Abstract
Lactococcus lactis subsp. cremoris MG1363 is an opportunistic lactic acid bacterium (LAB) that has emerged as one of the most promising candidate cell factories. The availability of genome-level information and U.S. Federal Drug administration’s designation of ‘generally recognized as safe’ (GRAS) are two of the more important key factors for its wide-ranging applications in numerous biotechnological processes. Several studies have shown that various physiological conditions, such as temperature, salinity and pH, can influence the physiological growth of L. lactis; agitation, in particular, can increase the production of amino acids and fermentation by-products. However, the effect of different agitation speeds on the growth of L. lactis’ has rarely been examined. In the study reported here, we used a gas chromatography–mass spectrometry-based metabolomics approach to investigate the effects of different agitation speeds on the production of proteinogenic amino acids (PAAs) by L. lactis MG1363. Lactococcus lactis MG1363 was grown under four different agitation speeds (50, 100, 150 and 200 rpm) at a constant temperature of 30 °C, and the differences in the specific growth rate and levels of PAAs were determined. Approximately 15 PAAs with concentrations ranging from 0 to 50 mmol/L were detected under all conditions. Partial least squares discriminant analysis (PLS-DA) revealed a distinct difference when L. lactis was incubated at 100 and 150 rpm. Heatmap analysis showed that the levels of pyruvate-, glutamate- and aspartate-based amino acids were varied under the different agitation conditions. The time-series analysis showed an increment of lysine when L. lactis’ cells were cultured with shaking at 50, 100 and 200 rpm. Taken together, these results highlight the changes in the levels of PAAs in L. lactis cells in response to agitation. In addition, the collected dataset will be useful for optimization of 13C-labeling based experiments in L. lactis.
Similar content being viewed by others
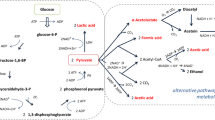
Introduction
Lactococcus lactis is a Gram-positive, mesophilic and microaerophilic lactic acid bacterium (LAB) that is of great importance in the fermentation industry (Miyoshi et al. 2003; Lahtvee et al. 2011). In fermentation processes, L. lactis strains are used as starter culture and can contribute to the taste and flavor of the fermented end-products by producing organoleptic properties (Brandsma et al. 2012; Flahaut et al. 2013; Dijkstra et al. 2014; van de Bunt et al. 2014). Lactococcus lactis is known to be auxotrophic for several amino acids, with the specific amino acids varying among strains. It has been shown that L. lactis strains require six essential amino acids, namely, methionine (Met), glutamate (Glu), valine (Val), histidine (His), leucine (Leu) and isoleucine (Ile), for growth (van Niel and Hahn-Hagerdal 1999; Oliveira et al. 2005). Like other LAB, L. lactis uses amino acids to perform physiological functions, such as intracellular pH control, the generation of metabolic energy or redox power and stress resistance (Fernández and Zúñiga 2006). Moreover, amino acids play important roles in the central metabolic pathway of L. lactis. For example, amino acids such as alanine (Ala), cysteine (Cys), glycine (Gly), serine (Ser), aspartate (Asp) and threonine (Thr) are responsible either directly or indirectly for the production of pyruvate (Pyr), which then leads to fermentation by-product metabolites of lactate, acetate and ethanol (Adamberg et al. 2012).
Consequently, during fermentation processes, L. lactis strains are exposed to a number of stresses that affect the growth and performance of L. lactis starters (Dijkstra et al. 2014). Several studies have reported physiological changes in L. lactis when exposed to stresses such as pH (Carvalho et al. 2013; de Jong et al. 2013), temperature (Ibrahim et al. 2010; Azizan et al. 2012), oxidative stress or aeration (Nordkvist et al. 2003; Ibrahim et al. 2010; Cretenet et al. 2014; Dijkstra et al. 2014) and nutrient starvation (Dressaire et al. 2011). More importantly, these studies were mainly conducted under anaerobic conditions to mimic the fermentation process conditions. As facultative anaerobes, L. lactis is able to grow under aerobic conditions, and it has an oxygen resistance mechanism that enables it to adapt to certain levels of toxic substances in the environment, such as superoxide (Pedersen et al. 2012). Its ability to tolerate oxygen at an early stage of growth is a great advantage in terms of maintaining cell viability during early cultivation. It has been reported that aeration during fermentation triggers resistance against heat stress, whereas high temperature leads to robustness to oxidative stress (Cretenet et al. 2014). Ibrahim et al. (2010) reported that recombinant L. lactis responds differently when grown at different agitation speeds and temperatures. The results of a previous study by our group suggest that L. lactis MG1363 produces various low-molecular-weight metabolites when grown under agitation (Azizan et al. 2012). In that study, levels of organic acids primary alcohols, aldehydes, amino acids and fatty acids increased when L. lactis MG1363 was grown at 30 °C and an agitation speed of 150 rpm (Azizan et al. 2012).
In order to understand the association between amino acid levels in L. lactis and agitation speed, it would be useful to analyze and measure the metabolism patterns of proteinogenic amino acids (PAAs) found in L. lactis. PAAs are relatively stable and abundant inside the cell (Szyperski 1995; Zamboni et al. 2009). Through analysis of these protein-bound amino acids, the response of metabolic pathways inside the cell to environmental stresses can be analyzed in more detail, specifically, central carbon and amino acid metabolism (Szyperski 1995).
In this study, we used a gas chromatography mass spectrometry (GC–MS)-based metabolomics approach to investigate the effects of different agitation speeds on the production of PAAs by L. lactis MG1363. Briefly, L. lactis was cultivated at 30 °C under four different agitation speeds, and the PAAs were extracted from the cell pellets and quantified. The results indicate notable differences in the levels of PAAs when L. lactis was exposed to different agitation speeds, suggesting that agitation speed plays an important role in increasing the viability of L. lactis when subjected to environmental perturbations.
Materials and methods
Bacterial cultivation
The Lactococcus lactis subsp. cremoris MG1363 used in this study was provided by Raha Abd. Rahim from Universiti Putra Malaysia, Serdang, Malaysia, courtesy of K. Leenhouts from University of Groningen, Groningen, The Netherlands. All cultivations were performed as batch cultivations with working volumes of 100 mL. Cells were grown in M17 medium (ascorbic acid, 0.5 g/L; MgSO4, 0.25 g/L; disodium glycerophosphate, 19 g/L; tryptone, 5 g/L; soytone, 5 g/L; beef extract, 2.5 g/L; yeast extract, 2.5 g/L; Oxoid Ltd. Hampshire, UK) media with glucose (0.5 g/L) as the carbon source, with a minimum of three biological replicates. Incubation was performed at 30 °C with shaking at agitation speeds of 50, 100, 150 and 200 rpm, respectively. The control was L. lactis cells grown at 30 °C without agitation. Cell growth was monitored by measuring optical density at 600 nm (OD600) on a spectrophotometer (model DU 800; Beckman Coulter, Brea, CA). The specific growth rate (μ) was calculated using a linear regression of the plots of ln(OD600) versus time during the exponential growth phase.
Extraction of PAAs
Cellular extracts for all experiments were obtained by harvesting the cells at different time points (3, 5, 6, 7 h) during the exponential and stationary phases. More specifically, the bacterial culture was centrifuged at 13000 rpm for 10 min at 4 °C, following which the pellet was washed twice with 1 mL phosphate buffer saline solution and transferred into a new glass vial. Approximately 200 μL of 6 M HCl was then added to the cell pellet, and the cell pellet subjected to cell hydrolysis by heating at 105 °C for 16 h. The cell hydrolysate was then further dried at 95 °C for 6 h.
Detection and quantification of PAAs
The derivative technique was used as described by Zamboni et al. (2009). Cell hydrolysates were heated for 10 min at 105 °C to dry the samples completely prior to derivatization. The dried samples were then dissolved in dimethylformamide and derivatized with N-tert-butyldimethylsilyl-N-methyltrifluoroacetamide with 1% tert-butyldimethylchlorosilane for 1 h at 85 °C. The derivatized samples were then transferred into new GC vials and analyzed by GC–MS (Zamboni et al. 2009; Azizan et al. 2012). The PAAs detected were then quantified using an external calibration curve.
GC-MS parameters
An aliquot of approximately 1 μL was injected into an Elite-5MS capillary column coated with 5 % diphenyl 95 % dimethyl polysiloxane (ID 30 × 0.25 mm, thickness 0.25 μm) (PerkinElmer, Waltham, MA). The injection temperature was set to 250 °C, and the ion source was set to 200 °C. The GC system was set to range from 70 to 300 °C with a constant helium gas flow at 1.1 min−1. Measurements were performed in a scan mode of m/z 180–550. Analysis was performed using the PerkinElmer TurboMass Clarus 600 spectrometer coupled to a quadruple mass selective detector with electron ionization operated at 70 eV.
Multivariate statistical analysis of GC-MS data
The general approach used for data analysis and validation was performed according to Villas-Bôas et al. (2006) and Smart et al. (2010). In summary, detected metabolites were identified using an in-house TMS library of derivatized pure standards and the 2008 National Institute of Standards and Technology (NIST) mass spectral database library (NIST, Gaithersburg, MD) with a similarity cut-off of 90%. The area of the peak was used to represent the abundance of the detected metabolites. The values were normalized by sample median followed by log transformation. One-way analysis of variance (ANOVA) followed by comparison using Fisher’s least significant difference and two-way ANOVA was used where necessary to statistically validate the values and method with a significance level of P ≤ 0.05. Visualization of the clean, validated data was then conducted using principal component analysis and partial least squares-discriminant analysis (PLS-DA) in Simca-P+ version 12.0 (Umetrics AB, Ume, Sweden) for group classification and discrimination analysis (Azizan et al. 2012).
Results
Growth characteristics of L. lactis MG1363 at different agitation speeds
Cultures of strain MG1363 were grown at four different agitation speeds (50, 100, 150, and 200 rpm) at 30 °C with a limited supply of glucose, resulting in variation in growth characteristics, including specific growth rate (μ) and optical density (OD600). Incubation at an agitation speed of 100 rpm resulted in the highest growth rate (0.890 h−1), and incubation at an agitation speed of 200 rpm resulted in the lowest growth rate (0.686 h−1). Overall, the growth rate was lower at 200 rpm than at 50, 100, and 150 rpm [Electronic Supplementary Material (ESM) Table 1].The maximum OD600 at the end of incubation period ranged from 0.45 to 0.47 (Fig. 1). There was no difference in the duration of lag phase of cultures grown at the different agitation speeds and, in addition, the different agitation speeds had no effect on the growth profiles of L. lactis. Before this bacterium entered the exponential phase it had a lag phase of 2 h. The mid-exponential phase of L. lactis was reached after 3–4 h of incubation, while the stationary phase started after 6 h of incubation.
Growth profiles of Lactococcus lactis during incubation at 30 °C and at different agitation speeds. Lightly-shaded long-dashed line, filled diamond 0 rpm, short-dashed/dotted line, filled circle 50 rpm, short-dashed line, filled square 100 rpm, dotted line, filled triangle 150 rpm, dark long-dashed line, X 200 rpm
PAAs and multivariate statistical analysis
Specifically, 16 PAAs were identified using GC-MS, as shown in ESM Fig. 1. The area of each PAAs was then further processed using multivariate statistical analysis (Fig. 2). Except for proline, all PAAs displayed p < 0.05.
Principal component analysis (PCA) score scatter plot (a) and loading scatter plot (b) of proteinogenic amino acid (PAA) profiles that distinguished L. lactis grown under non-agitated and agitated conditions. The proportions of variance between spectra explained by principal components 1 and 2 were 27.45 and 22.95 %, respectively. c Partial least squares-discriminant analysis (PLS-DA)-derived score plot analysis of metabolite profiles with latent variable (LV) 1 and 2 of 29.39 and 18.87%, respectively. d PLS-DA-derived loading scatter plot analysis of profiled metabolites. PLS-DA analysis with agitation speed as the y variable was used to identify the metabolites that distinguished L. lactis grown under non-agitated and agitated conditions. Open box (d) Metabolites with variable importance for projection (VIP) values of >1 were highlighted in red box. Agitation speeds: Cross 0 rpm, X 50 rpm, filled diamond 100 rpm, filled triangle 150 rpm, filled square 200 rpm
PCA was first used to show treatment-to-treatment variation and to identify variations in the level of PAAs when the culture was grown at different agitation speeds (Fig. 2a, b), and PLS-DA was then performed to provide a better visualization of the treatment clustering found by PCA. As shown in Fig. 2c, d, good clustering was observed in the score plot of PLS-DA, with a total variance of 48 % (LV1 29.39%, LV2 18.87%). The score plot showed clear discrimination of between cells grown at 100 and 150 rpm. The loading plot suggested that Ala, Gly and Met were the PAAs which most contributed towards the separation of clusters at 100 and 150 rpm. To evaluate the metabolic changes in the levels of PAAs, a heatmap was constructed to compare the overall levels of PAAs in response to different agitation speeds.
The heatmap in Fig. 3 revealed differences in the levels of PAAs detected when L. lactis was grown at the four different agitation speeds. The levels of Ala and Gly were higher at an agitation speed of 100 rpm and lower at 150 and 200 rpm, while those of Leu, Ile and lysine (Lys) were higher at 50 and 200 rpm but lower at 150 rpm. The heatmap showed that the levels of branched-chain amino acids (Ile, Leu) were higher at 200 rpm, at which agitation speed the culture showed a low specific growth rate. However, a comparison between cultures grown under agitation and the control showed that the levels of Leu, Ile, tyrosine (Tyr) and Met were higher when grown without agitation.
Pattern of PAA changes in response to time
To further characterize the metabolic changes at the level of PAAs in response to different agitation speeds, we harvested cell pellets at four different time points (3, 5, 6 and 7 h of incubation) and compared the PAA profiles. As shown in Fig. 4, the levels of the majority of PAAs decreased from 3 to 7 h of incubation. However, notable increases in the levels of some PAAs were observed at the end of the incubation period of 8 h, with the level of Lys increasing by 0.9, 0.3 and 0.25-fold at 50, 100, and 200 rpm, respectively. In addition, the level of ornithine (Orn) increased by 0.82-fold from 3 to 6 h and decreased slightly by 0.1-fold at 7 h of incubation at 200 rpm.
Levels of PAAs in the amino acid pathways. Each column of each section of each graph represents the growth phases studied (left to right incubation time: 3, 5, 6, 7 h). Patterns of bars as indicated in the Legends box indicate agitation speed. The pathway is adapted from the KEGG database. Glu Glucose, ACoA acetyl-CoA, CIT citrate, AKG α-ketoglutarate, OAA oxaloacetate, TCA tricarboxylic acid. Asterisk PAAs showing a significant change at P ≤ 0.05
Discussion
The variations in the levels of PAAs shown in Fig. 3 may suggest the response mechanisms of central metabolism of L. lactis towards agitation perturbations. The results may also provide an overview of the production and consumption of amino acids by L. lactis MG1363 at different agitation speeds.
To further demonstrate the relation between the specific growth rates of L. lactis MG1363 and the PAAs produced, we compared the levels of PAAs in L. lactis MG1363 cells cultured at different agitation speeds. The level of Orn was the highest at 200 rpm despite a low specific growth rate. Our findings are similar to those of Dressaire et al. (2008) and Lahtvee et al. (2011) who suggested that genes encoding enzymes in the Orn biosynthesis pathway through the arginine deiminase pathway and in Glu metabolism are upregulated at the lowest specific growth rate. These findings highlight the importance of Orn metabolism in the growth rate adaptation of L. lactis MG1363. Concomitantly, in our study a high specific growth rate was associated with a decrease in amino acid metabolism and low levels of Orn, which was also reported by Dressaire et al. (2011).
In our study the level of Lys increased throughout the exponential phase until the early stationary phase. The observed increase in the level of Lys might be due in part to the lack of a Lys transport system in L. lactis subsp. cremoris (Dreissen et al. 1989). Lys and arginine (Arg) are used by some lactococcal strains as cell-wall constituents. In the basic amino acid transport system, Arg is degraded into Orn through the arginine deiminase pathway, which yields ATP. This ATP is then used to generate proton-motive force, which acts as a driving force for Lys accumulation. Since L. lactis subsp. cremoris lacks the Arg:Orn antiporter, which somehow helps to counterflow the uptake of Lys and Arg, Lys is accumulated inside the cell. This interpretation is supported by the increase in the level of Lys detected inside the cell during the incubation period.
In L. lactis, branched-chain amino acids (BCAAs), namely, Val, Leu and Ile, are essential for protein synthesis and act as precursors for volatile compounds. BCAAs are degraded into α-keto acids by branched chain aminotransferase (BcaT) and aromatic aminotransferase (AraT). To produce volatile compounds, they are further converted to aldehydes and esters (Dressaire et al. 2008, 2011; García-Cayuela et al. 2012). The biosynthesis of BCAAs is regulated by the CodY repressor protein. CodY is mediated by Ile, so that an excess of Ile leads to growth inhibition by blocking CodY-dependent pathways. In our study, high levels of Ile were detected at 200 rpm, accompanied by lower specific growth rates of L. lactis, as also demonstrated by Guédon et al. (2005).
We observed that the level of Ala was higher when L. lactis cells were cultivated at 100 rpm but lower at 150 rpm. Ala is a Pyr-based amino acid which is produced through the reduction of Pyr by alanine dehydrogenase (Hols et al. 1999). Ala is also used by LAB as a cell-wall constituent (Chapot-Chartier and Kulakauskas 2014). This amino acid is of great interest to both the food and pharmaceutical industries in the context of bioengineering activity which would turn Lactococcus into a cell factory to produce Ala instead of lactate (Papagianni 2012). We found that the level of Gly was high at agitation speeds of 50, 100, and 150 rpm. This finding is in agreement with a study conducted by Lahtvee et al. (2011) in which the consumption of Gly was observed to increase with increasing growth rate. We found different levels of Met at the different agitation speeds. This sulfur-containing amino acid is known to be one of the essential amino acids for L. lactis growth, and it is an important component of flavor formation. Interestingly, Met can be used to regenerate NAD+ when arginine is used as the energy source (Brandsma et al. 2012). However, in our study, the different levels of Met detected did not correlate with the different agitation speeds used.
Lactococcus lactis is classified as a facultative anaerobe that is unable to fully oxidize sugar to CO2 via the tricarboxylic acid (TCA) cycle even in the presence of oxygen (Price et al. 2012). Wegmann et al. (2007) published the complete genome sequence of L. lactis subsp. cremoris MG1363 in which they mentioned the incomplete TCA cycle of this bacterium. In the presence of oxygen, the transcript levels for the TCA cycle are upregulated, while the transcript levels for glycolysis are downregulated (Ferreira et al. 2013), with the result that hetero-fermentative fermentation occurs. Bolotin et al. (2001) reported that hetero-fermentative fermentation will occur when the pentose phosphate pathway is active, as in this case glycolysis will produce more compounds to be converted to lactate and ethanol. In our study, we found that the level of amino acids originating from the TCA cycle by L. lactis varied at the different agitation speeds. Thee levels of amino acids originating from the TCA cycle at 0 rpm were expected to be low—but were instead high. This result strongly suggests that these amino acids may be produced from other precursors or pathways other than the TCA cycle; for example, Thr can be derived from Gly (Thr < − >Gly + acetaldehyde). This notion is supported by Oliveira et al.’s (2005) study. The ability to perform biphasic fermentative fermentation and respiration helps L. lactis to maintain better long-term survival if exposed to oxygen (Wegmann et al. 2007).
Furthermore, under aerobic conditions and limited carbon sources, Pyr is converted into acetyl-CoA by pyruvate dehydrogenase (Oliveira et al. 2005) and produces mixed-acid fermentation products. Thus, L. lactis MG1363 can switch to hetero-fermentative fermentation to generate more ATP than the homolactic pathway, even though the end-products of the mixed-acid pathway are more inhibitory than lactate to the bacterium itself (Price et al. 2012). This result strongly suggests that agitation will affect the production of TCA cycle-derived amino acids by supplying more dissolved oxygen to the medium, as demonstrated by Chen et al. (2013).
Conclusion
In this study, we demonstrated metabolic changes in PAAs in response to different agitation speeds. The PLS-DA score plot showed that Met, Ala and Gly were the PAAs which most contributed towards the separation of clusters at 100 and 150 rpm. The time-series analysis showed an increase of Lys at 50, 100 and 200 rpm. These results highlight the importance of PAA inter-relationships as an adaption response to environmental perturbation. Moreover, the results are useful for future optimization of 13C–labeling-based experiments in L. lactis.
References
Adamberg K, Seiman A, Vilu R (2012) Increased biomass yield of Lactococcus lactis by reduced overconsumption of amino acids and increased catalytic activities of enzymes. PloS ONE 7(10):e48223
Azizan KA, Baharum SN, Mohd Noor N (2012) Metabolic profiling of Lactococcus lactis under different culture conditions. Molecules 17:8022–8036
Bolotin A, Wincker P, Mauger S, Jaillon O, Malarme K, Weissenbach J, Ehrlich SD, Sorokin A (2001) The complete genome sequence of the lactic acid bacterium Lactococcus lactis ssp. lactis IL1403. Genome Res 11:731–753
Brandsma JB, van de Kraats I, Abee T, Zwietering MH, Meijer WC (2012) Arginine metabolism in sugar deprived Lactococcus lactis enhances survival and cellular activity, while supporting flavour production. Food Microbiol 29:27–32
Carvalho AL, Turner DL, Fonseca LL, Solopova A, Catarino T, Kuipers OP, Voit EO, Neves AR, Santos H (2013) Metabolic and transcriptional analysis of acid stress in Lactococcus lactis, with a focus on the kinetics of lactic acid pools. PloS ONE 8(7):e68470
Chapot-Chartier MP, Kulakauskas S (2014) Cell wall structure and function in lactic acid bacteria. Microb Cell Factories 13[Suppl 1]:S9
Chen J, Shen J, Solem C, Jensen PR (2013) Oxidative stress at high temperatures in Lactococcus lactis due to an insufficient supply of riboflavin. Appl Environ Microbiol 79:6140–6147
Cretenet M, Le Gall G, Wegmann U, Even S, Shearman C, Stentz R, Jeanson S (2014) Early adaptation to oxygen is key to the industrially important traits of Lactococcus lactis ssp. cremoris during milk fermentation. BMC Genomics 15:1054–1068
Dijkstra AR, Alkema W, Starrenburg MJ, Hugenholtz J, van Hijum SA, Bron PA (2014) Fermentation-induced variation in heat and oxidative stress phenotypes of Lactococcus lactis MG1363 reveals transcriptome signatures for robustness. Microb Cell Factories 13:1–11
Dreissen AJM, Van Leeuwen C, Konings WN (1989) Transport of basic amino acids by membrane vesicles of Lactococcus lactis. J Bacteriol 171:1453–1458
Dressaire C, Redon E, Milhem H, Besse P, Loubiere P, Cocaign-Bousquet M (2008) Growth rate regulated genes and their wide involvement in the Lactococcus lactis stress responses. BMC Genomics 9:343
Dressaire C, Redon E, Gitton C, Loubière P, Monnet V, Cocaign-Bousquet M (2011) Investigation of the adaptation of Lactococcus lactis to isoleucine starvation integrating dynamic transcriptome and proteome information. Microb Cell Factories 10[Suppl 1]:S18
Fernández M, Zúñiga M (2006) Amino acid catabolic pathways of lactic acid bacteria. Crit Rev Microbiol 32:155–183
Ferreira MT, Manso AS, Gaspar P, Pinho MG, Neves AR (2013) Effect of oxygen on glucose metabolism: Utilization of lactate in Staphylococcus aureus as revealed by in vivo NMR studies. PLoS One 8(3):e58277
Flahaut NAL, Wiersma A, van de Bunt B, Martens DE, Schaap PJ, Sijtsma L, Dos Santos VAM, de Vos WM (2013) Genome-scale metabolic model for Lactococcus lactis MG1363 and its application to the analysis of flavor formation. Appl Microbiol Biotechnol 97:8729–8739
García-Cayuela T, Gómez de Cadiñanos LP, Peláez C, Requena T (2012) Expression in Lactococcus lactis of functional genes related to amino acid catabolism and cheese aroma formation is influenced by branched chain amino acids. Int J Food Microbiol 159:207–213
Guédon E, Sperandio B, Pons N, Ehrlich SD, Renault P (2005) Overall control of nitrogen metabolism in Lactococcus lactis by CodY, and possible models for CodY regulation in Firmicutes. Microbiology 151:3895–3909
Hols P, Kleerebezem M, Schanck AN, Ferain T, Hugenholtz J, Delcour J, de Vos WM (1999) Conversion of Lactococcus lactis from homolactic to homoalanine fermentation through metabolic engineering. Nat Biotechnol 17:588–592
Ibrahim SB, Rahman NA, Mohamad R, Rahim RA (2010) Effects of agitation speed , temperature , carbon and nitrogen sources on the growth of recombinant Lactococcus lactis NZ9000 carrying domain 1 of aerolysin gene. Afr J Biotechnol 9:5392–5398
Jong A de, Hansen ME, Kuipers OP, Kilstrup M, Kok J (2013) The transcriptional and gene regulatory network of Lactococcus lactis MG1363 during growth in milk. PLoS One 8(1):e53085
Lahtvee PJ, Adamberg K, Arike L, Nahku R, Aller K, Vilu R (2011) Multi-omics approach to study the growth efficiency and amino acid metabolism in Lactococcus lactis at various specific growth rates. Microb Cell Factories 10:12
Miyoshi A, Rochat T, Gratadoux JJ, Le Loir Y, Oliveira SC, Langella P, Azevedo V (2003) Oxidative stress in Lactococcus lactis. Genet Mol Res 2:348–359
Niel EWJ van, Hahn-Hagerdal B (1999) Nutrient requirements of lactococci in defined growth media. Appl Mirobiol Biotechnol 52:617–627
Nordkvist M, Jensen NBS, Villadsen J (2003) Glucose metabolism in Lactococcus lactis MG1363 under different aeration conditions : requirement of acetate to sustain growth under microaerobic conditions. Appl Environ Microbiol 69:3462–3468
Oliveira AP, Nielsen J, Förster J (2005) Modeling Lactococcus lactis using a genome-scale flux model. BMC Microbiol 5:39
Papagianni M (2012) Metabolic engineering of lactic acid bacteria for the production of industrially important compounds. Comput Struct Biotechnol J 3:1–8
Pedersen MB, Gaudu P, Lechardeur D, Petit MA, Gruss A (2012) Aerobic respiration metabolism in lactic acid bacteria and uses in biotechnology. Annu Rev Food Sci Technol 3:37–58
Price CE, Zeyniyev A, Kuipers OP, Kok J (2012) From meadows to milk to mucosa—adaptation of Streptococcus and Lactococcus species to their nutritional environments. FEMS Microbiol Rev 36:949–971
Smart KF, Aggio RBM, Van Houtte JR, Villas-Bôas SG (2010) Analytical platform for metabolome analysis of microbial cells using methyl chloroformate derivatization followed by gas chromatography–mass spectrometry. Nat Protoc 5:1709–1729
Szyperski T (1995) Biosynthetically directed fractional 13C-labeling of proteinogenic amino acids. An efficient analytical tool to investigate intermediary metabolism. Eur J Biochem 232:433–448
van de Bunt B, Bron PA, Sijtsma L, de Vos WM, Hugenholtz J (2014) Use of non-growing Lactococcus lactis cell suspensions for production of volatile metabolites with direct relevance for flavour formation during dairy fermentations. Microb Cell Factories 13:176
Villas-Bôas SG, Noel S, Lane GA, Attwood G, Cookson A (2006) Extracellular metabolomics: a metabolic footprinting approach to assess fiber degradation in complex media. Anal Biochem 349:297–305
Wegmann U, O’Connell-Motherway M, Zomer A, Buist G, Shearman C, Canchaya C, Ventura M, Goesmann A, Gasson MJ, Kuipers OP, Van Sinderen D, Kok J (2007) Complete genome sequence of the prototype lactic acid bacterium Lactococcus lactis subsp. cremoris MG1363. J Bacteriol 189:3256–3270
Zamboni N, Fendt SM, Rühl M, Sauer U (2009) 13C-based metabolic flux analysis. Nat Protoc 4:878–892
Acknowledgments
This research was funded by the Ministry of Science, Technology and Innovation Malaysia (MOSTI) under ScienceFund grant (02-01-02-SF0987).
Author information
Authors and Affiliations
Corresponding author
Electronic supplementary material
ESM 1
(DOCX 70 kb)
Rights and permissions
About this article
Cite this article
Sharif, S., Azizan, K.A. & Baharum, S.N. GC-MS based metabolomics analysis reveals the effects of different agitation speeds on the level of proteinogenic amino acids in Lactococcus lactis subsp. cremoris MG1363. Ann Microbiol 67, 383–389 (2017). https://doi.org/10.1007/s13213-017-1268-0
Received:
Accepted:
Published:
Issue Date:
DOI: https://doi.org/10.1007/s13213-017-1268-0