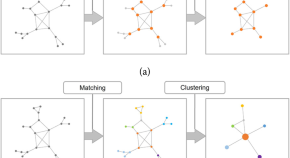
Collection
Intelligent Visualization and Visual Analytics
- Submission status
- Closed
Editors
-
Xiaoru Yuan
Xiaoru Yuan is a tenured faculty member in the School of Electronics Engineering and Computer Science. He received the Ph.D. degree in Computer Science in 2006, from the University of Minnesota at Twin Cities. His primary research interests are in the field of scientific visualization, information visualization and visual analytics. He has co-authored over 60 technical papers in IEEE Visualization, IEEE Information Visualization, IEEE TVCG, IEEE EuroVis, IEEE PacificVis and other major international visualization conference and journals.
-
Jun Tao
Jun Tao is an Associate Professor of Computer Science at Sun Yat-sen University and National Supercomputer Center in Guangzhou. He received a Ph.D. degree in Computer Science from Michigan Technological University in 2015, and worked as a postdoc researcher at the University of Notre Dame from 2015 to 2018. His major research interest is scientific visualization, especially on applying deep learning, information theory, and optimization techniques to interactive flow visualization and multivariate data exploration. He served on the program committees of IEEE VIS, IEEE PacificVis, ChinaVis, and other visualization conferences.
Articles (8 in this collection)
-
-
Understanding transitions in animated bar charts
Authors (first, second and last of 4)
- Datong Wei
- Can Liu
- Xiaoru Yuan
- Content type: Research
- Open Access
- Published: 10 July 2023
- Article: 13
-
Visualization facilitates uncertainty evaluation of multiple-point geostatistical stochastic simulation
Authors (first, second and last of 4)
- Qianhong Huang
- Qiyu Chen
- Zhesi Cui
- Content type: Research
- Open Access
- Published: 30 June 2023
- Article: 12
-
Re-understanding of data storytelling tools from a narrative perspective
Authors
- Pengkun Ren
- Yi Wang
- Fan Zhao
- Content type: Review
- Open Access
- Published: 25 June 2023
- Article: 11
-
Neural Monte Carlo rendering of finite-time Lyapunov exponent fields
Authors
- Yang Xi
- Wanna Luan
- Jun Tao
- Content type: Research article
- Open Access
- Published: 21 June 2023
- Article: 10
-
Reverse-engineering information presentations: recovering hierarchical grouping from layouts of visual elements
Authors (first, second and last of 5)
- Danqing Shi
- Weiwei Cui
- Nan Cao
- Content type: Research
- Open Access
- Published: 15 June 2023
- Article: 9
-
Visual analysis of inscriptions in the Tang Dynasty: a case study on the calligraphy style of Wang Xizhi
Authors (first, second and last of 7)
- Yang Zhang
- Ling Chen
- Guodao Sun
- Content type: Research article
- Open Access
- Published: 14 June 2023
- Article: 8
-
Colorslope: a balanced visualization of overview and details on ranks over time
Authors (first, second and last of 8)
- Hao Wang
- Xingyu Jiang
- Yingjie Chen
- Content type: Research
- Open Access
- Published: 01 June 2023
- Article: 7