Abstract
We illustrate how atomistic simulations can complement experimental efforts in the bottom-up synthesis of graphene-based nanostructures on noble metal surfaces. After a brief introduction to the field, we review the state of the art of relevant computational methods. We then proceed by example through questions related to adsorption and diffusion, reactions and electronic structure, indicating both the strengths and limitations of computational approaches.
Access provided by Autonomous University of Puebla. Download conference paper PDF
Similar content being viewed by others
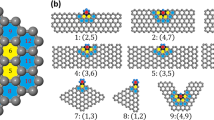
Keywords
- Density Functional Theory
- Scanning Tunneling Microscopy Image
- Molecular Precursor
- Zigzag Edge
- Scanning Tunneling Spectroscopy
These keywords were added by machine and not by the authors. This process is experimental and the keywords may be updated as the learning algorithm improves.
1 Introduction
In graphene-based nanotechnology, the motivation to explore bottom-up approaches emerges from a need for atomic precision that cannot be satisfied by traditional top-down fabrication methods.
Graphene, “a flat monolayer of carbon atoms tightly packed into a two-dimensional (2D) honeycomb lattice” [1], is a material with many extraordinary properties [2, 3]. From the perspective of applications in electronic switching devices, there is first of all the exceptional electron mobility that has enabled switching speeds exceeding 400 GHz [4]. Furthermore, the two-dimensional nature of graphene is beneficial to the electrostatics in transistor configurations, allowing to move source and drain contacts very close together before adverse short-channel effects set in [5]. And last, but not least, graphene is mechanically flexible, making it a promising candidate for applications in high-frequency organic electronics on flexible substrates. When it comes to room temperature digital logic applications, however, sufficient on-off ratios can be achieved only by introducing a sizeable band gap into the electronic structure of the semimetal graphene [5]. And one way of achieving this goal is by quantum confinement at the nanoscale.
There are different classes of nanostructures in this category. Prominent examples are stripes of graphene with widths in the low nm range, termed graphene nanoribbons (GNRs), but also their rolled-up counterparts, the single-wall carbon nanotubes (SWCNTs). While this chapter is dedicated to GNRs and other flat graphene nanostructures, we point out that on-surface chemistry is relevant also to the field of SWCNTs, where the surface-supported synthesis of specifically designed end-caps has recently enabled the controlled fabrication of monodisperse SWCNTs for the first time [6].
An obvious difference between GNRs and SWCNTs is that GNRs have edges, and it has been predicted early on that the atomic structure of the edges plays an important role in determining their electronic properties [58]. Therefore, the limited control over edge roughness and edge passivation in top-down approaches, such as lithography and etching [7], sonochemical cutting and breaking [8], or even unzipping of carbon nanotubes [9] seriously hampers the production of GNRs with reliable electronic transport characteristics. But while the need for atomically smooth edges seems to call for a bottom-up strategy, traditional solution-based polymerization chemistry faces a solubility problem, when macromolecules containing several hundreds to thousands of atoms are to be synthesized.
This is, where on-surface chemistry has come in to play, following pioneering works in the years 2007–2009 that described the surface-supported covalent assembly of networks and conjugated molecular wires [10–12]. Figure 1 summarizes the bottom-up approach for the synthesis of GNRs developed in collaboration between the nanotech@surfaces Laboratory at Empa, led by Roman Fasel, and the Department for Synthetic Chemistry at the Max Planck Institute for Polymer Research, led by Klaus Müllen [13]. The starting point is a molecular precursor that is designed specifically to yield a particular GNR. Figure 1 shows the 10,10′-dibromo-9,9′-bianthryl (DBBA) molecule, which yields an armchair graphene nanoribbon of width N = 7 (7-AGNR). The molecular precursor is then deposited onto a noble metal substrate under UHV conditions. Upon annealing at a characteristic temperature T 1, the molecules undergo dehalogenation and the radical intermediates start diffusing across the surface. When the radicals meet, they self-assemble into flexible polymer chains via aryl–aryl coupling, similar to the classical Ullmann reaction [14]. Finally, annealing at a temperature T 2 > T 1 activates the cyclodehydrogenation reaction, which transforms the polymers into planar GNRs.
Basic steps of surface-supported GNR synthesis. Top Dehalogenation of precursor monomers (here, 10,10′-dibromo-9,9′-bianthryl) after deposition on noble metal substrate. Center Formation of linear polymers by covalent coupling of diffusing dehalogenated intermediates. Bottom Formation of fully aromatic GNRs (here, 7-AGNR) by cyclodehydrogenation. Figure and caption reproduced with permission from Macmillan Publishers Ltd: [13], Copyright (2010)
While on-surface chemistry has proven to deliver a framework that works, this new route is not without its challenges. In particular, approaching the design of suitable precursors on the basis of trial and error risks substantial waste of resources both during precursor synthesis and subsequent on-surface coupling trials. Strong computational support can therefore be of great value, not only in order to avoid synthesis routes that are predicted to fail or to provide products with unwanted properties, but also in aiding the interpretation of microscopy and spectroscopy data as well as in unraveling the underlying reaction mechanisms, allowing for the systematic optimization of processing parameters.
In order to be successful at this ambitious task, atomistic calculations must reach appropriate levels of precision in describing the following aspects:
-
(I)
The closely related processes of adsorption and diffusion on the surface. This concerns questions about preferred adsorption sites, adsorption energies, the cleaving of weaker bonds (activating precursors for the reaction with other molecules), and diffusion coefficients.
-
(II)
The chemical reaction between molecules. This entails the prediction of reaction paths and activation energies that determine the required annealing temperatures, possibly including limiting factors such as the presence of hydrogen on the surface.
-
(III)
The electronic properties of the reaction product. Of interest are both its intrinsic properties in order to judge its potential for applications and the influence of the nearby surface in order to enable comparison with microscopy and spectroscopy experiments. Important figures of merit include the fundamental and optical gap, the density of states (band structure) and the optical absorption spectrum.
After an overview of the relevant tools offered by state-of-the-art atomistic simulations, the remaining sections of this chapter will address each of the above aspects through recent examples from the field of graphene-based nanostructures, illustrating how experiment and theory can join forces on the path toward a nanodesign strategy that is reliable, accurate, and efficient at the same time.
2 Computational Methods
The study of molecules on surfaces represents an interface between two well-established fields of computational research. Molecules traditionally are considered the domain of quantum chemistry, meaning wave-function-based methods with an underlying variational principle, using localized basis sets. Solids and their surfaces on the other hand are the domain of computational solid-state physics, with methods such as density functional theory (DFT) [15] using plane-wave basis sets with periodic boundary conditions. Combining molecules and surfaces thus requires some level of choice and compromise.
When choosing to adopt periodic boundary conditions, a typical unit cell is shown in Fig. 2. The molecule is supported by a slab of several atomic layers of the substrate. Spurious interactions between periodic replica of the molecule are minimized by increasing the lateral dimensions of the unit cell, while spurious interactions between slabs are avoided by introducing sufficient amounts of vacuum. Starting from a precursor molecule consisting of several tens of atoms, the unit cell may contain several hundreds, sometimes thousands of atoms. Systems of this size are currently out of reach for most quantum chemistry methods, and they remain challenging even for the most efficient DFT codes commonly used for large-scale ab initio simulations, such as CPMD, CP2K, or SIESTA [16–18].
Top and side view of slab geometry adapted from [19]. The cyclohexa-o-p-o-p-o-p-phenylene (CopP) molecule is supported by a Cu(111) slab of four atomic layers. While the molecule itself consists of 60 atoms, the unit cell (black lines) contains 420 atoms
In the following, we describe different ways of modeling the interaction between molecule and surface and their consequences for the simulation of on-surface chemistry (see also [20] for a recent review on the topic). The last part of the section is dedicated to the prediction of the valence electronic structure of graphene-based nanomaterials, both on a qualitative level and with the goal of comparing to (inverse) photoemission, tunneling, or optical absorption spectroscopy. For ab initio calculations of core-level [21, 22], infrared [23] and Raman spectra [23–25], we point the interested reader to the corresponding references.
2.1 Physisorption
If the molecule–substrate interactions are dominated by dispersion forces, a combination of quantum mechanics and molecular mechanics (QM/MM) [26, 27] can be considered. In the example illustrated by Fig. 3, the metallic substrate is treated within the empirical embedded atom method (EAM) [28]. The molecule is described by standard Kohn–Sham DFT [15, 29] and interacts with the substrate through an atomic pair potential [30] giving rise to an interaction energy
where the sum goes over all atoms A of the molecule and B of the slab. The first two terms describe the Pauli repulsion due to valence and core electrons of the metal substrate, while the third term describes the attractive dispersion force. The considerable computational advantage of such a QM/MM approach provides access to the dynamics of the system, allowing for an unbiased search for possible reaction paths. If the process of interest involves significant energy barriers, making it a “rare event,” the molecular dynamics need to be accelerated.
Schematic representation of the QMMM approach used in [31] to study the cyclodehydrogenation of CopP on a Cu(111) substrate. While the physisorbed molecule is described within DFT, the substrate is modeled with the EAM. The two systems are coupled via an empirical potential [30] mimicking van der Waals attraction and Pauli repulsion
In the case of direct metadynamics [32, 33], the system begins moving freely, but over time is biased against revisiting configurations that have already been explored. The repulsive bias potential is placed not on the set of atomic positions x visited by the system, but on the corresponding values of a limited number of collective variables (CVs) \(S_{\alpha } \left( x \right), \;\alpha = 1 \ldots d\), thereby drastically reducing the dimensionality of the problem. In typical applications, d = 2 or d = 3, and the CVs describe distances, angles, or coordination numbers that are deemed important indicators of the reaction of interest. By keeping track of the bias potential deposited during the time evolution of the system, the free energy surface can be constructed in the space of the collective variables.
We note that while the concept of collective variables is very flexible [34], finding adequate CVs for complex reactions can be delicate and attempts have been made to automate this process [35]. For a recent review, we refer interested readers to [36].
2.2 Chemisorption
In many cases, bond formation between the molecule and the substrate is an essential ingredient of the reaction. Since no empirical potentials are available that accurately model the formation of metal–organic bonds, a complete quantum mechanical description of the system is then the only option. Kohn–Sham DFT using (semi-)local approximations to the exchange-correlation functional has a very successful track record in applications of dense matter and covalently bound molecules. However, it was recognized early on that this type of approximation is, by construction, unable to capture the non-local nature of dispersion forces [37]. Since these forces are a crucial component of the interaction between molecules and surfaces, we discuss three common approaches to ameliorate the problem (for more details see [38]).
To some degree, it is possible to adapt semi-local exchange-correlation functionals to emulate dispersion effects at near-equilibrium atomic distances. At the expense of higher computational complexity, further improvements can be made by admixing a portion of the non-local Hartree–Fock exchange (“hybrid functionals”) and including the second derivative of the density as a variable (“meta functionals”) [39]. Nevertheless, a (semi-)local approximation of the correlation energy ultimately leads to an exponential decay of the interaction for large atomic separations [37]. These functionals can therefore not be expected to adequately describe systems such as large molecules or molecules on surfaces, if interactions over larger distances are important.
Another class of dispersion corrections approaches the problem in a semi-classical manner, neglecting all knowledge of the electron density and formulating the dispersion interaction in terms of an interatomic potential [38]:
Here, AB runs over all pairs of atoms, \(R_{AB}\) is their distance and \(C_{n}^{AB}\) denotes the averaged (isotropic) nth-order dispersion coefficient between atoms of the corresponding kind, which can be computed ab initio. This dispersion energy is then simply added to the total DFT energy, thus ensuring the asymptotic form \(1/(R_{AB} )^{6}\) of the interaction. In order to avoid double-counting of correlation, the dispersion interaction is damped at short distances by a continuous function \(f_{\text{damp}} (R_{AB} )\), where details of the damping function as well as the scaling factors \(s_{n}\) may be used to adapt to particular (semi-)local density functionals. Extensions of the scheme include the treatment of three-body interactions [40] as well as the effects of the hybridization state on the dispersion coefficients, based on fractional occupation numbers [40] or atomic volumes [41]. This class of corrections delivers a computationally cheap way to add dispersion forces on top of various (semi-)local exchange-correlation functionals, providing, in the case of DFT-D3, dispersion coefficients for the first 94 elements of the periodic table [40]. While this has lead to a widespread adoption, some criticism remains. The first concerns the dispersion coefficients, which need to be determined on one or several reference systems, be it computationally or experimentally. These are traditionally chosen to be either the bare elements or small molecules containing the elements in question [40], which is suitable for applications in molecular systems, but provides an electronic environment that can be very different from bulk materials. For the particular application of molecules on coinage metal surfaces, a scheme has been proposed to include screening and polarization effects at the level of atomic pairwise interactions [42]. The second and perhaps more worrying remark concerns the finding that molecular binding energies in many cases depend more sensitively on the empirical parameterization of the damping function than on the physically sound dispersion coefficients [43].
A third approach that has gained popularity in recent years is a density-based treatment of the non-local correlation energy, which takes the form
The kernel \(\phi\) is derived from first principles using a plasmon pole approximation to the local dielectric function and is a function of \(|r - r^{{\prime }} |\), the electron density n and its gradient at positions \(r,r^{{\prime }}\) [44, 45]. Treated self-consistently, the variational derivative \(V_{c}^{nl} (r) = \delta E_{c}^{nl} /\delta n(r)\) modifies the exchange-correlation potential \(V_{xc} = V_{xc}^{l} + V_{c}^{nl}\) and thus acts directly on the Kohn–Sham wave functions. One significant advantage over DFT-D methods is therefore that the effect of the electronic environment, including charge transfer, on dispersion interactions is captured in a general and physically sound manner [38]. Contrary to DFT-D methods, the non-local correlation term is not damped at short distances, meaning that particular care needs to be taken in selecting the (semi)-local exchange-correlation functional in order to avoid double-counting of correlation. Besides existing functionals, such as PW86 [45], some have thus been designed specifically for this purpose [46]. Regarding computational cost, linear scaling algorithms are available [47] that render the overhead of these so-called van der Waals density functionals negligible as compared to standard semi-local calculations.
Using DFT with dispersion corrections, the calculation of adsorption geometries and adsorption energies is feasible for systems containing several hundred [48] to more than thousand atoms. Dynamics and thus free energy profiles are typically out of reach. If the initial and final states of a process are known, the pioneering methods of Elber and coworkers [49], the nudged elastic band (NEB) [50, 51], and string [52] methods can be used to compute 0K reaction barriers and provide a description that can afterward be extended to finite-temperature free energy effects [53, 54].
As illustrated by Fig. 4, the NEB method requires not only the knowledge of the initial (green bead) and the final states (red bead), but also an initial guess for the reaction path that connects the two (white beads). After optimization of the “elastic” band, the intermediate configurations, also known as “images,” follow the minimum energy profile and one image (black bead) has climbed to the saddle point [51]. In simple cases, a first guess for the images is obtained by linear interpolation between initial and final coordinates or a sequence of constrained geometry optimizations. However, the initial guess constitutes a strong bias of the overall picture of the reaction and in general different paths have to be considered (on top of the possibility of different final states).
Pictorial representation of a NEB calculation describing a dehydrogenation step in the synthesis of tribenzo[a,g,m]coronene. Left panel Initial guess of the reaction path from initial state (green bead) to final state (red bead) on the potential energy surface. Right panel After optimization, the intermediate configurations (white beads) follow the minimum energy profile. One configuration (black bead) has climbed to the saddle point
2.3 Electronic Structure
Since the synthesis of graphene-based nanostructures is often aimed at electronics applications, a reliable prediction of the intrinsic electronic structure of different possible targets is very useful. The main focus tends to lie on the \(sp^{2}\)-hybridized carbons and their \(\pi\)-electronic structure. Some insights can be gained already with pen and paper, using intuitive chemistry rules such as Clar’s theory of the aromatic sextet [55]. These include qualitative information about the \(\pi\)–electron distribution, bond-length alternation and, in the case of zigzag edges, the presence of edge-localized unpaired electrons [56].
The next level of understanding is provided by tight-binding models. Considering only the \(\pi\)-electrons, the system is described by a Hamiltonian
where \(t \approx 3\) eV is the hopping integral, \(a_{i}^{\dag } ,a_{i}\) are the electron creation and annihilation operators at carbon site i and the sum runs over nearest neighbors [57, 58]. In the case of armchair and zigzag GNRs, this model can be solved analytically [59, 60], allowing for in-depth investigations of the width- and edge-dependence of the electronic band structure. Further physics can be introduced by including site-dependent potentials [61], hopping between 3rd-nearest neighbors [62], Coulomb interactions between electrons [63] or larger basis sets [64]. These models, however, need to be parameterized and are typically fitted to band structures calculated ab initio.
Kohn–Sham DFT with (semi-)local exchange-correlation functionals provides qualitatively correct answers to many relevant questions, including band ordering, band gap hierarchy, band dispersion, shape of wave functions, and spin polarization [65–67]. This makes Kohn–Sham DFT suitable for comparing the electronic structure of different nanostructures. Still, direct comparison with experiments is challenging, even at this level. One area, where DFT can be of great value, is the interpretation of scanning tunneling microscopy (STM) images. The shape of the Kohn–Sham orbitals is typically very accurate [68] and adequate adsorption geometries can be obtained by including the substrate together with a suitable dispersion correction. For a quantitative prediction of spectroscopic information, however, the independent-particle approximation is too simplistic. Not only does the Kohn–Sham gap significantly underestimate the band gap of bulk semiconductors [68], the discrepancy is amplified in low-dimensional materials, such as the quasi-one-dimensional GNRs or carbon nanotubes, where screening of the Coulomb interaction between electrons is strongly reduced [65].
One approach to tackle the problem is many-body perturbation theory (MBPT), in which the strongly interacting electrons are replaced by the concept of weakly interacting quasi-particles. In the form of the GW approximation [69], MBPT has provided very accurate quasi-particle band gaps of bulk materials [70] and is increasingly applied also to graphene-based nanomaterials [65, 71]. While we refer interested readers to [72] for an extensive introduction of the underlying theory, we stress one important fact: In nanostructures, where the Coulomb interaction is screened only weakly, the energy gap extracted from spectroscopy experiments strongly depends on the nature of the excitation. In the case of (inverse) photoemission spectroscopy and scanning tunneling spectroscopy (STS), the system under study is promoted from an N-electron ground state to an excited state with N ± 1 electrons. The fundamental gap associated with these charged excitations is defined as
where \(IP\) (\(EA\)) denote the ionization potential (electron affinity) of the system and \(E(N)\) the ground-state energy of a system with \(N\) electrons. \(\Delta\) coincides with the quasi-particle gap in the GW approximation. In optical absorption experiments, using techniques such as reflectance anisotropy spectroscopy, electron–hole pairs are created and the number of electrons is conserved. Within MBPT, these neutral excitations are described by the Bethe–Salpeter equation [73], often using the GW electronic structure as a starting point. The resulting optical gap is reduced with respect to the fundamental gap by the binding energy of the lowest-lying exciton. In graphene-based nanostructures, this energy can be substantial, even exceeding 1 eV [71, 74, 75].
Finally, additional complications arise from the presence of the nearby substrate, which significantly increases the screening of the Coulomb interaction with respect to the nanostructures in vacuum. The computational cost of GW calculations scales with \(M^{3} - M^{4}\), where M is the number of atoms in the system [76], making systems with M > 100 extremely challenging to handle. Full GW calculations of molecules on surfaces have thus far concentrated on small molecules and correspondingly small surface unit cells [77, 78, 81] and/or thin supporting substrates [79]. Alternatively, substrate effects may be included into GW calculations of the isolated molecule via classical image charge models [77, 80–82].
3 Adsorption and Diffusion
Once the molecular precursors are adsorbed on a metallic substrate, the factors influencing their diffusion are the surface geometry (the presence of steps, corrugations, defects, or adatoms), the surface–molecule interactions (dominated either by dispersion forces or by chemical bonding) and the molecule–molecule interactions. The study of diffusion mechanisms starts from the identification of stable adsorption sites and requires a systematic verification of all geometrically possible and chemically sound adsorption sites by means of geometry optimization. This analysis can be performed at different levels of theory, the system size typically being a limiting factor for beyond-DFT methods.
Some general insight into molecular self-assembly can be obtained from simple models, such as the “patchy-disk” model [83], accounting only for the geometry and the strength of the interaction. As illustrated in Fig. 5, Whitelam et al. concentrate on threefold-coordinated building blocks, which are represented as disks with angular domains that either allow for bond formation (green) or do not (blue). Bond strength and bond flexibility are characterized by the interaction energy \(-\epsilon\) and the opening angle \(2\omega\) of the interacting stripes. Varying only these two parameters within Monte Carlo simulations, Whitelam et al. are able to reproduce the characteristics of networks ranging from graphene to DNA-based polymers with building blocks spanning three orders of magnitude in length scale.
“Patchy-disk” model of threefold-coordinated building blocks. In equilibrium, the periodic honeycomb network [84] (A) or a non-periodic polygon network [85] (E) can be formed. Out-of-equilibrium structures include honeycomb polycrystals [86] (B), polygon networks that can evolve to the honeycomb [87] (C), or kinetically trapped polygon network glasses [88] (D). Figure and caption adapted with permission from [83]. Copyright (2014) American Physical Society
We now turn our focus to graphene-based nanostructures formed by polymerization through Ullman-type reactions [14] on noble metal surfaces. We concentrate on high-symmetry close-packed surfaces, in particular the (111) surface, although vicinal surfaces [82, 89] as well as the (110) surface [90] have also been explored.
A selection of successful molecular precursors is shown in Fig. 6. The molecules contain carbon–halogen (typically Br or I) bonds that are intended to be cleaved by the formation of metal–organic bonds on the surface. The broken C–halogen bonds are then the anchoring points for the self-assembly into polymers. In most of the cases (but not always [91]), dehalogenation occurs at low temperature and diffusion needs to proceed in the presence of metal–organic bonds that stabilize the radicals.
Chemical drawings of molecular precursors for the metal-supported synthesis of atomically precise graphene nanostructures. Besides DBBA (top) used for the synthesis of 7-AGNRs [13] and hexaiodo-substituted cyclohexa-m-phenylene (CHP) used for the synthesis of porous graphene [92], the dibromo-tetraphenyltriphenylene molecule provides chevron-like GNRs [13], including the possibility of atomically precise doping with nitrogen atoms [93, 94]
In the case of iodine, the dehalogenation process starts already below room temperature. Scission of C–I is reported at 166 K on Au(111) [95] and is completed at room temperature also on other noble metal substrates (see e.g., [92, 96]). However, iodine can still be present on the substrate at 500 K, as reported in [12] for Cu(110).
A simple case of the interplay between adsorption sites and the diffusion process is offered by the Ullmann coupling of two iodobenzene molecules on Cu(111). Starting from the evidence that iodobenzene undergoes dehalogenation already at room temperature, Nguyen and coworkers studied the adsorption sites and diffusion paths for phenyl radicals on the Cu(111) surface [97]. The formation of a single metal–organic bond leaves high rotational freedom to the adsorbed molecule. Figure 7 depicts the most energetically favorable adsorption geometry. The molecule is bound to the surface atom and may diffuse to its six first neighbors with equal probability. A direct jump between initial state (IS) and final state (FS) is associated with a high energy barrier, but a different initial guess for the NEB allows to identify a low-energy, two-step diffusion mechanism. This mechanism can be ported to the (111) surfaces of the other noble metals.
Possible high-energy (top) and low-energy (bottom) diffusion pathways for a phenyl radical on the (111) surface of a noble metal. Figure and caption adapted from [97] with permission from PCCP Owner Societies
As demonstrated by this simple example, the rotational freedom of the molecule and the number of metal–organic bonds formed with the substrate can play a pivotal role in the diffusion process. Increasing complexity of the molecular precursor is reflected by a more complex diffusion mechanism. A remarkable example of the diffusion of a large molecular precursor is provided by the case of hexaiodo-substituted cyclohexa-m-phenylene (CHP) used to synthesize networks of porous graphene [86]. Again, the experimental evidence, supported by ab initio calculations, suggests that the C–I bonds are broken upon adsorption and iodine desorbs, when the temperature is increased to promote diffusion. The molecule is able to form six metal–organic bonds, one for each lost iodine atom. Due to the strong anchoring to the substrate, a naive initial guess for a NEB calculation of the diffusion pathway leads to very high activation barriers. A low-energy diffusion path, where the molecule rotates around one of the metal–organic bonds to diffuse to neighboring sites, can be identified with appropriate NEB guesses.
Also in this case, the “geometric” details of the diffusion mechanism apply in a similar fashion to Ag(111), Cu(111) and Au(111). Diffusion barriers vary due to the different matching of the molecular size with the substrate lattice and the different nature of the metal–organic binding. However, the transition from a dendritic growth mode of the network to a 2D growth mode, reported as shown in Fig. 8, cannot be attributed solely to the difference in diffusion barriers. As will be discussed in the next section, the barrier to form the “irreversible” coupling between two molecular species also plays a crucial role.
The dramatic effect of the substrate on the network geometry obtained by self-assembly of CHP on noble metal substrates [Cu(111), Au(111) and Ag(111)] is explained by the interplay between mobility of the molecules at the surface and reactivity of the molecules toward C–C coupling. Figure and caption adapted with permission from [92]. Copyright (2010) American Chemical Society
Compared to the C–I bond, scission of the C–Br occurs at higher temperature. While the C–Br bond is reported to be cleaved at room temperature on the Cu(111), Cu(110) and Ag(110) surfaces [98], it is reported to remain intact on Au(111) and Au(110) [88, 90, 98, 99]. In the case of Ag(111), the onset of C–Br bond cleavage is reported at room temperature [98, 100].
Desorption of Br typically occurs at temperatures above 200 °C [90, 98, 101]. At least in the case of brominated precursors, halogen atoms are therefore present on the surface during the diffusion of the precursor molecules. We are not aware of any study that investigates how the presence of halogen atoms on the metallic substrate influences the diffusion of the molecular precursors and their assembly. An exhaustive unbiased analysis of possible processes is challenging for computational approaches, but would be highly welcome.
4 Reactions
The synthetic approach toward nanographenes described here relies on annealing procedures to activate the two sequential steps of the reaction. If the temperature ranges for intermolecular polymerization and intramolecular cyclodehydrogenation are not well separated, the formation of large homogeneous polymers can be seriously hampered by steric hindrance between dehydrogenated monomers or by saturation of the radical species with hydrogen [66]. For this reason, the characteristic activation temperatures for a particular combination of substrate and precursor molecule are valuable information that may be predicted by atomistic simulations.
Also in this case, mechanisms identified for simple precursors can help in understanding more complex scenarios. Regarding the polymerization through Ullmann coupling, we start again from the investigation of iodobenzene [97]. As illustrated in Fig. 9, NEB calculations reveal that the coupling requires the dehalogenated molecules to reach a common surface atom. As a result of the metal–organic bonds, the shared metal atom pops out of the surface plane. When the two molecules approach each other and form the C–C bond, the surface atom retracts to its equilibrium position. This simple mechanism was later confirmed by Björk and coworkers on different noble metal substrates, including an investigation of the dehalogenation process [102].
Final step of the Ullmann coupling of phenyl radicals on a noble metal substrate. The two radicals share a surface atom that pops out from the surface plane (left). The transition state shows a partial bending of the molecules and a partial retraction of the surface atom (center). Upon completion, the surface atom retracts to its equilibrium surface position (right). Figure and caption adapted from [97] with permission from PCCP Owner Societies
While the mechanism is simple, the intrinsic properties of the metal substrate, such as its cohesive energy, and the interplay between molecular geometry and molecule–substrate interactions can result in quite different barriers along the reaction path. One example mentioned previously is the polymerization of cyclohexa-m-phenylene radicals (CHPR). As in the case of phenyl radicals, the reaction pathway in Fig. 10 [92] suggests that coupling can proceed, once two precursor molecules bind to the same surface atom. However, the large differences in diffusion barriers and activation energies between Cu(111) and Ag(111) drive a dramatic change in the network topology, as shown in Fig. 8. Diffusion of CHPR on Cu(111) is more difficult compared to the Ag(111) substrate, since the perfect lattice match in the case of copper allows the molecule to anchor strongly with six metal–organic bonds. The imperfect matching with the Ag(111) substrate makes it much easier to break C–Ag bonds. On the other hand, the different cohesive properties of the two metals result in different barriers for the coupling step.
Energy diagrams of the reaction pathways for CHPR−CHPR coupling on Cu(111) (top) and Ag(111) (bottom) obtained via NEB calculations. Pictorial representations of the molecule−surface configuration are given for the initial (II), intermediate (IM), and final (FI) states. The energies below each configuration are given with respect to the total energy of the final state. On both surfaces, the orange sphere indicates the central metal atom bonded to both CHPRs prior to intermolecular bond formation. Figure and caption reproduced with permission from [92]. Copyright (2010) American Chemical Society
In analogy with the picture provided by the “patchy-disk” model [83], a simple Monte Carlo simulation reproduces the phenomenology of the networks depicted in Fig. 8 [92]. In this particular case, a single parameter, representing the ratio between diffusion probability and sticking probability, is sufficient. A nice rationalization of the interplay between diffusion and coupling reaction for this kind of systems is also given in [102].
Once polymerization of the molecular precursors is achieved, the temperature has to be increased to activate the cyclodehydrogenation reaction. While surface-supported cyclodehydrogenation reactions were reported as early as 1999 [103], no theoretical investigations of the atomistic details of the reaction were available at the time. In a pioneering study, Treier and coworkers [19] investigated the fundamental role played by van der Waals dispersion forces in these processes. Dispersion forces favor flat geometries, meaning that significant distortions of the molecules or polymers may be necessary in order to form metal–organic bonds. Activation energies are thus determined by a delicate interplay between short-range interactions during bond formation and long-range dispersion forces.
An example of this concept is provided by the first step in the cyclodehydrogenation of cyclohexa-o-p-o-p-o-p-phenylene (CopP). As shown in Fig. 11, the molecule contains three para-phenylene rings that are free to rotate (containing C atoms labeled from 1 to 6) and three “rigid” ortho-phenylene rings. When the annealing temperature is properly controlled, each para-phenylene ring looses two hydrogens, while the hydrogens of the para-phenylene rings all remain attached: In the case of o-H cleavage, the formation of a metal–organic bond would be associated with considerable distortion of the molecule, driving up the corresponding energy barrier. In the case of p-H, the rotational freedom of the mobile para-phenylene unit enables metal–organic bond formation with little distortion. The full reaction path from CopP to tribenzo[a,g,m]coronene is essentially a sequential repetition of simple reaction steps, where dispersion forces aid in the approach of flexible subunits, hydrogen atoms are detached due to the formation of metal–organic bonds and irreversible C–C bonds are formed.
Cyclodehydrogenation of CopP. As indicated in step 1, o-H and p-H denote hydrogen atoms of ortho- and para-phenylene units, respectively. Figure and caption adapted from [19] with permission
Another remarkable example of a cyclodehydrogenation reaction proceeding by the repetition of simple atomic mechanisms is given by the transformation of polyanthryl chains into 7-AGNRs. This particular reaction was studied first by Björk and coworkers [104] and later by Blankenburg and coworkers [105] in more detail. As illustrated in Fig. 12, the reaction is initiated by an approach of the terminal phenyl rings of two neighboring anthryl units. After the formation of a C–C bond, the hydrogen atom pointing downward is collected by the catalytic surface. The hydrogen atom pointing upward migrates to an edge carbon atom of the polymer, which adopts the sp 3 configuration. The mechanism is repeated until planarity of the full structure is reached. Provided that the temperature is high enough, the downward-pointing hydrogens at the edge are removed as well, leaving behind a monohydrogenated armchair edge.
First three steps in the cyclodehydrogenation reaction that transforms a polyanthryl chain into a 7-AGNR. Van der Waals interactions favor proximity of phenyl rings (S0) that then react forming a C–C bond (S1). After formation of the bond, hydrogen atoms pointing toward the surface are detached through catalytic action of the substrate and hydrogen atoms pointing upward migrate to the edge of the polymer (S2). These three basic steps are iterated during the whole dehydrogenation process. Figure and caption adapted with permission from [105]. Copyright (2012) American Chemical Society
The detailed understanding of this sequence revealed that the reaction, once started on one side of the polymer chain, tends to proceed on the same side. Guided by this insight, a consistent tuning of the annealing temperature allowed to obtain partially reacted structures that represented the first realization of an atomically precise heterojunction between two GNRs with different band gaps. Intraribbon quantum dots formed in between two successive heterojunctions were predicted earlier to have interesting optical properties with potential for optoelectronic applications [106].
5 Electronic Structure
The prediction of electronic properties can aid the synthesis of graphene-based nanostructures already at the design stage: only structures with desired electronic properties are worth the effort of synthesis experiments. This pruning of undesirable structures is typically based on the “gas-phase” calculations that consider the bare nanostructures without a supporting substrate. In the field of GNRs, early theoretical predictions of their edge-related electronic and magnetic properties date back to 1996 [57, 58]. Since then, they have been studied extensively, moving from the framework of tight binding [60] to ab initio methods such as DFT [61] and many-body perturbation theory [65, 74].
Apart from the well-studied armchair and zigzag GNRs, GNRs with chiral edges can also have interesting electronic and magnetic properties [107]. A more exotic example is a class of GNRs with a backbone of zigzag edges, surmounted by a triangular-notched region of variable size, as depicted in Fig. 13. The asymmetry in their atomic structure gives rise to a ferromagnetic ground state, whose total magnetization can be tuned by changing the imbalance between the “A” and “B” sublattices [108, 109]. Furthermore, substitutional doping by boron or nitrogen induces half-metallicity. The most effective doping sites can be inferred from the spatial distribution of the frontier electronic states of the undoped GNR (see Fig. 13). Experiments for the synthesis of polymers with very large spin have been described [110], and the analogous realization of magnetic graphene-derived structures can be envisaged for the future [111].
Spin-resolved band structure and density of states for pristine (top) and nitrogen-doped (bottom) asymmetric zigzag GNR. The right panels show Kohn–Sham states of the indicated band at selected wave vectors. Upon doping with nitrogen (position marked by an orange circle) a spin-down band crosses the Fermi energy, signaling half-metallicity. Figure and caption adapted from [109]
Another important question concerns the stability of electronic and magnetic properties with respect to defects. With the motivation of synthesizing corresponding GNRs in the laboratory, we have considered GNRs with cove defects as depicted in Fig. 14. While the case of cove defects facing each other leads to a monotonous decrease in band gap with increasing width of the GNR [112], the staggered geometry shown in Fig. 14 gives rise to an interesting alternation between finite and zero band gap within DFT using the PBE exchange-correlation functional [113]. We find that spin polarization is suppressed at least up to N = 9, with further investigations needed into possible antiferromagnetic solutions for wider GNRs.
Electronic structure of zigzag GNRs (ZGNRs) with cove defects. Top panel Geometry of freestanding GNR with unit cell indicated in red. Non-planarity arises from steric hindrance between nearby hydrogen atoms. Bottom panel a, b Band structures for odd and even cases of width N. c Band gap as a function of the width N indicating alternation between finite and zero gap. Unpublished data from the authors
We note, however, that the magnetism of freestanding GNRs may easily be quenched, when the GNRs are adsorbed on a substrate. The case of zigzag GNRs adsorbed on the (111) surfaces of the coinage metals has recently been investigated using DFT with dispersion corrections in the DFT-D2 scheme [114]. For unpassivated zigzag edges, edge magnetism is shown to be suppressed on Cu, Ag, and Au due to strong hybridization of the carbon 2p with the metal d states. But even for hydrogen-passivated zigzag edges, n-doping of the GNR is reported to suppress edge magnetism on the more reactive Cu and Ag surfaces.
Unfortunately, charge transfer predicted by Kohn–Sham DFT with semi-local exchange-correlation functionals is often unreliable due to its inherent self-interaction error [115, 116]. The case of hydrogen-passivated ZGNRs on metal surfaces is particularly delicate: it tends to fall in between the regimes of physisorption and chemisorption, meaning that the semi-local exchange-correlation functional and the dispersion correction need to join seamlessly in order to obtain correct adsorption geometries. Preliminary results from the authors indicate that varying the ribbon–substrate distance by as little as 0.2 Å can strongly affect its magnetization, making the outcome of calculations critically dependent on the van der Waals correction employed.
Besides guiding at the design stage, simulations can provide important insights also during and after the experimental synthesis, for example, by helping to interpret and rationalize spectroscopy data. While this often requires taking the substrate into account or going beyond mean-field theories, standard gas-phase DFT calculations can also provide very useful information.
One interesting example concerns the band bending in heterojunctions between pristine and nitrogen-doped chevron-type GNRs [94]. Figure 7 shows two different precursor monomers for obtaining chevron-type GNRs, in one of which four carbon atoms have been replaced by nitrogens. Heterojunctions between pristine and N-doped GNRs can simply be obtained by sequential deposition and polymerization of pristine and doped precursors. Since nitrogen is more electronegative than carbon, the band onsets in the N-doped domains are expected at lower energy than in the pristine domains and band bending has to occur at the interface. DFT predicts a band shift of 0.5 eV and an electric field of 0.2 V/nm at the interface, in agreement with STS experiments of the heterojunctions measured on the Au(111) substrate. While STS involves electronic excitation of the GNR, we note here that the electric field at the heterojunction (and thus in essence also the band bending) is a ground-state property, providing justification for the use of DFT. Based on the established agreement with experiment, DFT can now tell us how to design the details of the band bending (see Fig. 15), for example, in view of possible applications in photovoltaics and electronics.
Control of band bending in GNRs via monomer chemical substitution. Top Illustration of GNR consisting of molecules with increasing degrees of nitrogen substitution. Bottom Energies of valence band maxima (VBM) and conduction band minima (CBM) for GNRs with increasing degrees of nitrogen substitution. A linear fit yields a band shift of −0.13 eV per nitrogen atom. Energies are given with respect to the vacuum level. Figure and caption adapted with permission from [94]
Another frequent task is the simulation of STM images. Since the tunneling current is exponentially sensitive to the tip–sample distance, an accurate description of the adsorption geometry is mandatory. If metal–organic bond formation between substrate and adsorbate can be excluded, it can be sufficient to model the substrate with empirical potentials in a QM/MM approach (see Sect. 2.1). This is the case in the example of the polyanthryl chain on Au(111) shown in the left panel of Fig. 16.
Comparing STM simulations and experiments. The left panel shows STM images of the polyanthryl chains obtained in the synthesis of 7-AGNRs. STM simulations (top) were performed using an empirical model for the substrate. Figure and caption reproduced with permission from Macmillan Publishers Ltd: [13], copyright (2010). The right panel shows STM images of the 7-AGNR terminus (b–c experiment, d–g simulation) with different bonding partners (I–IV) for the central carbon atom. The substrate was treated within DFT using the DFT-D3 dispersion correction [40]. Figure reproduced with permission from [66]. Copyright (2010) American Chemical Society
If, however, the substrate interacts chemically with the adsorbate, both need to be treated on the quantum mechanical level. The right panel of Fig. 16 shows the termini of the 7-AGNR on Au(111), where simulations considered different possible bonding partners of the central carbon at the terminus. In case II, a metal–organic bond is formed between the substrate and the radical, leading to a downward bend of the central carbon atom by about 1Å. This geometrical effect distinguishes the electronically similar cases II and III and would be missed using an empirical model for the substrate.
Besides the adsorption geometry, one important question in STM simulations concerns the geometry of the tip. In experiments with metal tips, information on the tip shape is typically not available. The popular Tersoff–Hamann approximation [117] therefore assumes a tip wave function with perfect s-wave character, making both the calculation and the physical interpretation of STM images particularly easy. As illustrated by Fig. 16, this simple approximation can provide simulations in good qualitative agreement with experiment, if the sample wave functions are properly extrapolated into the vacuum region [118]. Tip wave functions with higher angular momentum, as required, for example, when using a CO molecule as the tip [119], can be treated in a similar fashion using Chen’s derivative rule [120]. For studies with quantitative aspirations, the tip shape can be taken into account through simple step functions [121] or even through explicit treatment of the tip atoms [122, 123].
Going beyond qualitative predictions, however, can be a challenging task. In the following, we present two recent attempts, where substrate effects have been shown to play an essential role in determining the quantitative electronic and optical properties.
Ruffieux and coworkers measured the band gap of 7-AGNRs supported on Au(111) [82] through scanning tunneling spectroscopy (STS). The reported value of \(\Delta \approx 2.3 {\text{eV}}\) appears to contradict the theoretical prediction of \(\Delta \approx 3.7 {\text{eV}}\) for the fundamental gap obtained from GW calculations [65]. The origin of the discrepancy lies in the screening of the Coulomb interaction by the nearby substrate. Unfortunately, accurate GW calculations of the 7-AGNR on the Au(111) substrate are not yet attainable with reasonable computational effort (although calculations on “simpler” substrates are being undertaken [79]). In this particular case, however, hybridization of molecular states with the substrate is weak and there is no appreciable charge transfer [82], making it possible to include the screening effect through classical image charge corrections [77, 124]. As illustrated in Fig. 17, the GW correction brings the DFT gap of the isolated 7-AGNR from 1.6 to 3.7 eV. The IC correction that mimics the presence of the metallic substrate reduces the energy gap by 1.0–1.4 eV. Overall, this results in an energy band gap of 2.3–2.7 eV for the 7-AGNR on Au(111), in agreement with the experimental value of 2.3 eV.
Density of states (DOS) and electronic band gap Δ of the 7-AGNR. Left Scanning tunneling spectra recorded on the GNR (red, offset for clarity) and on the Au(111) substrate (black). Right LDA and GW-corrected DOS for the 7-AGNR in the gas phase and band gap reduction via image charge corrections on Au(111). Figure and caption adapted with permission from [82]. Copyright (2012) American Chemical Society
More recently, the optical properties of 7-AGNRs aligned on stepped Au(788) surfaces have been measured with reflectance difference spectroscopy (RDS) [125]. RDS measures the difference in complex reflectance for incident light polarized along two orthogonal directions, chosen here to point along and across the aligned GNRs (Fig. 18a). Since Au is optically isotropic [126], the RD signal is determined essentially by the optical response of the GNRs, which absorb light polarized along the GNR axis much more efficiently. The RD spectra shown in Fig. 18b are used to construct the dielectric function of the GNR, which is characterized by three discrete transitions at 2.1, 2.3, and 4.2 eV. The energies of these optical transitions are compared to calculations for freestanding 7-AGNRs in the GW/Bethe–Salpeter framework, yielding 1.9, 2.3, and 4.1 eV. It is interesting to observe that the optical gap computed for freestanding GNRs is in good agreement with experiment, while their fundamental gap is strongly affected by screening from the substrate. This suggests that the substrate-induced reduction in the fundamental gap is outbalanced by a corresponding reduction in the electron–hole binding energy. As discussed in [81] in more detail, this is expected, when the corresponding transition dipole moment vanishes. In this particular study, the clear assignment of RDS spectra also allowed to discriminate between the signatures of precursor molecules, polymers, and the planar GNRs, thus making RDS a powerful tool to monitor the synthesis during the different annealing phases in real time.
Reflectance difference spectroscopy (RDS) on 7-AGNRs. a Schematic of the RDS experiment on 7-AGNRs aligned uniaxially on a vicinal surface. b STM topography image of 7-AGNRs grown on Au(788) (scale bar 20 nm) with inset in high resolution. c RD spectra (open circles) measured in situ after GNR synthesis. The bottom panel shows the differential dielectric function Δε obtained by applying the three-phase model [126] (open symbols). Δε is fitted to a model including three discrete harmonic oscillator transitions (lines). The blue bars indicate the energy position and oscillator strength of the determined optical transitions. Figure and caption adapted with permission from [125]
References
Geim, A.K., Novoselov, K.S.: The rise of graphene. Nat. Mater. 6, 183–191 (2007)
Novoselov, K.S., Geim, A.K., Morozov, S.V., Jiang, D., Zhang, Y., Dubonos, S.V., Grigorieva, I.V., Firsov, A.A.: Electric field effect in atomically thin carbon films. Science 306, 666–669 (2004)
Castro Neto, A.H., Peres, N.M.R., Novoselov, K.S., Geim, A.K.: The electronic properties of. Rev. Mod. Phys. 81, 109–162 (2009)
Schwierz, F.: Graphene transistors: status, prospects, and problems. Proc. IEEE 101, 1567–1584 (2013)
Schwierz, F.: Graphene transistors. Nat. Nanotechnol. 5, 487–496 (2010)
Sanchez-Valencia, J.R., Dienel, T., Gröning, O., Shorubalko, I., Mueller, A., Jansen, M., Amsharov, K., Ruffieux, P., Fasel, R.: Controlled synthesis of single-chirality carbon nanotubes. Nature 512, 61–64 (2014)
Han, M., Özyilmaz, B., Zhang, Y., Kim, P.: Energy band-gap engineering of graphene nanoribbons. Phys. Rev. Lett. 98, 206805 (2007)
Wu, Z.-S., Ren, W., Gao, L., Liu, B., Zhao, J., Cheng, H.-M.: Efficient synthesis of graphene nanoribbons sonochemically cut from graphene sheets. Nano Res. 3, 16–22 (2010)
Kosynkin, D.V.D., Higginbotham, A.A.L., Sinitskii, A., Lomeda, J.R., Dimiev, A., Price, B.K., Tour, J.M.: Longitudinal unzipping of carbon nanotubes to form graphene nanoribbons. Nature 458, 872–876 (2009)
Grill, L., Dyer, M., Lafferentz, L., Persson, M., Peters, M.V., Hecht, S.: Nano-architectures by covalent assembly of molecular building blocks. Nat. Nanotechnol. 2, 687–691 (2007)
Lafferentz, L., Ample, F., Yu, H., Hecht, S., Joachim, C., Grill, L.: Conductance of a single conjugated polymer as a continuous function of its length. Science 323, 1193–1198 (2009)
Lipton-Duffin, J.A., Ivasenko, O., Perepichka, D.F., Rosei, F.: Synthesis of polyphenylene molecular wires by surface-confined polymerization. Small 5, 592–597 (2009)
Cai, J., Ruffieux, P., Jaafar, R., Bieri, M., Braun, T., Blankenburg, S., Muoth, M., Seitsonen, A.P., Saleh, M., Feng, X., Müllen, K., Fasel, R.: Atomically precise bottom-up fabrication of graphene nanoribbons. Nature 466, 470–473 (2010)
Ullmann, F., Bielecki, J.: Ueber Synthesen in der Biphenylreihe. Berichte der Dtsch. Chem. Gesellschaft. 34, 2174–2185 (1901)
Hohenberg, P., Kohn, W.: Inhomogeneous electron gas. Phys. Rev. 155, B864 (1964)
CPMD v3.17 Copyright IBM Corp 1990–2013, Copyright MPI fuer Feskoerperforschung Stuttgart 1997-2001
Hutter, J., Iannuzzi, M., Schiffmann, F., VandeVondele, J.: CP2K: atomistic simulations of condensed matter systems. Wiley Interdiscip. Rev. Comput. Mol. Sci. 4, 15–25 (2014)
Artacho, E., Anglada, E., Diéguez, O., Gale, J.D., García, A, Junquera, J., Martin, R.M., Ordejón, P., Pruneda, J.M., Sánchez-Portal, D., Soler, J.M.: The SIESTA method; developments and applicability. J. Phys. Condens. Matter. 20, 064208 (2008)
Treier, M., Pignedoli, C.A., Laino, T., Rieger, R., Müllen, K., Passerone, D., Fasel, R.: Surface-assisted cyclodehydrogenation provides a synthetic route towards easily processable and chemically tailored nanographenes. Nature Chem. 3, 61–67 (2011)
Björk, J., Hanke, F.: Towards design rules for covalent nanostructures on metal surfaces. Chemistry 20, 928–934 (2014)
Iannuzzi, M., Hutter, J.: Inner-shell spectroscopy by the Gaussian and augmented plane wave method. Phys. Chem. Chem. Phys. 9, 1599–1610 (2007)
Ljungberg, M.P., Mortensen, J.J., Pettersson, L.G.M.: An implementation of core level spectroscopies in a real space projector augmented wave density functional theory code. J. Electron Spectros. Relat. Phenomena. 184, 427–439 (2011)
Baroni, S., de Gironcoli, S.: Phonons and related crystal properties from density-functional perturbation theory. Rev. Mod. Phys. 73, 515 (2001)
Lazzeri, M., Mauri, F.: First-principles calculation of vibrational raman spectra in large systems: signature of small rings in crystalline SiO2. Phys. Rev. Lett. 90, 036401 (2003)
Venezuela, P., Lazzeri, M., Mauri, F.: Theory of double-resonant Raman spectra in graphene: intensity and line shape of defect-induced and two-phonon bands. Phys. Rev. B. 84, 035433 (2011)
Warshel, A., Levitt, M.: Folding and stability of helical proteins: carp myogen. J. Mol. Biol. 106, 421–437 (1976)
Senn, H.M., Thiel, W.: QM/MM methods for biomolecular systems. Angew. Chem. Int. Ed. Engl. 48, 1198–1229 (2009)
Foiles, S., Baskes, M., Daw, M.: Embedded-atom-method functions for the fcc metals Cu, Ag, Au, Ni, Pt, and their alloys. Phys. Rev. B, Pd (1986)
Kohn, W., Sham, L.: Self-consistent equations including exchange and correlation effects. Phys. Rev. 140, A1133 (1965)
Levi, A.C., Calvini, P.: Elastic theory of surface deformation in C60 adsorption. Surf. Sci. 601, 1494–1500 (2007)
Pignedoli, C.A., Laino, T., Treier, M., Fasel, R., Passerone, D.: A simple approach for describing metal-supported cyclohexaphenylene dehydrogenation. Eur. Phys. J. B 75, 65–70 (2010)
Laio, A., Parrinello, M.: Escaping free-energy minima. Proc. Natl. Acad. Sci. U.S.A. 99, 12562–12566 (2002)
Laio, A., Gervasio, F.L.: Metadynamics: a method to simulate rare events and reconstruct the free energy in biophysics, chemistry and material science. Reports Prog. Phys. 71, 126601 (2008)
Pietrucci, F., Andreoni, W.: Graph theory meets ab initio molecular dynamics: atomic structures and transformations at the nanoscale. Phys. Rev. Lett. 107, 085504 (2011)
Tribello, G., Ceriotti, M., Parrinello, M.: Using sketch-map coordinates to analyze and bias molecular dynamics simulations. Proc. Natl. Acad. Sci. 109, 5196–5201 (2012)
Rohrdanz, M.A., Zheng, W., Clementi, C.: Discovering mountain passes via torchlight: methods for the definition of reaction coordinates and pathways in complex macromolecular reactions. Annu. Rev. Phys. Chem. 64, 295–316 (2013)
Rydberg, H., Dion, M., Jacobson, N., Schröder, E., Hyldgaard, P., Simak, S., Langreth, D., Lundqvist, B.I.: Van der Waals density functional for layered Structures. Phys. Rev. Lett. 91, 126402 (2003)
Grimme, S.: Density functional theory with London dispersion corrections. Wiley Interdiscip. Rev. Comput. Mol. Sci. 1, 211–228 (2011)
Zhao, Y., Truhlar, D.G.: Exploring the limit of accuracy of the global hybrid meta density functional for main-group thermochemistry, kinetics, and noncovalent interactions. J. Chem. Theory Comput. 4, 1849–1868 (2008)
Grimme, S., Antony, J., Ehrlich, S., Krieg, H.: A consistent and accurate ab initio parametrization of density functional dispersion correction (DFT-D) for the 94 elements H-Pu. J. Chem. Phys. 132, 154104 (2010)
Tkatchenko, A., Scheffler, M.: Accurate molecular Van der Waals Interactions from ground-state electron density and free-atom reference data. Phys. Rev. Lett. 102, 073005 (2009)
Ruiz, V.G., Liu, W., Zojer, E., Scheffler, M., Tkatchenko, A.: Density-functional theory with screened Van der Waals interactions for the modeling of hybrid inorganic-organic systems. Phys. Rev. Lett. 108, 146103 (2012)
Hanke, F.: Sensitivity analysis and uncertainty calculation for dispersion corrected density functional theory. J. Comput. Chem. 32, 1424–1430 (2011)
Dion, M., Rydberg, H., Schröder, E., Langreth, D.C., Lundqvist, B.I.: Van der Waals density functional for general geometries. Phys. Rev. Lett. 92, 246401 (2004)
Lee, K., Murray, É.D., Kong, L., Lundqvist, B.I., Langreth, D.C.: Higher-accuracy Van der Waals density functional. Phys. Rev. B. 82, 081101 (2010)
Hamada, I.: van der Waals density functional made accurate. Phys. Rev. B. 89, 121103 (2014)
Gulans, A., Puska, M., Nieminen, R.: Linear-scaling self-consistent implementation of the Van der Waals density functional. Phys. Rev. B. 79, 201105 (2009)
Björk, J., Stafström, S.: Adsorption of large hydrocarbons on coinage metals: a Van der Waals density functional study. Chem. Phys. Chem. 15, 2851–2858 (2014)
Elber, R., Karplus, M.: A method for determining reaction paths in large molecules: application to myoglobin. Chem. Phys. Lett. 139, 375–380 (1987)
Henkelman, G., Jónsson, H.: A dimer method for finding saddle points on high dimensional potential surfaces using only first derivatives. J. Chem. Phys. 111, 7010–7022 (1999)
Henkelman, G., Uberuaga, B.P., Jónsson, H.: A climbing image nudged elastic band method for finding saddle points and minimum energy paths. J. Chem. Phys. 113, 9901–9904 (2000)
Ren, W., Vanden-Eijnden, E.: String method for the study of rare events. Phys. Rev. B. 66, 052301 (2002)
Ren, W., Vanden-Eijnden, E.: Finite temperature string method for the study of rare events. J. Phys. Chem. B. 109, 6688–6693 (2005)
Branduardi, D., Gervasio, F.L., Parrinello, M.: From A to B in free energy space. J. Chem. Phys. 126, 054103 (2007)
Clar, E.: The aromatic sextet. J. Wiley (1972)
Wassmann, T., Seitsonen, A.: Clar’s theory, n-electron distribution, and geometry of graphene nanoribbons. J. Am. Chem. Soc. 132, 3440–3451 (2010)
Nakada, K., Fujita, M., Dresselhaus, G., Dresselhaus, M.: Edge state in graphene ribbons: nanometer size effect and edge shape dependence. Phys. Rev. B. 54, 17954–17961 (1996)
Fujita, M., Wakabayashi, K.: Peculiar localized state at zigzag graphite edge. J. Phys. Soc. Japan. 65, 1920–1923 (1996)
Wakabayashi, K., Sasaki, K., Nakanishi, T., Enoki, T.: Electronic states of graphene nanoribbons and analytical solutions. Sci. Technol. Adv. Mater. 11, 054504 (2010)
Enoki, T., Ando, T.: Physics and Chemistry of Graphene: Graphene to Nanographene. Pan Stanford (2013)
Son, Y.-W., Cohen, M.L., Louie, S.G.: Energy gaps in graphene nanoribbons. Phys. Rev. Lett. 97, 216803 (2006)
Gunlycke, D., White, C.: Tight-binding energy dispersions of armchair-edge graphene nanostrips. Phys. Rev. B. 77, 115116 (2008)
Girao, E., Cruz-Silva, E., Meunier, V.: Electronic transport properties of assembled carbon nanoribbons. ACS Nano 6, 6483–6491 (2012)
Boykin, T.B., Luisier, M., Klimeck, G., Jiang, X., Kharche, N., Zhou, Y., Nayak, S.K.: Accurate six-band nearest-neighbor tight-binding model for the π-bands of bulk graphene and graphene nanoribbons. J. Appl. Phys. 109, 104304 (2011)
Yang, L., Park, C.-H., Son, Y.-W., Cohen, M., Louie, S.: Quasiparticle energies and band gaps in graphene nanoribbons. Phys. Rev. Lett. 99, 186801 (2007)
Talirz, L., Söde, H., Cai, J., Ruffieux, P., Blankenburg, S., Jafaar, R., Berger, R., Feng, X., Müllen, K., Passerone, D., Fasel, R., Pignedoli, C.A.: Termini of bottom-up fabricated graphene nanoribbons. J. Am. Chem. Soc. 135, 2060–2063 (2013)
Ijäs, M., Ervasti, M., Uppstu, A., Liljeroth, P.: Electronic states in finite graphene nanoribbons: effect of charging and defects. Phys. Rev. B. 88, 075429 (2013)
Louie, S.G.: Conceptual Foundations of Materials—A Standard Model for Ground—and Excited-State Properties. Elsevier, Philadelphia (2006)
Hedin, L.: New method for calculating the one-particle green’s function with application to the electron-gas problem. Phys. Rev. 139, A796 (1965)
van Schilfgaarde, M., Kotani, T., Faleev, S.: Quasiparticle self-consistent GW theory. Phys. Rev. Lett. 96, 226402 (2006)
Spataru, C.D., Ismail-Beigi, S., Benedict, L.X., Louie, S.G.: Quasiparticle energies, excitonic effects and optical absorption spectra of small-diameter single-walled carbon nanotubes. Appl. Phys. A Mater. Sci. Process. 78, 1129–1136 (2004)
Onida, G., Reining, L., Rubio, A.: Electronic excitations: density-functional versus many-body Green’s-function approaches. Rev. Mod. Phys. 74, 601 (2002)
Salpeter, E., Bethe, H.: A relativistic equation for bound-state problems. Phys. Rev. 84, 1232 (1951)
Yang, L., Cohen, M.L., Louie, S.G.: Excitonic effects in the optical spectra of graphene nanoribbons. Nano Lett. 7, 3112–3115 (2007)
Prezzi, D., Varsano, D., Ruini, A., Marini, A., Molinari, E.: Optical properties of graphene nanoribbons: the role of many-body effects. Phys. Rev. B. 77, 041404 (2008)
Deslippe, J., Samsonidze, G., Strubbe, D.A., Jain, M., Cohen, M.L., Louie, S.G.: BerkeleyGW: a massively parallel computer package for the calculation of the quasiparticle and optical properties of materials and nanostructures. Comput. Phys. Commun. 183, 1269–1289 (2012)
Neaton, J., Hybertsen, M., Louie, S.: Renormalization of molecular electronic levels at metal-molecule interfaces. Phys. Rev. Lett. 97, 216405 (2006)
Freysoldt, C., Rinke, P., Scheffler, M.: Controlling polarization at insulating surfaces: quasiparticle calculations for molecules adsorbed on insulator films. Phys. Rev. Lett. 103, 056803 (2009)
Jiang, X., Kharche, N., Kohl, P., Boykin, T.B., Klimeck, G., Luisier, M., Ajayan, P.M., Nayak, S.K.: Giant quasiparticle bandgap modulation in graphene nanoribbons supported on weakly interacting surfaces. Appl. Phys. Lett. 103, 133107 (2013)
Li, Y., Lu, D., Galli, G.: Calculation of quasi-particle energies of aromatic self-assembled monolayers on Au(111). J. Chem. Theory Comput. 5, 881–886 (2009)
Garcia-Lastra, J.M., Thygesen, K.S.: Renormalization of optical excitations in molecules near a metal surface. Phys. Rev. Lett. 106, 187402 (2011)
Ruffieux, P., Cai, J., Plumb, N.N.C., Patthey, L., Prezzi, D., Ferretti, A., Molinari, E., Feng, X., Müllen, K., Pignedoli, C.A., Fasel, R.: Electronic structure of atomically precise graphene nanoribbons. ACS Nano 6, 6930–6935 (2012)
Whitelam, S., Tamblyn, I., Haxton, T.K., Wieland, M.B., Champness, N.R., Garrahan, J.P., Beton, P.H.: Common physical framework explains phase behavior and dynamics of atomic, molecular, and polymeric network formers. Phys. Rev. X. 4, 011044 (2014)
He, Y., Chen, Y., Liu, H.: Self-assembly of hexagonal DNA two-dimensional (2D) arrays. J. Am. Chem. Soc. 127, 12202–12203 (2005)
Lichtenstein, L., Heyde, M., Freund, H.-J.: Crystalline-vitreous interface in two dimensional silica. Phys. Rev. Lett. 109, 106101 (2012)
Bieri, M., Treier, M., Cai, J., Aït-Mansour, K., Ruffieux, P., Gröning, O., Gröning, P., Kastler, M., Rieger, R., Feng, X., Müllen, K., Fasel, R.: Porous graphenes: two-dimensional polymer synthesis with atomic precision. Chem. Commun. (Camb). 45, 6919–6921 (2009)
Palma, C.-A., Samorì, P., Cecchini, M.: Atomistic simulations of 2D bicomponent self-assembly: from molecular recognition to self-healing. J. Am. Chem. Soc. 132, 17880–17885 (2010)
Blunt, M.O., Russell, J.C., Champness, N.R., Beton, P.H.: Templating molecular adsorption using a covalent organic framework. Chem. Commun. (Camb.) 46, 7157–7159 (2010)
Linden, S., Zhong, D., Timmer, A., Aghdassi, N., Franke, J., Zhang, H., Feng, X., Müllen, K., Fuchs, H., Chi, L., Zacharias, H.: Electronic structure of spatially aligned graphene nanoribbons on Au(788). Phys. Rev. Lett. 108, 216801 (2012)
Batra, A., Cvetko, D., Kladnik, G., Adak, O., Cardoso, C., Ferretti, A., Prezzi, D., Molinari, E., Morgante, A., Venkataraman, L.: Probing the mechanism for graphene nanoribbon formation on gold surfaces through X-ray spectroscopy. Chem. Sci. 5, 4419–4423 (2014)
Pham, T.A., Song, F., Nguyen, M.-T., Stöhr, M.: Self-assembly of pyrene derivatives on Au(111): substituent effects on intermolecular interactions. Chem. Commun. 50, 14089 (2014)
Bieri, M., Nguyen, M.-T., Gröning, O., Cai, J., Treier, M., Aït-Mansour, K., Ruffieux, P., Pignedoli, C.A., Passerone, D., Kastler, M., Müllen, K., Fasel, R., Gröning, O.: Two-dimensional polymer formation on surfaces: insight into the roles of precursor mobility and reactivity. J. Am. Chem. Soc. 132, 16669–16676 (2010)
Bronner, C., Stremlau, S., Gille, M., Brauße, F., Haase, A., Hecht, S., Tegeder, P.: Aligning the band gap of graphene nanoribbons by monomer doping. Angew. Chem. Int. Ed. Engl. 52, 4422–4425 (2013)
Cai, J., Pignedoli, C.A., Talirz, L., Ruffieux, P., Söde, H., Liang, L., Meunier, V., Berger, R., Li, R., Feng, X., Müllen, K., Fasel, R.: Graphene nanoribbon heterojunctions. Nat. Nanotechnol. 9, 896–900 (2014)
Kanuru, V.K., Kyriakou, G., Beaumont, S.K., Papageorgiou, A.C., Watson, D.J., Lambert, R.M.: Sonogashira coupling on an extended gold surface in vacuo: reaction of phenylacetylene with iodobenzene on Au(111). J. Am. Chem. Soc. 132, 8081–8086 (2010)
Sykes, E.C.H., Han, P., Kandel, S.A., Kelly, K.F., McCarty, G.S., Weiss, P.S.: Substrate-mediated interactions and intermolecular forces between molecules adsorbed on surfaces. Acc. Chem. Res. 36, 945–953 (2003)
Nguyen, M.-T., Pignedoli, C.A., Passerone, D.: An ab initio insight into the Cu(111)-mediated Ullmann reaction. Phys. Chem. Chem. Phys. 13, 154–160 (2011)
Gutzler, R., Cardenas, L., Lipton-Duffin, J., El Garah, M., Dinca, L.E., Szakacs, C.E., Fu, C., Gallagher, M., Vondráček, M., Rybachuk, M., Perepichka, D.F., Rosei, F.: Ullmann-type coupling of brominated tetrathienoanthracene on copper and silver. Nanoscale. 6, 2660–2668 (2014)
Simonov, K., Vinogradov, N.A., Vinogradov, A.S., Generalov, A.V., Zagrebina, E.M., Martenson, N., Cafolla, A.A., Carpy, T., Cunniffe, J.P., Preobrajenski, A.B.: Effect of substrate chemistry on the bottom-up fabrication of graphene nanoribbons: combined core-level spectroscopy and STM study. J. Phys. Chem. C 118, 12532–12540 (2014)
Eichhorn, J., Strunskus, T., Rastgoo-Lahrood, A., Samanta, D., Schmittel, M., Lackinger, M.: On-surface Ullmann polymerization via intermediate organometallic networks on Ag (111). Chem. Comm. 50, 7680–7682 (2014)
Bronner, C., Björk, J., Tegeder, P.: Tracking and removing Br during the on-surface synthesis of a graphene nanoribbon. J. Phys. Chem. C 119, 486–493 (2015). doi:10.1021/jp5106218
Björk, J., Hanke, F., Stafström, S.: Mechanisms of halogen-based covalent self-assembly on metal surfaces. J. Am. Chem. Soc. 135, 5768–5775 (2013)
Weiss, K., Beernink, G., Dötz, F., Birkner, A., Müllen, K., Wöll, C.H.: Template-mediated synthesis of polycyclic aromatic hydrocarbons: cyclodehydrogenation and planarization of a hexaphenylbenzene derivative at a copper surface. Angew. Chem. Int. Ed. Engl. 38, 3748–3752 (1999)
Björk, J., Stafström, S., Hanke, F.: Zipping up: cooperativity drives the synthesis of graphene nanoribbons. J. Am. Chem. Soc. 133, 14884–14887 (2011)
Blankenburg, S., Cai, J., Ruffieux, P., Jaafar, R., Passerone, D., Feng, X., Fasel, R., Müllen, K., Pignedoli, C.A.: Intraribbon heterojunction formation in ultranarrow graphene nanoribbons. ACS Nano 6, 2020–2025 (2012)
Prezzi, D., Varsano, D., Ruini, A., Molinari, E.: Quantum dot states and optical excitations of edge-modulated graphene nanoribbons. Phys. Rev. B. 84, 041401 (2011)
Yazyev, O.V., Capaz, R.B., Louie, S.G.: Theory of magnetic edge states in chiral graphene nanoribbons. Phys. Rev. B. 84, 115406 (2011)
Wang, W., Yazyev, O., Meng, S., Kaxiras, E.: Topological frustration in graphene nanoflakes: magnetic order and spin logic devices. Phys. Rev. Lett. 102, 157201 (2009)
Adams, D.J., Gröning, O., Pignedoli, C.A., Ruffieux, P., Fasel, R., Passerone, D.: Stable ferromagnetism and doping-induced half-metallicity in asymmetric graphene nanoribbons. Phys. Rev. B. 85, 245405 (2012)
Rajca, A., Wongsriratanakul, J., Rajca, S.: Magnetic ordering in an organic polymer. Science (80-.). 294, 1503–1505 (2001)
Wang, W.L., Meng, S., Kaxiras, E.: Graphene nanoflakes with large spin. Nano Lett. 8, 241–245 (2008)
Wakabayashi, K., Okada, S., Tomita, R., Fujimoto, S., Natsume, Y.: Edge States and flat bands of graphene nanoribbons with edge modification. J. Phys. Soc. Japan. 79, 034706 (2010)
Perdew, J., Burke, K., Ernzerhof, M.: Generalized gradient approximation made simple. Phys. Rev. Lett. 77, 3865–3868 (1996)
Li, Y., Zhang, W., Morgenstern, M., Mazzarello, R.: Electronic and magnetic properties of zigzag graphene nanoribbons on the (111) surface of Cu, Ag, and Au. Phys. Rev. Lett. 110, 216804 (2013)
Ke, S.-H., Baranger, H.U., Yang, W.: Role of the exchange-correlation potential in ab initio electron transport calculations. J. Chem. Phys. 126, 201102 (2007)
Cohen, A.J., Mori-Sánchez, P., Yang, W.: Insights into current limitations of density functional theory. Science 321, 792–794 (2008)
Tersoff, J., Hamann, D.R.: Theory of the scanning tunneling microscope. Phys. Rev. B. 31, 805–813 (1985)
Tersoff, J.: Method for the calculation of scanning tunneling microscope images and spectra. Phys. Rev. B. 40, 11990–11993 (1989)
Gross, L., Moll, N., Mohn, F., Curioni, A., Meyer, G., Hanke, F., Persson, M.: High-resolution molecular orbital imaging using a p-Wave STM tip. Phys. Rev. Lett. 107, 086101 (2011)
Chen, C.: Tunneling matrix elements in three-dimensional space: the derivative rule and the sum rule. Phys. Rev. B. 42, 8841 (1990)
Gaspari, R., Blankenburg, S., Pignedoli, C.A., Ruffieux, P., Treier, M., Fasel, R., Passerone, D.: S-orbital continuum model accounting for the tip shape in simulated scanning tunneling microscope images. Phys. Rev. B. 84, 125417 (2011)
Blanco, J., González, C., Jelínek, P., Ortega, J., Flores, F., Pérez, R.: First-principles simulations of STM images: from tunneling to the contact regime. Phys. Rev. B. 70, 085405 (2004)
Lewis, J.P., Jelínek, P., Ortega, J., Demkov, A.A., Trabada, D.G., Haycock, B., Wang, H., Adams, G., Tomfohr, J.K., Abad, E., Wang, H., Drabold, D.A.: Advances and applications in the FIREBALL ab initio tight-binding molecular-dynamics formalism. Phys. Status Solidi B 248, 1989–2007 (2011)
Thygesen, K., Rubio, A.: Renormalization of molecular quasiparticle levels at metal-molecule interfaces: trends across binding regimes. Phys. Rev. Lett. 102, 046802 (2009)
Denk, R., Hohage, M., Zeppenfeld, P., Cai, J., Pignedoli, C.A., Söde, H., Fasel, R., Feng, X., Müllen, K., Wang, S., Prezzi, D., Ferretti, A., Ruini, A., Molinari, E., Ruffieux, P.: Exciton-dominated optical response of ultra-narrow graphene nanoribbons. Nat. Commun. 5, 4253 (2014)
Weightman, P., Martin, D.S., Cole, R.J., Farrell, T.: Reflection anisotropy spectroscopy. reports. Prog. Phys. 68, 1251–1341 (2005)
Acknowledgments
The authors acknowledge the Swiss National Science Foundation (SNF) for funding and the Swiss Supercomputing Centre (CSCS) for computational resources.
Author information
Authors and Affiliations
Corresponding author
Editor information
Editors and Affiliations
Rights and permissions
Copyright information
© 2016 Springer International Publishing Switzerland
About this paper
Cite this paper
Talirz, L., Shinde, P., Passerone, D., Pignedoli, C.A. (2016). Synthesis of Atomically Precise Graphene-Based Nanostructures: A Simulation Point of View. In: Gourdon, A. (eds) On-Surface Synthesis. Advances in Atom and Single Molecule Machines. Springer, Cham. https://doi.org/10.1007/978-3-319-26600-8_12
Download citation
DOI: https://doi.org/10.1007/978-3-319-26600-8_12
Published:
Publisher Name: Springer, Cham
Print ISBN: 978-3-319-26598-8
Online ISBN: 978-3-319-26600-8
eBook Packages: Chemistry and Materials ScienceChemistry and Material Science (R0)