Abstract
Making causal inferences regarding human behaviour is difficult given the complex interplay between countless contributors to behaviour, including factors in the external world and our internal states. We provide a non-technical conceptual overview of challenges and opportunities for causal inference on human behaviour. The challenges include our ambiguous causal language and thinking, statistical under- or over-control, effect heterogeneity, interference, timescales of effects and complex treatments. We explain how methods optimized for addressing one of these challenges frequently exacerbate other problems. We thus argue that clearly specified research questions are key to improving causal inference from data. We suggest a triangulation approach that compares causal estimates from (quasi-)experimental research with causal estimates generated from observational data and theoretical assumptions. This approach allows a systematic investigation of theoretical and methodological factors that might lead estimates to converge or diverge across studies.
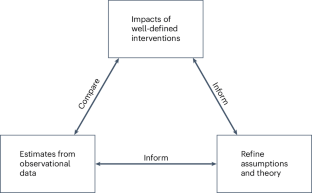
Similar content being viewed by others
Explore related subjects
Discover the latest articles, news and stories from top researchers in related subjects.References
Angrist, J. D. & Pischke, J.-S. The credibility revolution in empirical economics: how better research design is taking the con out of econometrics. J. Econ. Perspect. 24, 3–30 (2010).
Hernán, M. A. & Robins, J. M. Causal Inference: What If (Chapman & Hall/CRC, 2020).
Aronow, P. M. & Miller, B. T. Foundations of Agnostic Statistics (Cambridge Univ. Press, 2019).
Keele, L. The statistics of causal inference: a view from political methodology. Polit. Anal. 23, 313–335 (2015).
Foster, E. M. Causal inference and developmental psychology. Dev. Psychol. 46, 1454–1480 (2010).
Marinescu, I. E., Lawlor, P. N. & Kording, K. P. Quasi-experimental causality in neuroscience and behavioural research. Nat. Hum. Behav. 2, 891–898 (2018).
Rohrer, J. M. Thinking clearly about correlations and causation: graphical causal models for observational data. Adv. Methods Pract. Psychol. Sci. 1, 27–42 (2018).
Rigoux, L. & Daunizeau, J. Dynamic causal modelling of brain–behaviour relationships. NeuroImage 117, 202–221 (2015).
Gangl, M. Causal inference in sociological research. Annu. Rev. Sociol. 36, 21–47 (2010).
Winship, C. & Morgan, S. L. The estimation of causal effects from observational data. Annu. Rev. Sociol. 25, 659–706 (1999).
Imbens, G. W. & Rubin, D. B. Causal Inference in Statistics, Social, and Biomedical Sciences (Cambridge Univ. Press, 2015).
Pearl, J. Causality: Models, Reasoning, and Inference 2nd edn (Cambridge Univ. Press, 2009).
Hamaker, E. L. & Wichers, M. No time like the present. Curr. Dir. Psychol. Sci. 26, 10–15 (2017).
Angrist, J. D. & Pischke, J.-S. Mostly Harmless Econometrics: An Empiricist’s Companion (Princeton Univ. Press, 2009).
Gelman, A. & Imbens, G. Why Ask Why? Forward Causal Inference and Reverse Causal Questions Working Paper No. 19614 (NBER, 2013).
Alvarez-Vargas, D. et al. Hedges, mottes, and baileys: causally ambiguous statistical language can increase perceived study quality and policy relevance. PLoS ONE 18, e0286403 (2023).
Haber, N. A. et al. Causal and associational language in observational health research: a systematic evaluation. Am. J. Epidemiol. 191, 2084–2097 (2022).
Hernán, M. A. The C-word: scientific euphemisms do not improve causal inference from observational data. Am. J. Public Health 108, 616–619 (2018).
Rohrer, J. M. & Lucas, R. E. Causal effects of well-being on health: it’s complicated. Preprint at PsyArXiv https://doi.org/10.31234/osf.io/wgbe4 (2020).
Hoemann, K., Devlin, M. & Barrett, L. F. Comment: emotions are abstract, conceptual categories that are learned by a predicting brain. Emot. Rev. 12, 253–255 (2020).
Young, C. & Holsteen, K. Model uncertainty and robustness: a computational framework for multimodel analysis. Sociol. Methods Res. 46, 3–40 (2017).
Cinelli, C. & Hazlett, C. Making sense of sensitivity: extending omitted variable bias. J. R. Stat. Soc. B 82, 39–67 (2020).
Branwen, G. How often does correlation = causality? Gwern.net https://www.gwern.net/Correlation (2022).
Runge, J. Causal network reconstruction from time series: from theoretical assumptions to practical estimation. Chaos 28, 075310 (2018).
Oster, E. Health recommendations and selection in health behaviors. Am. Econ. Rev. Insights 2, 143–160 (2020).
VanderWeele, T. J. Constructed measures and causal inference: towards a new model of measurement for psychosocial constructs. Epidemiology 33, 141–151 (2022).
Greenland, S., Judea, P. & Robins, J. M. Causal diagrams for epidemiologic research. Epidemiology 10, 37–48 (1999).
Rosenbaum, P. R. From association to causation in observational studies: the role of tests of strongly ignorable treatment assignment. J. Am. Stat. Assoc. 79, 41–48 (1984).
Hoyle, R. H., Lynam, D. R., Miller, J. D. & Pek, J. The questionable practice of partialing to refine scores on and inferences about measures of psychological constructs. Annu. Rev. Clin. Psychol. 19, 155–176 (2023).
Cinelli, C., Forney, A. & Pearl, J. A crash course in good and bad controls. Sociol. Methods Res. https://doi.org/10.1177/00491241221099552 (2022).
Wysocki, A. C., Lawson, K. M. & Rhemtulla, M. Statistical control requires causal justification. Adv. Methods Pract. Psychol. Sci. 5, 251524592210958 (2022).
Elwert, F. & Winship, C. Endogenous selection bias: the problem of conditioning on a collider variable. Annu. Rev. Sociol. 40, 31–53 (2014).
Knox, D., Lowe, W. & Mummolo, J. Administrative records mask racially biased policing. Am. Polit. Sci. Rev. 114, 619–637 (2020).
Bryan, C. J., Tipton, E. & Yeager, D. S. Behavioural science is unlikely to change the world without a heterogeneity revolution. Nat. Hum. Behav. 5, 980–989 (2021).
Haslbeck, J. M. B. & Ryan, O. Recovering within-person dynamics from psychological time series. Multivar. Behav. Res. 57, 735–766 (2022).
Goldsmith-Pinkham, P., Hull, P. & Kolesár, M. Contamination Bias in Linear Regressions Working Paper No. 30108 (NBER, 2022).
Goodman-Bacon, A. Difference-in-differences with variation in treatment timing. J. Econ. 225, 254–277 (2021).
Wu, W., Carroll, I. A. & Chen, P.-Y. A single-level random-effects cross-lagged panel model for longitudinal mediation analysis. Behav. Res Methods 50, 2111–2124 (2018).
Rubin, D. B. Causal inference using potential outcomes. J. Am. Stat. Assoc. 100, 322–331 (2005).
Altmejd, A. et al. O brother, where start thou? Sibling spillovers on college and major choice in four countries. Q. J. Econ. 136, 1831–1886 (2021).
Heckman, J. & Karapakula, G. Intergenerational and Intragenerational Externalities of the Perry Preschool Project Working Paper No. 25889 (NBER, 2019).
Karbownik, K. & Özek, U. Setting a Good Example? Examining Sibling Spillovers in Educational Achievement Using a Regression Discontinuity Design Working Paper No. 26411 (NBER, 2019).
Bringmann, L. F. et al. Psychopathological networks: theory, methods and practice. Behav. Res Ther. 149, 104011 (2022).
Dietrich, J., Schmiedek, F. & Moeller, J. Academic motivation and emotions are experienced in learning situations, so let’s study them: introduction to the special issue. Learn. Instr. 81, 101623 (2022).
Robins, J. M., Scheines, R., Spirtes, P. & Wasserman, L. Uniform consistency in causal inference. Biometrika 90, 491–515 (2003).
VanderWeele, T. J. & Hernán, M. A. Causal inference under multiple versions of treatment. J. Causal Inference 1, 1–20 (2013).
Pearl, J. Does obesity shorten life? Or is it the soda? On non-manipulable causes. J. Causal Inference 6, 20182001 (2018).
Angrist, J. D. & Pischke, J.-S. Mastering ’Metrics: The Path from Cause to Effect (Princeton Univ. Press, 2014).
Eronen, M. I. Causal discovery and the problem of psychological interventions. N. Ideas Psychol. 59, 100785 (2020).
Scheines, R. The similarity of causal inference in experimental and non-experimental studies. Phil. Sci. 72, 927–940 (2005).
Bringmann, L. F., Elmer, T. & Eronen, M. I. Back to basics: the importance of conceptual clarification in psychological science. Curr. Dir. Psychol. Sci. 31, 340–346 (2022).
Spirtes, P. & Scheines, R. Causal inference of ambiguous manipulations. Phil. Sci. 71, 833–845 (2004).
Bollen, K. A. & Brand, J. E. A general panel model with random and fixed effects: a structural equations approach. Soc. Forces 89, 1–34 (2010).
Hamaker, E. L., Kuiper, R. M. & Grasman, R. P. P. P. A critique of the cross-lagged panel model. Psychol. Methods 20, 102–116 (2015).
Zyphur, M. J. et al. From data to causes I: building a general cross-lagged panel model (GCLM). Organ. Res. Methods 23, 651–687 (2020).
Voelkle, M. C., Oud, J. H. L., Davidov, E. & Schmidt, P. An SEM approach to continuous time modeling of panel data: relating authoritarianism and anomia. Psychol. Methods 17, 176–192 (2012).
Frangakis, C. E. & Rubin, D. B. Principal stratification in causal inference. Biometrics 58, 21–29 (2002).
Beltz, A. M. & Gates, K. M. Network mapping with GIMME. Multivar. Behav. Res. 52, 789–804 (2017).
Montoya, L. M. et al. The optimal dynamic treatment rule superlearner: considerations, performance, and application to criminal justice interventions. International J. Biostat. 19, 217–238 (2023).
Gische, C. & Voelkle, M. C. Beyond the mean: a flexible framework for studying causal effects using linear models. Psychometrika 87, 868–901 (2022).
Imai, K. & Kim, I. S. When should we use unit fixed effects regression models for causal inference with longitudinal data? Am. J. Polit. Sci. 63, 467–490 (2019).
Sobel, M. E. & Lindquist, M. A. Causal inference for fMRI time series data with systematic errors of measurement in a balanced on/off study of social evaluative threat. J. Am. Stat. Assoc. 109, 967–976 (2014).
Usami, S. Within-person variability score-based causal inference: a two-step estimation for joint effects of time-varying treatments. Psychometrika 88, 1466–1494 (2022).
Hamaker, E. L., Mulder, J. D. & van IJzendoorn, M. H. Description, prediction and causation: methodological challenges of studying child and adolescent development. Dev. Cogn. Neurosci. 46, 100867 (2020).
Lundberg, I., Johnson, R. & Stewart, B. M. What is your estimand? Defining the target quantity connects statistical evidence to theory. Am. Sociol. Rev. 86, 532–565 (2021).
Rohrer, J. M. & Murayama, K. These are not the effects you are looking for: causality and the within-/between-persons distinction in longitudinal data analysis. Adv. Methods Pract. Psychol. Sci. 6, 251524592211408 (2023).
Silberzahn, R. et al. Many analysts, one data set: making transparent how variations in analytic choices affect results. Adv. Methods Pract. Psychol. Sci. 1, 337–356 (2018).
Auspurg, K. & Brüderl, J. Has the credibility of the social sciences been credibly destroyed? Reanalyzing the ‘many analysts, one data set’ project. Socius 7, 237802312110244 (2021).
Shadish, W. R, Cook, T. D & Campbell, D. T. Experimental and Quasi-Experimental Designs for Generalized Causal Inference (Houghton, Mifflin, 2002).
Rhemtulla, M., van Bork, R. & Borsboom, D. Worse than measurement error: consequences of inappropriate latent variable measurement models. Psychol. Methods 25, 30–45 (2020).
Westfall, J. & Yarkoni, T. Statistically controlling for confounding constructs is harder than you think. PLoS ONE 11, e0152719 (2016).
Grosz, M. P., Rohrer, J. M. & Thoemmes, F. The taboo against explicit causal inference in nonexperimental psychology. Perspect. Psychol. Sci. 15, 1243–1255 (2020).
Deming, D. Early childhood intervention and life-cycle skill development: evidence from Head Start. Am. Econ. J. Appl. Econ. 1, 111–134 (2009).
Pion, G. M. & Lipsey, M. W. Impact of the Tennessee Voluntary Prekindergarten Program on children’s literacy, language, and mathematics skills: results from a regression-discontinuity design. AERA Open 7, 233285842110413 (2021).
Ritchie, S. J. & Tucker-Drob, E. M. How much does education improve intelligence? A meta-analysis. Psychol. Sci. 29, 1358–1369 (2018).
Steiner, P. M., Wong, V. C. & Anglin, K. A causal replication framework for designing and assessing replication efforts. Z. Psychol. 227, 280–292 (2019).
Munafò, M. R. & Davey Smith, G. Robust research needs many lines of evidence. Nature 553, 399–401 (2018).
Colnet, B. et al. Causal inference methods for combining randomized trials and observational studies: a review. Stat. Sci. 39, 165–191 (2024).
Wan, S., Brick, T. R., Alvarez-Vargas, D. & Bailey, D. H. Triangulating on developmental models with a combination of experimental and nonexperimental estimates. Dev. Psychol. 59, 216–228 (2022).
Gische, C., West, S. G. & Voelkle, M. C. Forecasting causal effects of interventions versus predicting future outcomes. Struct. Equ. Modeling 28, 475–492 (2021).
Imai, K., Kim, I. S. & Wang, E. H. Matching methods for causal inference with time‐series cross‐sectional data. Am. J. Polit. Sci. 67, 587–605 (2021).
Zyphur, M. J. et al. From data to causes II: comparing approaches to panel data analysis. Organ. Res. Methods 23, 688–716 (2020).
Lüdtke, O. & Robitzsch, A. A comparison of different approaches for estimating cross-lagged effects from a causal inference perspective. Struct. Equ. Modeling 29, 888–907 (2022).
Usami, S., Murayama, K. & Hamaker, E. L. A unified framework of longitudinal models to examine reciprocal relations. Psychol. Methods 24, 637–657 (2019).
Bond, T. N. & Lang, K. The evolution of the black–white test score gap in grades K–3: the fragility of results. Rev. Econ. Stat. 95, 1468–1479 (2013).
Larzelere, R. E., Cox, R. B. & Smith, G. L. Do nonphysical punishments reduce antisocial behavior more than spanking? A comparison using the strongest previous causal evidence against spanking. BMC Pediatr. 10, 10 (2010).
Oster, E. Unobservable selection and coefficient stability: theory and evidence. J. Bus. Econ. Stat. 37, 187–204 (2019).
Athey, S., Chetty, R., Imbens, G. W. & Kang, H. The Surrogate Index: Combining Short-Term Proxies to Estimate Long-Term Treatment Effects More Rapidly and Precisely Working Paper No. 26463 (NBER, 2019).
Weidmann, B. & Miratrix, L. Lurking inferential monsters? Quantifying selection bias in evaluations of school programs. J. Policy Anal. Manage. 40, 964–986 (2021).
Dehejia, R. H. & Wahba, S. Causal effects in nonexperimental studies: reevaluating the evaluation of training programs. J. Am. Stat. Assoc. 94, 1053–1062 (1999).
LaLonde, R. J. Evaluating the econometric evaluations of training programs with experimental data. Am. Econ. Rev. 76, 604–620 (1986).
Protzko, J. Effects of cognitive training on the structure of intelligence. Psychon. Bull. Rev. 24, 1022–1031 (2017).
Schmidt, F. L. Beyond questionable research methods: the role of omitted relevant research in the credibility of research. Arch. Sci. Psychol. 5, 32–41 (2017).
Meehl, P. E. Why summaries of research on psychological theories are often uninterpretable. Psychol. Rep. 66, 195–244 (1990).
Chaku, N., Kelly, D. P. & Beltz, A. M. Individualized learning potential in stressful times: how to leverage intensive longitudinal data to inform online learning. Comput. Hum. Behav. 121, 106772 (2021).
Moeller, J. et al. Generalizability crisis meets heterogeneity revolution: determining under which boundary conditions findings replicate and generalize. Preprint at PsyArXiv https://doi.org/10.31234/osf.io/5wsna (2022).
Dunning, T. et al. (eds). Information, Accountability, And Cumulative Learning: Lessons From Metaketa I (Cambridge Univ. Press, 2019).
Low, H. & Meghir, C. The use of structural models in econometrics. J. Econ. Perspect. 31, 33–58 (2017).
Todd, P. E. & Wolpin, K. I. Assessing the impact of a school subsidy program in Mexico: using a social experiment to validate a dynamic behavioral model of child schooling and fertility. Am. Econ. Rev. 96, 1384–1417 (2006).
Pearl, J., Glymour, M. & Jewell, N. P. Causal Inference in Statistics: A Primer (John Wiley & Sons, 2016).
Achen, C. H. Let’s put garbage-can regressions and garbage-can probits where they belong. Confl. Manage. Peace Sci. 22, 327–339 (2005).
Athey, S. & Imbens, G. Recursive partitioning for heterogeneous causal effects. Proc. Natl Acad. Sci. USA 113, 7353–7360 (2016).
Geng, E. H., Holmes, C. B., Moshabela, M., Sikazwe, I. & Petersen, M. L. Personalized public health: an implementation research agenda for the HIV response and beyond. PLoS Med. 16, e1003020 (2019).
Moeller, J. Averting the next credibility crisis in psychological science: within-person methods for personalized diagnostics and intervention. J. Pers. Oriented Res. 7, 53–77 (2021).
Pearl, J. & Bareinboim, E. Transportability of causal and statistical relations: a formal approach. Proc. AAAI Conf. Artif. Intell. 25, 247–254 (2011).
Wager, S. & Athey, S. Estimation and inference of heterogeneous treatment effects using random forests. J. Am. Stat. Assoc. 113, 1228–1242 (2018).
Benjamin-Chung, J. et al. Spillover effects in epidemiology: parameters, study designs and methodological considerations. Int. J. Epidemiol. 47, 332–347 (2018).
Hudgens, M. G. & Halloran, M. E. Toward causal inference with interference. J. Am. Stat. Assoc. 103, 832–842 (2008).
Imai, K., Jiang, Z. & Malani, A. Causal inference with interference and noncompliance in two-stage randomized experiments. J. Am. Stat. Assoc. 116, 632–644 (2021).
Tchetgen, E. J. T. & VanderWeele, T. J. On causal inference in the presence of interference. Stat. Methods Med. Res. 21, 55–75 (2012).
Zhang, C., Mohan, K. & Pearl, J. Causal inference with non-IID data using linear graphical models. Adv. Neural Inf. Process. Syst. 35, 13214–13225 (2022).
Eberhardt, F. & Scheines, R. Interventions and causal inference. Phil. Sci. 74, 981–995 (2007).
Mooij, J. M., Magliacane, S. & Claassen, T. Joint causal inference from multiple contexts. J. Mach. Learn. Res. 21, 3919–4026 (2020).
Peters, J., Bühlmann, P. & Meinshausen, N. Causal inference by using invariant prediction: identification and confidence intervals. J. R. Stat. Soc. B 78, 947–1012 (2016).
Aalen, O., Røysland, K., Gran, J., Kouyos, R. & Lange, T. Can we believe the DAGs? A comment on the relationship between causal DAGs and mechanisms. Stat. Methods Med. Res. 25, 2294–2314 (2016).
Driver, C. C. & Voelkle, M. C. in Continuous Time Modeling in the Behavioral and Related Sciences (eds Van Montfort, K. et al.) 79–109 (Springer International, 2018).
Røysland, K. A martingale approach to continuous-time marginal structural models. Bernoulli 17, 895–915 (2011).
Ryan, O. & Hamaker, E. L. Time to intervene: a continuous-time approach to network analysis and centrality. Psychometrika 87, 214–252 (2022).
Acknowledgements
This Review resulted from a cross-disciplinary workshop discussing such approaches (https://www.longitudinaldataanalysis.com/). The workshop and collaboration were funded by the Jacobs Foundation and CIFAR. The funders had no role in the decision to publish or in the preparation of this manuscript.
Author information
Authors and Affiliations
Corresponding author
Ethics declarations
Competing interests
The authors declare no competing interests.
Peer review
Peer review information
Nature Human Behaviour thanks Jörn-Steffen Pischke and Rebecca Johnson for their contribution to the peer review of this work.
Additional information
Publisher’s note Springer Nature remains neutral with regard to jurisdictional claims in published maps and institutional affiliations.
Rights and permissions
Springer Nature or its licensor (e.g. a society or other partner) holds exclusive rights to this article under a publishing agreement with the author(s) or other rightsholder(s); author self-archiving of the accepted manuscript version of this article is solely governed by the terms of such publishing agreement and applicable law.
About this article
Cite this article
Bailey, D.H., Jung, A.J., Beltz, A.M. et al. Causal inference on human behaviour. Nat Hum Behav 8, 1448–1459 (2024). https://doi.org/10.1038/s41562-024-01939-z
Received:
Accepted:
Published:
Issue Date:
DOI: https://doi.org/10.1038/s41562-024-01939-z
- Springer Nature Limited