally farmed inoculum-treated soil. Cyanobacteria were higher in pots growing Am. retroflexus, regardless of inoculum type, for three of the four organic farms. Results highlight the potential of cropping systems and species identity to modify soil bacterial communities, subsequently modifying plant growth and crop-weed competition.
Similar content being viewed by others
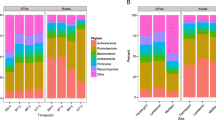
Explore related subjects
Discover the latest articles, news and stories from top researchers in related subjects.Avoid common mistakes on your manuscript.
Introduction
Soil microbial community characteristics are driven by a myriad of factors, including climate, soil physical and chemical traits, the abundance and diversity of plant species living at the site, and the influx of nutrients and minerals by water, animals, or plant detritus [1–4]. In general, an increase in microbial phylogenetic diversity leads to improved soil health, makes the system more resilient to physical or chemical disturbances, modifies plant competition, and increases plant production by facilitating access to nutrients [1, 5–9]. However, on a species-by-species basis, microorganisms can positively or negatively affect plant growth and health through various pathways (reviewed in [10]). Additionally, the interactions between soil microbiota and nutrients are complex, and increased microbial phylogenic diversity does not always lead to increased microbial functionality (reviewed in [11, 12]). Thus, more investigation into the dynamics between microorganisms, soil nutrients, and plants is needed to understand how these interactions shape microbial diversity and soil quality.
Although it is difficult to generalize the impact that farming/cropping systems have on soil biology, the following are common differences between conventional and organic crop management in general, and applicable to dryland farms in Montana. In organic farming, mechanical practices, such as tillage, are commonly used to control weeds and terminate cover crops, and have been associated with a decrease in microbial biomass [13] and diversity [14]. However, organic farms often have increased weed diversity [15–17], and higher plant diversity has been linked with increased microbial biomass [18], respiration, and fungal abundance [2]. In conventional farming, agriculture intensification with fertilizers and herbicides has a negative impact on meso- and macrobiota in the short term and microbiota over time [19]. Crop rotations also differ between systems, with the organic farms typically having diverse rotations and using cover crops, green manure crops, or animal manures as source of nutrients, while conventional farms typically grow only one or two crops and rely on synthetic fertilizers as a nutrient source. Previous studies have demonstrated that diverse crop rotations increase the diversity of soil biota [14]. Finally, organic versus conventional changes in soil microbiota differ with landscape complexity [20].
Plant growth can have a species-specific impact on soil microorganisms [21–23]. For example, a previous study showed that plant growth increased the density of microorganisms, especially pseudomonad bacteria, over unplanted soil [4]. Microbial density was most increased with clover (Trifolium repens) and ryegrass (Lolium perenne) over bentgrass (Agrostis capillaris) or wheat (Triticum aestivum) [4]. Likewise, canola (Brassica napus) and wheat selected for different bacterial communities, with wheat-associated soil having lower overall diversity yet more abundant Bacillus in the rhizoplane, and Cartobacter, Rathayibacter, Streptococcus, and other genera more abundant in canola roots [24].
A number of studies compared soil quality and microbial phylogenetic or functional diversity between ecosystems, including different farming/cropping systems [25–28]; however, very little work had been done using soil from these ecosystems to condition other soil as an inoculum. Most studies of this nature involve the addition of a single microorganism to soil [25–27], instead of using the entire soil microbiota [28]. The present study sought to further investigate the interactions between cropping systems, weed species, soil inoculum, and conditioned soil bacterial community structure. This was done by comparing bacterial 16S ribosomal RNA (rRNA) gene populations in potting soil that had received either a living or a sterilized soil inoculum taken from organically or conventionally farmed soil and had the problematic weed species Amaranthus retroflexus L. (redroot pigweed) or Avena fatua L. (wild oat) growing in it.
Methods
Sample Collection
Soil was collected on July 17–18, 2013, from eight paired USDA-certified organic and conventional farms. Each farm pair was located approximately 3.3 km (SD = 4.5) apart, with pairs located across north-central Montana (mean distance between pairs 137 km, SD = 40). Pairs included Big Sandy (BSa) organic (48.068895 N 110.011836 W) and conventional (48.066465 N 110.012038 W), Dutton (Dut) organic (47.778568 N 111.578379 W) and conventional (47.772951 N 111.565377 W), Havre (Hav) organic (48.850477 N 109.971366 W) and conventional (48.826182 N 110.076707 W), and Lewistown (Lew) organic (47.114102 N 109.715622 W) and conventional (47.118478 N 109.699570 W). Conventional farms practiced a no-tillage wheat-fallow rotation, and organic farms had been managed with intensive tillage and diverse crop rotations.
Fields for soil sampling were not randomly selected due to logistical constraints, such as the pairing of farms by cropping system, for fields to be planted with a wheat crop [except the organic Dutton farm planted with Triticum turanicum Jakubz. (Khorasan wheat var. Kamut), a close wheat relative], and obtaining permission from landowners. Sampled fields represented a range of soil characteristics (loams, clay loams, and silty clay loams), temperatures (mean max of 12.9–14.8 °C; mean min (−2.7 to −0.9 °C), and annual precipitation (265–388 mm) (see Table S1 [29]).
Within each field, soil was collected to a depth of 13 cm using 6-cm-diameter cores every 10 m along a 200-m W-shaped transect, for a total of 21 subsamples/transect. Transects were located at least 100 m from any field edge and positioned on relatively uniform slopes. Subsamples were pooled by field and refrigerated at 4 °C until the experiment began. Bulk soil samples from each field were sent for nutrient testing to Agvise Laboratories (Northwood, ND) (see Table S1 [29]). First, the soils were air-dried for 2 weeks and sifted through a 1-cm2 sieve. Half the soil was autoclave-sterilized to 120 °C at 15 psi for two 1-h periods with 24 h between sterilizations (sterilized, hereafter), and half was left unsterilized (living, hereafter).
Experimental Design
The greenhouse experiment was conducted at the Plant Growth Center at Montana State University, Bozeman, MT. The potting media included a steam pasteurized (70 °C for 4 h) soil mix of equal parts by volume loam soil, washed concrete sand, and Canadian sphagnum peat moss, along with AquaGro 2000 G wetting agent blended at 0.59 kg m−3. Pots were 2.5 L volume: 18 cm diameter × 14 cm height, and were filled with the potting mix. Pots were then inoculated with a small amount of soil (80 mL, approximately 3.5 % by volume), of either the biologically active (living) or autoclave-sterilized soil inoculum collected from a single farm location.
Two consecutive 8-week growing trials were conducted in two separate greenhouses, with a 2-week period in between. Soil samples (10 cm depth × 1 cm diameter) were collected at the end of the second growing period and analyzed for microbial communities. Pots were kept under a 16-h photoperiod of natural sunlight, supplemented with mercury vapor lamps (165 μE m−2 s−1) at 22 °C/18 C day/night, and arranged using a randomized block design, with three replicated blocks per greenhouse. Pots were seeded with either an average of 60 Am. retroflexus L. (redroot pigweed) (AMARE) or 12 Av. fatua L. (wild oat) (AVEFA), which were selected for their contrasting life history attributes (i.e., dicot vs. monocot), and their importance as agricultural weeds in the Northern Great Plains Region of North America. Prior to planting, seeds were surface-sterilized by soaking in 70 % ethanol for 2 min, then a 5 % bleach solution for 1 min, then rinsed 5 times with sterile water. Plants were thinned 10 days after emergence to either 10 or 6 evenly spaced individuals, respectively. Pots were watered as needed and were not fertilized. Further details of the soil collection and greenhouse study (i.e., plant biomass, nutrient content) can be found in Johnson et al. [29], specifically Table S1. Organic soil contained more organic matter than conventional soil (P < 0.05) but did not significantly differ in nitrogen content [29].
DNA Extraction and Sequencing
Nucleic acids were extracted from 1 g of soil using the PowerSoil 96-well Soil DNA Isolation Kit (MoBio Laboratories, Inc., Carlsbad, CA) following kit instructions specific to “centrifugation exclusively.” PCR was performed to amplify the V3-V4 region of the 16S rRNA gene using the KAPA HotStart PCR Kit (Kapa Biosystems, Wilmington, MA): 10 μL Kappa HotStart Mastermix, 6 μL molecular-grade water, 1 μL of each forward and reverse primer (10 mM concentration), and 2 μL sample DNA. Primers included the MiSeq adaptors (A for forward, B for reverse), the sample index/barcodes, the two-nucleotide linker, and the primers 341F (5′-CCTACGGGAGGCAGCAG-3′) [30] and 806R (5′-GGACTACHVGGGTWTCTAAT-3′) [31] which target the prokaryotic (bacterial and archaeal) 16S rRNA gene. The HotStart PCR protocol was as follows: 95 °C for 3 min; 5 cycles of denaturation at 98 °C for 20 s, annealing at 52 °C for 30 s, elongation at 72 °C for 45 s; 25 cycles of denaturation at 98 °C for 20 s, annealing at 60 °C for 30 s, elongation at 72 °C for 45 s; followed by storage at 4 °C. PhiX was used as a positive control at a 10 % spike-in, and molecular-grade sterilized water was used as a negative control.
PCR amplicon concentrations were checked on a TapeStation Bioanalyzer (Agilent Technologies, Santa Clara, CA) using the D1000 Screentapes with 3 μL of D1000 buffer and 1 μL of PCR product in each tube. Samples were diluted with molecular water and pooled at 2 ng of DNA per sample. Isopropanol was added at 10 % of the pool volume, then briefly vortexed and placed on a vacuum centrifuge at 45 °C until all liquid had evaporated. The concentrated pool was resuspended in 150 μL of molecular water and pipetted gently up and down to mix. The pooled PCR product was run on a 1.5 % agarose gel at 100 V, 200 mA for 30 min, and the amplicon band was excised from the gel and purified using the QIAquick gel extraction kit (QIAGEN, Valencia, CA) according to kit instructions. High-throughput sequencing was performed using an Illumina MiSeq (Illumina, San Diego, CA) and a 500-cycle V2 kit. Output data included demultiplexed fastq files, which had had the primer and index sequences removed. Sequencing data can be found in the Sequence Read Archive (SRA) at NCBI under BioProject301630.
DNA Analysis
Paired-end 16S rRNA sequences for each sample were assembled into contiguous segments, using a simple Bayesian algorithm with PANDAseq [32], which met default PANDAseq quality parameters along the overlap. First, sequences were trimmed after any homopolymer >8 b long using an in-house script, as homopolymer length is not directly tied to whole sequence error rate in Illumina MiSeq data [33]. Mothur ver. 1.35 [34, 35] was then used to cull sequences which were <250 or >580 b, or which contained any ambiguous bases (N). Sequences were aligned to the Silva v119 reference database, which contains bacterial and archaeal 16S rRNA sequences, using the Wang algorithm [36]. Samples containing >15,000 sequences were randomly subsampled down to 15,000 per sample for easier computing. Chimeras were identified and removed using the mothur-integrated version of UCHIME [37], and sequences were preclustered to consolidate sequences with <2 base differences [38]. Sequences were classified by the mothur-implemented RDP classifier using the Silva v119 taxonomy database at 80 % confidence cutoff, and any that would not classify to phylum were removed. Archaeal diversity was very low, either from low abundance in greenhouse pots or sequencing bias, and so sequences were removed from further analysis. Pairwise comparisons of taxonomic diversity were made using a Student’s t test.
Sequences were grouped by sampling location, and then subsampled to 5000 reads per group for statistical analysis, which removed six groups containing fewer than the cutoff. Distance was calculated and sequences were clustered into operational taxonomic units (OTUs) based on a 3 % genetic cutoff using the nearest-neighbor algorithm. The diversity measures, Chao [39], Jackknife [40], inverse Simpson [41], Good’s coverage [42], Shannon-Weiner diversity [43], analysis of molecular variance (AMOVA), analysis of similarity (ANOSIM) [44], and UniFrac [45] values are presented as group mean. Pairwise comparisons of diversity metrics Chao, inverse Simpson, Good’s coverage, and Shannon-Weiner were made using a Student’s t test. UniFrac unweighted compares tree structure between samples, while weighted also takes into account sequence abundance. Linear discriminant analysis (LDA) was run to detect discriminatory OTUs, using the Wilcoxon rank test to determine significance. A nonmetric multidimensional scaling (NMDS) plot was created in the statistical package R [46] using a mothur-generated NMDS calculation of Bray-Curtis dissimilarity phylogenetic distance calculations to display clustering using Euclidean distance [47]. OTU abundance from mothur was imported into PRIMER ver. 6 for analysis, normalized to relative abundance and square-root-transformed, then analyzed using PERMANOVA to assess which treatment contributed to data variation, ANOSIM, and metric multidimensional scaling (MDS) plot with vectors representing multiple regressions to show correlation coefficients using PERMANOVA, the length of which shows the strength of the correlation.
Predicted metagenomics were performed for bacteria and archaea based on previously described metagenomes using the PICRUSt [48] online version, available on the online Galaxy platform (https://huttenhower.sph.harvard.edu/galaxy/). A biom file was generated in mothur with a Greengenes core set reference database for 16S rRNA, then uploaded to Galaxy. The BIOM file was then normalized based on 16S rRNA copy number prior to metagenome prediction and subsequently categorized by function-based family-level taxonomy using the Kyoto Encyclopedia of Genes and Genomes (KEGG) pathways in Galaxy online. Statistical analysis and plot generation of the resulting file was performed with STAMP v2.0.9 [49]. Pairwise comparison of the KEGG pathways was performed applying a Welch’s (two-tailed) t test with 95 % confidence intervals, and values were considered significant at P < 0.05.
Results
A total of 9,609,169 contigs were assembled using PANDAseq (2531–579,315 per sample). After trimming for quality and removing sequences that did not align, a total of 9,587,618 remained (2519–577,843 per sample). These remaining sequences were then quality trimmed for chimeras (7.1 % of total sequences) and taxonomically unresolvable sequences (0.008 % of total sequences), leaving a total of 2,521,528 sequences (2416–14,679 per sample).
Taxonomic Classification
The relative abundance of bacteria at the phylum level varied as a function of geographic location and cropping system, as well as type of inoculum and weed species identity (Fig. 1). Archaeal sequences represented <0.001 % of total abundance and were not included in the taxonomic analysis (Supplementary Table 1). Only 17 samples contained archaea, 14 of which were living and 3 of which were sterile. Sixteen contained the phylum Euryarchaeota, and one contained Thaumarchaeota (BsaOr_Pig_Lv). Broadly speaking, bacteria belonging to the phylum Proteobacteria was most common across all samples, represented in 35–50 % of sequences in all samples, followed by Actinobacteria with 11–24 %.
Relative abundance of soil bacteria at the phylum level across soil samples that were inoculated with either a living or sterile inoculum collected from either organic or conventional farms and was conditioned by either Amaranthus retroflexus L. (redroot pigweed) or Avena fatua L. (wild oat). Abbreviations are as follows: AVEFA, Av. fatua; AMARE, Am. retroflexus; Lv living inoculum, St sterilized inoculum
Soil that received a sterilized inoculum differed from soil with living inoculum (Fig. 1, Table 1). Samples that received a living inoculum had different (P < 0.05) amounts of Acidobacteria, Chloroflexi, and unclassified bacteria, while samples inoculated with a sterile inoculum samples had more Bacteroidetes, Firmicutes, Gemmatimonadetes, and Verrucomicrobia. Specifically, Acidobacteria was twice as common in soil given a living inoculum (mean 7.2 % of sequences) than sterile inoculum (mean 3.8 % of sequences), and Verrucomicrobia were twice as abundant in soil inoculated with sterile inoculum (mean 3.9 % of sequences) than living inoculum (mean 1.5 % of sequences), regardless of the farm location or cropping system.
Several weed-specific trends were seen with respect to microbial phylogenetic diversity (Fig. 1, Table 1). Cyanobacteria was more abundant in soil growing Am. retroflexus for Lewistown organic farms for pots inoculated with either living or sterilized inoculum (11.6 and 9.6 % of sequences, respectively), as well as Dutton organic inoculated with sterilized inoculum (10.5 % of sequences), and Havre organic inoculated with living or sterile inoculum (9.8 and 8.9 % of sequences, respectively), than other weed and inoculum combinations (ranging from 1 to 7.7 % of sequences). Notably, Big Sandy organic and conventional farms had larger percentages of Firmicutes (19.7 and 22.6 % of sequences) for soil that had received a sterilized inoculum and grown Av. fatua than any other inoculum or weed species combination (range 2.3–6.9 % of sequences).
At a greater taxonomic resolution, a total of 854 genera or candidate genera were identified (Supplementary Table 2). Between 33 and 58 % of sequences were “unclassified” at the genus level. The most abundant genus identified was Arthrobacter (up to 13 % abundance), followed by (<10 % of sequences each) Adhaeribacter, Altererythrobacter, Bacillus, Blastocatella, Blastococcus, Brevundimonas, Croceicoccus, Devosia, Gemmatimonas, Iamia, Microcoleus, Nocardioides, Ohtaekwangia, Pseudolabrys, and Sphingomonas.
Free-living (Arthrobacter, Bacillus, Beijerinckia, Bradyrhizobium, Azospirillium, Clostridium, Corynebacterium, Flavobacterium, Nostoc, and Pseudomonas spp.) and symbiotic (Rhizobacter, Rhizobium, and Rhizomicrobium spp.) nitrogen-fixing species made up an average of 3.6–19.3 % of sequences that could be classified down to the genus level (Fig. 2). Organic and conventional farms had similar relative abundances of nitrogen fixers (P > 0.05). Samples that received a sterile inoculum had a higher relative abundance of nitrogen fixers than those receiving living inoculum (P < 0.05). Pots cultivated with Av. fatua had higher relative abundances of nitrogen fixers than those cultivated with Am. retroflexus (P < 0.05), regardless of receiving a living or sterile inoculum. Nitrogen fixers had higher (P < 0.05) relative abundances in pots that received organic sterile as compared to organic living inoculums, and in conventional sterile as compared to conventional living inoculums.
Diversity of nitrogen-fixing genera identified across soil samples that were inoculated with either a living or sterile soil collected from either organic or conventional farms and conditioned by either Amaranthus retroflexus L. (redroot pigweed) or Avena fatua L. (wild oat). Abbreviations are as follows: AVEFA, Av. fatua; AMARE, Am. retroflexus. Statistical significance (P < 0.05) using Student’s t test is designated by an asterisk
Pots that received living inoculum samples shared 30 % (132,839) of sequences in 10 OTUs: Pseudolabrys, Erythrobacteraceae, Geodermatophilaceae, Hyphomicrobiaceae, Microbacteriaceae, Micrococcaceae, Bacillales, and Sphingomonadales (3 OTUs). Organic soil samples shared 24.7 % (109,154) sequences in two OTUs that were classified as Micrococcaceae and Sphingomonadales. Dutton farms shared one Micrococcaceae OTU, and Lewistown farms shared one Sphingomonadales OTU. A linear discriminant analysis indicated that there were 178 significant discriminatory OTUs between soil collected in pots that received living or sterilized inoculums (1359 total OTUs) (Table 2), and 37 discriminatory OTUs between organic versus conventional farms (313 total OTUs) (Fig. 3). There were only 11 significant discriminatory OTUs (356 total OTUs) between farm locations; however, these came from only two sites: Lewiston: Brevundimonas (LDA = 2.705) and Pontibacter (LDA = 2.663), and Havre: Micrococcaceae (LDA = 3.813), Chloroflexi (LDA = 3.133 and 3.011), Rhizobiales (LDA = 3.046), Rhodoplanes (LDA = 2.995), Anaerolineaceae (LDA = 2.990), Solirubrobacterales (LDA = 2.902), Modestobacter (LDA = 2.679), and Anaerolineaceae (LDA = 2.653). There were no discriminatory OTUs when comparing between pots where either Av. fatua or Am. retroflexus grew.
Linear discriminant analysis of discriminatory OTUs between pots that received soil inoculums collected from either organic versus conventional farms, across soil samples inoculated with either living or sterile inoculum and conditioned by either Amaranthus retroflexus L. (redroot pigweed) or Avena fatua L. (wild oat). LDA detects discriminatory OTUs, and values shown are significant (P < 0.05) using the Wilcoxon rank test
Diversity Indices
AMOVA results indicated that samples had differing phylodiversity when comparing samples that received inoculum from living versus sterilized, organic versus conventional farms, and across farm locations (P < 0.001 for each comparison), by both weighted and unweighted UniFrac (Table 1). ANOSIM results indicated that samples were separated using OTU abundance when comparing pooled living versus sterilized inoculum (R = 0.596, P = 0.01) and inoculum type within organic versus conventional farms (R = 0.769, P = 0.01) (Table 1). Av. fatua and Am. retroflexus comparisons were only significant using UniFrac, and not with AMOVA or ANOSIM (Table 1). A total of 137 multivariate comparisons using phylogenetic distance were made when analyzing each sample separately, for which 107 were significant using AMOVA and 108 using ANOSIM (data not shown).
Soil inoculated with living inoculum consistently had higher levels of diversity over samples receiving a sterile inoculum (Table 3, individual results are presented in Supplementary Table 3). Pairwise group comparisons indicated that soil inoculated from organic farms had higher Shannon diversity over that inoculated with soil from conventional farms (P < 0.05), though inverse Simpson was not different (Table 3). Neither samples inoculated with organic nor conventional soil had different total numbers of sequences, although the unique (nonredundant) sequences were higher in either all living or all organic samples, showing that increased diversity measures were not reflective of higher sequencing output in certain groups (Table 3). No difference was seen between pots where Av. fatua or Am. retroflexus grew. When comparing overall diversity indices using ANOSIM, samples were not well separated overall (R < 0.25, P = 0.01), though with some separation of cropping systems by inoculum type (R = 0.295, P = 0.01), or inoculum by cropping system (R = 0.369, P = 0.01). For treatment group comparisons (ex. CvPigSt vs. OrPigSt), unweighted UniFrac values ranged from 0.951 to 0.998 and were significant (P < 0.05) with the exception of Av. fatua versus Am. retroflexus for conventional living, conventional sterile, or organic living, as well as conventional versus organic for Am. retroflexus living. Weighted UniFrac values ranged from 0.631 to 0.988 and were all significant (P < 0.001).
PERMANOVA comparisons indicated that when considering OTU presence and abundance, farm location, inoculum type, cropping systems, and weed species, all had an influence, as did the interactions between farm location/cropping system, farm location/inoculum, and cropping system/inoculum (Table 4). When considering differences in the diversity indices, only inoculum and cropping systems had a significant influence, as did the interactions between farm location/cropping system and inoculum/cropping system. Samples clustered by inoculum type and cropping system on an nMDS plot using phylogenetic distance (Fig. 4). Samples that received a sterile inoculum separated by cropping system, and were more influenced by farm location, than samples that received a living inoculum. When comparing only the living samples, samples clustered by cropping system (Fig. 5a) and by farm location (Fig. 5b), though not by weed species (data not shown), using an MDS plot of OTU abundance.
Nonmetric multidimensional scaling (nMDS) using Bray-Curtis dissimilarity calculations of Euclidean phylogenetic distance, across soil samples inoculated with either living or sterile inoculum collected from either organic and conventional farms and conditioned by either Amaranthus retroflexus L. (redroot pigweed) or Avena fatua L. (wild oat). Graph is colored by inoculum type: living = green, sterile = purple; cropping system: organic = filled in, conventional = hollow; and shapes designate farm locations. No significant clustering by weed species was seen; therefore, samples are not colored by weed
Nonmetric multidimensional scaling (nMDS) using Bray-Curtis dissimilarity calculations of OTU abundance, across soil samples inoculated with living inoculum collected from either organic and conventional farms and conditioned by either Amaranthus retroflexus L. (redroot pigweed) or Avena fatua L. (wild oat). Vectors calculated by multiple regression analysis using PERMANOVA show the direction and strength of Pearson’s correlations by distance to the equilibrium circle, vectors closer to the circle radius are considered important for that axis. Correlations for axis 1/axis 2 are as follows: farm, 0.216/−0.239; cropping system, −0.05/−0.251; and weed species, 0.104/0.097. Graph is colored by cropping system (a) or farm location (b)
Correlation Between Bacterial Taxa and Plant Growth
Significant Pearson’s correlations between wheat biomass, weed biomass, and the most abundant bacterial taxa are shown in Fig. 6. Living inoculum or organic cropping systems were positively correlated with a large number of taxa, while sterile inoculum or conventional cropping systems were negatively correlated with a large number of taxa. AVEFA biomass was positively correlated with the order Rhizobiales; the families Rhodobacteriaceae and Comamonadaceae; and the genera Ralstonia, Azohydromonas, Hirschia, and Arenimonas. AMARE biomass was positively correlated with the phylum Proteobacteria; the orders Sphingomonadales, Myxococcales, and Rhizobiales; the families Geodermatophilaceae, Micromonasporaceae, Phyllobacteriaceae, Xanthomonadaceae, Comamonadaceae, and Anaerolineaceae; and the genera Ohtaekwangia, Croceicoccus, Brevundimonas, Rhizomicrobium, Hirschia, and Fictobacillus. Winter wheat biomass was positively correlated with the phylum Proteobacteria; the orders Bacillales, Rhizobiales, Burkholderiales, and Myxococcales; the families Geodermatophilaceae, Micromonasporaceae, Microbacteriaceae, Bacillaceae, Hyphomicrobiaceae, Anaerolineaceae, and Comamonadaceae; and the genera Ralstonia, Caenimonas, Ohtaekwangia, Croceicoccus, Brevundimonas, Rhizomicrobium, Hirschia, and Fictobacillus. It was slightly negatively correlated with the order Sphingomonadales, the family Pseudomonadaceae, and the genus Adhaeribacter.
Predicted Metagenomics
Pooled organic or conventional samples had a number of slight differences in predicted functionality, with the largest differences in mean proportions shown in Supplemental Fig. 1. Pooled organic samples showed a predicted increase in the numbers of fatty and volatile fatty acid degradation genes, as well as DNA proteins and transcription factors. Pooled living or sterile sample did not show a large difference in predicted functionality, with only a few genes for DNA replication, ribosomal biogenesis, ANC transporters, and aminobenzoate degradation predicted to be more abundant in living samples (Supplemental Fig. 2). Av. fatua samples were predicted to have slightly more abundant phenylalanine, tyrosine, and tryptophan biosynthetic genes, as well as DNA replication and repair proteins and translation factors (Supplemental Fig. 3).
Discussion
There is growing interest in manipulating farm management practices to enhance soil biological characteristics in ways that positively impact agroecosystem health and services [5, 50]. However, while progress has been made in determining the role of soil biota in weed invasiveness [51], little is known about the impacts of increased cropping system diversification on soil biota effects and crop-weed interactions.
Living Versus Sterilized Soil
Inoculum type (living or sterile) was the largest driving factor determining the resulting soil microbial communities. In Johnson et al. [29], wheat biomass production was enhanced in pots that were inoculated with living soils, highlighting the importance of soil biota in determining plant growth. This is consistent with a previous study on reduced microbial diversity and functionality following a biota-terminating chemical disturbance [8]. Samples that received living inoculums all clustered separately from those that received sterilized soil. Interestingly, living inoculum samples did not cluster well with respect to farm location or cropping systems; however, the sterile samples did show separation between organic and conventionally farmed soil. This would indicate that heat-tolerant or endospore-forming bacteria survived the autoclaving process [52], for example Clostridium or Bacillus spp. [53]. In the present study, Bacillus spp. were higher in soil which received sterilized inoculum than living soil inoculum for either cropping system, though not present in relatively high abundance, and while Clostridium spp. were found, they were equally low in abundance in all samples. Thus, even after sterilization, surviving bacteria or nonliving biological differences in organically farmed soil were able to influence bacterial diversity weeks later. For example, though microorganisms would have been killed by the autoclaving process, residual DNA [54, 55] may have been available for use by colonizing soil bacteria to create a new community in pots. This may explain the increase in nitrogen-fixing species in pots that received sterile inoculum, as there would have been low microbial competition and plenty of nutrients (ex. nucleic acids, metals) for nitrogen cycling [56, 57]. Additionally, pots would have been exposed to and potentially colonized by bacteria in the greenhouse air, water, and environment. However, all pots would have been colonized equally as all received similar treatments after receiving inoculum, and pot placement in the greenhouse was randomized.
In the present study, the phylum Verrucomicrobia had twice the relative abundance in soil that received sterile inoculum than living inoculum, regardless of the farm location or cropping system. Previously, an abundance of Verrucomicrobia was associated with preagricultural native tallgrass prairie in the Midwestern USA, which drove carbon dynamics in the soil [58]. Verrucomicrobia is fairly ubiquitous in soils and has been found in higher relative abundance in grassland and prairie as compared to other soil ecosystems across the globe [59]. Verrucomicrobia have also previously been shown to contain the dnaE2 gene, which is commonly found in terrestrial bacteria and thought to promote better fitness in soil environments, including an increase in GC content, increased horizontal gene transfer, and genome size expansion as compared to aquatic bacteria [60].
Likewise, nitrogen fixers had higher relative abundances in samples from the organic sterile treatment as compared to organic living one, and in conventional sterile as compared to conventional living. Again, this was primarily due to Arthrobacter spp. having greater relative abundance in organic sterile and conventional sterile soils, and Bacillus spp. being twice as abundant in conventional sterilized soil than other samples. It is possible that nitrogen-fixing bacteria were selected for by plants in potted soil that had received sterilized soil [61], possibly to induce a regeneration of bacterial diversity, or simply because without competition from other bacteria, nitrogen fixers represented a larger proportion of diversity in pots with sterilized soil. In the complementary study on plant response, living inoculum soil did contain more nitrogen than sterilized soil before the weed conditioning phase, and after the weed conditioning, pots that had received a living inoculum had 1.73 times more nitrogen than pots with the sterile inoculum [29]. This confirms a reduced microbial functionality in sterilized soil in the present study.
In other previous studies, different management practices between cropping systems were selected for different microbial communities [2, 13, 14, 18–20]; thus, it is also likely that 18 weeks under greenhouse settings was enough for recolonization of sterilized soil and to select for certain microbial species.
Cropping System
Previous studies indicated that organic soil enriched for the bacteria Cytophaga, Pontibacter, Flavisolibacter (phylum Bacteroidetes), Bacillus, Ureibacillus, and Paenibacillus (phylum Firmicutes), while conventional soil enriched for the phyla Proteobacteria and Acidobacteria (mainly candidatus Solibacter) [3]. In the present study, organically farmed living inoculum resulted in higher microbial diversity, although not in any of those genera. In the complementary study, soil biota effects were more pronouncedly positive when the living inoculum was collected from organic farms than from conventional farms, possibly due to the increased organic matter present [29]. Previous research suggests that cropping systems modify the relative abundance of mutualistic and pathogenic organisms [62]. One caveat of this study is that only bacterial DNA was sequenced and positive feedbacks on plants are often influenced by microbial mutualists like arbuscular mycorrhizal and ectomycorrhizal fungi, in addition to symbiotic nitrogen fixers [63, 64]. Furthermore, one recent study suggests that plant extracellular DNA in soil is responsible for negative feedbacks [65], indicating that continuous monocultures may have an autotoxicity effect from the same plant litter (reviewed in [66]).
Soils that received an organic inoculum showed increased predicted functionality, though the factor of increase was not large. This may be due to the similar taxonomic classification of samples at the family level, which is used by PiCRUST to predict the presence and density of various genes based on the sequenced genomes of related ecosystems. Functional diversity is not interpreted at the genus level, and it may be that a study of transcriptomics in these samples would illuminate a clear and significant difference in actual functionality between different treatments. Interestingly, samples treated with organic inoculums had more functional genes for degrading benzoate (aromatic carboxylic acid), limonene (a hydrocarbon cyclic terpene), and pinene (a monoterpene) than samples which received soil from a conventional farm. This suggests that these samples from organic farms indeed exhibit a greater resiliency for chemical disturbance, which is likely a function of increased bacterial diversity. In the complementary study on plant response, cropping system had no effect on soil nitrogen content from bulk field soil, although organic matter was higher in organic fields [29].
Farm Location and Weed Species
Geographic location, a proxy for regional differences in soil microbial communities associated with soil physicochemical and/or site environmental conditions (i.e., soil geochemistry, pH, temperature, moisture), separated the Dutton organic and conventional farms from the farms sampled at the other three locations. However, when Dutton farm comparisons were removed, multivariate comparisons were not significantly changed (data not shown) indicating a low relative importance of geographic location in determining microbial communities characteristics in the current study. Thus, it is likely that farm management practices, regardless of the type of cropping systems used, were strong selective forces, which has previously been shown [67].
There were some location-specific trends. For example, Big Sandy organic and conventional farms had much larger percentages of Firmicutes for soil that had been given a sterilized inoculum and grown Av. fatua. Previously, Av. fatua was shown to increase Firmicutes over bulk soil [21, 68], especially at the root tip [21], where it may be promoting fungal mycorrhizal formation (reviewed in [69]). Am. retroflexus is another common weed which prevents germination and yield of many agricultural crops [70], and while not poisonous, its ability to concentrate nitrates can render it toxic to livestock. Am. retroflexus is known to produce large amounts of oxalate. In the present study, Cyanobacteria were more abundant in soil growing Am. retroflexus for three out of four organic farms. Cyanobacteria produce and use oxalate in glyoxylate reactions which ends in carbon dioxide production [71]; thus, it is possible that Cyanobacteria were being selected for. In addition, the free-living nitrogen-fixing Nostoc spp. were more than three times more abundant in Am. retroflexus than in Av. fatua samples, though it is unclear whether Nostoc spp. have previously been shown to use oxalate as a carbon source. However, Am. retroflexus is also high in iron, which is required by Nostoc spp. to prevent iron starvation-induced oxidative stress [72].
Other free-living (Arthrobacter, Bacillus, Beijerinckia, Bradyrhizobium, Azospirillium, Clostridium, Corynebacterium, Flavobacterium, Nostoc, and Pseudomonas spp.) and symbiotic (Rhizobacter, Rhizobium, and Rhizomicrobium spp.) nitrogen-fixing species represented up to 19.3 % of sequences in a sample at the genus level. Specifically, pots that had been given sterilized soil had a higher abundance of Arthrobacter spp., regardless of cropping system. Pots cultivated with Av. fatua had more nitrogen fixers than those cultivated with Am. retroflexus, regardless of receiving a living or sterile inoculum. This was largely attributable to Arthrobacter spp. (phylum Actinobacteria), which were twice as abundant in Av. fatua samples. In the complementary study on plant response, pots with living inoculum and Am. retroflexus had 1.58 times more nitrogen content that those conditioned with Av. fatua, though there was no difference in the sterile samples [29]. Thus, conditioning with Av. fatua consistently selected for nitrogen-fixing species diversity, even though it did not appear to be improving nitrogen-fixing functionality in the soil. This may indicate that more soil nitrogen was being taken up by Av. fatua.
Actinobacteria were previously enriched in rhizosphere-associated soil along Av. fatua root systems, particularly at root tips [21], and especially in the order Actinomycetales, are known for producing antimicrobial and insecticidal compounds. Av. fatua are known to release several phenolic exudates from roots into the soil, including syringic acid, vanillin, 4-hydroxybenzoic acid, syringaldehyde, ferulic acid, p-coumaric acid, and vanillic acid [73, 74]. It is thought that these phenolic compounds act as signaling agents between plants and soil bacteria [75], and nitrogen-fixing bacteria, including Arthrobacter, have been shown to use these and other phenolic compounds during intermediate electron transfers under low-oxygen conditions [76, 77]. In the present study, soil gas was not measured.
References
Schnitzer SA, Klironomos JN, HilleRisLambers J et al (2011) Soil microbes drive the classic plant diversity–productivity pattern. Ecology 92:296–303. doi:10.1890/10-0773.1
Zak DR, Homes WE, White DC et al (2003) Plant diversity, soil microbial communities, and ecosystem function: are there any links? Ecology 84:2042–2050
Hartmann M, Frey B, Mayer J et al (2015) Distinct soil microbial diversity under long-term organic and conventional farming. ISME J 9:1177–1194. doi:10.1038/ismej.2014.210
Grayston SJ, Wang SQ, Campbell CD, Edwards AC (1998) Selective influence of plant species on microbial diversity in the rhizosphere. Soil Biol Biochem 30:369–378. doi:10.1016/s0038-0717(97)00124-7
Brussaard L, de Ruiter PC, Brown GG (2007) Soil biodiversity for agricultural sustainability. Agric Ecosyst Environ 121:233–244. doi:10.1016/j.agee.2006.12.013
Schmidt TM, Waldron C (2015) Microbial diversity in soils of agricultural landscapes and its relation to ecosystem function. In: Hamilton SK, Doll JE, Roberston GP (eds) The ecology of agricultural landscapes: long-term research on the path to sustainability. Oxford University Press, New York, pp 135–157
Griffiths BS, Ritz K, Bardgett RD et al (2000) Ecosystem response of pasture soil communities to fumigation-induced microbial diversity reductions: an examination of the biodiversity-ecosystem function relationship. Oikos 90:279–294. doi:10.1034/j.1600-0706.2000.900208.x
Atlas RM, Horowitz A, Krichevsky M, Bej AK (1991) Response of microbial populations to environmental disturbance. Microb Ecol 22:249–256. doi:10.1007/BF02540227
van der Heijden MGA, Klironomos JN, Ursic M et al (1998) Mycorrhizal fungal diversity determines plant biodiversity, ecosystem variability and productivity. Nature 396:69–72. doi:10.1038/23932
Hayat R, Ali S, Amara U et al (2010) Soil beneficial bacteria and their role in plant growth promotion: a review. Ann Microbiol 60:579–598
Nannipieri P, Ascher J, Ceccherini MT et al (2003) Microbial diversity and soil functions. Eur J Soil Sci 54:655–670. doi:10.1046/j.1351-0754.2003.0556.x
Bardgett RD, van der Putten WH (2014) Belowground biodiversity and ecosystem functioning. Nature 515:505–511. doi:10.1038/nature13855
Karlen DL, Wollenhaupt NC, Erbach DC et al (1994) Long-term tillage effects on soil quality. Soil Tillage Res 32:313–327
Lupwayi NZ, Rice WA, Clayton GW (1998) Soil microbial diversity and community structure under wheat as influenced by tillage and crop rotation. Soil Biol Biochem 30:1733–1741. doi:10.1016/S0038-0717(98)00025-X
Menalled FD, Gross KL, Hammond M (2001) Weed aboveground and seedbank community responses to agricultural management systems. Ecol Appl 11:1586–1601
Pollnac FW, Maxwell BD, Menalled FD (2009) Using species-area curves to examine weed communities in organic and conventional spring wheat systems. Weed Sci 57:241–247. doi:10.1614/WS-08-159.1
Harbuck KSB, Menalled FD, Pollnac FW (2009) Impact of cropping systems on the weed seed banks in the northern Great Plains, USA. Weed Biol Manag 9:160–168. doi:10.1111/j.1445-6664.2009.00334.x
Thakur MP, Milcu A, Manning P et al (2015) Plant diversity drives soil microbial biomass carbon in grasslands irrespective of global environmental change factors. Glob Chang Biol 21:4076–4085. doi:10.1111/gcb.13011
Postma-Blaauw MB, de Goede RGM, Bloem J et al (2010) Soil biota community structure and abundance under agricultural intensification and extensification. Ecology 91:460–473
Flohre A, Rudnick M, Traser G et al (2011) Does soil biota benefit from organic farming in complex vs. simple landscapes? Agric Ecosyst Environ 141:210–214. doi:10.1016/j.agee.2011.02.032
DeAngelis KM, Brodie EL, DeSantis TZ et al (2009) Selective progressive response of soil microbial community to wild oat roots. ISME J 3:168–178
Wieland G, Neumann R, Backhaus H (2001) Variation of microbial communities in soil, rhizosphere, and rhizoplane in response to crop species, soil type, and crop development. Appl Environ Microbiol 67:5849–5854. doi:10.1128/AEM.67.12.5849-5854.2001
Duineveld BM, Kowalchuk GA, Keijzer A et al (2001) Analysis of bacterial communities in the rhizosphere of chrysanthemum via denaturing gradient gel electrophoresis of PCR-amplified 16S rRNA as well as DNA fragments coding for 16S rRNA. Appl Environ Microbiol 67:172–178. doi:10.1128/AEM.67.1.172-178.2001
Germida JJ, Siciliano SD, Renato de Freitas J, Seib AM (1998) Diversity of root-associated bacteria associated with field-grown canola (Brassica napus L.) and wheat (Triticum aestivum L.). FEMS Microbiol Ecol 26:43–50. doi:10.1111/j.1574-6941.1998.tb01560.x
Hao JJ, Davis RM (2009) Effect of soil inoculum density of Fusarium oxysporum f. sp. vasinfectum on disease development in cotton. Plant Dis 93:1324–1328
Ballaminutt N, Matheus DR (2007) Characterization of fungal inoculum used in soil remediation. Braz J Microbiol 28:249–252
White D, Crosbie JD, Atkinson D, Killham K (1994) Effect of an introduced inoculum on soil microbial diversity. FEMS Microbiol Ecol 14:169–178
Griffiths B, Ritz K, Wheatley R et al (2001) An examination of the biodiversity–ecosystem function relationship in arable soil microbial communities. Soil Biol Biochem 33:1713–1722. doi:10.1016/S0038-0717(01)00094-3
Johnson SP, Miller ZJ, Lehnhoff EA, Miller PR, Menalled FD (2016) Cropping systems modify the impacts of biotic plant-soil feedbacks on wheat (Triticum aestivum L.) growth and competitive ability. Weed Res (in press)
Muyzer G, de Waal EC, Uitterlinden AG (1993) Profiling of complex microbial populations by denaturing gradient gel electrophoresis analysis of polymerase chain reaction-amplified genes coding for 16S rRNA. Appl Environ Microbiol 59:695–700
Caporaso JG, Lauber CL, Walters WA et al (2011) Global patterns of 16S rRNA diversity at a depth of millions of sequences per sample. Proc Natl Acad Sci U S A 108(Suppl):4516–4522. doi:10.1073/pnas.1000080107
Masella AP, Bartram AK, Truszkowski JM et al (2012) PANDAseq: paired-end assembler for illumina sequences. BMC Bioinforma 13:31. doi:10.1186/1471-2105-13-31
Luo C, Tsementzi D, Kyrpides N et al (2012) Direct comparisons of Illumina vs. Roche 454 sequencing technologies on the same microbial community DNA sample. PLoS One 7:e30087. doi:10.1371/journal.pone.0030087
Schloss PD, Westcott SL, Ryabin T et al (2009) Introducing mothur: open-source, platform-independent, community-supported software for describing and comparing microbial communities. Appl Environ Microbiol 75:7537–7541
Kozich JJ, Westcott SL, Baxter NT et al (2013) Development of a dual-index sequencing strategy and curation pipeline for analyzing amplicon sequence data on the MiSeq Illumina sequencing platform. Appl Environ Microbiol 79:5112–5120. doi:10.1128/AEM.01043-13
Wang Q, Garrity GM, Tiedje JM, Cole JR (2007) Naïve Bayesian classifier for rapid assignment of rRNA sequences into the new bacterial taxonomy. Appl Environ Microbiol 73:5261–5267
Edgar RC, Haas BJ, Clemente JC et al (2011) UCHIME improves sensitivity and speed of chimera detection. Bioinformatics 27:2194–2200. doi:10.1093/bioinformatics/btr381
Huse SM, Welch DM, Morrison HG, Sogin ML (2010) Ironing out the wrinkles in the rare biosphere through improved OTU clustering. Environ Microbiol 12:1889–98. doi:10.1111/j.1462-2920.2010.02193.x
Chao A, Shen T-J (2010) Program SPADE (Species Prediction And Diversity Estimation). Available at: http://chao.stat.nthu.edu.tw.
Burnham KP, Overton WS (1979) Robust estimation of population size when capture probabilities vary among animals. Ecology 60:927. doi:10.2307/1936861
Jost L (2006) Entropy and diversity. Oikos 113:363
Good IJ (1953) On population frequencies of species and the estimation of population parameters. Biometrika 40:237–264
Shannon CE, Weaver W (1949) The mathematical theory of communication. University of Illinois Press, Urbana
Clarke KR (1993) Non-parametric multivariate analyses of changes in community structure. J Ecol 18:117–143. doi:10.1111/j.1442-9993.1993.tb00438.x
Lozupone C, Knight R (2005) UniFrac: a new phylogenetic method for comparing microbial communities. Appl Envir Microbiol 71:8228–8235. doi:10.1128/AEM.71.12.8228-8235.2005
R Core Team (2015) R: a language and environment for statistical computing. R Foundation for Statistical Computing, Vienna
Beals EW (1984) Bray-Curtis ordination: an effective strategy for analysis of multivariate ecological data. Adv Ecol Res 14:1–55
Langille MGI, Zaneveld J, Caporaso JG et al (2013) Predictive functional profiling of microbial communities using 16S rRNA marker gene sequences. Nat Biotechnol 31:814–821. doi:10.1038/nbt.2676
Parks DH, Tyson GW, Hugenholtz P, Beiko RG (2014) STAMP: statistical analysis of taxonomic and functional profiles. Bioinformatics 30:3123–3124. doi:10.1093/bioinformatics/btu494
Tiemann LK, Grandy AS, Atkinson EE et al (2015) Crop rotational diversity enhances belowground communities and functions in an agroecosystem. Ecol Lett 18:761–771. doi:10.1111/ele.12453
Wolfe BE, Klironomos JN (2005) Breaking new ground: soil communities and exotic plant invasion. Bioscience 55:477. doi:10.1641/0006-3568(2005)055[0477:BNGSCA]2.0.CO;2
Bollen GJ (1969) The selective effect of heat treatment on the microflora of a greenhouse soil. Neth J Plant Pathol 75:157–163. doi:10.1007/BF02137211
Margosch D, Ehrmann MA, Buckow R et al (2006) High-pressure-mediated survival of Clostridium botulinum and Bacillus amyloliquefaciens endospores at high temperature. Appl Environ Microbiol 72:3476–3481. doi:10.1128/AEM.72.5.3476-3481.2006
Yap JM, Goldsmith CE, Moore JE (2013) Integrity of bacterial genomic DNA after autoclaving: possible implications for horizontal gene transfer and clinical waste management. J Hosp Infect 83:247–249. doi:10.1016/j.jhin.2012.11.016
Rocha EPC (2016) Using sex to cure the genome. PLoS Biol 14:e1002417. doi:10.1371/journal.pbio.1002417
Tillman D (1982) Resource competition and community structure. Princeton University Press, Princeton
Smith JL, Paul EA (1990) The significance of soil microbial biomass estimations. In: Bollag J, Stotsky G (eds) Soil biochemistry. Marcel Dekker, New York, pp 357–396
Fierer N, Ladau J, Clemente JC et al (2013) Reconstructing the microbial diversity and function of pre-agricultural tallgrass prairie soils in the United States. Science 342:621–624. doi:10.1126/science.1243768
Bergmann GT, Bates ST, Eilers KG et al (2011) The under-recognized dominance of Verrucomicrobia in soil bacterial communities. Soil Biol Biochem 43:1450–1455. doi:10.1016/j.soilbio.2011.03.012
Wu H, Fang Y, Yu J, Zhang Z (2014) The quest for a unified view of bacterial land colonization. ISME J 8:1358–69. doi:10.1038/ismej.2013.247
Burns JH, Anacker BL, Strauss SY, Burke DJ (2015) Soil microbial community variation correlates most strongly with plant species identity, followed by soil chemistry, spatial location and plant genus. AoB Plants 7:plv030. doi:10.1093/aobpla/plv030
Matson PA (1997) Agricultural intensification and ecosystem properties. Science 277:504–509. doi:10.1126/science.277.5325.504
Bever JD, Platt TG, Morton ER (2012) Microbial population and community dynamics on plant roots and their feedbacks on plant communities. Annu Rev Microbiol 66:265–283. doi:10.1146/annurev-micro-092611-150107
Wagg C, Jansa J, Schmid B, van der Heijden MGA (2011) Belowground biodiversity effects of plant symbionts support aboveground productivity. Ecol Lett 14:1001–1009
Mazzoleni S, Bonanomi G, Incerti G et al (2015) Inhibitory and toxic effects of extracellular self-DNA in litter: a mechanism for negative plant-soil feedbacks? New Phytol 205:1195–210. doi:10.1111/nph.13121
Singh HP, Batish DR, Kohli RK (1999) Autotoxicity: concept, organisms, and ecological significance. CRC Crit Rev Plant Sci 18:757–772. doi:10.1080/07352689991309478
Wood SA, Almaraz M, Bradford MA et al (2015) Farm management, not soil microbial diversity, controls nutrient loss from smallholder tropical agriculture. Front Microbiol 6:90. doi:10.3389/fmicb.2015.00090
Shi S, Nuccio E, Herman DJ et al (2015) Successional trajectories of rhizosphere bacterial communities over consecutive seasons. MBio 6:e00746. doi:10.1128/mBio.00746-15
Frey-Klett P, Garbaye J, Tarkka M (2007) The mycorrhiza helper bacteria revisited. New Phytol 176:22–36. doi:10.1111/j.1469-8137.2007.02191.x
Qasem JR (1995) Allelopathic effects of Amaranthus retroflexus and Chenopodium murale on vegetable crops. Allelopath J 2:49–66
Eisenhut M, Ruth W, Haimovich M et al (2008) The photorespiratory glycolate metabolism is essential for cyanobacteria and might have been conveyed endosymbiontically to plants. Proc Natl Acad Sci U S A 105:17199–17204
Yingping F, Lemeille S, Talla E et al (2014) Unravelling the cross-talk between iron starvation and oxidative stress responses highlights the key role of PerR (alr0957) in peroxide signalling in the cyanobacterium Nostoc PCC 7120. Environ Microbiol Rep 6:468–475. doi:10.1111/1758-2229.12157
Schumacher WJ, Thill DC, Lee GA (1983) Allelopathic potential of wild oat (Avena fatua) on spring wheat (Triticum aestivum) growth. J Chem Ecol 9:1235–1245. doi:10.1007/BF00982225
Iannucci A, Fragasso M, Platani C, Papa R (2013) Plant growth and phenolic compounds in the rhizosphere soil of wild oat (Avena fatua L.). Front Plant Sci 4:509. doi:10.3389/fpls.2013.00509
Mandal SM, Chakraborty D, Dey S (2010) Phenolic acids act as signaling molecules in plant-microbe symbioses. Plant Signal Behav 5:359–368
Barkovskii A, Bouillant ML, Monrozier LJ, Balandreau J (1995) Azospirillum strains use phenolic compounds as intermediates for electron transfer under oxygen-limiting conditions. Microb Ecol 29:99–114. doi:10.1007/BF00217426
Westerberg K, Elväng AM, Stackebrandt E, Jansson JK (2000) Arthrobacter chlorophenolicus sp. nov., a new species capable of degrading high concentrations of 4-chlorophenol. Int J Syst Evol Microbiol 50 Pt 6:2083–92. doi:10.1099/00207713-50-6-2083
Acknowledgments
The authors would like to thank Dr. Tiffanie Nelson, Montana State University, for her instruction in PRIMER and R. We also thank the farmers who allowed us to collect soil samples from their fields, Ali Thornton, Jesse Hunter, Madi Nixon, Ceci Welch, and Ethan Mayes who provided assistance with the greenhouse study. Further thanks to Subodh Adhikari, Sean McKenzie, and Wyatt Holmes for their help in gathering soil samples, and Dr. Cathy Zabinski for her insight on belowground ecology.
Author information
Authors and Affiliations
Corresponding authors
Ethics declarations
Funding
This work was conducted with funding provided by the USDA-OREI (Grant MONB00365), USDA-ORG (2011-04960), and the Montana University System Research Initiative: 51040-MUSRI2015-02.
Electronic Supplementary Material
Below is the link to the electronic supplementary material.
Supplementary Table 1
(DOCX 19 kb)
Supplementary Table 2
(DOCX 38 kb)
Supplementary Table 3
(DOCX 23 kb)
Supplementary Fig. 1
(DOCX 527 kb)
Supplementary Fig. 2
(DOCX 279 kb)
Supplementary Fig. 3
(DOCX 320 kb)
Rights and permissions
About this article
Cite this article
Ishaq, S.L., Johnson, S.P., Miller, Z.J. et al. Impact of Cropping Systems, Soil Inoculum, and Plant Species Identity on Soil Bacterial Community Structure. Microb Ecol 73, 417–434 (2017). https://doi.org/10.1007/s00248-016-0861-2
Received:
Accepted:
Published:
Issue Date:
DOI: https://doi.org/10.1007/s00248-016-0861-2