Abstract
Aims
To evaluate the relationship between anthropometric indices, including abdominal volume index (AVI), waist-to-hip ratio (WHR), waist-to-height ratio (WHtR), conicity index (C index), body mass index (BMI), body roundness index (BRI), body adiposity index (BAI), A body shape index (ABSI) and cardiovascular risk factors, and their abilities to predict metabolic syndrome (MetS) in adults.
Methods
A cross-sectional study of 76,915 participants (30,912 females and 46,003 males) aged between 14 and 100 years was conducted. AVI, WHR, WHtR, BMI, conicity index (C index), BRI, BAI, and ABSI were measured and calculated. Pearson correlation analysis and linear regression analysis were used to examine the relationship between anthropometric indicators and the components of MetS, while binary logistic regression analysis was used to assess the relationship between anthropometric indicators and overall MetS. The receiver operating characteristic curve (ROC) was used to analyze and calculate the area under the curve (AUC). Then, a 95% confidence interval (95% CI) was calculated to evaluate the ability of anthropometric indicators to predict MetS and determine the optimal cutoff value of each anthropometric indicator. The optimal cutoff value was determined by the Youden index.
Results
MetS prevalence was 21.71% in males and 9.5% in females. Participants with MetS were older and had higher values of glucose, triglyceride, low-density lipoprotein cholesterol (LDL-C), total cholesterol (TC), systolic blood pressure (SBP), diastolic blood pressure (DBP) than those without MetS. The high-density lipoprotein cholesterol (HDL-C) values of males and females with MetS were lower than the values found in those without MetS. Mean values of all anthropometric indicators in men and women with MetS were significantly higher than the mean values in those without MetS. After adjusting for age, alcohol consumption, and smoking, anthropometric indexes AVI, WHR, WHtR, C index, BMI, and BRI were all associated with cardiovascular risk factors (p < 0.001). Among men over the age 60 years old, an AVI cutoff of 16.0 predicted MetS with a sensitivity of 74.70% and a specificity of 84.90%. The area under the ROC curve was 0.84 (p < 0.001). Among women over the age of 60 years, an AVI cutoff of 12.8 predicted MetS with a sensitivity of 90.13% and a specificity of 63.72%. The area under the ROC curve was 0.80 (p < 0.001). Among men aged 30–60 years, an AVI cutoff of 16 predicted MetS with a sensitivity of 80.44% and a specificity of 82.36%. The area under the ROC curve was 0.85 (p < 0.001). Among women aged 30–60 years, an AVI cutoff of 12.82 predicted MetS with a sensitivity of 87.72% and a specificity of 83.47%. The area under the ROC curve was 0.90 (p < 0.001). Among men under the age of 30 years, an AVI cutoff of 16.22 predicted MetS with a sensitivity of 87.97% and a specificity of 88.65%. The area under the ROC curve was 0.92 (p < 0.001). Among women under the age of 30 years, an AVI cutoff of 12.79 predicted MetS with a sensitivity of 95.92% and a specificity of 93.79%. The area under the ROC curve was 0.97 (p < 0.001). AVI showed the strongest ability to distinguish MetS across genders and different age groups.
Conclusion
AVI, WHR, WHtR, BMI, C index, and BRI were all associated with cardiovascular risk factors. The anthropometric index is a useful screening tool for MS, its components, and cardiovascular risk factors. Among all the indices examined, AVI can best distinguish MetS.
Similar content being viewed by others
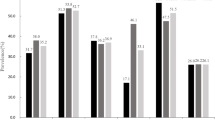
Explore related subjects
Discover the latest articles, news and stories from top researchers in related subjects.Avoid common mistakes on your manuscript.
Introduction
Cardiovascular disease (CVD) is the leading cause of death in the world, and its prevalence varies with territory and culture. The prevalence, morbidity, and mortality of CVD are on the rise in China, and CVD is the number one leading cause of death, with percentages as 44.60% and 42.51% of total deaths in rural and urban areas [1]. The mortality rate from CVD in rural areas has exceeded and continued to exceed the urban level since 2009. The increasing burden of CVD has become a major public health concern. In this sense, the prevention of CVD has become a public health priority, especially for those considered at high cardiovascular risk. Clinically, effective strategies must be developed to fully identify these patients.
Metabolic syndrome (MetS) describes a cluster of conditions that pose as risk factors for CVD and other illnesses such as type 2 diabetes and stroke. It has become a major health problem in both developing and developed countries, with prevalence rising globally. According to the National Cholesterol Education Program [2], MetS is made up of the presence of any three or more of the following conditions: (a) blood glucose greater than 5.6 mmol/L (100 mg/dL) or drug treatment for elevated blood glucose 2; (b) high-density lipoprotein (HDL) cholesterol < 1.0 mmol/L (40 mg/dL) in men, < 1.3 mmol/L (50 mg/dL) in women or drug treatment for low HDL-C; (c) blood triglycerides > 1.7 mmol/L (150 mg/dL) or drug treatment for elevated triglycerides; (d) waist circumference > 102 cm in men, or > 88 cm in women; (e) blood pressure > 130/85 mmHg or drug treatment for hypertension. Individuals meeting at least three of the five components of MetS are considered as having the condition. People with MetS have a higher risk of insulin resistance, low levels of chronic inflammation, and oxidative stress [3]. MetS can also contribute to the early development of coronary heart disease (CHD) [4]. Therefore, it is crucial to identify MetS early and determine which anthropometric indicators have the highest predictive ability for MetS.
The use of methods to determine body composition began in the 1940s and has been extended to various methods as indicators of health status, treatment progress, and functional status in medicine and research. The measurements and indices make it easy for researchers to use anthropometry as a method of assessing cardiovascular risk because it is simple to measure, low cost, and clinically applicable. Additionally, they provide invaluable information on risk factors that can help prevent and treat diseases. Perissinotto et al. [5] found that non-pathological factors, such as age, sex, and geographical area, may influence anthropometric measurement and should be considered. Anthropometric indices have been the focus of much research on obesity, cardiovascular health, and diseases. However, certain issues such as the possible redistribution of fat [6], the selection of the most appropriate statistical method, and the optimal measurement technique are all important factors to consider for meaningful measurement in any given population.
The assessment of obesity was extrapolated from anthropometric measurements. With the increasing prevalence of obesity, the development of a reliable anthropometric obesity index has become an important public health issue. Obesity not only closely reflects the risk of glucose metabolism disorders but identifying it also helps clinicians advise patients on health promotion and awareness. Body mass index (BMI), an anthropometric parameter, is the most commonly used index for assessing overweight and obesity. It has long been used to assess obesity because of its simplicity, ease of calculation, and clear association with mortality and obesity-related complications. However, BMI alone does not reflect the amount or the distribution of body fat that comes from the variation in any given population under study, including factors such as age, sex, ethnicity, and type of obesity. While overall obesity is measured by BMI, measurements of waist circumference (WC) and hip circumference (HC) and their associated ratios have been identified as better indicators of body size and obesity-related risks. A common form of abdominal obesity is the waist-to-hip ratio (WHR), a ratio calculated by dividing WC over HC, or WC, and overall obesity is measured by body mass index (BMI). WHR [7] is determined by dividing waist circumference by hip circumference. It is used to describe how body fat is distributed, and if it is concentrated centrally or in the limbs. Both overall obesity and poor body fat distribution are independently associated with the development of type 2 diabetes mellitus (DM). Central obesity as assessed by WHR or WC is a better indicator of type 2 diabetes risk than BMI is. Sometimes weight gain or loss causes similar changes in WC and HC without changing the final ratio. For the reason, WHR is not very useful in assessing changes in weight change. Also, WC does not account for variations in height. Therefore, it may underestimate the risks in short people, while overestimating the risks of tall people. While both WHR and WC are based on longitudinal measurements, the reliability of the estimation of central obesity by WC and WHR might also be affected in individuals whose umbilical line falls below the buttocks and the pendulum abdomen.
To address these issues, the abdominal volume index (AVI) [8] is developed as an anthropometric obesity assessment. As the name implies, it measures total volume. It is a theoretical estimate of total abdominal volume, including abdominal fat and adipose tissue volume as measured between the pubic symphysis and the xiphoid process, and is calculated by using WC and HC according to the formula [2 cm (WC) 2 + 0.7 cm (WC-HC) 2]/1000. The formula is derived from the formula for calculating the volume of a cylinder and the volume formula of a cone. It is easier to estimate volume with the AVI formula than to estimate the volume with a truncated cone. Women’s fat distribution is mainly concentrated in the lower body, while men are mainly distributed in the upper body. The geometric figures of the obesity pattern in women and men are upright and inverted cones, respectively. The obesity pattern based on which AVI is calculated is similar to calculating the volume of a truncated cone. The advantage of using AVI to estimate abdominal volume is that it predicts the possible occurrence of both obesity patterns. Overseas studies [9] have found it to be a reliable measurement, closely linked to impaired glucose tolerance (IGT) and type 2 diabetes (DM). However, studies on AVI and its ability to predict other diseases including cardiovascular risk factors are still rare.
Conicity index (C index) is an indicator of abdominal obesity proposed by Ruperto et al. [10], based on geometric reasoning models. It is built upon the fact that the less fat individuals have in the center, the closer they resemble a cylinder. However, if individuals accumulate fat around the waist, their body shape seems to shift from the cylinder to the tip cone. The C index has no units and ranges theoretically from 1.00 (perfect cylinder) to 1.73 (double cone, namely two cones, where the base is the same). It is obtained by using WC, height, and weight of any given individual according to the following formula: “WC (m) / (0.109 × \(\sqrt{\mathrm{weight }[\mathrm{kg}]/\mathrm{height }[\mathrm{m}]}\)).” Compared with WHR, the C index adjusts for the height as well as the weight of the individual, and can directly compare abdominal fat across individuals and even across different populations without requiring hip measurement [11].
There is growing evidence suggesting that abdominal fat plays a role in the development of MetS. Developing new anthropometric indicators to evaluate abdominal fat is thus necessary. To address this issue, several new measurements have been developed including the body adiposity index (BAI) by Bergman and colleagues [11], the A body shape index (ABSI) by Krakauer and Krakauer [12], and the body roundness index (BRI) by Thomas and colleagues [13]. These indices aim to estimate body fat distribution. BMI is known to have limited accuracy, as its value may differ for men and women with similar percentages of body fat. To make up for this deficiency, Bergman et al. proposed that BAI as an indicator for evaluating body fat composition. It is calculated based on height and HC and shows a high correlation with adult body fat percentage (BF%) [11]. BAI can be used to reflect the percentage of body fat of adult men and women of different races without numerical correction. ABSI [14] is an index that standardizes WC by BMI and height, while ABSI assesses visceral, abdominal, and general obesity at the same time, and is a better predictor of premature death than WC and BMI [15]. BRI is based on a new geometric model that quantifies a person’s body shape independently of height. It can predict body fat percentage and visceral adipose tissue and can be used as a visual tool for health assessment.
Finally, waist-to-height ratio (WHtR) is also an indicator of abdominal obesity and has been used to assess metabolic disorders in studies. WHtR shows that individuals’ WC should not exceed half their height, and show better sensitivity in assessing health risks than measurements of isolated WC in different populations.
There is no complete agreement on the best anthropometric indicators for assessing MetS status and risk. The best measure of obesity as a predictor of cardiometabolic risk remains elusive. Due to differences in race, genetic makeup, diet and lifestyle, culture, and economic conditions between foreign and domestic populations, more research is needed in the Chinese population. Additionally, the diversity of anthropometric methods, particularly the newly proposed AVI and other anthropometric indicators, and their ability to predict MetS remain controversial and have not been widely analyzed or studied. The purpose of this study was to assess the relationship between AVI and cardiovascular risk factors and to compare the ability of AVI and other anthropometric indicators in predicting MetS in adults.
Methods
Data collection
A cross-sectional study of 76,915 participants (30,912 females and 46,003 males) aged between 14 and 100 years was conducted between December 2013 and December 2014 in the metropolis of Chengdu city in west China, the details of which had been documented elsewhere [16]. Inclusion criteria were that the participant was a resident of metropolitan Chengdu and willing to participate in the study. Exclusion criteria were the following: unwilling participants, patients with severe heart, liver and kidney diseases, malignant tumors, mental disorders, persons unable to take care of themselves, persons diagnosed with eating difficulties, pregnant women, and persons with physical disabilities. Demographic information including sex, age, medical history (hypertension or diabetes mellitus), family history, smoking status (current smoking defined as ≥ 20 cigarettes/month for ≥ 6 months), drinking status (current alcohol use defined as at least once/week for ≥ 6 months), and other relevant information were collected.
Anthropometric assessment
Body weight, height, blood pressure, WC, and HC were measured and recorded by skilled nurses. All participants were requested to stand upright with arms hanging freely in light clothing without shoes. All measurements were taken using inelastic tape on the skin. WC was measured straight at the midpoint between the last rib and the iliac crest to the nearest 0.1 cm at the end of a normal breath [16]. The average of three measurements was recorded. HC was measured at the widest portion of the buttock. Height was measured by a nonelastic ruler to the nearest 0.5 cm. Weight was measured to the nearest 0.1 kg in light clothing and without shoes using a Weight scale (Wujin, RGT-120, Wujin Medical Equipment Co. Ltd., Jiangsu, China). Blood pressure was measured seated, by a sphygmomanometer (Yuyue, GB3053-93, Yuyue Medical Equipment Co., Ltd., Jiangsu, China) on the right arm after a 5-min rest. The mean of two measurements with at least a 1-min interval was taken. Anthropogenic indices were calculated by the following formulas:
Laboratory measurements
Blood samples were drawn from the antecubital vein after fasting for more than 12 h. Without being placed in the freezer, the blood samples were centrifuged at 3000 rpm for 10 min and then tested. Fasting plasma glucose (FPG), total cholesterol(TC), high-density lipoproteins cholesterol (HDL-C), low-density lipoproteins cholesterol (LDL-C), triglycerides (TG), Cystatin C (Cys-c), alanine aminotransferase (ALT), aspartate aminotransferase (AST), γ-glutamyltransferase (γ-GGT), total bilirubin (TB), direct bilirubin (DB), serum uric acid (UA), serum creatinine (Scr), and blood urea nitrogen (BUN) were analyzed by a biochemical autoanalyzer (ROCHE Cobas 8000, ROCHE Diagnostics, Basel, Switzerland) at the Department of Clinical Laboratory Diagnostics, West China Hospital Sichuan University [16]. TB and DB were assayed by the Vanadate oxidase method. FPG concentrations were assayed by the hexokinase method. TGs in plasma were analyzed by glycerol oxidation. TC was assayed by means of the enzymic method. HDL-C and LDL-C were analyzed by homogeneous enzyme colorimetry. ALT, AST, and γ-GGT were measured through the enzyme rate method. UA and BUN were measured by means of the enzyme coupling method. Cystatin C (Cys-c) was assayed by emulsion enhanced immune projection turbidimetry. Scr was measured by means of the picric acid method.
Diagnostic criteria for MetS and its components
MetS was diagnosed when the participant shows the presence of three or more following factors according to the National Cholesterol Education Program-Adult Treatment Panel III(NCEP-ATPIII)criteria [2]: abdominal obesity: WC ≥ 90 cm in men or WC ≥ 80 cm in women; Increased TG ≥ 150 mg/dL (1.7 mmol/L); Decreased HDL-C, defined as HDL-C < 40 mg/dL (1.03 mmol/L) in male or < 50 mg/dL (1.29 mmol/L) in female; blood pressure ≥ 130/85 or receiving treatment; fast blood glucose (FBG) ≥ 100 mg/dL (5.6 mmol/L), or type 2 diabetes mellitus or receiving treatment.
Statistical analysis
The normality of the distribution was evaluated using the Kolmogorov–Smirnov test. Results were expressed as a number (percentages) or mean ± standard deviations. IBM SPSS v24.0 (SPSS Inc., Chicago, USA) was used for statistical analysis. Student’s t-test was used to assess mean differences between males and females. Comparisons of means were assessed by analysis of variance (ANOVA), followed by Tukey’s test. Pearson correlation analysis and linear regression analysis were used to examine the relationship between anthropometric indicators and the components of MetS, while binary logistic regression analysis was used to assess the relationship between anthropometric indicators and MetS. The receiver operating characteristic curve (ROC) and the area under the Curve (AUC) of the ROC were designed to assess the abilities of the anthropometric indexes to predict MetS, and determine the cutoff points for each indicator. Cutoff points were got after calculating the Youden’s index (sensitivity + specificity-1). The AUC was compared using MedCalc by DeLong et al.’s [17] (version 13.0; MedCalc Software, Mariakerke, Belgium). Statistical significance was considered at p < 0.05.
Results
Basic information on cardiovascular risk factors and anthropometric indicators
Table 1 shows baseline characteristics of 76,915 participants with a mean age of 44.36 years ± 12.51 years (59.81% for males and 40.19% for females). Among these, 12,927 individuals (16.81%) are with MetS, while 63,988 subjects (83.19%) are without MetS. The prevalence of MetS was found to be 21.71% in males and 9.5% in females. Participants with MetS are older and have higher values of FBG, TG, LDL-C, TC, SBP, DBP, PP, heart rate, cystatin C, UA, Scr, BUN, ALT, AST, GGT, TB, and IB than those without MetS. Participants with MetS had lower HDL-C and DB values than those without MetS. Both women and men with MetS had significantly higher mean values for all anthropometric indicators than those without MetS. There was no statistical difference in Scr and IB values between men with and without MetS. There was no statistical difference in TB value between women with and without MetS. Both men and women who have MetS are significantly older than those who do not have MetS. Participants with MetS had higher mean values of anthropometric indices than those without MetS (all p < 0.05). Participants with MetS had higher TG, TC, LDL-C, FBG, SBP, DBP, PP, and LDL-C than those without MetS (all p < 0.05). Participants with MetS were more likely to have a history of hypertension and diabetes than those without MetS (p < 0.05). Interestingly, among female participants, those without MetS have higher smoking and drinking rates than their counterparts with MetS. Among male participants, those with MetS have higher drinking and smoking rates than their counterparts without MetS.
Prevalence of MetS overall and by number of its components across gender and age groups
Table 2 shows that the prevalence of MetS in the study sample is 16.81%, while the value is at 21.71% for men and 9.5% for women. Of the total 76,915 participants, 9527 participants (12.39%) are under 30 years old, 58,655 participants (76.26%) are between 30 and 60 years old, and 8733 participants (11.35%) are over 60 years old. MetS prevalence rate is 4.18% (n = 398) in those under 30 years old, 16.83% (n = 9870) in those aged 30–60 years old, and 30.45 (n = 2659) in those over 60 years old. MetS prevalence rate increases with age (p < 0.05). Among the MetS components, increasing WC accounts for 27.40% of the total number of participants (n = 21,076), hypertriglyceridemia 31.22% (n = 24,011), low HDL-C 15.63% (n = 12,019), hypertension 40.36% (n = 31,042), and hyperglycemia 21.47% (n = 16,668), while 33.38% of the study participants (n = 25,676) show no abnormal MetS components (which are increased WC, hypertriglyceridemia, decreased HDL-C, high blood pressure, elevated glycemia). As the number of MetS abnormal components increases, the percentage within the population falls. There are statistically significant differences in the prevalence of MetS across genders and age groups. The prevalence of MetS increases with the increase of age.
Distribution of anthropometric indices in different age groups
The values for all anthropometric indices increase with the increase in age within our study sample, for both the male and female population, with statistically significant differences across age groups (p < 0.001; Table 3). There were significant differences among different age groups (p < 0.001; Table 3).
Correlation between anthropometric indices and cardiovascular risk factors
Table 4 shows results from Pearson correlation analysis between anthropometric indicators and components of the MetS. We find that the values of AVI, WHR, WHtR, C index, BMI, BRI, BAI, and ABSI all correlate with cardiovascular risk factors (p < 0.05). All the correlations are statistically significant, but the correlation coefficients are small. Among anthropometric indices, AVI shows the greatest positive correlation with DBP, TG, and VLDL, and the greatest negative correlation with HDL-C. WHtR shows the greatest correlation with TC, SBP, and LDL-C, and WHR shows the greatest correlation with FBG.
Multiple linear regression analysis of MetS components and anthropometric indices
After adjusting for age, alcohol consumption, and smoking, anthropometric indices AVI, WHR, WHtR, C index, BMI, and BRI are all associated with cardiovascular risk factors (Table 5; p < 0.001), while ABSI and BAI are not. BAI values correlate with all cardiovascular risk factors with the exception of TC. ABSI values correlate with all the cardiovascular risk factors with the exception of SBP and TC. Anthropometric indices are negatively correlated with HDL-C. With the exception of BAI and ABSI, all the other indices are negatively correlated with TC.
ROC curve analysis of different anthropometric indices to predict MetS components
Tables 6 and 7 show results from the ROC analysis results of anthropometric indices predicting the presence of MetS components. These component indicators are abdominal obesity, hypertriglyceridemia, hyperglycemia, hypertension, and low HDL-cholesterol. AVI had an obvious differential effect on abdominal obesity, with an AUC value of 1.00 for both sexes. In addition, the values of WHR, WHtR, C index, BMI, and BRI are also able to identify abdominal obesity with AUC values above 0.90, while the AUC values for BAI and ABSI are above 0.80, but below 0.90. For hypertriglyceridemia, the highest AUC values correspond to BMI for men, WHtR, and BRI for women (0.688 for men, 0.747 for women). For hyperglycemia, the highest AUC values for males and females correspond to WHtR and BRI (0.650 for males, 0.712 for females). For hypertension, the highest AUC values for men and women correspond to BRI and WHtR (0.658 for men, 0.701 for women). For low HDL-C, the highest AUC for men and women correspond to BMI (0.677 for men, 0.696 for women).
Logistic analysis of MetS and anthropometric indices
Results from binary Logistic regression analysis (Table 8) show that anthropometric values in the study population independently correlate with MetS even after adjusting for age, gender, drinking, and smoking.
ROC curve analysis of anthropometric indicators for MetS in the general population
Table 9 shows that AVI among all the anthropometric indices has the largest AUC and thus the strongest ability to predict MetS.
ROC curve analysis of anthropometric indices to identify MetS aged ≥ 60 years
Results from ROC curve analysis in Table 10 and Fig. 1A, and Table 11 and Fig. 1B show that AVI has the largest AUC in those aged ≥ 60 years. Among men over the age of 60 years, an AVI cutoff of 16 predicted MetS with a sensitivity of 74.70% and a specificity of 84.90%. The area under the ROC curve is 0.84 (p < 0.001). Among women over the age of 60 years, an AVI cutoff of 12.8 predicted MetS with a sensitivity of 90.13% and a specificity of 63.72%. The area under the ROC curve is 0.80 (p < 0.001).
ROC curve analysis of anthropometric indices to identify MetS in participants aged 30–60 years
Results from ROC curve analysis in Table 12 and Fig. 1C, and Table 13 and Fig. 1D show that AVI has the largest area under the curve in participants aged 30–60 years. Among men in this age group, an AVI cutoff of 16.0 predicts MetS with a sensitivity of 80.44% and a specificity of 82.36%. The area under the ROC curve is 0.85 (p < 0.001). Among women aged 30–60 years, an AVI cutoff of 12.82 predicted MetS with a sensitivity of 87.72% and a specificity of 83.47%. The area under the ROC curve is 0.90 (p < 0.001).
ROC curve analysis of anthropometric indices to identify MetS in participants aged < 30 years old
Results from ROC curve analysis in Table 14 and Fig. 1E, and Table 15 and Fig. 1F show that AVI has the largest AUC in the participants aged < 30 years. Among men in this age group, an AVI cutoff of 16.22 predicts MetS with a sensitivity of 87.97% and a specificity of 88.65%. The area under the ROC curve is 0.92 (p < 0.001). Among women aged < 30 years, an AVI cutoff of 12.79 predicts MetS with a sensitivity of 95.92% and a specificity of 93.79%. The area under the ROC curve is 0.97 (p < 0.001).
AVI showed the strongest ability to predict MetS among different genders and age groups.
Discussion
This is the first large-scale cross-sectional study comparing eight anthropometric indices (AVI, WHR, WHtR, CI, BMI, BRI, BAI, ABSI) with cardiovascular risk factors and MetS. Our results show that AVI, among the eight indices examined, correlates more strongly with cardiovascular risk factors and MetS, and it has the strongest ability to predict MetS. This result provides a new means by which to screen for cardiovascular risk factors and MetS.
This study reveals that the prevalence rate of MetS was 16.81% in a sample of 76,915 participants, which is similar to the results in a group of Chinese Singaporeans (16.8%) [18]. This may be attributed to the fact that both studies focus on participants of Chinese ethnicity. It is reported that Indians and Malays have higher MetS prevalence rates than the Chinese. In our study, among the population which meets the criteria for MetS, the most commonly occurring component of MetS is hypertension (40.36%), while the least common is reduced HDL-cholesterol (15.63%). The same components are identified in the study by Ceolin and colleagues in a group of 60–100 year-olds from Brazil. This is the same as the results of Ceolin et al [19].
In our study sample, the AVI of those under the age of 30 years has the largest area under the ROC curve in both male and female populations, consistent with the results of Perona and colleagues [20]. Their study identifies AVI as well as WC as the most powerful discriminating anthropometric indicators of MetS in Spanish adolescents. Studies have shown that WC and AVI can predict MetS in adolescents, but only when using the International Diabetes Federation Diagnostic Criteria [21]. In line with these findings, in our study, we find similar patterns in people under 30 years old using NCEP-ATP diagnostic criteria for MetS.
In a study on postmenopausal women in Brazil, researchers find that anthropometric indices including WC, WHtR, BMI, BAI, and CI have different degrees of correlation with MetS [22]. Compared with the percentage of body fat derived from dual-energy X-ray absorptiometry (DXA), which is considered as the gold standard, indicators of central obesity (such as WC and WHtR) are more strongly correlated with MetS. In that study, their cutoff values for predicting MetS were 88 cm for WC, 0.57 cm/cm for WHtR, 26.85 kg/m2 for BMI, 36.34% for BAI, and 1.24 units for C index. In our study, although we do not employ DXA to measure body fat composition, similarly, we find ABSI and WHtR to be more strongly correlated with MetS. The cutoff value of predicting MetS in women over 60 years old is 79 cm for WC, 0.531 cm/cm for WHtR, 22.99 kg/m2 for BMI, 30.68% for BAI, and 1.22 units for C index. The area under the curve of AVI is superior to that of WHtR, showing a stronger ability for predicting MetS.
In this study, we also assess the ability of anthropometric indicators to distinguish MetS. The results suggest that all anthropometric indexes are capable of significantly differentiating individuals with MetS from those without MetS. Since there is a strong correlation between insulin resistance and dyslipidemia in visceral obesity, it is important to have a high discriminability of obesity index in predicting MetS [23]. Different studies have evaluated anthropometric indicators that best predict metabolic risk factors in different ethnic groups, but the results are inconsistent. There is no consensus on the best predictor of MetS or its major components. For instance, previous studies have shown that WC can predict MetS better than BMI, WHR, and WHtR [24] [21]. However, a cross-sectional study of the Chinese adult population reports that WC, WHR, and BMI are equivalent indicators in distinguishing MetS [25]. Another study concludes that BMI may be a better indicator for screening Chinese men aged 40 and over for obesity, dyslipidemia, and other risk factors, while WHtR may be more suitable for Chinese women, especially those aged 70 and over [26]. Our study indicates that AVI was a better predictor of MetS than BMI in men and women of all ages.
Young people tend to be more active than older people are, and a greater proportion of their weight is attributable to muscle rather than fat. Thus, BMI is not an effective measure of body fat composition, and it is unlikely to be used as a MetS screening indicator in younger populations. A prospective cohort study on the 1552 Qatari adult population demonstrates that WC has better predictive power for MetS compared to other indices such as BMI and WHR [27]. Quaye et al.’s study [28] in a population of 160 apparently healthy normoglycemic normotensive adults showed a better correlation between BMI and WC, with MetS and other cardiovascular risk factors than do AVI, BAI, and C index. The AUCs that BMI and WC predict MetS and its components, as they are larger than that of AVI, BAI, and C index. However, after accounting for gender differences, the AUCs that AVI and C index predict MetS in women, while BMI is still the optimal indicator in men. In this study, some anthropometric indicators are found to be associated with cardiovascular risk factors, which are similar to the results of Yang, et al. [24], Sinaga et al. [29], Tian et al. [30], Lawrence Quaye et al. [28], among other studies. However, our study notes that the AUC of AVI is the largest among all indices examined, and is the best predictor for the MetS. The AUC of C index predicts MetS in all females, but its value is lower than that of BMI. Such as, AVI is the optimal indicator to predict MetS.
Mamtani and Kuljiarni [31] found no correlation between CI and fasting and postprandial glucose, while in this study, it was found that CI was correlated with fasting blood glucose, with a correlation coefficient of 0.208 (p < 0.05), which was still correlated after adjusting for age, gender, smoking, and drinking. Motamed [32] and Wang [33] et al. found that CI and AVI performed better in women after gender stratification.
In the AVI formula, when WC is lower than HC, an increase in HC will lead to an increase in AVI [32]. Since HC of women is larger than WC, an increase in HC usually leads to an increase in AVI. Thus, the predictive ability of AVI for the two genders will be different. As shown in this study, the ability of AVI in women to predict MetS is greater than that in men. AVI demonstrates good differentiation ability in MetS screening. As such, in addition to the more classic obesity indicators of WC, WHR, and WHtR, the newer AVI can also be considered as an appropriate obesity index for MetS screening. Previous studies show that AVI is closely associated with impaired glucose tolerance and type 2 diabetes [32]. The obesity patterns we calculated for AVI are similar to the truncated cones of lower-body obesity in women and upper body obesity in men. The advantage of estimating abdominal volume with AVI is that it predicts what is likely to happen with both patterns. Meanwhile, the belly volume underestimated by the AVI formula is likely to be minimal and does not affect the overall volume estimate.
Mamtani and Kulkarni [31] report no correlation between C index and fasting or postprandial glucose. However, in this study, we find that the C index is correlated with fasting blood glucose, with a correlation coefficient of 0.208 (p < 0.05), even after adjusting for age, gender, smoking, and drinking status. Both Motamed et al. [32] and Wang et al. [33] find that C index and AVI perform better in predicting MetS in women after accounting for gender differences.
Differences in results between studies can be attributed to differences in anthropometric characteristics between ethnicities and nationalities. With regard to ethnic differences, a study on a Kazak sample of adults in Xinjiang China suggests that WHtR was a better indicator screening MetS in Kazak men and women [34], compared to our finding of AVI to be the best predictor of MetS in men and women. These differences may be attributed to racial differences and the use of different diagnostic criteria [35, 36]. Concerning differences between countries, a study of Korean men at MetS screening reports that WHtR shows greater discrimination compared to BMI. They find WHR to be the best screening tool and is more effective than either BMI or WC in screening for adult metabolic risk factors [37], compared with AVI in our study. The San Antonio Heart Study [38] in non-Hispanic whites and Mexican–American reports that BMI and WC have similar predictive effects for MetS development. The variations of findings may be related to different characteristics of obesity based on ethnicity [39].
AVI has a good differentiation ability in MetS screening. Therefore, besides WC, WHR, and WHtR, AVI can also be considered as the appropriate obesity index for MetS screening. AVI is extrapolated from anthropometry and is closely related to impaired glucose tolerance and type 2 diabetes [8].
Moreover, studies in China showed that the overall burden of CVD in China has significantly increased, with obvious regional differences [40]. In this study, we note that AVI has a strong MetS discrimination ability in a sample of urban residents in west China. Taken together the advantages of AVI and results from our study, it is of great practical significance to be able to use AVI for MetS screening in Chengdu metropolitan area.
In our study, we note that the means of BAI in women are higher than that of men; the BAI values increase with the increase of age, with the highest value over 60 years of age. The reason may come from changes in body composition in postmenopausal women. Decreased estrogen production leads to increased age-related obesity and metabolic disorders. Women of this age group show less physical activity, which may lead to decreased thigh muscle mass and increased visceral fat accumulation [41]. In the normal distribution of male and female adipose tissue, women tend to accumulate excess fat in the lower buttocks (thighs and buttocks). Later, they tend to accumulate excess fat in the upper body, giving rise to central obesity [42]. In addition, women naturally store fat under the skin, while men store fat in the gut.
In our study, we report that ABSI shows the lowest AUC across genders and different age groups in predicting MetS, indicating that ABSI is the weakest predictor of MetS in the population. Zhou et al. [17] find that ABSI and C index are the weakest indicators of MetS in middle-aged and elderly maintenance hemodialysis patients in China. This is similar to our findings on ABSI. Similarly, Zhang et al.’s study [43] report that ABSI shows the lowest AUC in discriminating MetS and most cardiometabolic risk factors. Therefore, ABSI could not be used as an alternative index.
Our study utilizes an anthropometric approach to predict the optimal cutoff point for MetS, which can be easily used in clinical practice. We find that the critical value of other anthropometric indicators with exception of ABSI can be used to identify MetS. Additionally, some anthropometric indicators, such as BAI, predict MetS in women more strongly than in men. As such, applying the measures selectively, such as using the BAI for women only, taking into account gender differences in clinical practice is crucial and more efficient.
This study has some limitations. First, this is a cross-sectional study that provides less evidence over time than results from longitudinal or randomized epidemiological studies. The cross-sectional nature of the design does not allow for the direction of the assessment of risk factors and health outcomes. Further cohort studies are needed to confirm the findings. Second, because the study is conducted in a single ethnic group, caution should be exercised in extending these results to other ethnic groups. Well-designed longitudinal studies across different ethnic groups are needed to extend our findings. Despite the limitations, we believe our study sample size is substantially large, to contribute significantly to the current literature on identifying anthropometric indices that can most effectively predict MetS.
Conclusion
AVI, WHR, WHtR, BMI, C index, and BAI are anthropometric indices significantly associated with cardiovascular risk factors. Anthropometric indices are useful screening tools for MetS or its components, and cardiovascular risk factors. Of all the anthropometric indices studied, AVI is the best index in distinguishing MetS.
Data availability
The data used in this study were collected from the Health Management Center of West China Hospital. The data belongs to West China hospital. Those data are not publicly obtainable.
References
Ma LY, Chen WW, Gao RL, Liu LS, Zhu ML, Wang YJ, et al. China cardiovascular diseases report 2018: an updated summary. J Geriatr Cardiol. 2020;17(1):1–8. https://doi.org/10.11909/j.issn.1671-5411.2020.01.001.
Cleeman JI, Grundy SM, Becker D, Clark LT, Cooper RS, Denke MA, et al. Executive summary of the Third Report of the National Cholesterol Education Program (NCEP) expert panel on detection, evaluation, and treatment of high blood cholesterol in adults (Adult Treatment Panel III). Jama-Journal of the American Medical Association. 2001;285(19):2486–97. https://doi.org/10.1001/jama.285.19.2486.
Saklayen MG. The global epidemic of the metabolic syndrome. Curr Hypertens Rep. 2018;20(2):12. https://doi.org/10.1007/s11906-018-0812-z.
Gonzalez-Chávez A, Chávez-Fernández JA, Elizondo-Argueta S, González-Tapia A, León-Pedroza JI, Ochoa C. Metabolic syndrome and cardiovascular disease: a health challenge. Arch Med Res. 2018;49(8):516–21. https://doi.org/10.1016/j.arcmed.2018.10.003.
Perissinotto E, Pisent C, Sergi G, Grigoletto F. Anthropometric measurements in the elderly: age and gender differences. Br J Nutr. 2002;87(2):177–86. https://doi.org/10.1079/bjn2001487.
Steen B. Body composition and aging. Nutr Rev. 1988;46(2):45–51. https://doi.org/10.1111/j.1753-4887.1988.tb05386.x.
Almeida NS, Rocha R, Cotrim HP, Daltro C. Anthropometric indicators of visceral adiposity as predictors of non-alcoholic fatty liver disease: a review. World J Hepatol. 2018;10(10):695–701. https://doi.org/10.4254/wjh.v10.i10.695.
Guerrero-Romero F, Rodriguez-Moran M. Abdominal volume index. An anthropometry-based index for estimation of obesity is strongly related to impaired glucose tolerance and type 2 diabetes mellitus. Arch Med Res. 2003;34(5):428–32. https://doi.org/10.1016/s0188-4409(03)00073-0.
Patil VC, Parale GP, Kulkarni PM, Patil HV. Relation of anthropometric variables to coronary artery disease risk factors. Indian J Endocr Metab. 2011;15(1):31–7. https://doi.org/10.4103/2230-8210.77582.
Ruperto M, Barril G, Sanchez-Muniz FJ. Conicity index as a contributor marker of inflammation in haemodialysis patients. Nutr Hosp. 2013;28(5):1688–95. https://doi.org/10.3305/nh.2013.28.5.6626.
Bergman RN, Stefanovski D, Buchanan TA, Sumner AE, Reynolds JC, Sebring NG, et al. A better index of body adiposity. Obesity. 2011;19(5):1083–9. https://doi.org/10.1038/oby.2011.38.
Krakauer NY, Krakauer JC. A new body shape index predicts mortality hazard independently of body mass index. PLoS One. 2012;7(7):e39504. https://doi.org/10.1371/journal.pone.0039504.
Thomas DM, Bredlau C, Bosy-Westphal A, Mueller M, Shen W, Gallagher D, et al. Relationships between body roundness with body fat and visceral adipose tissue emerging from a new geometrical model. Obesity. 2013;21(11):2264–71. https://doi.org/10.1002/oby.20408.
Nevill AM, Duncan MJ, Lahart IM, Sandercock GR. Scaling waist girth for differences in body size reveals a new improved index associated with cardiometabolic risk. Scand J Med Sci Sports. 2017;27(11):1470–6. https://doi.org/10.1111/sms.12780.
Lee DY, Lee MY, Sung KC. Prediction of mortality with a body shape index in young Asians: comparison with body mass index and waist circumference. Obesity. 2018;26(6):1096–103. https://doi.org/10.1002/oby.22193.
Wu Q, Qin K, Wang Y. Central obesity is associated with helicobacter pylori infection: a large-scale cross-sectional retrospective study in West China. Int J Diabetes Dev Ctries. 2019;40(1):52–60. https://doi.org/10.1007/s13410-019-00765-8.
Zhou CM, Zhan L, Yuan J, Tong XY, Peng YH, Zha Y. Comparison of visceral, general and central obesity indices in the prediction of metabolic syndrome in maintenance hemodialysis patients. Eating and Weight Disorders-Studies on Anorexia Bulimia and Obesity. 2020;25(3):727-34. doi:10.1007/s40519-019-00678-9.
Nurjono M, Lee J. Waist circumference is a potential indicator of metabolic syndrome in Singaporean Chinese. Ann Acad Med Singapore. 2013;42(5):241–5.
Ceolin J, Engroff P, Mattiello R, Schwanke CHA. Performance of Anthropometric Indicators in the Prediction of Metabolic Syndrome in the Elderly. Metabolic Syndrome and Related Disorders. 2019;17(4):232-9. doi:10.1089/met.2018.0113.
Perona JS, Rio-Valle JS, Ramirez-Velez R, Correa-Rodriguez M, Fernandez-Aparicio A, Gonzalez-Jimenez E. Waist circumference and abdominal volume index are the strongest anthropometric discriminators of metabolic syndrome in Spanish adolescents. European Journal of Clinical Investigation. 2019;49(3):e13060. doi:10.1111/eci.13060.
Perona JS, Schmidt-RioValle J, Fernandez-Aparicio A, Correa-Rodriguez M, Ramirez-Velez R, Gonzalez-Jimenez E. Waist Circumference and Abdominal Volume Index Can Predict Metabolic Syndrome in Adolescents, but only When the Criteria of the International Diabetes Federation are Employed for the Diagnosis. Nutrients. 2019;11(6):1370. doi:10.3390/nu11061370.
Gadelha AB, Myers J, Moreira S, Dutra MT, Safons MP, Lima RM. Comparison of adiposity indices and cut-off values in the prediction of metabolic syndrome in postmenopausal women. Diabetes Metab Syndr. 2016;10(3):143–8. https://doi.org/10.1016/j.dsx.2016.01.005.
Despres J-P, Lemieux I. Abdominal obesity and metabolic syndrome. Nature. 2006;444(7121):881–7. https://doi.org/10.1038/nature05488.
Yang YJ, Park HJ, Won KB, Chang HJ, Park GM, Kim YG, et al. Relationship between the optimal cut-off values of anthropometric indices for predicting metabolic syndrome and carotid intima-medial thickness in a Korean population. Medicine. 2019;98(42):e17620. https://doi.org/10.1097/md.0000000000017620.
Ko K-P, Oh D-K, Min H, Kim C-S, Park J-K, Kim Y, et al. Prospective study of optimal obesity index cutoffs for predicting development of multiple metabolic risk factors: the Korean genome and epidemiology study. J Epidemiol. 2012;22(5):433–9. https://doi.org/10.2188/jea.JE20110164.
He YH, Chen YC, Jiang GX, Huang HE, Li R, Li XY, et al. Evaluation of anthropometric indices for metabolic syndrome in Chinese adults aged 40 years and over. Eur J Nutr. 2012;51(1):81–7. https://doi.org/10.1007/s00394-011-0195-2.
Bener A, Yousafzai MT, Darwish S, Al-Hamaq AOAA, Nasralla EA, Abdul-Ghani M. Obesity index that better predict metabolic syndrome: body mass index, waist circumference, waist hip ratio, or waist height ratio. J Obes. 2013. https://doi.org/10.1155/2013/269038.
Quaye L, Owiredu W, Amidu N, Dapare PPM, Adams Y. Comparative Abilities of Body Mass Index, Waist Circumference, Abdominal Volume Index, Body Adiposity Index, and Conicity Index as Predictive Screening Tools for Metabolic Syndrome among Apparently Healthy Ghanaian Adults. Journal of Obesity. 2019;2019:8143179. doi:10.1155/2019/8143179.
Sinaga M, Worku M, Yemane T, Tegene E, Wakayo T, Girma T, et al. Optimal cut-off for obesity and markers of metabolic syndrome for Ethiopian adults. Nutr J. 2018;17(1):109. https://doi.org/10.1186/s12937-018-0416-0.
Tian T, Zhang J, Zhu Q, Xie W, Wang Y, Dai Y. Predicting value of five anthropometric measures in metabolic syndrome among Jiangsu Province, China. BMC Public Health. 2020;20(1):1317. https://doi.org/10.1186/s12889-020-09423-9.
Mamtani MR, Kulkarni HR. Predictive performance of anthropometric indexes of central obesity for the risk of type 2 diabetes. Arch Med Res. 2005;36(5):581–9. https://doi.org/10.1016/j.arcmed.2005.03.049.
Motamed N, Sohrabi M, Poustchi H, Maadi M, Malek M, Keyvani H, et al. The six obesity indices, which one is more compatible with metabolic syndrome? A population based study. Diabetes Metab Syndr. 2017;11(3):173–7. https://doi.org/10.1016/j.dsx.2016.08.024.
Wang H, Liu A, Zhao T, Gong X, Pang T, Zhou Y, et al. Comparison of anthropometric indices for predicting the risk of metabolic syndrome and its components in Chinese adults: a prospective, longitudinal study. BMJ Open. 2017;7(9):e016062. https://doi.org/10.1136/bmjopen-2017-016062.
Zhang XH, Zhang M, He J, Yan YZ, Ma JL, Wang K, et al. Comparison of anthropometric and atherogenic indices as screening tools of metabolic syndrome in the Kazakh adult population in Xinjiang. Int J Environ Res Public Health. 2016;13(4):428. https://doi.org/10.3390/ijerph13040428.
Gharipour M, Sadeghi M, Dianatkhah M, Bidmeshgi S, Ahmadi A, Tahri M, et al. The cut-off values of anthropometric indices for identifying subjects at risk for metabolic syndrome in Iranian elderly men. Journal of obesity. 2014;2014:907149. https://doi.org/10.1155/2014/907149.
Llinas MG, Janer PE, Agudo SG, Casquero RG, Gonzalez IC. Usefulness in nursing of different anthropometric and analytical indices to assess the existence of metabolic syndrome with the NCEP ATP III and IDF criteria in Spanish Mediterranean population. Medicina Balear. 2017;32(1):26–34. https://doi.org/10.3306/medicinabalear.32.01.26.
Ashwell M, Gunn P, Gibson S. Waist-to-height ratio is a better screening tool than waist circumference and BMI for adult cardiometabolic risk factors: systematic review and meta-analysis. Obesity Reviews. 2012;13(3):275-86. doi:10.1111/j.1467-789X.2011.00952.x.
Han T, Williams K, Sattar N, Hunt K, Lean M, Haffner S. Analysis of obesity and hyperinsulinemia in the development of metabolic syndrome: San Antonio Heart Study. Obes Res. 2002;10:923–31. https://doi.org/10.1038/oby.2002.126.
Guzman de la Garza FJ, Salinas-Martinez AM, Gonzalez-Guajardo E, Palmero-Hinojosa MG, Castro-Garza J, Ramirez-Zuniga JC, et al. Threshold values of sagittal abdominal diameter for the detection of cardio-metabolic risk factors in Northeastern Mexico: a cross-sectional study. Nutricion Hospitalaria. 2016;33(3):268. https://doi.org/10.20960/nh.268.
Zhang GS, Yu CH, Luo LS, Li YC, Zeng XY. Trend analysis of the burden of ischemic heart disease in China, 1990 to 2015. Zhonghua yu fang yi xue za zhi [Chinese journal of preventive medicine]. 2017;51(10):915-21. doi:10.3760/cma.j.issn.0253-9624.2017.10.009.
Arthur FK, Adu-Frimpong M, Osei-Yeboah J, Mensah FO, Owusu L. Prediction of metabolic syndrome among postmenopausal Ghanaian women using obesity and atherogenic markers. Lipids Health Dis. 2012;11:101. https://doi.org/10.1186/1476-511x-11-101.
Williams CM. Lipid metabolism in women. Proceedings of the Nutrition Society. 2004;63(1):153–60. https://doi.org/10.1079/pns2003314.
Zhang J, Zhu WH, Qiu LF, Huang LJ, Fang LZ. Sex- and age-specific optimal anthropometric indices as screening tools for metabolic syndrome in Chinese adults. Int J Endocrinol. 2018. https://doi.org/10.1155/2018/1067603.
Acknowledgements
We give our thanks to the research group members in Health Management Center, West China Hospital, Sichuan University, for their generous support.
Funding
This work was supported by the Sichuan Science and Technology Program (Grant No. 2017RZ0046, Grant No. 2018SZ0087, Grant No. 2018HH0099), a grant from the Sichuan province health department (Grant No. Chuanganyan2012-111), and the Youth Teacher Research Startup Fund of Sichuan University (2016SCU11016).
Author information
Authors and Affiliations
Contributions
Qinqin Wu and Hu Nie Designed the study, executed the study, analyzed the results, and contributed to the drafting of the manuscript. Qinqin Wu and Ken Qin Analyzed and interpreted the data in the revised version. Qinqin Wu and Youjuan Wang Contributed to designing the study and discussion of results, and the final manuscript. All authors have read and approved the final manuscript.
Corresponding author
Ethics declarations
Ethics approval and consent to participate
This study was approved by the Ethics Committee of West China Hospital of Sichuan University. As this is a retrospective research, informed consent was not essential according to the Ethical Guidelines for Epidemiological Research. The study was allowed by the Ethics Committee of West China, Sichuan University.
Consent for publication
Not applicable.
Competing interests
The authors declare no competing interests.
Additional information
Publisher's note
Springer Nature remains neutral with regard to jurisdictional claims in published maps and institutional affiliations.
Rights and permissions
About this article
Cite this article
Wu, Q., Qin, K., Wang, Y. et al. Anthropometric indices and their predictive ability on metabolic syndrome in west China. Int J Diabetes Dev Ctries 42, 666–682 (2022). https://doi.org/10.1007/s13410-021-01020-9
Received:
Accepted:
Published:
Issue Date:
DOI: https://doi.org/10.1007/s13410-021-01020-9