Abstract
A strategy was proposed for constructing core collections by least distance stepwise sampling (LDSS) based on genotypic values. In each procedure of cluster, the sampling is performed in the subgroup with the least distance in the dendrogram during constructing a core collection. Mean difference percentage (MD), variance difference percentage (VD), coincidence rate of range (CR) and variable rate of coefficient of variation (VR) were used to evaluate the representativeness of core collections constructed by this strategy. A cotton germplasm collection of 1,547 accessions with 18 quantitative traits was used to construct core collections. Genotypic values of all quantitative traits of the cotton collection were unbiasedly predicted based on mixed linear model approach. By three sampling percentages (10, 20 and 30%), four genetic distances (city block distance, Euclidean distance, standardized Euclidean distance and Mahalanobis distance) combining four hierarchical cluster methods (nearest distance method, furthest distance method, unweighted pair-group average method and Ward’s method) were adopted to evaluate the property of this strategy. Simulations were conducted in order to draw consistent, stable and reproducible results. The principal components analysis was performed to validate this strategy. The results showed that core collections constructed by LDSS strategy had a good representativeness of the initial collection. As compared to the control strategy (stepwise clusters with random sampling strategy), LDSS strategy could construct more representative core collections. For LDSS strategy, cluster methods did not need to be considered because all hierarchical cluster methods could give same results completely. The results also suggested that standardized Euclidean distance was an appropriate genetic distance for constructing core collections in this strategy.
Similar content being viewed by others
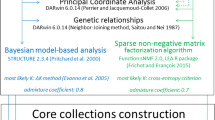
Avoid common mistakes on your manuscript.
Introduction
The concept of core collection was proposed by Frankel (1984). A core collection is defined as a representative sample of the whole collection with minimum repetitiveness and maximum genetic diversity of a crop species and its relatives (Frankel and Brown 1984a, b; Brown 1989). The core collection is served as a working collection that could be evaluated and utilized preferentially, which could solve the problem of large size of collection hindering the preservation and utilization of germplasm resource. Core collection is a convenient way to study and utilize germplasm resources and has been received the extensive attention all over the world.
Cluster analysis has been widely used as an important tool to group accessions for constructing core collection (Hintum 1995; Zhang et al. 2004). For example, cluster analysis was used to separate similar accessions to establish chickpea core collection and chickpea mini core subset (Upadhyaya and Ortiz 2001). Zewdie et al. (2004) used cluster analysis to classify accessions of capsicum based on the data of morphological traits. Then they established the capsicum core collection by three sampling methods based on results of the cluster analysis. Cluster analysis was also adopted in grouping data based on molecular markers in some researches of core collection (Baranger et al. 2004; Chabane and Valkoun 2004). However, there are several cluster methods that can be chosen during the course of cluster. Zhang et al. (2000) compared eight cluster methods when researching the construction of sesame core collection, and found that Ward’s method was most feasible. Some researches suggested that cluster methods should be combined with corresponding sampling methods during constructing core collection (Hu et al. 2000c; Li et al. 2004).
There are different strategies for sampling core accessions, such as random strategy, constant strategy, proportional strategy, logarithmic strategy and genetic diversity-dependent strategy (Brown 1989; Yonezawa et al. 1995). Hu et al. (2000a, b, c) suggested three sampling methods to select core accessions by stepwise clusters, which could construct more reliable core collections because method of stepwise clusters could avoid the unequal size of subgroups and unsymmetrical sampling. However, most of those strategies are based on cluster analysis and random sampling. The constructing results of those strategies are greatly affected by the cluster methods. Therefore, before constructing core collections, many work needs to be done to find an appropriate cluster method. The present paper proposed a strategy for constructing more reliable core collections based on the least distance stepwise sampling that did not need to consider cluster methods. Genetic diversity of core collections constructed by this method was evaluated to assess the validity of the method. Optimal parameters for constructing core collections based on this strategy were selected by simulations.
Materials and methods
Materials
An initial collection of 1,547 cotton genotypes served to construct core collections. All the 1,547 genotypes were planted for 2 years with two replications per year. The observed data of 18 quantitative traits were recorded. There were nine agronomy traits (plant height, height of fruit branch, length of fruiting node, length of boll stalk, number of fruiting branch per plant, bolls per plant, growth period, boll weight and lint percentage), five fiber traits (length, uniformity, strength, elongation and micronaire) and four seed traits (seed length, seed width, ratio of length to width and kernel weight) in the initial collection.
Genetic models and statistical methods
In the genetic experiments for evaluating germplasm resources in a single environment with at least two replications, the observed values could be expressed as \(Y_{{k(ij)}} = \mu + R_{i} + C_{j} + G_{{k(ij)}} + \varepsilon _{{k(ij)}} ,\) where μ is the population mean; R i is the fixed effect of the ith row; C j is the fixed effect of the jth column; G k(ij) is the random effect of the kth genotype within the ith row and the jth column, G k(ij) ∼ (0, σ 2 G ); ε k(ij) is the residual effect, ε k(ij) ∼ (0, σ 2ε ). In the complicated genetic experiments, which are conducted for multiple environments with at least two replications per environment, the observed values could be expressed as \(Y_{{hk(ij)}} = \mu + E_{h} + R_{{i(h)}} + C_{{j(h)}} + G_{{k(ij)}} + GE_{{hk(ij)}} + \varepsilon _{{hk(ij)}} ,\) where μ is the population mean; E h is the fixed effect of the hth environment; R i(h) is the fixed effect of the ith row within the hth environment; C j(h) is the fixed effect of the jth column within the hth environment; G k(ij) is the random effect of the kth genotype within the ith row and the jth column, G k(ij ) ∼ (0, σ 2 G ); GE hk(ij) is the random effect of the interaction between the hth environment and the kth genotype, GE hk(ij) ∼ (0, σ 2 GE ); ε hk(ij) is the residual effect, ε hk(ij) ∼ (0, σ 2ε ).
Minimum norm quadratic unbiased estimation (MINQUE) method could be used to estimate the variance component of the genotypic effect, and the genotypic value of each accession could be unbiasedly predicted by adjusted unbiased prediction (AUP) method based on the variance component of the genotypic effect (Zhu 1993; Zhu and Weir 1996).
Constructing core collections
-
1.
Constructing core collections by least distance stepwise sampling (LDSS) strategy: first, a precise sampling percentage of the core collection to the initial collection is given based on other researches. Next, the genetic distances between accessions are calculated and accessions are grouped by hierarchical cluster analysis based on the genetic distance. One accession from a subgroup with the least distance (this subgroup is unique in the whole dendrogram) is randomly removed and another accession of the subgroup is sampled. Then, the genetic distances among the remained accessions are calculated again, and the sampling is performed by the same way. The stepwise samplings are performed until the percentage of the remained accessions reaches to the given sampling percentage. By this way, a core collection is successfully constructed.
-
2.
For comparing purpose, core collections by stepwise clusters with random sampling (SCR) strategy (Hu et al. 2000c) were constructed. The process is: first, the genetic distances among accessions of the initial collection are calculated. Next, accessions are grouped by hierarchical cluster analysis. One accession from each subgroup with two accessions at the lowest level of dendrogram is randomly sampled. Then, the genetic distances among the remained accessions are calculated again, which are used for the next procedure of cluster. The sampling is performed by the same way. The stepwise clusters are performed until the size of the remaining collection reaches the scale 20–30% (Yonezawa et al. 1995) of the initial collection. Thus, a core collection is successfully constructed.
Four distances (city block distance, Cityblock; Euclidean distance, Euclid; standardized Euclidean distance, Seuclid; Mahalanobis distance, Mahal) were used to assess genetic distances among accessions. Four hierarchical cluster methods (nearest distance method, Single; furthest distance method, Complete; unweighted pair-group average method, Average; and Ward’s method, Ward) were used to perform clustering to construct different core collections by combining four genetic distances.
The evaluating parameters for core collection
The representativeness of a core collection could be evaluated by mean, variance, range and coefficient of variation. A homogeneity test (F test) for variances and a t test for means (α = 0.05) can be performed to determine the difference of traits between core collection and the initial collection (Hu et al. 2000c). Based on the calculated results of t test, F test, range and coefficient of variation, four more important evaluating parameters are calculated. There are mean difference percentage (MD), variance difference percentage (VD), coincidence rate of range (CR) and variable rate of coefficient of variation (VR) (Hu et al. 2000b, c). These four parameters are formulated as follows:
-
\( {\text{MD}} = {\left( {\raise0.7ex\hbox{${S_{t} }$} \!\mathord{\left/ {\vphantom {{S_{t} } n}}\right.\kern-\nulldelimiterspace} \!\lower0.7ex\hbox{$n$}} \right)} \times 100, \) where S t is the number of traits which have significant difference (α = 0.05) of means between the initial collection and core collection; n is total number of traits.
-
\( {\text{VD}} = {\left( {\raise0.7ex\hbox{${S_{F} }$} \!\mathord{\left/ {\vphantom {{S_{F} } n}}\right.\kern-\nulldelimiterspace} \!\lower0.7ex\hbox{$n$}} \right)} \times 100, \) where S F is the number of traits which have significant difference (α = 0.05) of variances between the initial collection and core collection; n is total number of traits.
-
\( {\text{CR}} = \frac{1} {n}{\sum\limits_{i = 1}^n {\frac{{R_{{{\text{C}}(i)}} }} {{R_{{{\text{I}}(i)}} }} \times 100} }, \) where R C(i) is the range of the ith trait of core collection; R I(i) is the range of the corresponding trait of the initial collection; n is total number of traits.
-
\( {\text{VR}} = \frac{1} {n}{\sum\limits_{i = 1}^n {\frac{{{\text{CV}}_{{{\text{C}}(i)}} }} {{{\text{CV}}_{{{\text{I}}(i)}} }} \times 100} }, \) where CVC(i) is the coefficient of variation of the ith trait of core collection; CVI(i) is the coefficient of variation of the corresponding trait of the initial collection; n is total number of traits.
The core collection can be considered to represent the genetic diversity of the initial collection if MD ≤ 20% and CR ≥ 80% at the same time (Hu et al. 2000c). Moreover, in the same sampling percentage, smaller MD leads to more representative core collections, and core collections with larger CR or VR are more representative.
Simulations
In order to draw consistent, stable and reproducible results, repeated samples (bootstrap) were conducted (Chandra et al. 2002). Four hundred and twelve genotypes from the same growing region were selected among 1,547 cotton genotypes to perform simulations. There were k = 1,000, 1,500 and 2,000 independent random samples from the initial collection of a particular combination (a sampling percentage combining with a genetic distance and a cluster method). In each sample, the core collection was constructed and the four evaluating parameters above were calculated. Therefore, each combination generated four resampling populations of evaluating parameters when k = 1,000, 1,500 or 2,000. Based on the results of simulations, normality tests were performed and mean, median, upper-0.025-quantile and upper-0.975-quantile were calculated in each population.
The validation of core collections
Four hundred and twelve genotypes from the same growing region were treated by the principal components analysis to valid the core collections. Distribution of the core accessions and the reserved accessions was plotted by the first two principal components in the sampling percentage of 10 and 30%.
Data management
Before constructing core collections, genotypic values of each trait were standardized (μ = 0, σ = 1; where μ is the population mean of the trait and σ is the standard deviation of the trait). Normality tests were performed using Univariate procedure in SAS software (version 8.01). Other experiments were conducted in MATLAB software (version 6.5).
Results
Comparison between LDSS strategy and SCR strategy in the same sampling percentage
Constructing core collections from the 1,547 cotton genotypes in the same sampling percentage, the cluster times of LDSS strategy were far more than those of SCR strategy, and could be formulated as follows: cluster times = the number of initial accessions − the number of core accessions (Table 1). All MDs of core collections constructed by the two strategies were 0% and all CRs of core collections constructed by the two strategies were over 90%. Most VDs of core collection constructed by the two strategies were 0%. The CR and VR of core collections constructed by LDSS strategy were larger than those of core collections constructed by SCR strategy in the same combination (Table 1).
The representation of LDSS strategy in different cluster methods
When the same sampling percentage and genetic distance were used to construct core collections by LDSS strategy, the four cluster methods produced the same values for each evaluating parameters. By comparing the accessions in each core collection, all those four core collections with the same sampling percentage and genetic distance were composed of completely same accessions whether based on simulated data or true data. Table 2 showed the representation of LDSS strategy based on true data.
Comparison of genetic distances for LDSS strategy by simulation
The results were similar for k = 1,000, 1,500 and 2,000. Therefore, only results for k = 1,000 were listed and discussed. Normality tests showed that all resampling populations of evaluating parameters were not normal distribution. Therefore, median could be considered as estimate instead of mean, and upper-0.025-quantile and upper-0.975-quantile formed confidence interval at the significant level of 0.05 of the evaluating parameter. Since all cluster methods generated the same core collections under the same sampling percentage and genetic distance, the results of simulations on core collections constructed just by single-cluster method combining with four genetic distances were listed in present paper (Table 3). Except for Seuclid in 10% sampling percentage, all medians of MD were 0% and all medians of CR were over 85% (Table 3). Except for Mahal in the sampling percentage of 10%, all genetic distances had zero upper-0.025-quantile and the same upper-0.975-quantile of MD in all the three sampling percentages (Table 3). Compared to the two genetic distances of Cityblock and Euclid, Mahal and Seuclid generated larger median, upper-0.025-quantile and upper-0.975-quantile of VD, CR and VR in the same sampling percentage. Mahal generated slightly larger median, upper-0.025-quantile and upper-0.975-quantile of CR compared to Seuclid in the same sampling percentage, while those parameters of VR of Seuclid were larger than Mahal especially in small sampling percentage. Cityblock generated larger median, upper-0.025-quantile and upper-0.975-quantile of CR and VR in the same sampling percentage compared to Euclid (Table 3). Changes of VD for Cityblock and Euclid were similar (Table 3).
Validation of core collections by the principal components analysis
The above results suggested that core collections constructed by LDSS strategy were more representative than those constructed by SCR strategy, and Seuclid was more suitable for constructing core collections than Cityblock, Euclid and Mahal based on LDSS strategy. The principal component analysis was conducted further to validate core collections constructed by Seuclid based on LDSS strategy. Core accessions were selected symmetrically throughout the whole collection in all the two sampling percentages (Fig. 1). Most extreme accessions were selected in 10% sampling percentage and almost all those were selected in 30% sampling percentage (Fig. 1). The plots illustrated that the genetic diversity of the initial collection was organized in some degree. By LDSS strategy, only one accession was selected from each region with similar accessions, which avoided redundance efficiently (Fig. 1).
Discussion
For the research of constructing core collection, phenotypic values are mainly used (Zhang et al. 2000; Fundora et al. 2004; Volk et al. 2005). To achieve phenotypic values of germplasm materials, field experiments are required. Most traits of germplasm materials are quantitative traits under the control of polygenes, which means that they are easily affected by field conditions and experimental errors. Moreover, the effects of interaction between gene and environment (GE effects) exist in phenotypic values (Hu et al. 2000c). Therefore, stratification based on phenotypic values could not essentially reflect genetic relationship among accessions, and core collection based on phenotypic values may not accurately represent genetic diversity of the initial collection (Tanksley and McCouch 1997). Genotypic values could be predicted from phenotypic values by mixed linear model approach, which eliminate effects of experimental errors, environmental effects and GE effects. Stratification based on genotypic values can reflect genetic relationship among accessions more accurately. Therefore, a core collection constructed based on genotypic values will be more representative than that constructed based on phenotypic values (Hu et al. 2000c). Two types of mixed linear model were introduced in the present paper. One is suitable for analyzing experimental data in single environment; the other is suitable for analyzing experimental data in multiple environments. When genetic experiment is performed in multiple environments, the environmental effects and GE effects could be decomposed from the observed values by the mixed linear model described before, which leads to more precise predicting values of genotypic effects than in single environment. Therefore, performing genetic experiment in multiple environments will draw more accurate results in constructing core collections. In present research, core collections were constructed based on genotypic values from multiple environments genetic experiment.
The genetic diversity of a collection was not randomly dispersed but may be organized to varying degrees (Balakrishnan et al. 2000); the principal components analysis of present research proved it. Accessions from the same growing region have more similarity than those from different growing region. A population consisted of accessions with small genetic difference is more efficient to investigate the validity of different constructing strategies. Present results showed that the population size of 412 genotypes was available to evaluate different genetic distances. Moreover, the running time of the simulating program for LDSS strategy was too long to be afforded if the number of accessions were over 500, even in high-powered computers. Therefore, 412 genotypes from the same growing region were used in present research.
Both SCR strategy and LDSS strategy are based on hierarchical cluster. In the process of using SCR strategy to construct core collections, each procedure of sampling is performed in all subgroups at the lowest level of the dendrogram, and redundant accessions in these subgroups are removed. Different cluster methods will generate different subgroups at the lowest level of the dendrogram. However, the subgroup with the least distance is unique in the dendrogram, and all common used hierarchical cluster methods (nearest distance method, furthest distance method, centroid method, unweighted pair-group average method, weighted pair-group average method and Ward’s method) generate the same least distance subgroups (Yang et al. 1989). LDSS strategy performs sampling in the subgroup with the least distance of the dendrogram in each procedure of stepwise sampling. Therefore, given the same random sampling order, all hierarchical cluster methods will construct core collections with same accessions.
In general methods of constructing core collections by clusters, cluster method is one of the important factors that will affect the results of core collection. However, while using LDSS strategy, as long as the genetic distance and the sampling percentage were fixed, cluster methods need not be considered because of the properties of LDSS strategy. Serving for plant breeding is an important aim for constructing core collection. A well-representative core collection is an extremely useful resource for breeders, because it can save much expense and time in the course of plant breeding. Present results showed that constructing core collections by LDSS strategy with Seuclid distance seems to be an excellent strategy to assist constructing well-representative core collections.
References
Balakrishnan R, Nair NV, Screenivasan TV (2000) A method for establishing a core collection of Saccharum officinarum L.germplasm based on quantitative-morphological data. Genet Resour Crop Evol 47:1–9
Baranger A, Aubert G, Arnau G, Laine AL, Deniot G, Potier J, Weinachter C, Lejeune-Hénaut I, Lallemand J, Burstin J (2004) Genetic diversity within Pisum sativum using protein- and PCR-based markers. Theor Appl Genet 108:1309–1321
Brown AHD (1989) Core collection: a practical approach to genetic resources management. Genome 31:818–824
Chabane JCK, Valkoun J (2004) Characterisation of genetic diversity in ICARDA core collection of cultivated barley (Hordeum vulgare L.). Czech J Genet Plant Breed 40:134–136
Chandra S, Huaman Z, Hari Krishna S, Ortiz R (2002) Optimal sampling strategy and core collection size of Andean tetraploid potato based on isozyme data—a simulation study. Theor Appl Genet 104:1325–1334
Frankel OH (1984) Genetic perspectives of germplasm conservation. In: Arber WK, Llimensee K, Peacock WJ, Starlinger P (eds) Genetic manipulation: impact on man and society. Cambridge University Press, Cambridge, pp 161–170
Frankel OH, Brown AHD (1984a) Current plant genetic resources—a critical appraisal. In: Chopra VL, Joshi BC, Sharma RP, Bansal HC (eds) Genetics: new frontiers, vol 4. Oxford and IBH, New Delhi, pp 1–11
Frankel OH, Brown AHD (1984b) Plant genetic resources today: a critical appraisal. In: Hoden HW, Williams JT (eds) Crop genetic resources: conservation and evaluation. George Allen and Urwin, London, pp 249–257
Fundora MZ, Hernandez M, Lopez R, Fernandez L, Sanchez A, Lopez J, Ravelo I (2004) Analysis of the variability in collected peanut (Arachis hypogaea L.) cultivars for the establishment of core collections. Plant Genet Res Newsl 137:9–13
van Hintum ThJL (1995) Hierarchical approaches to the analysis of genetic diversity in crop plants. In: Hodgkin T, Brown AHD, van Hintum ThJL, Morales EAV (eds) Core collections of plant genetic resources. Wiley, Chichester, pp 23–34
Hu J, Xu HM, Zhu J (2000a) Constructing core collection of crop germplasm by multiple clusters based on genotypic values. J Biomath 15:103–109
Hu J, Xu HM, Zhu J (2000b) A method of constructing core collection reserving special germplasm materials. J Biomath 16:348–352
Hu J, Zhu J, Xu HM (2000c) Methods of constructing core collections by stepwise clustering with three sampling strategies based on the genotypic values of crops. Theor Appl Genet 101:264–268
Li CT, Shi CH, Wu JG, Xu HM, Zhang HZ, Ren YL (2004) Methods of developing core collections based on the predicted genotypic value of rice (Oryza sativa L.). Theor Appl Genet 108:1272–1176
Tanksley SD, McCouch SR (1997) Seed bank and molecular maps: Unlocking genetic potential from the wild. Science 277:1063–1066
Upadhyaya HD, Ortiz R (2001) A mini core subset for capturing diversity and promoting utilization of chickpea genetic resources in crop improvement. Theor Appl Genet 102:1292–1298
Volk GM, Richards CM, Reilley AA, Henk AD, Forsline PL, Aldwinckle HS (2005) Ex situ conservation of vegetatively propagated species: development of a seed-based core collection for Malus sieversii. J Am Soc Hortic Sci 130:203–210
Yang WQ, Liu LT, Lin HZ (1989) Multivariate statistical analysis. Higher Education Press, Beijing, pp 205–226
Yonezawa K, Nomura T, Morishima H (1995) Sampling strategies for use in stratified germplasm collections. In: Hodgkin T, Brown AHD, van Hintum ThJL, Morales EAV (eds) Core collections of plant genetic resources. Wiley, Chichester, pp 35–53
Zewdie Y, Tong NK, Bosland P (2004) Establishing a core collection of Capsicum using a cluster analysis with enlightened selection of accessions. Genet Resour Crop Evol 51: 147–151
Zhang X, Zhao Y, Cheng Y, Feng X, Guo Q, Zhou M, Hodgkin T (2000) Establishment of sesame germplasm core collection in China. Genet Resour Crop Evol 47:273–279
Zhang GY, Wang XF, Liu SJ, Ma ZY (2004) Cluster analysis and sampling methods for core collection construction in glandless cotton. Cotton Sci 16:8–12
Zhu J (1993) Methods of prediction genotype value and heterosis for offspring of hybrids. J Biomath 8:32–44
Zhu J, Weir BS (1996) Diallel analysis for sex-linked and maternal effects. Theor Appl Genet 92:1–9
Acknowledgment
The research was supported by the National Natural Science Foundation of China (No. 30270759).
Author information
Authors and Affiliations
Corresponding author
Additional information
Communicated by H. Becker.
Rights and permissions
About this article
Cite this article
Wang, J.C., Hu, J., Xu, H.M. et al. A strategy on constructing core collections by least distance stepwise sampling. Theor Appl Genet 115, 1–8 (2007). https://doi.org/10.1007/s00122-007-0533-1
Received:
Accepted:
Published:
Issue Date:
DOI: https://doi.org/10.1007/s00122-007-0533-1