Abstract
In our study, we collected 146 surface soil samples in Xiamen City and measured the concentrations of five heavy metals (Cr, Cu, Pb, Ni, and Zn) and one metalloid (As). Multivariate statistics, geostatistics and Random Forest methods were applied to identify the potential sources and spatial variation of the six elements. The results revealed that As, Cr, and Ni originated mainly from industrial activities, and higher concentrations were found in developed areas. The amounts of Cu, Pb, and Zn in soils were mainly predetermined by soil parent material and agricultural activities. Besides, the atmospheric deposition rather than industrial activities substantially influenced the accumulation of Pb in the soils near the boundary between Tong’an and Quanzhou City, because there were few industries but many forests in this area. Because of the connections of the six elements with factor values of factor analysis, appropriate and accessible covariates could be used in co-kriging to increase the accuracies of interpolation of heavy metal and metalloid concentrations relative to that in ordinary kriging.
Similar content being viewed by others
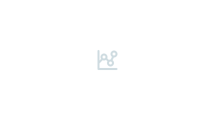
Explore related subjects
Discover the latest articles, news and stories from top researchers in related subjects.Avoid common mistakes on your manuscript.
With the acceleration of urbanization, the accumulation of heavy metals in soil has become particularly hazardous, causing a growing public concern (Möller et al. 2005; Yang et al. 2009). Excessive amounts of heavy metals affect soil physicochemical properties, inhibit microbial activity, and reduce the supply of nutrients to plants. In addition, heavy metals are transmitted to organisms through oral ingestion, dermal contact, and inhalation, significantly threatening human health and the environment (Wuana and Okieimen 2011). Since heavy metals were difficult to be removed by natural processes, their accumulations in the soil over time lead to potential negative effects on the ecosystem and humans (Zhuang et al. 2009).
The sources of heavy metals in the soil are generally divided into natural and anthropogenic inputs. Pedogenic processes are considered as the main natural source of soil heavy metals, whereas anthropogenic inputs originate from various human activities, such as agriculture, smelting, manufacturing, mining, coal burning, transportation, and atmospheric deposition (Zhang 2006). Moreover, the major sources of heavy metals in most Chinese urban and suburban soils vary widely. The modernization of transportation and industry in urban areas have exacerbated the problem of heavy metal pollution because of excessive emissions, whereas heavy metal contaminants of suburban soils are mostly derived from farming activities, such as the application of fertilizers, insecticides, and sewage irrigation (Cai et al. 2012; Sebai et al. 2007; Wei and Yang 2010). Many researchers found that the content of heavy metals such as Pb, Cu, Zn, Ni and Cd increased during sewage sludge additions (McGrath and Cegarra 1992). As a metalloid element commonly used in industry activities, arsenic is easily accumulated in the urban soils, and has been included in many heavy metals studies (Chen et al. 2016; Luo et al. 2012). Thus, arsenic pollution is considered as an important part of this study. The identification of the sources of soil heavy metal and metalloid pollutant in a city with both urban and suburban areas is more complex because of the manifold anthropogenic impacts. Therefore, before taking measures to control soil heavy metal and metalloid pollution and protect soil environment, it is necessary to identify not only the source of pollution, but also the scale of contamination.
Generally, multivariate statistical analyses, including correlation analysis, principal component analysis and cluster analysis, are applied to identify the sources of pollutants (Praveena et al. 2008; Shukla et al. 2017; Sun et al. 2013). Factor analysis (FA) is closely bound up with PCA, and the purpose is to decrease the multivariate datasets dimensionality and create a set of variables as dominant factors to evaluate data. FA can be used to determine the pollution source as well (Lin et al. 2012). Moreover, spatial distribution is usually determined using geostatistical methods, which are the most effective approach to studying spatial differences in soil heavy metals (Facchinelli et al. 2001; Chen et al. 2016). Ordinary kriging (OK), which is a univariate interpolation method, was applied widely, for determination of the spatial patterns of heavy metals (Ma et al. 2016). Yet without auxiliary information, the interpolation accuracy of OK is not high and need to be improved (Wu et al. 2003). Thus, to obtain an accurate spatial distribution map, different interpolation methods were used and compared, and many studies have revealed the efficiency of co-kriging (CK) (Chen et al. 2015; Wang et al. 2012a, b).
Xiamen City is one of China’s first four national-level Special Economic Zones (SEZs), which is located on the southeast coast of China, facing the Taiwan Strait (Tang et al. 2013). In recent years, the economic development and intensified industrialization might increase the soil heavy metal and metalloid contamination of the Xiamen City area. However, few studies have investigated the heavy metal and metalloid pollution of the city, and the spatial distribution of heavy metals and metalloid is unavailable. Therefore, it was crucial to investigate the impact of rapid urbanization on soil heavy metal and metalloid pollution to promote the ecological environment protection of Xiamen City. Urban soils are generally regarded as an important component of urban ecosystem, and excessive inputs of pollutants may deteriorate the soil environment and alter soil physical and chemical properties. A more serious problem is that these pollutants may travel from urban soils to humans via various pathways (e.g., direct inhalation, ingestion, and skin contact absorption) and negatively impact human health. Our research findings can contribute to much-needed reference values for controlling soil pollution of Xiamen. The objectives of this work were as follows: (1) to identify the potential sources of soil heavy metals and metalloid in Xiamen; (2) to simulate the spatial distribution of these elements using CK interpolation with available covariates; and (3) to quantify the contributions of selected factors influencing heavy metals and metalloid accumulation. These results will contribute to a more effective governmental control and mitigation of heavy metal and metalloid pollution resulting from urban sprawl.
Materials and Methods
With an area of 1700 hectares and 4 million residents, Xiamen City is one of the most developed cities in the southern part of Fujian Province (24°23′N to 24°54′N, 117°53′E to 118°26′E) in South China (Fig. 1). The weather of the city is monsoon humid subtropical climate own to its geographic location. The average annual temperature and rainfall are 21 °C and 1513.3 mm respectively (Huang et al. 2018). Xiamen City consists of six districts: Siming and Huli are urban areas with high degree of urbanization, Haicang, Jimei, Tong’an, and Xiang’an are suburban areas (Fig. 1).
Xiamen Island, including Huli and Siming Districts, is the commercial and political center of Xiamen City. We divided Xiamen Island into 1.5 × 1.5 km sized grids. The other four districts outside Xiamen Island were relatively undeveloped and homogeneous, and their grid size was increased to 4 × 4 km. Based on land use and topographic conditions, we selected one sample site from each grid. We collected samples from all districts and the samples represented different types of land use to insure the data to be reasonably representative. A total number of 146 topsoil samples were obtained in Xiamen City from September to October 2016 using a systematic sampling strategy. Each composite sample comprised five subsamples obtained from a 5 × 5 m area using a stainless-steel trowel. The depth of the subsamples was 0–20 cm. The locations of the sampling sites were determined as central points obtained by a GPS device. Each composite soil sample was sealed in a plastic bag. The soil samples were firstly air-dried for three days at room temperature (25 °C), filtered through a 2 mm polyethylene sieve to remove coarse materials and large debris and then further ground to particles with a diameter of less than 200 μm with agate mortar. After that, we stored the homogenized powdered samples in plastic bags until analysis.
After digestion with a mixed solution of HNO3-HF-HClO4, these soil samples were prepared for quantification of five heavy metals (Cr, Cu, Pb, Ni, and Zn) and one metalloid (As). For determination of the concentrations of these elements, PEAA800 flame atomic absorption spectrophotometer (Perkin Elmer, Fremont, CA, USA) was used. A separate bulk sample was tested after every 10 analyses as an analytical control. A procedural blank was performed for every 10 sample analyses. The analyzed precision was between 5% and 6% and did not exceed 8%. Quality assurance and quality control (QA/QC) were accomplished via the determinations of the studied parameters in the certified standard soil samples (soil GBW07405). The recoveries of the six elements ranged between 87.6% and 100%.
Geostatistics is a statistical tool used to study and predict the spatial distribution of regional variables that is now extensively used in environmental research because of its utility (Chen et al. 2016). As a multivariate extension of OK, CK uses auxiliary variables to increase the accuracy of interpolated results in the prediction process, which can remedy the distortion due to interpolation (Chen et al. 2016). CK uses covariate information and considers additional correlates to improve the prediction precision of spatial distribution, which requires the creation of cross-variograms, which calculated by the following formula (Chen et al. 2009).
where \({\gamma }_{ij}\left(h\right)\) is the cross-semivariance of two random variables as a function of h, and \(N\left(h\right)\) is the number of pairs of \({Z}_{i}\left(x\right)\) and \({Z}_{j}\left(x\right)\) at a distance h. We used Pearson correlation coefficient between the measured and predicted values (r) and root mean square error (RMSE) to examine the accuracy of interpolations of OK and CK. Moreover, we employed a criterion, according to which lower RMSE values and higher r values indicated a smaller estimation error and increased prediction accuracy in our investigation.
Random Forest is a commonly applied method that performs well in improving the model prediction performance and assessing variables importance in the classification (Breiman 2001). The environmental variables we collected were routinely considered as potential sources of heavy metals and metalloids, including the density of ordinary factories (e.g. for production of food, clothing, stones, etc.), and the densities of metallurgy and machine and electronics factories, as well as that of construction, pharmaceutical, and agricultural companies, and road density, land use types, and population density. These variables were converted into raster data and divided into 88 cells (5 × 5 km) (Figs. S1, S2). The mean values of all density types in each cell were used as independent variables in the Random Forest. The data of all kinds of factories and companies were from the points of interest (POIs) data gathered from Gaode Maps in 2015. The Xiamen City Road Atlas in 2015 was transformed into the road network vector data by Yang et al. (2018) for calculating road density. The land use data for 2015 were obtained from Landsat TM remote-sensing images and were represented as the area percent of five types in each cell: farmland, forest, grassland, water area, and built-up land.
In this study, multivariate statistical analyses, including correlation analysis, FA were carried out with SPSS (version 20.0, IBM, USA). The geostatistical analysis was conducted by GS+ (version 10.0, Gamma Design Software LLC., USA). The CK interpolations of soil heavy metal and metalloid contents were carried out in ArcGIS (version10.2, ESRI Inc., USA). Random Forest analysis was implemented in R 3.2.1 software.
Results and Discussion
Table 1 summarizes the basic statistical attributes of five heavy metals and one metalloid as well as their background values of soils in Fujian Province. The background values of Fujian Province in our study were determined by other researchers and the result was published on the Chinese Journal of Environmental Science (ISSN: 0250-3301) in 1992 (Chen et al. 1992). Of note, the mean values of As, Cr, Cu, and Pb exceeded the background values. We also evaluated the data using the second criterion of the Chinese Environmental Quality Standard for Soils (GB 15618–1995), whose values can be employed as thresholds for agricultural soils (Luo et al. 2012). The first and second criteria are from the Chinese Environmental Quality Standard for Soils (GB 15618–1995) (SEPAC 1995), in which class I criteria represented natural background values, while class II were mainly used as the threshold values for agricultural soils to protect human health (Wang et al. 2012a, b). We found that the concentrations of As and Pb in the all soil samples were lower than the threshold levels, whereas the concentrations of Cr, Cu, Ni and Zn in one, two, one and four sites were exceeded the corresponding thresholds, respectively, suggesting that a few sites in Xiamen pose a risk to human health.
Pearson's correlation coefficients among the six elements are listed in Table S1. Additionally, As, Cr, Cu, Ni, and Zn significantly correlated with each other, whereas only that of Pb correlated weakly with Cr and Ni. The Kaiser–Meyer–Olkin (KMO) value (0.721) and Bartlett's test (403.150, p = 0.000) showed that the data were suitable for FA. Factor 1 (F1) was dominated by Cu and Zn (0.87 and 0.88, respectively), explaining 54.13% of the total variance (Fig. 2). Moreover, Cr and Ni were closely related to the second factor (F2) with high scores (0.95 and 0.79, respectively), accounting for 16.98% of the total variance (Fig. 2). Arsenic was represented mainly by the third factor (F3) and accounted for 11.32% of the total variance. The fourth factor (F4) accounted for 10.78% of the total variance, and the dominant metal was Pb, indicating that Pb was clearly distinct from the other elements in the FA results. However, the eigenvalues of factors 3 and 4 were below 1 before factor rotation. Therefore, factors 1 and 2 were extracted, accounting for 71.11% of the total variation in heavy metal and metalloid concentrations and were sufficient to explain the sources of the heavy metals and metalloid in soils (Table 2). The results of correlation coefficients and FA suggest that both As and Pb might have a source different from those of the other four elements. The ordination of the 146 samples showed that the samples from built-up land were partly separated with the samples from farmland (Fig. 3a). In addition, the sample sites from Huli, Jimei and Siming had higher densities of road and factory compared with other districts (Fig. 3b). Moreover, the densities of road and factory of farmland sample sites were the lowest (Fig. 3c).
The parameters of the semivariogram analysis for each element are displayed in Table S2. As a spatial structure indicator, the comparisons of range values could express the spatial variability of these metals, the higher range of the values in the semivariogram analysis indicates better spatial dependency and a lower anthropogenic influence (Chen et al. 2009). Comparing with other metals, a metal with relatively a short range has high a spatial variability and will be more vulnerable to human activities. “Shorter range” and “Longer range” of the metals were defined by comparing each other in this study. Our results revealed that As, Cr, and Ni had shorter ranges (3.99 km, 1.92 km, and 2.85 km, respectively) than Cu, Pb, and Zn (17.49 km, 18.60 km, and 24.07 km, respectively), suggested that Cu, Pb, and Zn were mainly controlled by soil parent material, whereas As, Cr, and Ni were primarily influenced by anthropogenic sources with higher spatial variability. Based on FA, it was shown that each metal connected closely to a factor. In practical terms, various valuable auxiliary factors are used for increasing interpolation accuracy. In this study, we attempted to use factor values in FA as covariates to obtain spatial patterns of heavy metals and metalloid with a higher accuracy. Because the significant positive relationship between Cr and Ni as well as Cu and Zn, we developed variate–covariate models such as Cr–Ni, Ni–Cr, Cu–Zn, Zn–Cu in the CK interpolation as well. The RMSE and r values of OK and CK with different covariates are showed in Table S3. Compared with OK, CK had lower RMSE and higher r values when the covariate was the FA factor the metal belonged to, indicating that CK got more accurate interpolations. While the most appropriate covariate of CK for Cr was Ni, instead of any FA factor. The highest estimated r values by CK interpolations for As, Cr, Pb, Ni, and Zn were markedly higher than those in OK (0.506** to 0.942**, 0.114 to 0.751**, 0.424** to 0.568**, 0.232** to 0.752**, 0.459** to 0.839** and 0.235** to 0.882, respectively), indicating that the results of the CK interpolation were closer to reality than the ones of the OK interpolation. In this research, we also considered the effects of land use, roads, human population, organic matter contents and elevation on metal/metalloid concentrations. We calculated their Pearson correlation coefficients between the measured and predicted RMSE and r values to examine the accuracy of interpolations. We used the proportions of forest and built-up land-use types at a given sampling site grid to represent the effects of different land use, used road density to represent the effects of traffic, used human population density to represent the effects of residential activities, and used organic matter contents (OMC) and elevation to represent the effects of soil properties and geography. The results, as showed in the Table S4, indicate that the accuracies of these interpolations are not higher than the former ones.
The variate–covariates of CK with the highest accuracy of the six elements were as follows: As-F3, Cr-Ni, Cu-F1, Pb-F4, Ni-F2, and Zn-F1. The spatial distribution of five heavy metals and one metalloid in the soil of Xiamen City are illustrated in Fig. 4. As, Cr, and Ni showed similar trends in their distribution, with high concentrations in the urban and near-urban areas of the city and low concentrations in the north suburban areas. Hotspots of As, Cr, and Ni pollution were identified in the Haicang, South Jimei and Huli, with high degree of urbanization and industrialization in Xiamen City. Therefore, the levels of accumulation of As, Cr, and Ni were associated with the action of multiple factors, of which industrial activities might be the most important. As, Cr and Ni were highly accumulated in areas near the city center, while the spatial distribution of Cu, Pb, and Zn showed that these heavy metals were also abundant in the suburban areas far away from the downtown. In addition, Pb accumulated at the boundary between Xiamen City and Quanzhou City.
Based on the CK map, the mean values of six elements contents in each cell were calculated and used as the dependent variables in the Random Forest analysis. For better comparison, importance scores of all influencing factors affecting the accumulation of the soil heavy metals and metalloid were rescaled to sum to 1 (Fig. S3). The results of Random Forest showed that the industrial influencing factors had a higher effect on As, Cr, and Ni pollution than on that with Cu, Pb, and Zn. The result of FA showed that Cr and Ni were associated with each other, suggesting that they might have come from the same pollution source. It was found that high Cr and Ni concentrations have often been associated with urbanization process, such as industrial activities, coal combustion and traffic emission (Chen et al. 2016; Li and Feng 2010). Yuswir et al. (2015) had found that electronics and semiconductor industry might be the major source of Cr, Cd and Co. A well-developed electronics industry might increase the concentrations of Cr and Ni in the surrounding soils (O’Connell et al. 2008), causing Cr and Ni pollution in the soils of Haicang, Jimei and Huli. According to findings of previous studies, the enrichment of As in soil was related to natural origin and the effects of human activities, such as fossil coal burning and exhaust emission from metallurgical industry (Chen et al. 2016; Ma et al. 2016). In our results, the contamination with As was primarily influenced by human activities as well, and might have been caused by exhaust emission during industrial activities.
It is noteworthy that except for the natural sources, agriculture seemed to be a common potential anthropogenic source of Cu, Pb and Zn pollution, due to the frequent their use through the application of chemical fertilizers and pesticides (Chen et al. 2009). Kheir et al. (2010) found that agricultural land use significantly increased the Zn content in soil, which is consistent with the results of Random Forest. Machine and electronics factory density also influenced the accumulation of Cu, indicating that industrial activities had a certain effect on Cu contents (Hao et al. 2016; Ma et al. 2016). Besides, the geostatistical analysis showed that the spatial distribution of Pb was different from that of the other elements (Fig. 4). Vehicle emissions have been largely reported as the most common source of high Pb contents in urban soils (Chen et al. 2016; Ma et al. 2016). Furthermore, atmosphere deposition has been implicated as a main source of Pb in recent studies (Dach and Starmans 2005). In our investigation, the results of the correlation analysis and FA showed that the source of Pb was distinct from those of the other metals. Importantly, the areas with high Pb concentration in the spatial mapping were not the areas with high road density (Fig. S2), which indicated that the accumulation of this heavy metal element in the soil was not significantly associated with traffic emission. Therefore, we considered that one of the main sources of Pb in Xiamen City, especially in the suburban areas, might from coal combustion and industry emission, and transport through atmospheric deposition.
References
Breiman L (2001) Random forests. Mach Learn 45(1):5–32
Cai L, Xu Z, Ren M et al (2012) Source identification of eight hazardous heavy metals in agricultural soils of Huizhou, Guangdong Province, China. Ecotox Environ Safe 78:2–8
Chen Z, Chen C, Liu Y, Wu Y, Yang S, Lu C (1992) The study on background value of soil environment in Fujian Province. Environ Sci. 4:70–75 (In Chinese)
Chen T, Liu X, Li X et al (2009) Heavy metal sources identification and sampling uncertainty analysis in a field-scale vegetable soil of Hangzhou, China. Environ Pollut 157(3):1003–1010
Chen T, Chang Q, Clevers J, Kooistra L (2015) Rapid identification of soil cadmium pollution risk at regional scale based on visible and near-infrared spectroscopy. Environ Pollut 206:217–226
Chen T, Chang Q, Liu J, Clevers J, Kooistra L (2016) Identification of soil heavy metal sources and improvement in spatial mapping based on soil spectral information: a case study in northwest China. Sci Total Environ 565:155–164
Dach J, Starmans D (2005) Heavy metals balance in Polish and Dutch agronomy: actual state and previsions for the future. Agr Ecosyst Environ 107(4):309–316
Facchinelli A, Sacchi E, Mallen L (2001) Multivariate statistical and GIS-based approach to identify heavy metal sources in soils. Environ Pollut 114(3):313–324
Hao L, Tian M, Zhao X, Zhao Y, Lu J, Bai R (2016) Spatial distribution and sources of trace elements in surface soils, Changchun, China: insights from stochastic models and geostatistical analyses. Geoderma 273:54–63
Huang S, Wang L, Zhao Y (2018) Ecological risk assessment from the perspective of soil heavy metal accumulations in Xiamen city, China. Int J Sust Dev World 1–9.
Kheir RB, Greve MH, Abdallah C, Dalgaard T (2010) Spatial soil zinc content distribution from terrain parameters: a GIS-based decision-tree model in Lebanon. Environ Pollut 158(2):520–528
Li X, Feng L (2010) Spatial distribution of hazardous elements in urban topsoils surrounding Xi’an industrial areas (NW, China): controlling factors and contamination assessments. J Hazard Mater 174(1):662–669
Lin CY, Abdullah MH, Praveena SM, Yahaya AHB, Musta B (2012) Delineation of temporal variability and governing factors influencing the spatial variability of shallow groundwater chemistry in a tropical sedimentary island. J Hydrol 432:26–42
Luo XS, Yu S, Zhu YG, Li XD (2012) Trace metal contamination in urban soils of China. Sci Total Environ 421–422(3):17–30. https://doi.org/10.1016/j.scitotenv.2011.04.020
Ma L, Yang Z, Li L, Wang L (2016) Source identification and risk assessment of heavy metal contaminations in urban soils of Changsha, a mine-impacted city in Southern China. Environ Sci Pollut R 23(17):17058–17066
McGrath SP, Cegarra J (1992) Chemical extractability of heavy metals during and after long-term applications of sewage sludge to soil. J Soil Sci 43(2):313–321
Möller A, Müller HW, Abdullah A, Abdelgawad G, Utermann J (2005) Urban soil pollution in Damascus, Syria: concentrations and patterns of heavy metals in the soils of the Damascus Ghouta. Geoderma 124(1):63–71
O’Connell DW, Birkinshaw C, O’Dwyer TF (2008) Heavy metal adsorbents prepared from the modification of cellulose: a review. Bioresour Technol 99(15):6709–6724
Praveena SM, Ahmed A, Radojevic M, Abdullah MH, Aris AZ (2008) Heavy metals in mangrove surface sediment of Mengkabong Lagoon, Sabah: multivariate and geo-accumulation index approaches. Int J Environ Res 2(2):139–148
Sebai T, Lagacherie B, Soulas G, Martin-Laurent F (2007) Spatial variability of isoproturon mineralizing activity within an agricultural field: geostatistical analysis of simple physicochemical and microbiological soil parameters. Environ Pollut 145(3):680–690
Shukla K, Kumar B, Agrawal R, Priyanka K, Venkatesh M (2017) Assessment of Cr, Ni and Pb pollution in rural agricultural soils of tonalite-trondjhemite series in central India. Bull Environ Contam Toxicol 98(6):856–866
Sun C, Liu J, Wang Y, Sun L, Yu H (2013) Multivariate and geostatistical analyses of the spatial distribution and sources of heavy metals in agricultural soil in Dehui, Northeast China. Chemosphere 92(5):517–523
Tang L, Zhao Y, Yin K, Zhao J (2013) Xiamen. Cities 31:615–624
Wang M, Bai Y, Chen W, Markert B, Peng C, Ouyang Z (2012a) A GIS technology based potential eco-risk assessment of metals in urban soils in Beijing, China. Environ Pollut 161:235–242
Wang M, Markert B, Chen W, Peng C, Ouyang Z (2012b) Identification of heavy metal pollutants using multivariate analysis and effects of land uses on their accumulation in urban soils in Beijing China. Environ Monit Assess 184(10):5889–5897
Wei B, Yang L (2010) A review of heavy metal contaminations in urban soils, urban road dusts and agricultural soils from China. Microchem J 94(2):99–107
Wu J, Norvell WA, Hopkins DG, Smith DB, Ulmer MG, Welch RM (2003) Improved prediction and mapping of soil copper by kriging with auxiliary data for cation-exchange capacity. Soil Sci Soc Am J 67(3):919–927
Wuana RA, Okieimen FE (2011) Heavy metals in contaminated soils: a review of sources, chemistry, risks and best available strategies for remediation. ISRN Ecol 2011.
Yang P, Mao R, Shao H, Gao Y (2009) An investigation on the distribution of eight hazardous heavy metals in the suburban farmland of China. J Hazard Mater 167(1):1246–1251
Yang X, Wang L, Wu T, Qiu Q (2018) Ecological risk assessment based on road network development analysis of Xiamen city, China. Int J Sust Dev World 1–10.
Yuswir NS, Praveena SM, Aris AZ, Ismail SNS, Hashim Z (2015) Health risk assessment of heavy metal in urban surface soil (Klang District, Malaysia). Bull Environ Contam Toxicol 95(1):80–89
Zhang C (2006) Using multivariate analyses and GIS to identify pollutants and their spatial patterns in urban soils in Galway, Ireland. Environ Pollut 142(3):501–511
Zhuang P, Zou H, Shu W (2009) Biotransfer of heavy metals along a soil-plant-insect-chicken food chain: field study. J Environ Sci 21(6):849–853
Acknowledgements
This work was supported by the National Key R&D Program of China (2016YFC0502902) and the National Natural Science Foundation of China (71533003).
Author information
Authors and Affiliations
Corresponding author
Electronic Supplementary Material
Below is the link to the electronic supplementary material.
Rights and permissions
About this article
Cite this article
Huang, S., Shao, G., Wang, L. et al. Spatial Distribution and Potential Sources of Five Heavy Metals and One Metalloid in the Soils of Xiamen City, China. Bull Environ Contam Toxicol 103, 308–315 (2019). https://doi.org/10.1007/s00128-019-02639-5
Received:
Accepted:
Published:
Issue Date:
DOI: https://doi.org/10.1007/s00128-019-02639-5