Abstract
The determination of the properties of bio composites is the key subject of current research. The mechanical characteristics of these new materials depend on several factors, we can note the characteristics of the constituents, the process followed during the manufacture, etc. Several solutions have been made available to determine these properties such as experience tests, the methods of homogenization and the finite element method. Currently, thanks to artificial intelligence, smart solutions have been able to give better results, particularly in the prediction of the mechanical properties of materials. In this article, we will use artificial neural networks to predict the Young’s modulus of polypropylene loaded at 15% with horn fibers. Using Mori–Tanaka’s model, which is a form of the homogenization method, we will generate a dataset to feed our feed forward back propagation and demonstrate that this bio composite is gaining in terms of elasticity with a very good value of coefficient regression, 099, and with a best validation of performance, 0.034737 obtained at epoch 11.
Access this chapter
Tax calculation will be finalised at checkout
Purchases are for personal use only
Similar content being viewed by others
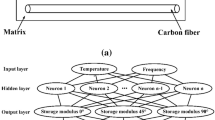
References
Li, X., Liu, Z., Cui, S., Luo, C., Li, C., Zhuang, Z.: Predicting the effective mechanical property of heterogeneous materials by image based modeling and deep learning. Comput. Methods Appl. Mech. Eng. 347, 735–753 (2019). https://doi.org/10.1016/j.cma.2019.01.005
Ye, S., Li, B., Li, Q., Zhao, H.P., Feng, X.Q.: Deep neural network method for predicting the mechanical properties of composites. Appl. Phys. Lett. 115(16) (2019). https://doi.org/10.1063/1.5124529
Sun, Q., Ertekin, T.: Screening and optimization of polymer flooding projects using artificial-neural-network (ANN) based proxies. J. Pet. Sci. Eng. 185, 106617 (2020)
Hassan, A.K.F., Jassim, R.J., Jafaar, M.M.: Neural network and genetic algorithm based finite element model for optimal die shape design in Al-1100 cold forward extrusion. Univers. J. Mech. Eng. 4(2), 25–33 (2016). https://doi.org/10.13189/ujme.2016.040202
Balokas, G., Czichon, S., Rolfes, R.: Neural network assisted multiscale analysis for the elastic properties prediction of 3D braided composites under uncertainty. Compos. Struct. 183, 550–562 (2018)
Chohan, J.S., et al.: Mechanical strength enhancement of 3D printed acrylonitrile butadiene styrene polymer components using neural network optimization algorithm. Polymers (Basel) 12(10), 2250 (2020)
Abueidda, D. W., Almasri, M., Ammourah, R., Ravaioli, U., Jasiuk, I. M., Sobh, N.A.: Prediction and optimization of mechanical properties of composites using convolutional neural networks. Compos. Struct. 227, 111264 (2019). https://doi.org/10.1016/j.compstruct.2019.111264
Mondol, J.A.M., Panigrahi, S., Gupta, M.M.: Neural networks approach to biocomposites processing. Proceedings of IEEE Pacific Rim Conference on Communications Computers and Signal Processing—Proceedings, Aug 2011, pp. 742–746 (2011). https://doi.org/10.1109/PACRIM.2011.6032986
Su, H., Qi, W., Yang, C., Sandoval, J., Ferrigno, G., De Momi, E.: Deep neural network approach in robot tool dynamics identification for bilateral teleoperation. IEEE Robot. Autom. Lett. 5(2), 2943–2949 (2020)
Fardioui, M., Guedira, T., Qaiss, A.E.K., Bouhfid, R.: A comparative study of Doum fiber and Shrimp Chitin based reinforced low density polyethylene biocomposites. J. Polym. Environ. 26(2), 443–451 (2018). https://doi.org/10.1007/s10924-017-0955-z
Moumen, A., Jammoukh, M., Zahiri, L., Mansouri, K.: Numerical modeling of the thermo mechanical behavior of a polymer reinforced by horn fibers. Int. J. 9(4) (2020)
Lakhdar, A., Moumen, A., Zahiri, L., Jammoukh, M., Mansouri, K.: Experimental and numerical study of the mechanical behavior of bio-loaded PVC subjected to aging. Adv. Sci. Technol. Eng. Syst. 5(5), 607–612 (2020). https://doi.org/10.25046/AJ050574
Chen, Z., Xie, Y.M., Wang, Z., Li, Q., Wu, X., Zhou, S.: A comparison of fast Fourier transform-based homogenization method to asymptotic homogenization method. Compos. Struct. 238, 111979 (2020)
Moumen, A., Lakhdar, A., Jammoukh, M., Zahiri, L., Mansouri, K.: Optimization of the mechanical and morphological properties of polypropylene bio-loaded by Argan nut shell particles with different theoretical and numerical models. In: 2020 IEEE 2nd International Conference on Electronics, Control, Optimization and Computer Science (ICECOCS), pp. 1–6 (2020)
Moumen, A., Jammoukh, M., Zahiri, L., Mansouri, K.: Study Of the optimal micromechanical behavior of a polymer reinforced by snail shell particles using the Mori-Tanaka numerical model. In: 2020 IEEE International Conference of Moroccan Geomatics (Morgeo), pp. 1–6 (2020)
Lakhdar, A., Moumen, A., Zahiri, L., Jammoukh, M., Mansouri, K.: Experimental and numerical study of the mechanical behavior of bio-loaded PVC subjected to aging. Adv. Sci. Technol. Eng. Syst. J. 5(5), 607–612 (2020)
Moumen, A., Lakhdar, A., Mansouri, K.: Numerical study of the mechanical behavior of polyamide 66 reinforced by Argan nut shell particles with the finite element method and the Mori-Tanaka model. Int. J. Adv. Trends Comput. Sci. Eng. 9(5), 7723–7730 (2020). https://doi.org/10.30534/ijatcse/2020/115952020
Author information
Authors and Affiliations
Editor information
Editors and Affiliations
Rights and permissions
Copyright information
© 2022 The Author(s), under exclusive license to Springer Nature Singapore Pte Ltd.
About this paper
Cite this paper
Laabid, Z., Moumen, A., Lakhdar, A., Mansouri, K. (2022). Toward the Prediction of the Elasticity of Bio Loaded Polypropylene Using Artificial Neural Networks. In: Saeed, F., Al-Hadhrami, T., Mohammed, E., Al-Sarem, M. (eds) Advances on Smart and Soft Computing. Advances in Intelligent Systems and Computing, vol 1399. Springer, Singapore. https://doi.org/10.1007/978-981-16-5559-3_5
Download citation
DOI: https://doi.org/10.1007/978-981-16-5559-3_5
Published:
Publisher Name: Springer, Singapore
Print ISBN: 978-981-16-5558-6
Online ISBN: 978-981-16-5559-3
eBook Packages: Intelligent Technologies and RoboticsIntelligent Technologies and Robotics (R0)