Abstract
At present, the availability of nonrenewable sources and their usage for electric vehicle technology is reducing gradually because of their disadvantages are more environmental pollution, direct effect on human health, less reliability, and taking more time to start functioning. So, in this article, the proton exchange membrane fuel cell (PEMFC) is considered for the automotive application because of its advantages quick startup, more power density, more safety to handle, high efficiency, and capability of operating at very low operational temperature conditions. However, the drawback of PEMFC is very difficult to identify the accurate MPP position of the fuel system. Here, the improved variable step genetic algorithm is added with the adaptive neuro-fuzzy inference system for tracking the operational point of the proposed system with high efficiency. These hybrid MPPT controller features are easy to understand, more accurate, have a better dynamic response, and have low design complexity. The evaluated proposed MPPT controller operational efficiency, and settling time of the converter voltage at different fuel stack temperature conditions are 98.7402%, and 0.01607 s respectively. Finally, the boost converter is used in this work to enhance the voltage supply capability of the entire system. The proposed system is investigated by applying the MATLAB tool.
Similar content being viewed by others
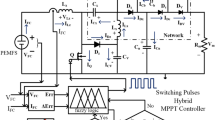
Explore related subjects
Discover the latest articles, news and stories from top researchers in related subjects.Introduction
From the present power production scenario, the utilization of conventional power systems is reduced because its drawbacks are less robust, more system operational cost, require more time to install, high catchment area needed, create more environmental disturbances, and produce greenhouse gas emissions. These demerits of nonrenewable power production systems are limited by focusing on the present renewable power networks1. The available renewable systems in nature are wind networks, tidal power supply systems, solar Photovoltaic (PV) energy supply networks, and fuel cells. In2, the researchers studied wind energy technologies and their associated installation possibility areas. Here, the wind power systems transfer the wind kinetic energy into mechanical power by using the rotating wind blades, and finally, the extracted mechanical energy is converted into useful energy which is consumed by the industries. The challenges involved in the wind energy networks are not useful for all locations, creating more noise, less predictability, and more impact on wildlife. Especially, in India, it creates more visual pollution3. In some places, sunlight-dependent PV panels are located to produce uniform energy for the people of rural areas. Each sunlight PV cell delivers a maximum possibility of 0.96 V and its power flow nature is exactly similar to the P-N diode. However, the one-cell power production is not enough to meet the automotive industry load4.
In5, the research scholars explained about the sunlight power production enhancement. Here, the multiple types of solar networks are interlinked with each other to enhance the overall system energy generation thereby the ability to supply the solar peak power to the consumers. The merits of sunlight modules are reducing the utility of conventional power systems, helping consumers to optimize their electricity bills, low-level dependence on the central power grid, more savings, low maintenance cost, and ease of installation. However, the drawbacks of sunlight networks are more upfront cost, complete dependence on solar light, more space constraints, and high atmospheric impact of PV module manufacturing. Also, the solar networks produce nonuniform energy which is not directly accepted by the electric vehicles. The tidal energy supply system is selected in6 to limit the drawbacks of solar energy. In this tidal system, the ocean tides directly hit the bidirectional rotor for operating the generator thereby transmitting the electricity to the urban people. However, the demerits of tidal networks are high operational costs, more difficulty in the development of the barrage near the ocean place, and disturbance to the ocean's living fishes. The hydropower networks are much more useful when associated with the tidal systems because of their uniform energy production nature, and it is very clean sources. However, this system has the drawbacks of limited system location, excessive initial cost, more carbon emissions with methane, and flood risk7.
The disadvantages of hybrid renewable systems are limited by selecting the fuel cell renewable power. From the literature study, fuel cell technology is a very new and present trend for automotive networks because its advantages are continuous energy production, less air pollution, more reliable energy sources, good scalability, and high energy conversion efficiency8. Now, in the market, there are various categories of fuel power generation technologies exist which are differentiated as MCFS, Solid Oxide dependent Fuel Stack (SOFS), AFS, DMFS, phosphoric-based fuel stack, and Proton Exchange Membrane Fuel Stack (PEMFS). In9, the researchers referred to the direct methanol-based fuel system for the video surveillance system because it does not require a fuel reformer. Due to the absence of a fuel reformer in the system, the entire network size, and implementation cost are optimized. Also, the applications of direct methanol cells are remote monitoring systems, better charging, mobile communication systems, remote traffic control, and supporting the power supply in hospitalized conditions. Also, this system works as an autonomous power source for testing industrial instruments. The demerits of this fuel network are needing more purified methanol chemicals, comprehensively very low operational efficiency, storage of chemicals at below room temperature is quite difficult, more possibility of membrane damage, methanol cross which directly affects the membrane efficiency, and more platinum catalyst material is required10.
The Phosphoric Acid-based Fuel Stack (PAFS) technology is utilized in aerospace networks to compensate for the demerits of direct methanol cells11. Also, it is applicable for producing low-pressurized steam, space heating, and domestic water heating applications. The PAFS has many advantages which are less electrolyte volatility, long life stability, very easy development, and low space for fixing. Also, it uses the H3PO4 effectively without any atmospheric disturbances. The maximum possible operational temperature of PAFS is 220 °C which is very low. Due to this low operating temperature, the PAFS ionic conductivity is low12. Here, the electrodes are manufactured by selecting the Pt, CO, and iron materials, and the entire catalyst is covered by carbon paper. Finally, the 1 kW PAFS development cost is between 40 k$ to 50 k$. So, the present electricity-based running vehicles are not utilizing this type of fuel cell. The alkaline-based fuel power production network is considered in the article13 for onboard energy consumption. Also, this fuel stack is used as the backup power source for industrial and commercial applications. In addition to that this stack is acceptable for the remote located buildings.
From the literature review, the significant features of the AFS are capable of working at low operational temperature, giving high operational efficiency at high input supply temperature conditions, and the possibility of utilizing the nonnoble catalyst14. The major drawbacks of alkaline cells are heavy, have larger sizes when associated with the phosphoric acid cell, and create more issues with carbon monoxide. Also, its raw material cost is excessive15. The present fuel stack usage strategy is mentioned in Fig. 1. So, in this work, the polymer membrane fuel stack technology is considered for giving continuous electricity to hydrogen-based vehicles. The attractive advantages of PEMFS are the ability to function at all temperature conditions, safe handling, easy understanding, quick power production, and more efficiency. The main challenge of this fuel stack is the continuous fluctuation of the functioning point of the system which is stabilized by applying the various categories of power point identifiers for achieving the maximum possible peak voltage of the system. In16,17,18, the scholars applied the fractional voltage concept for moving the operational point of the solid oxide system from any local position to a global place thereby optimizing the power distribution loss. This fractional voltage open-loop controller development and understanding are easier because it does not need a high number of sensors, and requires low fixing cost. However, this method is a completely assumption-oriented controller and provides low fuel stack energy.
The fractional current adjustment block is interlinked with the fuel stack and sunlight system for obtaining the peak voltage from the sunlight network in the morning time, and evening time, the fuel stack module is applied to the electric vehicle battery charging systems for uniform charging19. In this current controller, only one sensor is selected for controlling the fuel module temperature, and sunlight irradiations. The features of this block are fast system response, optimal size, low cost for development, and high robustness. It provides fluctuated fuel stack system voltage, gives a low level of accuracy for identifying the MPP, and has less capability for handling the quick variations of the fuel system hydrogen pressure. The limitations of these fractional network voltage plus fractional source currents are overcome by selecting a Modified Perturb and Observe (MP&O) network 20. In this MP&O network, the fuel stack operational water membrane value, and pressurized hydrogen are adjusted until the system provides the maximum possible peak voltage. Also, the quadratic converter system switching signals value is modified to achieve high source voltage conversion efficiency. The demerits of this type of MP&O system are less relevant to the fast deviation of the fuel stack water membrane value, more fuel system current disturbances, and more heating issues21,22,23.
The variable fuel stack network resistance-based MPPT methodology is considered in the article24 for matching the wind network source voltage with fuel stack-dependent electric vehicle voltage. In this equivalent system resistance variation controller, the current density of the fuel stack, and hydrogen pressure variables are collected as the source signals to this MPPT block for evaluating the suitable duty value for the interleaved two-phase DC–DC power conversion circuit. The merits of this incremented fuel stack resistance MPPT controller are providing low oscillated converter voltage, better accuracy when associated with the P&O network, more flexibility, plus good steady-state behavior25. However, it may not give a proper converter load power, and high difficult to handle the controller operation. The slider technology is proposed in the article26 for capturing the whole sunlight intensity, and fuel stack hydrogen pressure to run the smart grid network with a uniform power factor thereby enhancing the consumer power utilization factor. Here, the sliding concept is helpful for through-the-day power extortion from the hybrid fuel stack, and sunlight power production network. However, these conventional methodologies take more convergence time, require more space for fixing, and are unable to work at fast changes in fuel stack operational temperature, and water membrane value conditions27. In this work, the advanced hybrid IVSGA-ANFIS controller is proposed for a polymer membrane fuel system to optimize the fuel stack production current thereby reducing the overall system heating and power conduction losses. The features of this hybridization method are quick power point identification, ease of handling with more system efficiency, good fuel stack supply power, and low-level load voltage distortions. The integration of the IVSGA-ANFIS controller with a boost DC–DC power conversion circuit is given in Fig. 2.
Finally, the fuel system voltage supply capability is low for hydrogen power automotive systems. So, the DC–DC converter circuits are applied to the all-electric vehicle networks to boost the consumer load efficiency. From the literature study, the converters are in two categories which are isolated supply-based DC–DC circuits, plus transformerless power DC–DC circuits. The flyback isolated technology is selected in28,29,30 for the battery and fuel stack-based four-wheeler hydrogen vehicle network for quick charging of the battery and this converter has the capability of wide load voltage conversion ratio and is capable of giving multiple types of load voltages. However, this flyback technology generates more distortion and noise load power. Also, its functioning efficiency and power density are less. So, the feedforward technology-based converter topology is developed in the article31 for supplying energy to the electric vehicle charging substation. The merits of this topology are quick system response to external disturbances, more voltage transmission efficiency, and helps the automotive system to run in bad weather conditions. However, the isolated technology troubles the hydrogen vehicle by increasing its size and weight. Also, it consists of a separate rectifier for increasing the voltage gain of the system. Due to these additional components, the entire network cost is increased excessively. In this work, a single-switch conventional power conversion circuit is utilized for enhancing the fuel system voltage.
Analysis and implementation of PEM fuel cell
From the literature study, the conventional power production networks needed more space for installation and were unable to carry along with the electric vehicle systems. So, the present electric vehicle manufacturing company is focusing on renewable sources for the effective utilization of battery-dependent EV networks32. The sunlight networks and wind energy systems are not used for the electrical vehicle industry because of less continuity in the energy supply and their operational behavior is entirely dependent on the nature conditions. Also, this category of renewable energy networks is useful for only battery-related four-wheeler vehicles. So, heavy-duty electric vehicles refer to the fuel cell-based renewable power source for operating the vehicle without any external disturbances. In33, the researchers studied the solid oxide-oriented fuel stack technology for backup power application. Also, this cell is utilized in auxiliary power supply and distribution energy networks. The utilized source chemicals for this solid oxide cell are CO, O2, H2, and pure atmospheric air. The maximum operational temperature, efficiency, and power wattage of this fuel system are 700–900 °C, 58%, and 3 MW. The advantages of this cell are fuel flexibility, the electrolyte being completely solid, suitable for hybridization of wind and solar systems, better water membrane efficiency, and the ability to work with various electrolyte chemicals. However, the demerits of the solid oxide system are high corrosion issues, and easy breakdown of its external elements34.
In this work, the PEM fuel cell device is considered for giving energy to the standalone system. The working structure of the polymer electrolyte fuel system and its electrical operational circuit are given in Fig. 3a,b. The major features of this utilized fuel stack are the ability to operate at low supply temperature, the best suitable for supplying the energy to the battery, less corrosion issues because of the polymer electrolyte, less electrolyte management issues, and quick system dynamic response even at very low-level system temperature. The main challenge involved in this cell is costly electrolytes which is compensated by improving the operational efficiency of the system. Here, from Fig. 3a, the pure oxygen and H2 chemicals are sent to the inputs of the fuel stack at the cathode and anode for producing the electricity. In this stack, the electrodes are covered by using the porous material, and the polymer electrolyte is merged in between the platinum alloy materials for running the overall system at various operational temperature conditions. From Fig. 3b, the chemical reactions of the selected fuel stack system are given in Eq. (1), and Eq. (3). The fuel network generated I-V and I-P curves are given in Fig. 4a, and Fig. 4b.
Based on Eq. (1), the fuel cell network produced voltage (VFC) is below the nominal value which may not be sufficient for the automotive networks. So, the multiple cells (n) are integrated to form a single module to improve the voltage production capability of the entire system. From the equivalent circuit, the total generated fuel system voltage (Ventire) is derived in Eq. (4). From Eq. (4), the entire fuel system voltage is completely dependent on the active region generated voltage (VAok), open circuited-based fuel system voltage (VOpv), plus concentrated fuel stack voltage (VCoc). Also, the PEM fuel stack operational efficiency is affected by the ohmic region power losses of the network. From Eq. (6), the parameters TFtc, \({\text{P}}_{{{\text{O}}_{2} }}\), and \({\text{P}}_{{{\text{H}}_{2} }}\) are named as fuel system operational temperature, oxygen pressure at the cathode, and anode side hydrogen pressure. The cell anode electrode humidity pressure and cathode electrode humidity pressures are defined as \({\text{RH}}_{{{\text{Ace}}}}\), and \({\text{RH}}_{{{\text{Cce}}}}\) respectively. Finally, the cell-produced current and its available water vapor are defined as Icc, and \({\text{P}}_{{{\text{H}}_{2} {\text{O}}}}^{{{\text{sat}}}}\). Similarly, the empirical variables of the PEM fuel cell and its associated resistance and Faraday constants are represented as M1, M2, M3, M4, R, and F. The fuel stack permeability at different operational water membrane conditions is mentioned in Eq. (15). The utilized values for the design of the selected fuel stack are mentioned in Table 1.
Comprehensive analysis of MPPT controllers
From the previously existing articles, it is observed that the fuel stack is one of the renewable power production systems and its behavior is completely nonlinear. As a result, the entire fuel system's operational position changes from one local region to another local region, and the extraction of peak voltage from the source is highly difficult35. So, the scholars referred to the power point identifier for the renewable energy network for achieving more source power. In the introduction section, it has been observed that the unique artificial intelligence controllers may not be suitable for the rapid change of the system operational temperature and also, it depends on the fuel stack modeling36. In this article, the improved adaptive GA is developed along with the ANFIS block for obtaining more power from the source network with high functioning efficiency. Here, the proposed power point identifier is compared with Improved Hill Climb-Adaptive Fuzzy Logic Controller (IHC-AFLC)37, Modified Differential Evolutionary included FLC (MDV-FLC)38, Modified Variable Beta parameter based FLC (MVB-FLC)39, Differentiated Step Incremental Conductance with FLC (DSIC-FLC), Adaptive Marine Predators Optimized FLC (AMPO-FLC)40 in terms of development complexity of controller, fuel system MPP tracking speed, accuracy of the utilized controller, and dependence on fuel stack modeling.
Improved hill climb-adaptive fuzzy logic controller
The most frequently applied power point tracking method in the remote traffic controlling system is hill climb because its design is very easy, low level of complexity in development, low power rating sensors are required to sense the fuel stack parameters, and it low space is utilized in the traffic controlling device for fixing41. However, this single controller may not apply to the electric vehicle application. So, the hill climb block is added with the fuzzy network to reduce the tracing time of the fuel stack operational point. In this hill climb-fuzzy methodology, at the starting point of the V-I curve of the fuel network, the fuzzy technology is considered for moving the functioning point of the proposed fuel stack network from the general local MPP region to the actual peak power point position. Later the fuzzy controlled fuel stack error signal is feedback to the hill climb block for stabilizing the functioning point of the overall system42. The fuzzy error parameter (e), and adjustment in its error (δe) value are mentioned in Eq. (16).
where the parameters \({\text{P}}_{{{\text{FSn}}}} \left( {\text{j}} \right),{\text{V}}_{{{\text{FSn}}}} \left( {\text{j}} \right),{\text{P}}_{{{\text{FSn}}}} \left( {{\text{j}} - 1} \right)\), and \({\text{V}}_{{{\text{FSn}}}} \left( {{\text{j}} - 1} \right)\) are represented as the overall fuel system generated powers, and voltages at an instant and past. Similarly, the constant, and variable duty signals generated from the IHC-AFLC are D(j), and D(j − 1) respectively.
Modified differential evolutionary included FLC MPPT controller
The artificial neural network controllers have the demerits of more fuel stack functioning data sets being required, huge computational complexity, less accuracy in the identification of the fuel system operational point, large data samples needed for achieving the required target, and less robustness. So, the research scholars proposed the differential evolutionary concept for the electric vehicle system to improve the functioning speed of the system43,44,45. The merits of this differential evolutionary block in the fuel system are low MPP identifying time and fewer operators to achieve the best optimal solution. Also, its design is very easy and helps to do the global search for identifying the exact MPP place. Here, there are two-dimensional vectors are used in each iteration of the differential evolutionary controller for searching the overall system target value. The required papulation (Pn) size in this algorithm is much less. After receiving the 1st target results of the fuel stack, there are three more additional vectors and mutation factor (g) are selected for adjusting the weights of the population as mentioned in Eq. (19).
where the variable Vdor is identified as the donor vector, and the terms Ps1, Ps2, and Ps3 are available papulations. From Eq. (20), the donor vector (Vdor) vector value is added with the mutation value for generating the trailing vector (tvec). Based on this tvec variable, the fuel stack works at the global peak power position of the network. However, this differential algorithm creates the oscillations in the system voltage which are optimized by utilizing the fuzzy concept.
Modified variable beta parameter-based FLC MPPT controller
From the literature review, the fuzzy sets are derived from the modified classical sets and its degree of membership value is constant which is either zero or one46. The fuzzy systems are utilized in the defense, aerospace systems, electronic devices, transportation systems, and marine networks. However, the main issue of the fuzzy network is it does not have a particular strategy to sort out the particular complex problem. Also, it gives less accurate results for the imprecise supply data. So, in47, the variable step-oriented Beat (β) methodology is applied for the hybrid fuel stack, battery, and wind energy systems for producing the switching waveforms for the three-phase quadratic DC–DC conversion circuit. This beta technology helps the hybrid energy system produce more supply voltage of the system under quick variations of the sunlight intensity conditions. Also, this beta block helps the fuzzy network identify the accurate membership values for the hybrid MPPT controller48. After completion of beta technology, the fuzzy tries to maintain a uniform power supply to the automotive system. The gravitational methods and min–max technologies are selected for the efficient fuzzification process in the proposed controller. The identification of the global maximum peak voltage of the system by using this beta hybrid controller is illustrated in Eq. (21).
where the variables N define the step variation of the algorithm and VFsn, IFsn is the fuel network voltage supply and current supply.
Differentiated step incremental conductance (IC) with FLC MPPT controller
The fractional current, and power feedback MPPT controllers give high power distortion in the battery and fuel stack system. As a result, the fuel stack system gets heated up and its associated fuel system membrane gets damaged49. So, the incremental conductance technology is considered in50 for controlling the battery charging systems. In this controller, the selected fuel stack source signals are voltage and load current as mentioned in Fig. 5. From Fig. 5, the incremental conductance methodology is selected to identify the exact slope value of the fuel stack V-I curve. Here, the generally available slope value of the fuel stack system is compared with the last stored V-I curve slope value. From the fuel stack slope comprehensive analysis, the local operational point of the system moves to the actual functioning point of the system. However, this conventional method makes disturbances in the converter load power and gives excessive oscillations in the MPP position under rapid variation of the fuel stack water membrane conditions. So, this differential step IC is combined with the fuzzy network for optimizing the slope error of the V-I curve. From Fig. 5, the terminologies ΨPFc, and ΨVFc are the adjustable fuel system power and its associated supply voltages. Similarly, the variables RP, RP-new, and RP-old are the fuel network I-V curve present slope, newly obtained slope, and old slope value.
Marine predators algorithm-based fuzzy MPPT controller
The adjusted marine predator’s technology is developed from the widespread foraging behavior and it needs a high number of iteration counts for selecting the suitable fuel network MPP position. As a result, the marine predator block produces high-level interleaved converter voltage distortions51. Also, it needs more convergence time and provides more operational efficiency under different fuel stack constant temperature conditions. The disadvantages of this marine predator controller are low accuracy, high development cost, low robustness, and less useful for the quick variation of atmospheric conditions. So, this optimization block is added to the modified fuzzy system to improve the fuel stack system efficiency52. The fuzzy system has two parameters which are fuel stack source voltage, and fuel network current. In this system, there are 7-membership values are used which are Zero level state (Z-state), 1st negative state (NS-1), 2nd negative state (NS-2), 3rd negative state (NS-3), 1st positive state (PS-1), 2nd positive state (PS-2), and 3rd positive state (PS-3). Here, the seven fuzzy levels generate the 49 rules as mentioned in Table 2. The identified fuzzy membership shapes for this hybrid power point identifier are given in Fig. 6 and its absolute error value depends on the variation of the fuel system power and source current.
where s represents the fuzzy rule for the particular membership (W) and the sawtooth waveform is compared with the fuzzy output signal for operating the boost converter circuit fed electric vehicle system.
Proposed IVS genetic algorithm-ANFIS MPPT controller
Identification of the peak power point of the fuel stack-powered hydrogen vehicle is a very difficult task because of its continuous changes in the hydrogen pressure, rate change of oxidization reaction, and fast change of water membrane values53. So, the modified genetic optimization methodology dependent ANFIS controller is developed in this article for improving the power conversion efficiency of the hydrogen-based vehicle. This proposed hybrid GA with ANFIS methodology consists of three stages which are the selection of the suitable temperature value for the fuel system, and in the 2nd state, the selection of the different data sets for training the ANFIS block. Finally, in the 3rd state, the identification of suitable switching signals to the power converter circuit. Here, the ANFIS block data sets are selected by utilizing the Backpropagation concept and this backpropagation helps the hybrid network for extracting the maximum possible output power from the fuel system under various hydrogen pressure conditions. The working flow of the genetic operation and its operator selection are mentioned in Fig. 7. Also, the production of the duty signal for the converter network by using ANFIS is illustrated in Fig. 8.
From Fig. 8, the fuel stack source voltage and its associated load currents are fed to the ANFIS first layer. From the first layer, the ANFIS block involves the four membership variables which are S1, S2, K1. and K2. Here, the Takagi–Sugeno concept is included in this network for obtaining the rules of the proposed system which are given in Eq. (25), and Eq. (26). From Eq. (26), the variables t, y, and q are subsequent parameters. Finally, the variables N, j, \({\text{n}}_{{1,{\text{j}}}}\), and \(\S\) are the output of the network, total nodes in the ANFIS structure, and membership values for the source signals Q and R.
Performance analysis of power boost DC–DC converter
From the literature study, the isolated technology-based power transformation circuits involve additional power switches and transformers. As a result, the overall fuel stack power production system cost and its associated size are increased54. In this work, a transformerless power DC–DC conversion circuit is integrated with the hydrogen-powered electric vehicle network to improve the power transformation efficiency of the fuel system. Due to this single-switch boost conversion circuit, the fuel network power conduction and membrane heating losses are optimized55. Also, it does not need any extra four switches rectifier DC–DC circuits. The features of this selected power converter circuit are low-level understanding complexity, high simplicity in design, more flexibility for all atmospheric conditions, more robustness, and best suitable for medium to high voltage applications. The switching states of the selected transformerless power transformation circuits are given in Fig. 9a–c56. From Fig. 9b, the switch starts running from the ideal state to forward bias mode then the maximum source voltage is transferred to load which is mentioned in Eq. (34).
where the variables Ti, Vj, VFC, IFC, and Ij are named as converter circuit operating time, fuel stack source, and resistive load voltages and currents. Finally, the terminologies Rj, and RFC are the load equivalent resistance and fuel system resistance.
Comprehensive analysis of simulation results
The proposed conventional power transmission-based hydrogen power network is designed by selecting MATLAB/Simulink tool. Here, the polymer membrane-based fuel module is utilized because its advantages are more energy density, fast system dynamic behavior, better steady-state reliability, simplicity in maitainence, long life operation, and low electrolyte handling problems. Also, it does not have any corrosion-related issues. Due to these merits, the PEM fuel stack is used for remotely located household applications, battery charging applications, and most useful for distribution power generation systems. The mathematical modeling of the polymer membrane fuel system and its development parameters selection are mentioned in Section "Analysis and implementation of PEM fuel cell". However, the major problem of the fuel network is more current production to the load. Due to this excess current flow in the distribution network, the overall hydrogen-powered vehicle system efficiency is reduced. So, the single switch voltage transmission DC–DC circuit is connected to the load of the fuel stack for enhancing the fuel stack utilization factor. The design structure of the proposed converter is mentioned in Fig. 9.
Study of the IVSGA-ANFIS fed PEM fuel cell at static 344Kelvin temperature
From Fig. 9, the selected proposed converter source capacitive (Cin) value is 180µF which helps the system to reduce the source power fluctuations and improve the voltage stability of the consumer load. Similarly, the utilized source inductor (Lk) value is 2.24mH and it suppresses the fuel stack current distortions thereby supplying the uniform current to the electric vehicle. In this utilized circuit, the Metal Oxide Semiconductor Field Effect Transistor (MOSFET) is included to withstand the high voltages of the fuel system. Due to this MOSFET, the converter can function at high operational temperature and frequency conditions. The operational frequency of this fuel system power production network is 22kHZ. In addition to this feature, the selected switch provides low-level source resistance and extended level voltage transformation ratio of the system. The load capacitive (Cj), and resistive element (Rj) values are 240µF, and 67Ω respectively.
The initial testing temperature of this fuel system is 344 K as mentioned in Fig. 10. At this static testing source temperature condition, the evaluated fuel network, and DC–DC circuit fed DSIC-FLC, IHC-AFLC, MVB-FLC, MDV-FLC, AMPO-FLC, and IVSGA-ANFIS MPPT controllers produced currents, voltages, available powers and their associated efficiencies are 109.68 A, 42.238 V, 4632.77 W, 9.0654 A, 499.09 V, 4523.67 W, 97.6459%, 110.10 A, 42.489 V, 4678.11 W, 9.1782 A, 499.45 V, 4584.08 W, 97.9967%, 110.22 A, 42.428 V, 4676.45 W, 9.1946 A, 499.86 V, 4596.02 W, 98.2896%, 110.78 A, 42.114 V, 4665.40 W, 9.2015 A, 499.97 V, 4600.56 W, 98.6190%, 111.90 A, 42.353 V, 4739.40 W, 9.316 A, 501.84 V, 4675.42 W, 98.6549%, 112.89 A, 42.074 V, 4749.83 W, 9.324 A, 502.99 V, 4689.99 W, and 98.7402% respectively. From Fig. 11a, the maximum possible fuel supply voltage by integrating the MDV-FLC power point tracking controller is more when associated with the DSIC-FLC and IHC-AFLC controllers. Also, its development complexity is moderate and gives low-level fuel system current distortions as mentioned in Fig. 11b. From Fig. 11c, the proposed power point identifying controller-fed PEM fuel system power is high when equated with the conventional methodologies. Similarly, the fuel network-produced voltage is improved by applying the single-switch voltage transmission converter which is illustrated in Fig. 11d. Here, the converter boosts up the source voltage from twelve to thirteen times the supply voltage. Also, the converter steps down the entire fuel system source current to optimize the system heating losses. From Fig. 11e, the generated converter current and its related settling time by selecting the IVSGA-ANFIS MPPT controller are 9.324 A, and 0.01607 s respectively. Finally, From Fig. 11f, the converter production power at this static operational temperature is uniform from 0 s to 0.3 s, and it varies whenever the fuel stack supply temperature varies. Also, the fuel stack performance changes concerning its hydrogen decomposition and chemical reaction rate.
Study of the IVSGA-ANFIS fed PEM fuel cell at dynamic 344 K, 324 K, and 304 K
In this case, the continuous variation of the fuel network temperature is utilized for investigating the proposed power point identifier which is equal to 344 K, 324 K, and 304 K respectively. In this case, the fuel cell-based converter system produced voltage more when equated to the static condition because of its sudden increment of the operational temperature of the source. Here, the converter functioning duty values at this dynamic operational temperature condition by applying the MVB-FLC, MDV-FLC, and AMPO-FLC controllers are 0.54, 0.58, and 0.61 respectively.
At 304 K, from Fig. 12b, 12(a), 12(c), 12(e), 12(d), and 12(f), the measured fuel stack and DC–DC circuit produced currents, generated voltages and powers by interfacing the DSIC-FLC, IHC-AFLC, MVB-FLC, MDV-FLC, AMPO-FLC, and IVSGA-ANFIS methodologies and its obtained associated efficiencies are 103.20 A, 37.381 V, 3857.72 W, 7.6677 A, 482.43 V, 3699.17 W, 95.8972%, 103.21 A, 37.399 V, 3860.90 W, 7.6845 A, 486.65 V, 3739.67 W, 96.8629%, 104.00 A, 37.701 V, 3921.08 W, 7.7825 A, 488.16 V, 3799.14 W, 96.897%, 104.14 A, 38.255 V, 3983.91 W, 7.899 A, 489.89 V, 3869.66 W, 97.1326%, 104.99 A, 38.254 V, 4016.32 W, 7.9250 A, 492.19 V, 3900.65 W, 97.1203%, 107.31 A, 37.826 V, 4059.11 W, 7.9651 A, 494.89 V, 3941.89 W, and 97.112% respectively. The converter voltage settling time and its power-generated distortions are mentioned in Table 3.
Conclusion
In this work, the first objective is the identification of a suitable fuel cell for the electric vehicle application. Here, the polymer membrane fuel system is considered because of its advantages are more energy density, less corrosion effect on polymer membrane, easy handling, and better dynamic behavior at rapid change of operational temperature conditions. Also, it gives a very fast system response. However, the main challenge of this fuel stack is the handling of nonlinear power generation and it is not acceptable for the electric vehicle application. So, the IVSGA-ANFIS controller is proposed in this article for tracking the functioning point of the fuel cell system thereby extracting the maximum possible output voltage from the proposed system. From the simulation results, this proposed hybrid MPPT controller gives more accurate fuel stack voltage, less convergence time, less steady-state oscillations of the MPP, more peak power extraction efficiency, and easy understanding. Finally, in the third objective, the single-switch DC–DC converter circuit is integrated with the proposed system to optimize the fuel system generation current. The advantages of this converter are easy design and low development cost.
Data availability
The datasets used and/or analyzed during the current study are available from the corresponding author upon reasonable request.
References
Sebestyén, V. Renewable and sustainable energy reviews: Environmental impact networks of renewable energy power plants. Renew. Sustain. Energy Rev. 151, 111626 (2021).
Pathak, P. K., Yadav, A. K., & Alvi, P. A. Maximum power operation of SPV system using advanced FL based control strategy. In: 2019 8th International Conference on Power Systems (ICPS) (IEEE, 2019).
Nassar, Y. F. et al. Assessing the viability of solar and wind energy technologies in semi-arid and arid regions: A case study of Libya’s climatic conditions. Appl. Solar Energy 60(1), 149–170 (2024).
Pathak, P. K., Yadav, A. K. & Alvi, P. A. A state-of-the-art review on shading mitigation techniques in solar photovoltaics via meta-heuristic approach. Neural Comput. Appl. 66, 1–39 (2022).
Pathak, P. K. et al. Fuel cell-based topologies and multi-input DC–DC power converters for hybrid electric vehicles: A comprehensive review. IET Gener. Transm. Distrib. 16(11), 2111–2139 (2022).
Basha, C. H. H. & Rani, C. Different conventional and soft computing MPPT techniques for solar PV systems with high step-up boost converters: A comprehensive analysis. Energies 13(2), 371 (2020).
Govinda Chowdary, V., et al. Hybrid fuzzy logic-based MPPT for wind energy conversion system. In: Soft Computing for Problem Solving: SocProS 2018, Volume 2 (Springer, 2020).
Yaghoubi, E., et al. A systematic review and meta-analysis of machine learning, deep learning, and ensemble learning approaches in predicting EV charging behavior. Eng. Appl. Artif. Intell. 135, 108789 (2024).
Lu, Y. et al. Adaptive disturbance observer-based improved super-twisting sliding mode control for electromagnetic direct-drive pump. Smart Mater. Struct. 32(1), 017001 (2022).
Prashanth, V. et al. Implementation of high step-up power converter for fuel cell application with hybrid MPPT controller. Sci. Rep. 14(1), 3342 (2024).
Rafikiran, S., Basha, C. H. H. & Dhanamjayulu, C. A novel hybrid MPPT controller for PEMFC fed high step-up single switch DC–DC converter. Int. Trans. Electr. Energy Syst. 1, 9196747 (2024).
Mariprasath, T. et al. A novel on high voltage gain boost converter with cuckoo search optimization based MPPTController for solar PV system. Sci. Rep. 14(1), 8545 (2024).
Yang, Y. et al. Electrocatalysis in alkaline media and alkaline membrane-based energy technologies. Chem. Rev. 122(6), 6117–6321 (2022).
Ozturk, M. & Dincer, I. A comprehensive review on power-to-gas with hydrogen options for cleaner applications. Int. J. Hydrog. Energy 46(62), 31511–31522 (2021).
Yang, J. & He, Q. Scheduling parallel computations by work stealing: A survey. Int. J. Parallel Prog. 46(2), 173–197. https://doi.org/10.1007/s10766-016-0484-8 (2018).
Basha, C. H. H. & Rani, C. A new single switch DC–DC converter for PEM fuel cell-based electric vehicle system with an improved beta-fuzzy logic MPPT controller. Soft Comput. 26(13), 6021–6040 (2022).
Rafikiran, S. et al. Design and performance analysis of hybrid MPPT controllers for fuel cell fed DC–DC converter systems. Energy Rep. 9, 5826–5842 (2023).
Murali, M., et al. Design and analysis of neural network-based MPPT technique for solar power-based electric vehicle application. In: Proceedings of Fourth International Conference on Inventive Material Science Applications: ICIMA 2021 (Springer, 2022).
Zhang, J. et al. A Novel Multiple-Medium-AC-Port Power Electronic Transformer. IEEE Trans. Ind. Electron. https://doi.org/10.1109/TIE.2023.3301550 (2023).
Mousa, H. H. H., Youssef, A.-R. & Mohamed, E. E. M. State of the art perturb and observe MPPT algorithms based wind energy conversion systems: A technology review. Int. J. Electr. Power Energy Syst. 126, 106598 (2021).
Raiker, G. A. & Loganathan, U. Current control of boost converter for PV interface with momentum-based perturb and observe MPPT. IEEE Trans. Ind. Appl. 57(4), 4071–4079 (2021).
Reddy, K. R. et al. A novel on energy management strategy with maximum exploitation of renewables and EV storage in distribution networks. Int. Trans. Electr. Energy Syst. 1, 1365608 (2023).
Basha, C. H. H. et al. "A novel on intelligent energy control strategy for micro grids with renewables and EVs. Energy Strategy Rev. 52, 101306 (2024).
Puppala, R. et al. Framework for smart grid to implement a price elasticity-based peak time rebate demand response program. Front. Energy Res. 10, 1079695 (2023).
Jately, V. et al. Voltage and current reference based MPPT under rapidly changing irradiance and load resistance. IEEE Trans. Energy Convers. 36(3), 2297–2309 (2021).
Yu, F., Lu, C., Zhou, J. & Yin, L. Mathematical model and knowledge-based iterated greedy algorithm for distributed assembly hybrid flow shop scheduling problem with dual-resource constraints. Expert Syst. Appl. 239, 122434. https://doi.org/10.1016/j.eswa.2023.122434 (2024).
Moghadari, M. et al. Operating cost comparison of a single-stack and a multi-stack hybrid fuel cell vehicle through an online hierarchical strategy. IEEE Trans. Veh. Technol. 72(1), 267–279 (2022).
Mou, J. et al. Biologically inspired machine learning-based trajectory analysis in intelligent dispatching energy storage system. IEEE Trans. Intell. Transp. Syst. 24(4), 4509–4518. https://doi.org/10.1109/TITS.2022.3154750 (2023).
Huang, Y. et al. Collaborative on-demand dynamic deployment via deep reinforcement learning for IoV service in multi edge clouds. J. Cloud Comput. 12(1), 119. https://doi.org/10.1186/s13677-023-00488-6 (2023).
Basha, C. H. H., Rani, C. & Odofin, S. A review on non-isolated inductor coupled DC–DC converter for photovoltaic grid-connected applications. Int. J. Renew. Energy Res. 7(4), 1570–1585 (2017).
Bao, Y. et al. Adaptive feedforward compensation for voltage source disturbance rejection in DC–DC converters. IEEE Trans. Control Syst. Technol. 26(1), 344–351 (2017).
Basha, C. H. & Murali, M. A new design of transformerless, non-isolated, high step-up DC–DC converter with hybrid fuzzy logic MPPT controller. Int. J. Circuit Theory Appl. 50(1), 272–297 (2022).
Pathak, P. K. & Yadav, A. K. Design of battery charging circuit through intelligent MPPT using SPV system. Solar Energy 178, 79–89 (2019).
Yang, Z. et al. A short review of cathode poisoning and corrosion in solid oxide fuel cell. Int. J. Hydrog. Energy 42(39), 24948–24959 (2017).
Zhang, J. et al. An embedded DC power flow controller based on full-bridge modular multilevel converter. IEEE Trans. Ind. Electron. 71(3), 2556–2566. https://doi.org/10.1109/TIE.2023.3265041 (2023).
Dida, A. & Attous, D. B. Adaptive hill-climb searching method for MPPT algorithm based DFIG system using fuzzy logic controller. Int. J. Syst. Assur. Eng. Manag. 8, 424–434 (2017).
Wati, D. A. R. Maximum power point tracking of photovoltaic systems using simple interval type-2 fuzzy logic controller based on hill climbing algorithm. In: 2016 International Seminar on Intelligent Technology and Its Applications (ISITIA) (IEEE, 2016).
Tey, K. S. et al. Improved differential evolution-based MPPT algorithm using SEPIC for PV systems under partial shading conditions and load variation. IEEE Trans. Ind. Inform. 14(10), 4322–4333 (2018).
Li, X. et al. A novel beta parameter based fuzzy-logic controller for photovoltaic MPPT application. Renew. Energy 130, 416–427 (2019).
Aly, M. et al. Marine predators algorithm optimized reduced sensor fuzzy-logic based maximum power point tracking of fuel cell-battery standalone applications. IEEE Access 9, 27987–28000 (2021).
Yu, F., Yin, L., Zeng, B., Lu, C. & Xiao, Z. A self-learning discrete artificial bee colony algorithm for energy-efficient distributed heterogeneous L–R fuzzy welding shop scheduling problem. IEEE Trans. Fuzzy Syst. 32(6), 3753–3764. https://doi.org/10.1109/TFUZZ.2024.3382398 (2024).
Rezk, H. et al. Design and hardware implementation of new adaptive fuzzy logic-based MPPT control method for photovoltaic applications. IEEE Access 7, 106427–106438 (2019).
Yu, F., Lu, C., Yin, L. & Zhou, J. Modeling and optimization algorithm for energy-efficient distributed assembly hybrid flowshop scheduling problem considering worker resources. J. Ind. Inf. Integr. 40, 100620. https://doi.org/10.1016/j.jii.2024.100620 (2024).
Mahmood, T. et al. An approach toward decision-making and medical diagnosis problems using the concept of spherical fuzzy sets. Neural Comput. Appl. 31, 7041–7053 (2019).
Ullah, K. et al. On some distance measures of complex pythagorean fuzzy sets and their applications in pattern recognition. Complex Intell. Syst. 6, 15–27 (2020).
Bisht, R. & Sikander, A. An improved method based on fuzzy logic with beta parameter for PV MPPT system. Optik 259, 168939 (2022).
Gong, Q., Li, J., Jiang, Z. & Wang, Y. A hierarchical integration scheduling method for flexible job shop with green lot splitting. Eng. Appl. Artif. Intell. 129, 107595. https://doi.org/10.1016/j.engappai.2023.107595 (2024).
Liang, J. et al. ETS-based human–machine robust shared control design considering the network delays. IEEE Trans. Autom. Sci. Eng. https://doi.org/10.1109/TASE.2024.3383094 (2024).
Selman, N. H. & Mahmood, J. R. Comparison between perturb & observe, incremental conductance and fuzzy logic MPPT techniques at different weather conditions. Int. J. Innov. Res. Sci. Eng. Technol. 5(7), 12556–12569 (2016).
Liang, J. et al. A direct yaw moment control framework through robust T–S fuzzy approach considering vehicle stability margin. IEEE/ASME Trans. Mechatron. 29(1), 166–178. https://doi.org/10.1109/TMECH.2023.3274689 (2024).
Farayola, A. M., Hasan, A. N. & Ali, A. Implementation of modified incremental conductance and fuzzy logic MPPT techniques using MCUK converter under various environmental conditions. Appl. Solar Energy 53, 173–184 (2017).
Cao, B. et al. Multiobjective evolution of fuzzy rough neural network via distributed parallelism for stock prediction. IEEE Trans. Fuzzy Syst. 28(5), 939–952. https://doi.org/10.1109/TFUZZ.2020.2972207 (2020).
Tao, H. et al. A novel MPPT controller in PV systems with hybrid whale optimization-PS algorithm based ANFIS under different conditions. Control Eng. Pract. 112, 104809 (2021).
Mumtaz, F. et al. Review on non-isolated DC–DC converters and their control techniques for renewable energy applications. Ain Shams Eng. J. 12(4), 3747–3763 (2021).
Alhurayyis, I., Elkhateb, A. & Morrow, J. Isolated and nonisolated DC-to-DC converters for medium-voltage DC networks: A review. IEEE J. Emerg. Sel. Top. Power Electron. 9(6), 7486–7500 (2020).
Lakshmi, M. & Hemamalini, S. Nonisolated high gain DC–DC converter for DC microgrids. IEEE Trans. Ind. Electron. 65(2), 1205–1212 (2017).
Acknowledgements
This work was supported by the Researchers Supporting Project Number (RSPD2024R646), King Saud University, Riyadh, Saudi Arabia.
Funding
The authors did not receive support from any organization for the submitted work.
Author information
Authors and Affiliations
Contributions
All authors contributed to the study, conception, and design. All authors commented on the manuscript. All authors read and approved the final manuscript.
Corresponding author
Ethics declarations
Competing interests
The authors declare no competing interests.
Ethical approval
This paper does not contain any studies with human participants or animals performed by any of the authors.
Additional information
Publisher's note
Springer Nature remains neutral with regard to jurisdictional claims in published maps and institutional affiliations.
Rights and permissions
Open Access This article is licensed under a Creative Commons Attribution-NonCommercial-NoDerivatives 4.0 International License, which permits any non-commercial use, sharing, distribution and reproduction in any medium or format, as long as you give appropriate credit to the original author(s) and the source, provide a link to the Creative Commons licence, and indicate if you modified the licensed material. You do not have permission under this licence to share adapted material derived from this article or parts of it. The images or other third party material in this article are included in the article’s Creative Commons licence, unless indicated otherwise in a credit line to the material. If material is not included in the article’s Creative Commons licence and your intended use is not permitted by statutory regulation or exceeds the permitted use, you will need to obtain permission directly from the copyright holder. To view a copy of this licence, visit http://creativecommons.org/licenses/by-nc-nd/4.0/.
About this article
Cite this article
Rafi Kiran, S., Alsaif, F. A novel advanced hybrid fuzzy MPPT controllers for renewable energy systems. Sci Rep 14, 21104 (2024). https://doi.org/10.1038/s41598-024-72060-4
Received:
Accepted:
Published:
DOI: https://doi.org/10.1038/s41598-024-72060-4
- Springer Nature Limited