Abstract
We prove a uniform large deviation principle for the law of the solutions to a class of semilinear stochastic partial differential equations driven by a Brownian sheet, where the uniformity is with respect to initial conditions that have bounded Euclidean norms on [0, 1]. Our proof is based on the weak convergence method.
Similar content being viewed by others
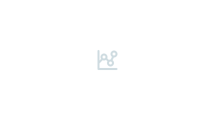
Avoid common mistakes on your manuscript.
1 Introduction
Large deviation analysis for infinite-dimensional dynamical systems such as stochastic partial differential equations (SPDEs) by employing the weak convergence approach has been an active area of research for nearly a decade now. While large deviations for SPDEs by exploiting the classical approach was already a subject of research (see e.g., [5, 19]) prior to that, the weak convergence approach provided a new perspective on the topic and enabled researchers to study large deviations for certain SPDEs, which were not easily feasible otherwise.
The starting point for the weak convergence approach is the equivalence between the large deviation principle (LDP) and the Laplace principle (LP) for Polish space valued random elements [10]. In this approach the expectations appearing in the LP are represented by value functions of minimal cost functionals of certain stochastic control problems. The proof of the LDP is then reduced to the asymptotic behavior of these variational representations and the subsequent identification of their limits. The key element in proving the LDP for certain infinite-dimensional dynamical systems including the one that we study is a variational representation for positive functionals of a Hilbert space valued Wiener process. This representation was formulated by Budhiraja and Dupuis [3] and was then generalized by Budhiraja, Dupuis and Maroulas [4] to provide sufficient conditions under which, the law of the solutions to certain SPDEs driven by a Brownian sheet satisfies an LDP. The verification of these sufficient conditions amounts to proving well-posedness, tightness and convergence of sequence of controlled analogues of the original SPDE and is much more tractable than the previous approaches.
Standard approaches to LDP for infinite-dimensional models are mainly due to Azencott [1] and involve several discretization and approximation arguments. These approximations require justifications that could be made through exponential continuity in probability and exponential tightness estimates, and are often highly technical and not available under optimal conditions. Another approach, which was developed by Feng and Kurtz [11, 12] relies on nonlinear semigroup theory and Hamilton-Jacobi-Bellman equations. The difficulty in this method is mainly due to a uniqueness requirement for infinite-dimensional nonlinear partial differential equations. This requirement is often technical and appears to differ significantly when the underlying model changes. In comparison, the weak convergence method bypasses the technical exponential estimates and uniqueness requirements present in the previous approaches and instead requires certain sufficient conditions, whose verification does not majorly deviate from the analysis of the underlying equations.
Recently, Salins [16] provided a sufficient condition under which, a uniform LDP is said to hold with uniformity being over initial conditions that belong to bounded but not not necessarily compact sets. He introduced the definition of the equicontinuous uniform Laplace principle (EULP), and showed that the EULP is equivalent to the Freidlin and Wentzell definition of the uniform large deviations principle. He also provided sufficient conditions, under which a measurable function of an infinite-dimensional Wiener process satisfies an EULP. His work was motivated by the study of exit time of a stochastic process from a domain, where the large deviations of the stochastic process must be uniform over initial conditions that belong to bounded but not necessarily compact sets.
In this paper, we consider a family of nonlinear, parabolic and semilinear SPDEs indexed by \(0<\varepsilon \le 1\)
for all \(t\in [0,T], ~~x\in [0,1]\), with Dirichlet boundary conditions
\({\displaystyle u^{\varepsilon }_\eta (t,0) = u^{\varepsilon }_\eta (t,1)=0 , ~~~t \in (0,T] , ~~~x \in [0,1],}\)
and initial condition
\({\displaystyle u^{\varepsilon }_\eta (0,x) = \eta (x) \in L^2([0,1]).}\)
Here W(t, x) denotes the Brownian sheet [4] carried by \((\Omega , {\mathcal F}, \{{\mathcal F}_t\}, {\mathbb {P}}),\) \(t\in [0,T]\) where \(\varepsilon \in (0,1]\) denotes the noise intensity. The functions \(f:=f(t,x,r)\), \(g:=g(t,x,r)\), and \(\sigma :=\sigma (t,x,r)\) are Borel functions of \((t,x,r)\in \mathbb R_{+}\times [0,1]\times \mathbb R\) and satisfy suitable regularity conditions. The existence and uniqueness of a strong solution to Eq. (1) was proven by Gyöngy [15], where the results of Bertini et al. [2], Da Prato et al. [7], and Da Prato and Gatarek [8] were generalized. We note that Salins and Setayeshgar [17] recently studied Eq. (1) perturbed by a finite-dimensional Brownian motion in any space dimension.
The main contribution of this paper is proof of uniform large deviations for Eq. (1) where the uniformity is with respect to initial conditions that are bounded and do not necessarily belong to a compact set. This is different from, and also generalizes the work of Foondun and Setayeshgar [13], where the initial conditions necessarily belong to a compact set. Our work uses the sufficient condition introduced in [16] as opposed to that of Budhiraja et al. [4], which cannot be used in this setting, and is motivated by its potential to study exit time problems. The paper also generalizes the results of Cerrai and Röckner [6] by adding the Burgers-like term, \(\displaystyle {\partial }g(t,x,u^\varepsilon _\eta (t,x))/{\partial x}\), and is an extension of the work of Cardon-Weber [5], as well.
The organization of the paper is as follows. In Sect. 2 we state the preliminaries and assumptions used throughout the paper. The existence and uniqueness of a strong solution to Eq. (1) is also stated in this section. In Sect. 3 we state the definition for the uniform large deviation principle (ULDP) as well as a sufficient condition under which the ULDP holds. We also introduce the controlled and skeleton equations and prove their existence and uniqueness. Section 4 is devoted to deriving an a priori bound on the controlled process, which is used in proving the main theorem. In Sect. 5 we state the main theorem of the paper. Finally Sect. 6 presents the proof of the main theorem.
1.1 Notations
Unless otherwise noted, we adopt the following notation throughout the paper. C denotes a free constant which may take on different values, and may depend upon other parameters. We use \(|q(t,\cdot )|_p :=|q(t)|_p\) to denote the \(L^p({\mathbb R})\)-norm of a function \(q := q(t,x)\) with respect to the variable \(x\in {\mathbb R}\). If q(t, x) is only defined for \(x\in [0,1]\), then \(|q(t)|_p\) denotes the \(L^p([0,1])\)-norm. If \(q := q(t,x)\) is a random field and E is a function space, then saying that q is almost surely in E means that q has a stochastic modification which is in E, almost surely. For any metric space, \((E, \rho )\), the distance between an element \(x \in E\) and a set \(B \subset E\) is defined by
Finally the form \(,\langle \cdot , \cdot \rangle ,\) denotes the \(L^2\)-inner product on an inner product space X. It is linear in the first variable and satisfies the Schwarz inequality \(\displaystyle |\langle x, y\rangle | \le \langle x, x\rangle ^{1/2} \langle y, y\rangle ^{1/2}\) for all \(x, y \in X\).
2 Preliminaries and assumptions
In this Section we introduce some assumptions and preliminaries necessary for the formulation of the problem. The functions \(f:=f(t,x,r)\), \(g:=g(t,x,r)\), and \(\sigma :=\sigma (t,x,r)\) are Borel functions of \((t,x,r)\in \mathbb R_{+}\times [0,1]\times \mathbb R\) and have the following assumptions
-
(B1)
There exists a constant \(K>0\) such that for all \((t,x,r)\in [0,T]\times [0,1]\times \mathbb R\) we have
$$\begin{aligned} \sup _{t\in [0,T]}\sup _{x\in [0,1]}|f(t,x,r)| < K(1+|r|). \end{aligned}$$ -
(B2)
The function g is of the form \(g(t,x,r):=g_1(t,x,r)+g_2(t,r),\) where \(g_1\) and \(g_2\) are Borel functions satisfying
$$\begin{aligned} |g_1(t,x,r)|< K(1+|r|)\quad \text {and}\quad |g_2(t,r)| < K(1+|r|^2). \end{aligned}$$ -
(B3)
\(\sigma \) is bounded and for every \(T > 0\) there exists a constant \(L > 0\) such that for \((t,x,p,q)\in [0,T]\times [0,1]\times {\mathbb R}^2\) we have \(|\sigma (t,x,p) -\sigma (t,x,q)| < L|p-q|.\) Furthermore, f and g are locally Lipschitz with linearly growing Lipschitz constant, i.e.
$$\begin{aligned} |f(t,x,p) -f(t,x,q)|&< L(1 +|p|+|q|)|p-q|,\\ |g(t,x,p)-g(t,x,q)|&< L(1 +|p|+|q|)|p-q|. \end{aligned}$$
Definition 1
(Mild solution)labeldefn1 A random field \(u^{\varepsilon }_\eta := \{u^{\varepsilon }_\eta (t,x) : t\in [0,T], x\in [0,1]\}\) is called a mild solution of Eq. (1) with initial condition \(\eta \) if \((t,x)\rightarrow u^{\varepsilon }_{\eta }(t,x)\) is continuous a.s., and \(u^{\varepsilon }_\eta (t,x)\) is \(\{{\mathcal F}_t\}\)-measurable for any \(t\in [0,T]\), and \(x\in [0,1]\), and if
The function \(G_t(.,.)\) is the heat kernel associated with the linear operator \(\partial /\partial t - \partial ^2/\partial ^2x\) with Dirichlet boundary conditions. The following result of Gyöngy [15, Theorem 2.1] asserts the existence and uniqueness of a solution to Eq. (1).
Theorem 1
(Existence & uniqueness of solution mapping)
Let \((\Omega , {\mathcal F}, P, \{{\mathcal F}_t\})\), \(t\in [0,T]\) be a stochastic basis carrying a Brownian sheet and let \(\eta \in L^p([0,1])\), \(p\ge 2\). There exists a measurable map
such that \(u^{\varepsilon }_\eta := \mathscr {G}^{\varepsilon }(\eta , \sqrt{\varepsilon }W)\), (where \(\eta \) denotes the initial condition) is the unique, mild solution of Eq. (1).
3 Uniform large deviation principle
In this section, we provide the definition of Freidlin Wentzell unifrom large deviation principle (FWULDP) [14] and a sufficient condition under which, an EULP holds.
Let \(({\mathcal {E}}, \rho )\) be a Polish space and \(\mathcal {E}_0\) a set. For any \(\eta \in \mathcal {E}_0\), definition of rate function, \(I_\eta :\mathcal {E} \rightarrow [0,+\infty ]\), refers to (8), which is below. Let \(\Phi _\eta (s)\) denote the level set \(\Phi _\eta (s) := \{h \in \mathcal {E}: I_\eta (h)\le s\}.\) Further, let \(\mathscr {J}\) be a collection of subsets of \(\mathcal {E}_0\) and \(b(\varepsilon )\) a function converging to zero as \(\varepsilon \) approaches zero.
Definition 2
(FWULDP) A family of \({\mathcal E}\)-valued random variables \(\{Y^{\varepsilon }_{\eta }\}^{\varepsilon \in (0,1]}_{\eta \in {\mathcal E_0}}\) is said to satisfy an FWULDP with speed \(b(\varepsilon )\) and rate function \(I_\eta \), uniformly over \(\mathscr {J}\) if
-
1.
For any \(J \in \mathscr {J}\), \(s_0\ge 0\), and \(\delta >0\)
$$\begin{aligned} \liminf _{\varepsilon \rightarrow 0} \inf _{\eta \in J} \inf _{h \in \Phi _\eta (s_0)} \left( b(\varepsilon )\log {\mathbb {P}}(\rho (Y^\varepsilon _\eta ,h)<\delta ) + I_\eta (h) \right) \ge 0. \end{aligned}$$(4) -
2.
For any \(J \in \mathscr {J}\), \(s_0\ge 0\), and \(\delta >0\)
$$\begin{aligned} \limsup _{\varepsilon \rightarrow 0} \sup _{\eta \in J} \sup _{s \in [0,s_0]} \left( b(\varepsilon ) \log {\mathbb {P}}({\text{ dist }}_\mathcal {E}(Y^\varepsilon _\eta , \Phi _\eta (s))\ge \delta ) + s \right) \le 0. \end{aligned}$$(5)
An equivalent formulation to the ULDP is the EULP [16, Theorem 2.10]. Recall that a family \(Q \subset C_b ({\mathcal E})\) of functions is equibounded and equicontinuous if
Definition 3
(EULP) A family of \(\mathcal {E}\)-valued random variables \(\{Y^{\varepsilon }_\eta \}^{{\varepsilon }\in (0,1]} _{\eta \in \mathcal {E}_0}\) is said to satisfy an EULP with speed \(b({\varepsilon })\) and rate function \(I_\eta \), uniformly over \(\mathscr {J}\) if for any \(J \in \mathscr {J}\) and any equicontinuous and equibounded family \(Q \subset C_b(\mathcal {E})\)
Theorem 2
[16, Theorem 2.10] FWULDP and EULP are equivalent.
We now present a sufficient condition under which, a family of random variables satisfies the EULP and therefore also the FWULDP. To that end, for any \(N>0\) and \(T>0\) let \(\mathcal {P}_2^N\) be the collection of \(\mathcal {F}_t\)-adapted processes such that \({\displaystyle {\mathbb {P}}(|\varphi |_{L^2([0,T]\times [0,1])} \le N) = 1}.\)
Assumption 1
Assume that there exists a family of measurable maps \(\mathscr {H}^{{\varepsilon }}: {\mathcal E}_0 \times C([0,T] \times [0,1]; \mathbb {R}) \rightarrow \mathcal {E}\) indexed by \({\varepsilon }\in (0,1]\), \(\eta \in \mathcal {E}_0\) and \(\varphi \in {\mathcal P}_2^N\). Let W be a Brownian sheet and \(Y^{{\varepsilon }, \varphi }_\eta := \mathscr {H}^{\varepsilon } ({\sqrt{\varepsilon }}W + \int _0^\cdot \int _0^\cdot \varphi (s) ds)\). Let \(\mathscr {H}^{0}\) denote the limiting case of \(\mathscr {H}^{\varepsilon }\) as \(\varepsilon \rightarrow 0\). Further, Let \(\mathscr {J}\) be a collection of subsets of \(\mathcal {E}_0\) and assume that for any \(\delta >0\), \(J \in \mathscr {J}\) and \(N>0\)
Theorem 3
([16, Theorem 2.13]) If Assumption 1 holds then the family \(Y^{{\varepsilon }}_\eta := \mathscr {H}^{{\varepsilon }}(\eta , \sqrt{{\varepsilon }} W)\) satisfies an EULP uniformly over \(\mathscr {J}\), with speed \(b({\varepsilon })={\varepsilon }\), and rate function \(I_\eta : \mathcal {E} \rightarrow \mathbb {R}\)
where the infimum is taken over all \(\varphi \in L^2([0,T] \times [0,1])\) such that
We use the convention that \(\inf (\varnothing )=+\infty \).
4 A priori bound on the controlled process
Before deriving the a priori estimate, we need to introduce the controlled and skeleton equations. The unique mild solution to Eq. (1) is a strong solution in the probabilistic sense. Therefore, for any \({\varepsilon }\in (0,1]\) and initial condition \(\eta \in L^2([0,1])\), there exists a measurable map \({\mathscr {G}}^{\varepsilon }: L^2([0,1]) \times C([0,T] \times [0,1]; \mathbb R) \rightarrow C\big ([0,T] ; L^2([0,1])\big ),\) such that \(u^{\varepsilon }_\eta := {\mathscr {G}}^{{\varepsilon }}(\eta , \sqrt{{\varepsilon }}W)\). Recall that for any \(N>0\) and \(T>0\), \(\mathcal {P}_2^N\) is the collection of \(\mathcal {F}_t\)-adapted processes such that \({\displaystyle {\mathbb {P}}(|\varphi |_{L^2([0,T]\times [0,1])} \le N) = 1}.\) Further, let \(v^{\varepsilon , \varphi }_\eta := {\mathscr {G}}^{\varepsilon }\big (\eta , \sqrt{\varepsilon }W+\int _0^\cdot \int _0^{{\cdot }} \varphi (s)ds\big ).\) Then \(v^{{\varepsilon },\varphi }_\eta \) solves
The deterministic controlled equation in the absence of noise is \(v^{0,\varphi }_\eta \), and is referred to as the so-called skeleton equation. Finally, for \(h\in C\big ([0,T]; L^2([0,1])\big )\) we define the following action functional or rate function
where the infimum is taken over all \(\varphi \in L^2([0,T]\times [0,1])\) such that
The existence and uniqueness of controlled process (10) follows by Theorem 3.2 in [13] with the main ingredient of the proof being the Girsonov’s theorem [9, Theorem 10.14].
We now attend to the a priori bound. Recall the stochastic controlled process (10)
Let \(\displaystyle \tau ^{\varepsilon , \varphi }_\eta (t,x) := {\sqrt{\varepsilon }}\int _0^t\int _0^1 G_{t-s}(x,y) \sigma (s,y, v^{\varepsilon , \varphi }_{\eta } (s, y))W(dy, ds)\), and set
In order to prove that \(v^{\varepsilon . \varphi }_\eta \) is bounded in probability uniformly in \(\varepsilon \), \(\eta \) and \(\varphi \), it suffices to show that, \(\tau ^{\varepsilon , \varphi }_\eta \) and \(w^{\varepsilon , \varphi }_\eta \) are bounded in probability uniformly in \(\varepsilon \), \(\eta \) and \(\varphi \). We begin with \(\tau ^{\varepsilon , \varphi }_\eta \).
Notice that by boundedness of \(\sigma \), Corollary 4.3 of [15] and a slight modification of Lemma 4.7 of [18], one can deduce that
Define
then since (15) holds, by Chebyshev’s inequality , \(\displaystyle \Lambda ^{\varepsilon , \varphi }_\eta \) is bounded in probability uniformly in \(\varepsilon \), \(\varphi \) and \(\eta \). Next we prove the uniform boundedness in probability of \(w^{\varepsilon , \varphi }_\eta \).
Lemma 1
(boundedness in probability of \(w^{\varepsilon , \varphi }_\eta \))
The random field \(\displaystyle \sup _{t\in [0, T]} |w^{\varepsilon , \varphi }_\eta (t)|_2\) is bounded in probability, i.e., for any given \(T>0, N>0\), and \(R>0\)
Proof
Throughout this proof C denotes a free constant, which is independent of \(\varepsilon \) and \(\eta \) but may depend upon other parameters. Notice that \(w^{\varepsilon , \varphi }_\eta \) satisfies the following equation
with Dirichlet boundary condition
\(w^{\varepsilon , \varphi }_\eta (t,0) = w^{\varepsilon , \varphi }_\eta (t,1) = 0, ~~~t\in (0,T]\),
and initial condition
\(w^{\varepsilon , \varphi }_\eta (0,x) = \eta (x), ~~x\in [0,1].\)
By the energy inequality [15, (4.18)] we have
where
Notice that by Assumption (B1) and the same analogy as proof of Theorem 2.1 in [15]
where Hölder’s inequality has been used. Next consider B(t) and let \(B(t) := B_1(t) + B_2(t)\) where
By Assumption (B3)
where Hölder’s inequality and Young’s inequality have been used. Due to the boundary conditions and Assumption (B3), \(B_2(t)\) is equal to zero. Consequently
Finally consider C(t). By Assumption (B3)
where Hölder’s inequality and Young’s inequality have been used. Next consider D(t) and observe that by boundedness of \(\sigma \), Hölder’s inequality, and boundedness of controls in \(L^2([0,T]\times [0,1])\) we have
Combining (20), (22), (23) and (24), and using (16)
Consequently
for all \(t\in [0,T]\). Therefore, by Grönwall’s inequality we have
for all \(t\in [0,T]\), \(\varepsilon \in (0,1]\), \(\varphi \in {\mathcal P}_2^N\), and \(\eta \in L^2[0,1]\). Thus, since \(\Lambda ^{\varepsilon , \varphi }_\eta \) is bounded in probability, Chebyshev’s inequality would imply that \(\sup _{t\le T}|w^{\varepsilon , \varphi }_\eta (t)|_2\) is also uniformly bounded in probability.
Proposition 1
(A priori estimate)
The random field \(\displaystyle \sup _{t\in [0, T]} |v^{\varepsilon ,\varphi }_{\eta }(t)|_2\) is bounded in probability, i.e., for any given \(T>0, N>0\) and \(R>0\)
Proof
5 Main theorem
In this section we state the main theorem of this paper.
Theorem 4
(Main Theorem)
The processes \(u^\varepsilon _\eta := \{{u}^{\varepsilon }_\eta (t,x): t\in [0,T],~ x\in [0,1]\}\) where \({\varepsilon \in (0,1]}, ~{\eta \in L^2([0,1])}\) satisfy a ULDP on \(C\big ([0,T]; L^{2}([0,1])\big )\), where the uniformity is over \(L^2([0,1])\)-bounded sets of initial conditions, with rate function \(I_{\eta },\) given by (11) if
-
1.
For any \(R>0\), \(\delta >0\), and \(s_0\ge 0\)
$$\begin{aligned} \liminf _{{\varepsilon }\rightarrow 0} \inf _{|\eta |_2 \le R} \inf _{h\in \Phi _\eta (s_0)}\left( {\varepsilon }\log {\mathbb {P}}(|{u}^{\varepsilon }_\eta - h|_{C([0,T]; L^2([0,1]))}<\delta )+ I_\eta (h) \right) \ge 0. \end{aligned}$$ -
2.
For any \(R>0\), \(\delta >0\), and \(s_0\ge 0\)
$$\begin{aligned} \limsup _{\varepsilon \rightarrow 0} \sup _{|\eta |_2\le R} \sup _{s \in [0,s_0]} \left( \varepsilon \log {\mathbb {P}}( {\text{ dist }}_{C([0,T]; L^2([0,1]))}({u}^\varepsilon _\eta ,\Phi _\eta (s)) > \delta ) + s \right) \le 0. \end{aligned}$$In the above expressions
$$\begin{aligned} \Phi _\eta (s) := \{h \in C([0,T]; L^2([0,1)): I_\eta (h)\le s\}. \end{aligned}$$
6 Proof of Main Theorem
We prove Theorem 4 via Theorem 2 and Theorem 3. To that end, we must show that Assumption 1 holds in the \(C([0,T]; L^2([0,1]))\) topology. Proposition 2 verifies this assumption.
Proposition 2
(Uniform convergence in probability) For any \(\delta >0\), \(R>0\), \(N>0\), and \(T>0\)
Proof
Throughout this proof C denotes a free constant, which is independent of \(\varepsilon \) and \(\eta \) but may depend upon other parameters. For any \(\varphi \in {\mathcal P}_2^N\), \(|\eta |_2 \le R\), and \(\varepsilon \in (0,1]\) we have
First consider \(I_2^\varepsilon \). By corollary 3.4 of [15], \(I_2^\varepsilon \) satisfies the definition of the linear operator J(v)(t, x) [15, Eq. (3.1)]. Therefore, in Lemma 3.1 of [15] we can let \(\rho =2\), \(q=1\), and \({\displaystyle \kappa = \frac{1}{2}}\). We have
where Lipschitz continuity of g with linearly growing Lipschitz constant, Minkowski’s inequality and Hölder’s inequality have been used. For \(K>0\), \(\varepsilon \in (0,1]\) \(\varphi \in {\mathcal P}_2^N\), \(|\eta |_{2}\le R\), and \(T>0\) define
Then
where Hölder’s inequality has been used.
Next consider \(I_3^\varepsilon \). In Lemma 3.1 of [15], let \(\rho =2\), \(q=1\), and \({\displaystyle \kappa = {1}/{2}}\). We have
where Lipschitz continuity of f with linearly growing Lipschitz constant, Minkowski’s inequality and Hölder’s inequality have been used. Again by definition of \(\Gamma ^\varepsilon _K\), and Hölder’s inequality
Lastly
where Lipschitz continuity of \(\sigma \), Hölder’s inequality, boundedness of controls and estimate 4.7 in [15] have been used. Therefore
Finally by (30), Minkowski’s inequality, estimates (33), (35), (37) and the fact that \(t\le T\)
Let \(\alpha >8\). By Hölder’s inequality
Using Fubini’s theorem and Grönwall’s inequality we have
Let \(\beta >0\). Notice that by Proposition 1, there exists \(K>0\) large enough such that
By estimates (41), (42), Markov’s inequality, boundedness of \(\sigma \) and Corollary (4.3) of [15], for any \({\varepsilon }\in (0,1]\), \(\eta \in L^2([0,1])\) and \(\varphi \in {\mathcal P}_2^N\)
Finally, by arbitrariness of \(\beta \)
This completes the proof.
Theorem 4
By Proposition 2, Assumption 1 is satisfied. This completes the proof.
Data Availability
Not applicable.
References
Azencott, R.: Grandes Deviations et Applications. É’cole d’Éé de Probabiliités de Saint-Flour VIII. Lecture Notes in Mathematics, vol. 774, pp. 1–176. Springer, Berlin (1980)
Bertini, L., Cancrini, N., Jona-Lasinio, L.: The stochastic Burgers’ equation. Commun. Math. Phys. 165, 211–232 (1994)
Budhiraja, A., Dupuis, P.: A variational representation for positive functional of infinite dimensional Brownian motions. Probab. Math. Stat. 20, 39–61 (2000)
Budhiraja, A., Dupuis, P., Maroulas, V.: Large deviations for infinite dimensional stochastic dynamical systems. Ann. Probab. 36, 1390–1420 (2008)
Cardon-Weber, C.: Large Deviations for a Burgers-type SPDE. Stoch. Process. Appl. 84, 53–70 (1999)
Cerrai, S., Röckner, M.: Large deviations for invariant measures of stochastic reaction-diffusion systems with multiplicative noise and non-Lipschitz reaction term. Ann. Probab. 32, 1–40 (2004)
Da Prato, G., Debussche, A., Temam, R.: Stochastic Burgers’ equation. Nonlinear Differ. Equ. Appl. 1, 389–402 (1995)
Da Prato, G., Gatarek, D.: Stochastic Burgers’ equation with correlated noise. Stoch. Stoch. Rep. 52, 29–41 (1995)
Da Prato, G., Zabczyk, J.: Stochastic Equations in Infinite Dimensions. Cambridge Univ. Press, Cambridge, UK (1992)
Dupuis, P., Ellis, R.S.: A Weak Convergence Approach to the Theory of Large Deviations. Wiley, New York (1997)
Feng, J.: Large deviations for diffusion and Hamilton–Jacobi equation in Hilbert space. Ann. Probab. 34, 321–385 (2006)
Feng, J., Kurtz, T.: Large Deviations of Stochastic Processes. Amer. Math. Soc., Providence (2006)
Foondun, M., Setayeshgar, L.: Large deviations for a class of semilinear stochastic partial differential equations. Stat. Probab. Lett. 121, 143–151 (2017)
Freidlin, M.I., Wentzell, A.D.: Random Perturbations of Dynamical Systems. Springer, New York (1984)
Gyöngy, I.: Existence and uniqueness results for semi-linear stochastic partial differential equations. Stoch. Process. Appl. 73, 271–299 (1998)
Salins, M.: Equivalence and counter examples between several definitions of the uniform large deviations principle. Probab. Surv. 19, 12–15 (2019)
Salins, M, Setayeshgar, L.: Uniform large deviations for a class of Burgers-type stochastic partial differential equations in any space dimension. Potential Anal. (2021) (online first)
Setayeshgar, L.: Large deviations for a stochastic Burgers’ equation. Commun. Stoch. Anal. (COSA) 8, 141–154 (2014)
Sowers, R.: Large deviations for a reaction-diffusion equation with non-Gaussian perturbations. Ann. Probab. 20, 504–537 (1992)
Author information
Authors and Affiliations
Corresponding author
Ethics declarations
Conflict of interest
Not applicable.
Additional information
This article is part of the section “Applications of PDEs” edited by Hyeonbae Kang.
Rights and permissions
Springer Nature or its licensor (e.g. a society or other partner) holds exclusive rights to this article under a publishing agreement with the author(s) or other rightsholder(s); author self-archiving of the accepted manuscript version of this article is solely governed by the terms of such publishing agreement and applicable law.
About this article
Cite this article
Setayeshgar, L. Uniform large deviations for a class of semilinear stochastic partial differential equations driven by a Brownian sheet. Partial Differ. Equ. Appl. 4, 3 (2023). https://doi.org/10.1007/s42985-022-00220-0
Received:
Accepted:
Published:
DOI: https://doi.org/10.1007/s42985-022-00220-0
Keywords
- Large deviations
- Weak convergence method
- Stochastic Partial differential equations
- Infinite dimensional dynamical systems.