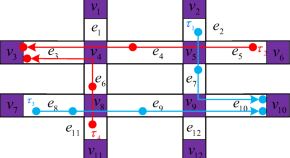
Collection
Special issue on spatiotemporal data management and analytics for recommender systems
- Submission status
- Closed
Recent advances in geo-positioning services and online social networks have fundamentally enhanced user experience in a variety of location-aware applications, including real-time route planning, online food ordering and delivery, location-aware crowdsourcing, and trip advisories. As such, one of a most popular and prominent research topics regarding location-aware applications is developing effective location-based recommender systems to enable users to acquire their preferred results in a timely manner over massive-scale spatiotemporal data.
In order to develop effective location-based recommender systems, the first step for data scientists is to get high-quality spatial data efficiently, which lay foundation for subsequent recommendations. Basically, spatiotemporal data is formulated by a single time-stamped spatial point or a sequence of time-stamped spatial points augmented with other information (e.g., textual description, attribute-value pairs). Some representative examples include Points of Interest (PoIs) and check-in data from online map services, user trajectories generated from wearable devices, and vehicle trajectories generated by online ride hailing applications. Thanks to the big data era and recent developments in spatiotemporal data management and recommender systems, research communities of recommender systems have been paying much attention towards developing effective recommender systems based on spatiotemporal data acquired from various location-aware applications. As such, users are able to be fed with a variety of recommendation results that may satisfy their location-based preferences. However, how to cope with spatiotemporal data streams of multisource and multimodality to maximize their usability to recommender systems while ensuring privacy and reliability is still an open challenge.
Analytics of multisource and multimodal spatiotemporal data enable us to extract timely information and knowledge that are useful to recommender systems, which may further improve the effectiveness, reliability, and efficiency of various recommender systems. This special issue aims to develop effective spatiotemporal data management techniques, novel spatiotemporal database frameworks, multisource streaming data processing techniques, privacy-preserving spatial data analytics, and location-aware query authentication to establish a variety of effective and efficient location-based recommender systems.
Topics of Interest:Topics include, but are not limited to, the following:
-Spatiotemporal data preprocessing, including data cleaning, feature selection and extraction, data clustering, and map-matching
-Deep learning/reinforcement learning/federated learning on spatiotemporal data
-Spatiotemporal data mining
-Next-generation location-based recommender systems
-Multisource data stream analytics
-Effective processing of multimodal data
-Optimization of location-based recommender systems
-Location-based services and location-based social networks
-Privacy-preserving management of spatial data
-Location-aware query results authentications
Guest Editors:Prof. Shuo Shang (jedi.shang@gmail.com), Professor, University of Electronic Science and Technology of China (Managing Guest Editor)
Prof. Xiangliang Zhang (xzhang33@nd.edu), Associate Professor, University of Notre Dame, USA
Prof. Panos Kalnis (panos.kalnis@kaust.edu.sa), Professor, King Abdullah University of Science and Technology, Saudi Arabia
Important datesSubmissions due: extended to 30 June, 2022
First round of reviews: 30 August, 2022
Author revision deadline: 30 October, 2022
Final decision notification: 30 December, 2022
Editors
-
Shuo Shang
Prof. Shuo Shang (jedi.shang@gmail.com), Professor, University of Electronic Science and Technology of China
-
Prof. Xiangliang Zhang
Prof. Xiangliang Zhang (xzhang33@nd.edu), Associate Professor, University of Notre Dame, USA
-
Prof. Panos Kalnis
Prof. Panos Kalnis (panos.kalnis@kaust.edu.sa), Professor, King Abdullah University of Science and Technology, Saudi Arabia
Articles (18 in this collection)
-
-
Continuous spatial keyword search with query result diversifications
Authors
- Ying Zhong
- Jianmin Li
- Shunzhi Zhu
- Content type: OriginalPaper
- Published: 25 November 2022
- Pages: 1935 - 1948
-
PreCLN: Pretrained-based contrastive learning network for vehicle trajectory prediction
Authors (first, second and last of 5)
- Bingqi Yan
- Geng Zhao
- Junyu Dong
- Content type: OriginalPaper
- Published: 17 November 2022
- Pages: 1853 - 1875
-
GCMT: a graph-contextualized multitask spatio-temporal joint prediction model for cellular trajectories
Authors (first, second and last of 4)
- Yu Sang
- Yuan Xu
- Zhenping Xie
- Content type: OriginalPaper
- Published: 12 October 2022
- Pages: 1649 - 1665
-
HMGCL: Heterogeneous multigraph contrastive learning for LBSN friend recommendation
Authors (first, second and last of 6)
- Yongkang Li
- Zipei Fan
- Xuan Song
- Content type: OriginalPaper
- Published: 06 October 2022
- Pages: 1625 - 1648
-
Maximizing the influence of bichromatic reverse k nearest neighbors in geo-social networks
Authors (first, second and last of 7)
- Pengfei Jin
- Lu Chen
- Christian S. Jensen
- Content type: OriginalPaper
- Published: 03 October 2022
- Pages: 1567 - 1598
-
Spatiotemporal contrastive modeling for video moment retrieval
Authors (first, second and last of 6)
- Yi Wang
- Kun Li
- Meng Wang
- Content type: OriginalPaper
- Published: 26 September 2022
- Pages: 1525 - 1544
-
Spatial-temporal fusion graph framework for trajectory similarity computation
Authors (first, second and last of 5)
- Silin Zhou
- Peng Han
- Xiangliang Zhang
- Content type: OriginalPaper
- Published: 22 September 2022
- Pages: 1501 - 1523
-
Distributed processing of spatiotemporal ocean data: a survey
Authors (first, second and last of 10)
- Xiaoyong Li
- Jingyun Gu
- Zichen Xu
- Content type: OriginalPaper
- Published: 20 September 2022
- Pages: 1481 - 1500
-
Towards robust trajectory similarity computation: Representation-based spatio-temporal similarity quantification
Authors (first, second and last of 5)
- Ziwen Chen
- Ke Li
- Shuo Shang
- Content type: OriginalPaper
- Published: 09 August 2022
- Pages: 1271 - 1294
-
Multi-source and heterogeneous marine hydrometeorology spatio-temporal data analysis with machine learning: a survey
Authors (first, second and last of 6)
- Song Wu
- Xiaoyong Li
- Zichen Xu
- Content type: Research
- Published: 08 July 2022
- Pages: 1115 - 1156
-
Self-supervised end-to-end graph local clustering
Authors
- Zhe Yuan
- Content type: OriginalPaper
- Published: 08 July 2022
- Pages: 1157 - 1179
-
CDAML: a cluster-based domain adaptive meta-learning model for cross domain recommendation
Authors (first, second and last of 4)
- Jiajie Xu
- Jiayu Song
- Lihua Yin
- Content type: OriginalPaper
- Published: 11 June 2022
- Pages: 989 - 1003
-
Graph-aware collaborative reasoning for click-through rate prediction
Authors
- Xin Zhang
- Zengmao Wang
- Bo Du
- Content type: OriginalPaper
- Published: 08 June 2022
- Pages: 967 - 987
-
Continuous similarity join over geo-textual data streams
Authors
- Hongwei Liu
- Yongjiao Sun
- Guoren Wang
- Content type: OriginalPaper
- Published: 02 June 2022
- Pages: 933 - 947
-
Continuous spatial keyword query processing over geo-textual data streams
Authors
- Hongwei Liu
- Yongjiao Sun
- Guoren Wang
- Content type: OriginalPaper
- Published: 11 May 2022
- Pages: 889 - 903
-
A fault-tolerant optimization mechanism for spatiotemporal data analysis in flink
Authors (first, second and last of 6)
- Hangxu Ji
- Gang Wu
- Yuchen Fan
- Content type: OriginalPaper
- Published: 04 April 2022
- Pages: 867 - 887
-
Learning to effectively model spatial-temporal heterogeneity for traffic flow forecasting
Authors (first, second and last of 7)
- Minrui Xu
- Xiyang Li
- Jiajie Xu
- Content type: OriginalPaper
- Published: 31 March 2022
- Pages: 849 - 865