Abstract
The change in climate elements such as rainfall and temperature are determinant factors of hydrological components (e.g., streamflow, water yield, evapotranspiration). Thus, understanding the trends of hydro-climate variables are imperative for planning water resources management measures. This study examines the trends of precipitation and temperature (1980–2014) as well as streamflow (1990–2008) changes in the Jemma sub-basin of the Upper Blue Nile (Abbay) Basin. A homogeneity test was performed for pre-processing data to inspect the statistical nature of data homogeneity viz., Pettitt’s, SNHT, Buishand range test, and Von Neumann test (VNT). The Mann–Kendall (MK) test, Modified Mann–Kendall (MMK) test, Sen’s slope estimator, and Innovative trend analysis (ITA) were applied to compute the existence of trend and the magnitude of change at an annual timescale. In a nutshell, the study portrays a change in the trends of hydro-climate variables when using different statistical tests. The result showed that the mean annual temperature in many stations depicted upward trends. There is a significant increasing trend (p < 0.05) by 0.029 °C per year in the mean annual temperature of all climatic stations. Based on Sen’s slope estimator, the annual precipitation and streamflow were increased by 1.781 mm/year and 0.085 m3/s, respectively. But no significant trends were detected in precipitation and streamflow when using Sen’s slope estimator test. The results of this study are worthwhile for evaluating the trends of hydro-climatic variables in other areas of Ethiopia in particular and elsewhere in the world in general, which are fundamental for planning water resource management measures.
Article Highlights
-
The annual precipitation of the sub-basin has shown an increasing trend.
-
A considerable upward trend in the annual average temperature was found.
-
The observed rainfall and temperature trends are associated with the hydro-climate trends.
Similar content being viewed by others
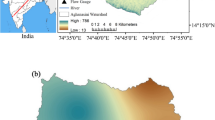
Avoid common mistakes on your manuscript.
1 Introduction
Globally, population growth, urbanization, and industrialization have increased the amount of greenhouse gas emissions [1,2,3,4,5]. The increase in the concentration of greenhouse gas in the atmosphere is among the best-documented global changes [5]. Because of the high emissions of greenhouse gases into the atmosphere, the climate system is altering noticeably and become a global problem. According to Sharma and Ravindranath [6] and Pachauri et al. [7], rising temperatures and sea levels, shrinking glaciers and snow, unpredictable precipitation, and climate extremes are among the commonly witnessed impacts of climate change across the world in recent decades. The earth’s surface was successively warmer in the last three decades (1980–2010) than in any other decades since 1850 [2]. The change in precipitation was non-conclusive which shows an increase in the Shale and Southern Africa, but a shrink in the East Africa region was observed during 1983-2010 periods [3].
The two key elements of climate (i.e., precipitation and temperature) are an integral part of the hydrological cycle and the changing pattern of these climate variables as a consequence of natural and anthropogenic forcing are a great concern of water resource managers, hydrologists and agriculturalists [7,8,9]. Monthly and seasonal changes of precipitation and temperature will trigger a change in the agricultural and water management activities [10]. The effect of precipitation and temperature changes on agriculture is relatively high for countries who are highly reliance on rain-fed agriculture like Ethiopia [11].
Hydro-climate variables are playing a key role in providing worthwhile information for sustainable water management [12,13,14,15,16,17,18] and decision-makers [5]. Results from the trends of precipitation, temperature, and streamflow are non-trivial for researchers and resource managers to identify the spatial and time inconsistency and insufficient water resources for sustainable economic enlargement. Essentially, the study of hydro-climatic trends is important to detect compound extremes which is the simultaneous or sequential incidence of hydro-climatic extremes in a particular area/region [11, 12]. Compound extremes have tremendous impacts on the hydro-ecosystem, agriculture, and society of the region [13]. The occurrence of compound extremes could also exacerbate the repercussion of climate change and other environmental changes. Subsequently, the detailed characterization of trends and spatial distributions of hydro-climatic variables and compound extremes is non-trivial [10]. Checking the data reliability/homogeneity is crucial for any climate and hydrologic data before doing further analysis. The interpretation of homogenous and checked data leads to making appropriate conclusions [11].
Several studies have been undertaken concerning the trends of temperature and precipitation in different parts of Ethiopia [14,15,16,17,18,19,20]. An increase in temperature and an inconsistent trend of rainfall was observed in different regions of Ethiopia [14,15,16]. For instance, a decline in main season rainfall and annual rainfall was investigated in the eastern, southern, and southwestern parts of Ethiopia, and in the Baro-Akobo, Omo-Ghibe, Rift Valley, and Blue Nile River (Abbay) basin in the last four decades [15, 17]. These studies have used different linear, parametric, and non-parametric statistical approaches to detect the trends of rainfall and temperature. By using linear trend analysis, a statistically non-significant growing trend of precipitation was detected in the Upper Blue Nile (Abbay) Basin [19]. On the other hand, Worku et al. [20] has assessed the observed changes in the extremes of daily rainfall and temperature trends in the Jemma sub-basin of the Upper Blue Nile (Abbay) Basin using Mann Kendall and Sen’s slope estimator. However, applying the classical Mann–Kendall (MK) trend test has restrictions for detecting significant trends [20]. The Modified Mann–Kendall (MMK) test is the nonparametric trend detection test with free distribution in contradiction outliers [22].
In general, although trend analysis of hydro-climate variables is important for planning water resources management measures, there are scanty of studies on trends of hydro-climate variables in Ethiopia in general and in the Jemma sub-basin of the Upper Blue Nile (Abbay) Basin (study sub-basin) in particular. In Ethiopia, most of the existing studies are focused on the trend analysis of precipitation and temperature alone [16]. In addition, majority of previous studies were used the classical MK trend test [18,19,20]. Furthermore, most of the preceding studies in Ethiopia did not evaluate the homogeneity of the data before applying them for trend analysis [19]. Therefore, this study is aimed to identify the trends of hydro-climate variables by means of the MK test, modified (MMK) test, Sen’s slope estimator, and Innovative trend analysis (ITA). Additionally, in the current investigation, the whole autocorrelation structure was taken into account to depict its impact in trend analysis.
2 Material and methods
2.1 Description of the study area
The Jemma sub-basin is part of the Upper Blue Nile Basin with an approximate area of 15,000 km2. The sub-basin lies between 10.57 N—9.12 N and 38.7 E -39.48 E. The annual precipitation of the sub-basin has an even distribution pattern that ranges from 700–1400 mm. The two rainfall seasons in the region are summer (June–August) and spring (March–May). The northern part of the Jemma sub-basin is characterized by low precipitation. The sub-basin experienced an average temperature ranging from 9 to 24 °C. Extreme temperature and rainfall events, as well as periodic droughts, are characteristics of the Jemma sub-basin [20]. The majority of the population relies on rain-fed agriculture for their living, which is constantly impacted by climate change [37]. The sub-basin comprises different agroecology’s which range from cold moist sub-afro alpine in the eastern part of the sub-basin to warm sub-moist lowlands in the central and western part of the sub-basin [23]. The study area has a mix of cropland (56.72%), grazing land (14.64%), bare land (10.45%), shrubland (6.44%), woodland (6.05%), and forest areas (1.20%) as its primary land uses/covers while Eucalyptus plantations, afro-alpine vegetation, and water bodies cover the remaining parts [37].
2.2 Data sources
This study used climate (1980–2014) and hydrological (1990–2008) data from seven representative meteorological stations and four streamflow gauges, respectively that are located within the study area. The rainfall and temperature data were obtained from the National Meteorology Agency (NMA) of Ethiopia. The climatic stations are distributed in different parts and represent different agro-ecologies of the sub-basin (Fig. 1). The streamflow data were acquired from the Ministry of Water and Energy (MoWI). The streamflow data was collected from the Beressa, Chacha, Robit and Alelitu hydrological gauges (Fig. 1; Table 1).
2.3 Homogeneity test
Homogeneity assessment for hydro-climate data is crucial before test detection on account of the observed long-term data series affected by external factors. This test was adopted for pre-processing data to explore the statistical nature of data homogeneity [24,25,26]. The four homogeneity tests viz., Pettitt’s, SNHT, Buishad range test, and Von Neumann test (VNT) were performed to assess the data series homogeneity at different stations. Using many homogeneity tests was important to minimize unreliability issues in the data series.
2.4 The Pettitt-Whitney–Mann test
The Pettitt test was applied in trend detection to decipher the most considerable change point. The Pettitt test considers a series with N samples as a two sub-sample represented by × 1 … xt, and xt + 1 …xN, and a test statistics version of the Mann–Whitney.
The null hypothesis H0: No change or τ = T tested against the alternative hypothesis Ha: change or 1 ≤ τ < T using the non-parametric statistic KT = Max|Ut, T|, 1 ≤ τ < T.
The change point is located at KT
where, n is the number of observations and Xi and Xj are the Jth and Kth data; sgn denotes the significance function.
The significance level related with KT is calculated as:
where, P refers the probability of the existence of a change point. KT is the location of change point.. A p-value of less than 0.05 (95%) was defined as a significant increasing and descending change in the time series considering at 5% significance level.
2.5 Standard normal homogeneity test (SNHT)
Many studies used the Standard Normal Homogeneity Test (SNHT) frequently to detect inhomogeneities in climatological and hydrological time series. The test statistic for the SNHT test is calculated as:
Where, \({\mathrm{T}(}_{\mathrm{K}})\) is used to compare the mean of the first k years with mean of the last n-k years, n is the last year and k is the first year.
The critical value is
Where, \(\upsigma\) is the variance the data; the year k is the change point if the value of the Tk is maximum. If the test statistic is greater than the critical value, the null hypothesis is rejected.
2.6 Buishand’s test
Buishand’ test is one the most popular and commonly used non-parametric test to become aware of homogeneity time series. According to [14], Buishand’ test is more sensitive to breaks in the middle of time series. The test statistic for Buishand’ test is computed as:
where, Sk is test statistic for Buishand’ test; n is the last year and k is the first year.
2.7 The von neumann test (VNT)
The Von Neumann test (VNT) examines the randomness and change point detection of the time series. The Von Neumann test (VNT) test statistic can be computed as:
where N is the test static value of VNT, x is observed time-series data, \(\overline{x }\) refers to the mean of observed time-series data.
Homogenous time series data can be found if the expected value of N is 2. The value of N is less than 2 can show a break pattern.
2.8 Trend analysis
The trends of annual hydro-climatological series from hydrometric stations located in the Jemma sub-basin were assessed using Mann–Kendall (MK) test, Modified Mann–Kendall (MMK) test, Sen’s slope estimator, and Innovative trend analysis (ITA). The reasons behind using different statistical tests were to reduce the possible error from a single method as well as to advance the confidence in the existence of detecting trends in hydro climatological time series. Sen’s slope estimator was adopted to estimate the degree of trends in hydro-climatological time series. The significance level of α = 0.05 and 0.01 was used to analyse changes in statistical tests. R programming/software (R Development Core Team) and different packages in-built in to the software were used for the data analysis [27].
2.9 Mann–Kendall test
The Mann–Kendall trend test is used to detect the statistical significance of increasing or decreasing trends in long-term hydro-climate data. The MK trend test is based on two hypotheses; one is the null hypothesis (Ho), and the other is the alternative hypothesis (H1). The null hypothesis articulates the presence of no trend while the H1 explains a significant increasing or decreasing trend in precipitation data [28]. The Mann–Kendall test trends are popularly used and not significantly influenced by the outliers occurring in the data series [29,30,31].
In the current study, the Modified Mann–Kendall trend test essentially developed from the Mann–Kendall trend test [22, 31] was used to detect the change in precipitation, average temperature, and streamflow.
The Mann–Kendall trend test statistics S is computed as:
where S is the Mann–Kendall test statistic, n is the number of data points, Xi, and Xj are the data values in time series I and j (j > i)
where m is the number of the attached group; n is the number of data points; and tk represents the number of extent k.
where, Zs is standardized test statistics; Var is for variance.
The non-parametric Mann–Kendall trend test is widely adopted to detect monotonic trends in a series of environmental data and hydro-climate data. Nevertheless, the output of the test may have an error if an autocorrelation exists in the data series. Pre-whitening was carried out to eliminate the autocorrelation in the data time series.
where, R represents the first autocorrelation coefficient (i.e. lag-1) of the time series xt, xt represents the average of the data, n is the number of the data points in the data time series
When the lag-1autocorrelation coefficient is found to be within the interval defined by the second equation, it can be concluded that the time series does not reveal significant autocorrelation and the Mann–Kendall trend test can be applied to the original Xt data series. On the contrary, if the calculated lag-1 autocorrelation coefficient is found to be outside of the interval, it can be said that the data series how significant autocorrelation at a 5% significant level and is removed from the series using Eq. 14.
where Y1 indicates a series without an auto-regressive part. At last, the linear trend is added to the new series based on Eq. 15 and the Mann –Kendall test can be applied to the original Xt data series.
where \({Y}_{2}\) is a new data series without auto-regressive and linear trend in the original data series.
2.10 Sen’s slope estimator
Sen’s slope estimator, the non-parametric procedure has been developed by Şen [33] to estimate the sloping trend in the sample of N pair of data. It can be computed as:
where, Xj, and Xk are the data value at tilt j and k (j > k), respectively.
The median is calculated from the N observations of the slope Qi. The N values of Qi area ranked are from lowest to highest, and then, Sen’s slope estimator was computed:
where, Q is Sen’s estimator of slope; Q is the median of Sen’s estimator of the slope; N number of observations. A positive Q-value represents an increasing trend, while a negative Q-value represents decreasing trend over time.
2.11 Innovative trend analysis (ITA)
The Innovative Trend Analysis is developed by Sen [27] that has been employed by different researchers [26,27,28,29] to detect the trend in hydro-climate variables. The original time series data were divided into two equal subseries and then arranged each subseries in increasing order. The two identical time series are plotted against each other while the points are distributed in a straight line (1:1) and ± 5 and 10% error lines on the plane coordinate system. If the time series data fall below the straight line (1:1), there is a downward trend. If the series data points exist in the top triangle, then it is the implication of a positive trend. If the data points lay on a 1:1 line, there is no trend in the data. Furthermore, the trend can be analysed as low, medium, and high values since the 45° (1:1) line can be separated into three categories. The trend indicator (D) is computed as:
where D is the trend indicator, n is the number of observations in each subseries, \(\overline{x }\) is the average of the first half subseries data, xi and yi are the values of the first and the second subseries at ith scatter point. 10 is the scaling coefficient [33].
3 Results
3.1 Homogeneity test results
The annual meteorological and streamflow data were tested and checked for their homogeneity using Pettitt’s, SNHT, Buishand range test, and VNT. The results revealed that there was homogeneity in the data at many stations annually. The alpha value of 95% (0.05) was applied to detect homogeneity in annual precipitation, temperature, and streamflow data series. Accordingly, the projected test statistics for annual precipitation, temperature, and streamflow data series which are greater than the alpha values were taken into consideration as homogeneous, while P values (the bold) one lower than the 0.05 significance level were considered to be inhomogeneous (Table 2).
From the point of working conditions of the homogeneity test, one should have to take into consideration if the null hypothesis is accepted by all homogeneity tests at a 0.05 significance level [34]. The decision criterion for meteorological and hydro-gauging stations provides a full confirmation of the homogeneity of the data throughout all four testing models in the study area. The evaluation of the annual precipitation homogeneity test results is also presented in Table 2. Based on their level of significance, results were identified in the status of homogeneity and non-homogeneity. Homogenous data series (labeled as bold) was observed in the Fiche station based on the Pittett test and SNHT. Precipitation homogenous data series were detected in all and three of four homogeneity tests at Lemi, Debrebirhan, Gohatsion, Wereilu, Alemketema, and Mehal Meda stations. Likewise, the temperature data were subjected to homogeneity tests in major stations such as Fiche, Debrebirhan, Alemketema, and Lemi have shown homogeneous data series. Homogeneity tests were performed for streamflow data. The streamflow data were indicated useful in two gauges and one is suspect among three stations.
3.2 Annual trend assessment
The final results of annual trend detection and estimation for the streamflow and climate variables in the Jemma sub-basin are summarized in Table 2. In the present study, the correction factors developed by Hamed and Ramachandra Rao [35], and Yue, and Wang [28] (denoted by MK.CF1, and MK.CF2 respectively), Sen.’s slope estimator (SSE), Trend Free Pre-whitened Time Series (TFPW), Mann–Kendall Test of Pre-whitened (ZPW), Spearman's Rank Correlation Test (r) were performed for annual trend analysis of temperature and precipitation and streamflow. Trend analysis for precipitation and mean temperature were carried out for the period between 1986 and 2014. As shown in Table 2, the MK.CF2 results for temperature were Alemketema (-1.094), Debrebirhan (2.461), Lemi (0.168), Fiche (2.776), Gohatsion (0.928), Mehal Meda (− 0.219), and Wereilu (1.319). There were significantly increasing trends of temperature at Wereilu and Lemi stations at the rate of 0.078 °C cm and 0.09 °C per year, respectively.
Wereilu station has shown the highest increase of 17.46 mm/year of precipitation over the last 29 years. Based on Sen’s slope estimator (SSE), the annual precipitation time data series showed an increasing trend except for the Alemketema station (decreased by 5.15 mm/year). The MK-CF1 and MK-CF2 test also show a notable increasing trend of precipitation in the Debrebirhan, Lemi, Fiche, Gohatsion, and Wereilu climatic stations (Table 3). Based on the findings, one can determine that the annual precipitation shows positive (Sen’s slope magnitude for Lemi = 2.33, Debrebirhan = 4.06, Fichie = 7.483, Gohatsion = 3.18, Wereilu = 17.46 and negative trends (Sen’s slope magnitude for Alemketema = − 5.15 mm/year, Mehal Meda = − 1 mm/year) in different stations.
The output of the trend examination for the discharge of all stations by using MK.CF1 and MK. CF2, SSE, TFPW, Mann–Kendall Test of Pre-whitened, and Spearman's Rank Correlation test (r) are presented in Table 3. Increasing trends were also detected in the data time series of streamflow at three stations for the year 1990–2008. There were raising trends at Chacha, Alelitu, and Robe by the rate of 0.082, 0.014, and 0.294 m3/s/year respectively. Based on the SSE result, the annual discharge of Beressa is significantly decreasing at the rate of 0.124 m3/s/year. It corroborates streamflow is decreasing in the cool sub-moist highlands (Beressa) than in other gauges of the sub-basin located in the temperate moist and sub-moist mid-highlands (Alelitu and Robe) (Fig. 2).
As shown in Fig. 3, the temperature exhibited increasing trends, except Alemketema, Fiche and Debrebirhan stations. There are significantly increasing trends at Wereilu and Lemi hydrological stations. However, temperature unfolds spatially non-consistent trends among the climatic stations of the study sub-basin. The findings imply a slightly warming tendency in the study area.
The annual mean temperature in the sub-basin illustrated a growing trend (the value of ZPW = 1.442, MK = 3.247, ZTFPW = 3.417, MK.CF1 = 1.873 MK.CF2 = 2.344 R = 0.583 SSE = 0.0289). From the result of Sen’s slope estimator, the trend has a significance increment by the value of 0.178 °C. As shown in the statistics Table 4, although the stations’ trend shows variation, the annual precipitation depicted an increasing trend but not significant (ZPW = 1.007, MK = 0.506, ZTFPW = 0.928, MK.CF1 = 0.506, MK.CF2 = 0.117, R = 0.117 SSE = 1.781). The annual discharge of the study watershed depicted an increasing (not significant) trend with the value of ZPW = 1.287, MK = 1.435, ZTFPW = 1.742, MK.CF1 = 1.239, MK.CF2 = 1.439, and R = 0.282 SSE = 0.085).
The ITA method is applied to the annual precipitation, temperature, and streamflow records leading to graphs in Fig. 2–4. It is noticeable that there are visual inconsistencies among graphs in the ITA method. According to the ITA, there are increasing trends of temperature in Gohatsion, Lemi, Mehal Meda, and Wereilu stations. However, there are decreasing trends at Fiche, Debrebirhan, and Alemketema stations. Furthermore, there exist monotonic trends of temperature at three stations i.e., Lemi, Mehal Meda, and Wereilu. On contrary, there are no –monotonic trends at the rest stations (Fig. 3).
From ITA, all the stations in the Jemma watershed display nonmonotonic trends in annual precipitation. As indicated in Fig. 4, one can be noticed that there are decreasing precipitation trends at Lemi, Mehal Meda, Fiche, and Alemketema. The trend is significant at Lemi, Mehal Meda. At Wereilu, Gohatsion, and Debrebirhan stations, increasing trends for precipitation can be observed. No significant trend is observed except in the Wereilu station.
As streamflow ITA trend analysis at an annual scale shows that the streamflow has increasing trends at two stations i.e. Alelitu, and Robi. As shown in Fig. 2. There is a significance trend at Chacha and Beressa stations.
4 Discussion
The homogeneity test is an important pre-processing task to remove erroneous and assess the data reliability in climate and hydrological trend analysis. Based on the three employed homogeneity tests, the obtained results show the null hypothesis a is independent and identically distributed [14]. On account of this, homogenous is assumed in all three test methods. The data series were considered useful homogeneity when they satisfied the null hypothesis of three out of the four homogeneity tests performed in the study (the calculated p-value for each test was greater than the 0.05 significance level) [14, 36].
Numerous research utilizing nonparametric and parametric methods have been carried out recently on a global scale on spatial and temporal trend assessments of hydroclimatic data [14, 19, 20, 24, 35]. The results of scientific work generally agree with those of this study's ITA, MMK, MK test, and Sen's slope results. Nevertheless, in their examination, they did not do homogeneity and stream flow trend analysis with in-depth assessments of annual-based trends, necessitating additional thorough research.
This study examined homogeneity trend analysis at hydro gauging stations, rainfall stations, and temperature stations over the Jemma subbasin. In the Jemma sub-basin, both positive and negative trends of hydro-climatic variables were revealed by using MK.CF1 and MK.CF2, SSE, TFPW, Mann–Kendall Test of Pre-whitened, Spearman's Rank Correlation test (r), and ITA. Based on the aforementioned, the annual average temperature shows temporal variation between the years 1986–2014. The watershed reveals an overall increasing tendency of precipitation [5, 10, and 18]. The results revealed that findings are highly consistent with 2 previous study in Ethiopia [37].
The annual mean temperature has increasing trends in MK, MMK, ITA and Sen’s slope estimator. The findings of the present study are in general agreement with the results of trend analysis [19]. The MK.CF1 and MK.CF2, SSE, TFPW, Mann–Kendall Test of Pre-whitened, Spearman's Rank Correlation test (r), and ITA show both negative and positive trends in different stations. But the annual precipitation of the study sub basin portrays increasing (not significant). Worku et al. [37] and Mohammed et al. [10] also, corroborate this conclusion who modelled the hydrological process and indicating that precipitation under baseline climate scenario proved a rising trend but not significant. Though the above-mentioned studies supported the finding of temperature and rainfall trends, they didn’t correlate with stream flow. For that matter, this study emphasizes assessing hydro-climate variables using the ITA.
Spearman's rank correlation coefficient test (r) was employed to study the relationship between variables at each station with time. The results were summarized and presented in Table 3. As indicated in the result, the correlation test of five precipitation stations i.e., Lemi, Debrebirhan, Gohatsion, Wereilu, and Fiche have shown a positive correlation coefficient while Alemketema and Mehal Meda with a negative correlation coefficient. Except for Fiche station (54%), other stations are not significant. The correlation coefficient test of temperature in the stations indicated that two stations (Mehal Meda and Gohatsion) have lower R results. Unlike the other two variables (rainfall and streamflow), the temperature in many stations depicted higher correlation coefficient values. In the time series trend, the streamflow of Beressa gauge has shown negative correlation coefficient values (− 0.501). All in all, the average annual precipitation and streamflow data series have shown increasing but not significant. Whereas, the average annual temperature has revealed a significant positive trend. Though the result is not significant it proved there was a positive relationship between streamflow and rainfall. The study's findings, which are in agreement with those of [10, 14, 15, 19, and 20], demonstrated that there is a general tendency toward increasing temperature, and a trend toward decreasing rainfall across the stations. Besides, the monthly, seasonal, and yearly rainfall Z statistics values that were positive and negative, respectively, suggested rising and falling monthly rainfall patterns [5].
Understanding the trends of hydro-climate variables would have critical implications for planning, management and sustainable use of water resources. As a consequence, this study provides rudimentary information about the changes in precipitation, temperature, and streamflow at an annual scale using the available observed data. The findings of the study in turn can help in making decisions concerning climate change threat diminution and management.
5 Conclusion
The present study examined the trends of hydro-climate elements in the Jemma sub-basin using Mann Kendall (MK), Modified Mann Kendall (MK), Innovative trend analysis (ITA), and Sen’s slope estimator. Before further analysis, the study presented the application of different statistical tests i.e., Pettitt’s, SNHT, Buishand range test and VNT to examine homogeneity and trends in precipitation, temperature (1980–2014 year), and stream flow (1990-2008). The homogeneity tests showed that most of the stations were homogenous. The innovative trend analysis (ITA) method provides detailed information on trends of hydro-climate variables. The general results obtained from the ITA technique are greatly consistent with those found by the MK trend test and its modification.
The annual precipitation of the sub-basin has shown an increasing trend but is not statistically significant. Likewise, the average annual temperature has revealed increasing trends in the majority of stations. The annual streamflow of the stations depicted increasing trends except for Beressa station. As stated in the results and discussion, in terms of stations it can be determined that there are increasing and decreasing trends of precipitation, temperature, and streamflow. The implication of the results shows the variation of trends in hydro- climatological variables. Hence, the output from the present study is fundamental for water resource management experts, land use planners, and policymakers. The limitation of this study is that it does not assess the spatial variations of hydro-climate variables through interpolation of the station data, which is attributed to the unavailability of many numbers of stations in the sub-basin. In spite of this limitation, the study will provide important insight for evaluating the trends of hydro-climate variables using a compressive trend analysis test (MK, MMK, Sen’s slope, and ITA). Of these trend tests, the application of ITA for assessing the trends of hydro-climate variables is a relatively a new method, and hence the method can be applied in other areas in Ethiopia and elsewhere in the world.
References
Change, I. C. (2014). Impacts, adaptation, and vulnerability. Part A: global and sectoral aspects. Contribution of working group II to the fifth assessment report of the intergovernmental Panel on Climate Change, 1132.
Stocker TF, Qin D, Plattner GK, Tignor MM, Allen SK, Boschung J, Midgley PM (2014) Climate Change 2013: The physical science basis contribution of working group I to the fifth assessment report of IPCC the intergovernmental panel on climate change. Cambridge University Press, Cambridge
Maidment RI, Allan RP, Black E (2015) Recent observed and simulated changes in precipitation over Africa. Geophys Res Lett 42(19):8155–8164
Field CB (ed) (2015). Cambridge University Press, Cambridge
Orke YA, Li MH (2021) Hydroclimatic variability in the Bilate watershed Ethiopia. Climate 9(6):98. https://doi.org/10.3390/cli9060098
Sharma J, Ravindranath NH (2019) Applying IPCC framework for hazard-specific vulnerability assessment under climate change. Environ Res Commun 1(5):051004. https://doi.org/10.1088/2515-7620/ab24ed
Pachauri, R.K., et al. (2014) AR5 Synthesis Report: Climate Change 2014. Contribution of Working Groups I, II and III to the Fifth Assessment Report of the Intergovernmental Panel on Climate Change. Intergovernmental Panel on Climate Change, Geneva.
Wu S, Bates B, Zbigniew Kundzewicz AW, Palutikof J (2008) Climate change and water. Technical Paper of the Intergovernmental Panel on Climate Change, Geneva
Praveen B, Talukdar S, Shahfahad MS, Mondal J, Sharma P, Islam ARMT, Rahman A (2020) Analyzing trend and forecasting of rainfall changes in India using non-parametrical and machine learning approaches. Sci Rep 10(1):10342
Mohammed JA, Gashaw T, Tefera GW, Dile YT, Worqlul AW, Addisu S (2022) Changes in observed rainfall and temperature extremes in the Upper Blue Nile Basin of Ethiopia. Weather Clim Extrem 37:100468
World Bank, (2006) “Managing water resources to maximize sustainable growth: a country water resources assistance strategy for Ethiopia. World Bank, Washington, DC,” no. 36000. 1–119.
Sedlmeier K, Mieruch S, Schädler G, Kottmeier C (2016) Compound extremes in a changing climate–a Markov chain approach. Nonlinear Process Geophys 23(6):375–390. https://doi.org/10.5194/npg-23-375-2016
Hao Z, Singh V, Hao F, Hao Z, Singh VP, Hao F (2018) Compound extremes in hydro climatology: a review. Water 10:718. https://doi.org/10.3390/w10060718
Daba MH, Ayele GT, You S (2020) Long-term homogeneity and trends of hydroclimatic variables in Upper Awash River Basin Ethiopia. Adv Meteorol 12:5. https://doi.org/10.1155/2020/8861959
Bewket W, Conway D (2007) A note on the temporal and spatial variability of rainfall in the drought-prone Amhara region of Ethiopia. Int J Climatol: A J Royal Meteorol Soc 27(11):1467–1477. https://doi.org/10.1002/joc.1481
Cheung WH, Senay GB, Singh A (2008) Trends and spatial distribution of annual and seasonal rainfall in Ethiopia. Int J Climatol: A J Royal Meteorol Soc 28(13):1723–1734. https://doi.org/10.1002/joc.1623
Change, E. P. O. C. (2015). First Assessment Report Working Group Ii-Climate Change Impact, Vulnerability, Adaptation and Mitigation V.
Malede DA, Agumassie TA, Kosgei JR, Linh NTT, Andualem TG (2022) Analysis of rainfall and streamflow trend and variability over Birr River watershed, Abbay basin Ethiopia. Environ Chall 7:100528
Mengistu D, Bewket W, Lal R (2014) Recent spatiotemporal temperature and rainfall variability and trends over the Upper Blue Nile River Basin Ethiopia. Int J Climatol 34(7):2278–2292. https://doi.org/10.1002/joc.3837
Worku G, Teferi E, Bantider A, Dile YT (2019) Observed changes in extremes of daily rainfall and temperature in Jemma Sub-Basin, Upper Blue Nile Basin Ethiopia. Theor Appl Climatol 135(3):839–854. https://doi.org/10.1007/s00704-018-2412-x
Dinpashoh Y (2019) Trend analysis of groundwater level, using mann-kendall non parametric method (Case Study: Tabriz Plain). JWSS-Isfahan Univ Technol 23(2):335–348. https://doi.org/10.29252/jstnar.23.2.335
Brema J (2018) Rainfall trend analysis by Mann–Kendall test for Vamanapuram river basin, Kerala. Int J Civil Eng Technol 9(13):1549–1556
MoA, 1998 Agro-Ecological Zones of Ethiopia. Natural Resources Management & Regulatory Department. Addis Ababa. 1998.
Taxak AK, Murumkar AR, Arya DS (2014) Long term spatial and temporal rainfall trends and homogeneity analysis in Wainganga basin, Central India. Weather Clim Extrem 4:50–61. https://doi.org/10.1016/j.wace.2014.04.005
Patakamuri SK, Muthiah K, Sridhar V (2020) Long-term homogeneity, trend, and change-point analysis of rainfall in the arid district of Ananthapuramu, Andhra Pradesh State India. Water 12(1):211. https://doi.org/10.3390/w12010211
Javari M (2016) Trend and homogeneity analysis of precipitation in Iran. Climate 4(3):44. https://doi.org/10.3390/cli4030044
R Core Team, 2015 R. core Team, and R. D. C. Team, “R: A language and environment for statistical computing.,” R A Lang. Environ. Stat. Comput
Yue S, Wang C (2004) The Mann-Kendall test modified by effective sample size to detect trend in serially correlated hydrological series. Water Resour Manage 18(3):201–218. https://doi.org/10.1023/B:WARM.0000043140.61082.60
Thapa S, Li B, Fu D, Shi X, Tang B, Qi H, Wang K (2020) Trend analysis of climatic variables and their relation to snow cover and water availability in the Central Himalayas: a case study of Langtang Basin. Nepal Theor Appl Climatol 140(3):891–903. https://doi.org/10.1007/s00704-020-03096-5
Şen Z (2014) Trend identification simulation and application. J Hydrol Eng 19(3):635–642
Şen Z (2012) Innovative trend analysis methodology. J Hydrol Eng. https://doi.org/10.1061/(asce)he.1943-5584.0000556
Almazroui M, Şen Z (2020) Trend analyses methodologies in hydro-meteorological records. Earth Systems and Environment 4(4):713–738. https://doi.org/10.1007/s41748-020-00190-6
Şen Z (2012) Innovative trend analysis methodology. J Hydrol Eng 17(9):1042–1046
Wu H, Qian H (2017) Innovative trend analysis of annual and seasonal rainfall and extreme values in Shaanxi, China, since the 1950s. Int J Climatol 37(5):2582–2592. https://doi.org/10.1002/joc.4866
Hamed KH, Rao AR (1998) A modified Mann-Kendall trend test for autocorrelated data. J Hydrol 204(1–4):182–196. https://doi.org/10.1016/S0022-1694(97)00125-X
Funk C, Rowland J, Eilerts G, Kebebe E, Biru N, White L, Galu G (2012) A climate trend analysis of Ethiopia. US Geol Survey, Fact Sheet 3053(5):6
Worku, G., Teferi, E., Bantider, A., & Dile, Y. T. (2021). Modelling hydrological processes under climate change scenarios in the Jemma sub-basin of upper Blue Nile Basin, Ethiopia. Climate Risk Management, 31, 100272. https://doi.org/10.1016/j.crm.2021.100272
Wijngaard JB, Klein Tank AMG, Können GP (2003) Homogeneity of 20th century European daily temperature and precipitation series. Int J Climatol 23(6):679–692
Acknowledgements
The National Meteorology Agency (NMA) and Ministry of Water and Energy (MoWE), respectively, provided the study with the climate and hydrological data. These organizations were acknowledged with providing the data by the authors. The journal's editor and the two anonymous reviewers' constructive comments is also greatly appreciated by the authors.
Author information
Authors and Affiliations
Corresponding author
Additional information
Publisher's Note
Springer Nature remains neutral with regard to jurisdictional claims in published maps and institutional affiliations.
Rights and permissions
Open Access This article is licensed under a Creative Commons Attribution 4.0 International License, which permits use, sharing, adaptation, distribution and reproduction in any medium or format, as long as you give appropriate credit to the original author(s) and the source, provide a link to the Creative Commons licence, and indicate if changes were made. The images or other third party material in this article are included in the article's Creative Commons licence, unless indicated otherwise in a credit line to the material. If material is not included in the article's Creative Commons licence and your intended use is not permitted by statutory regulation or exceeds the permitted use, you will need to obtain permission directly from the copyright holder. To view a copy of this licence, visit http://creativecommons.org/licenses/by/4.0/.
About this article
Cite this article
Lebeza, T., Gashaw, T., Tefera, G.W. et al. Trend analysis of hydro-climate variables in the Jemma sub-basin of Upper Blue Nile (Abbay) Basin, Ethiopia. SN Appl. Sci. 5, 129 (2023). https://doi.org/10.1007/s42452-023-05345-4
Received:
Accepted:
Published:
DOI: https://doi.org/10.1007/s42452-023-05345-4