Abstract
This paper aims to identify and analyze critical success factors (CSFs) of Lean Six Sigma (LSS) implementation in context to Industry 4.0 (I4.0) in Indian manufacturing industries. Twenty CSFs are identified from literature and expert’s opinion. A survey was conducted through administration of designed questionnaire in Indian manufacturing industries and reliability of the factors was tested calculating Cronbach’s alfa (α) value for all responses. Thereafter, out of twenty CSFs, sixteen were found reliable. Further, these sixteen factors were analyzed employing Interpretive Structural Modeling (ISM) technique and leveled as per developed model. The MICMAC analysis is employed for determining driving and dependence power of CSFs. The developed model provides a platform for the practitioners/researchers to design a framework for successful implementation of LSS in view of current manufacturing paradigm of I4.0. On analyzing the data using ISM technique, the ‘Organizational culture and belief’, ‘Effective top management commitment and attitude’ and ‘Motivated and skilled manpower’ are observed to be the most significant CSFs which drive the path for proper implementation of LSS in Indian manufacturing industries. The developed model will enable the practitioners to draw the effective strategy for proper implementation of LSS in view of Industry 4.0. The results will give an edge to the management to think strategically for improvements in this competitive environment.
Similar content being viewed by others
Explore related subjects
Discover the latest articles, news and stories from top researchers in related subjects.Avoid common mistakes on your manuscript.
1 Introduction
Manufacturing scenario of twenty-first century is described by customized products and it requires complex production planning and control (Bhamu and Sangwan 2014). Manufacturing industries in India are the major drivers to GDP of the country. The ‘Make In India’ and ‘Digital India’ are the major initiatives started by Indian government to propel the growth in manufacturing sectors. These campaigns had been started by the government to transform the country as world class manufacturing hub. It is need of the hour to map current approaches of quality improvements with advanced manufacturing practices (Dewangan et al. 2015). Hence, to meet the dynamic demands of the customers, manufacturers are examining different competitive weapons as quality improvement methods. Lean Six Sigma is business strategy used for eliminating non-value-added operations and deviations in a process through statistical analysis to enhance organizational effectiveness (Thomas et al. 2016). Current manufacturing scenario is shifting towards customized from mass production, and the industries have to accept digitization and intelligentization in their operations (Vaidya et al. 2018). The Indian manufacturing organization also need to plan strategies for implementing Industry 4.0 concepts in existing lean operations to map with customization (Chiarini and Kumar 2020).
Effective digitalization of Lean practices such as jidoka, value stream mapping, poka yoke etc., can propose active track to the existing industrial values and the keys offered by Industry 4.0, can remove the prevailing fences in application of the Lean practices. Six sigma is data driven methodology that can be fused effectively with I4.0 technologies to achieve better results in processes (Zwetsloot et al. 2018). An I4.0 technology involves business process automation and has critical importance during decision makings. Panigrahi et al. (2022) explored the interrelationship between inventory automation practices and productivity in three steel manufacturing firms in India using structural equation modelling. The alignment of LSS with I4.0 real-time data techniques empowers the improvement throughout and ease the lessening of complications in an organization’s products and processes while revealing the full potential (Shamsi and Alam 2018). Manufacturing organizations can get big heights by integrating I4.0 technologies and Lean Six Sigma tools (Chiarini and Kumar 2020). Industry 4.0 technologies are major drivers for the companies to help in customized production with on time delivery of the products. Industry 4.0 enhances product quality and profitability (Machado et al. 2019). Smart Advanced Manufacturing and Rapid Transformation Hub (SMARTH)-Udyog Bharat 4.0 had been launched by the Indian government under Ministry of Heavy Industry and Public Enterprises to achieve the target of smart and intelligent manufacturing. The major vision of this program is to incorporate Industry 4.0 concept in Indian manufacturing by 2025. But numerous challenges have confronted by Indian manufacturing industries that create hindrances in implementation of existing quality and productivity tools with this new paradigm. Singh et al. (2024) uncovered the barriers to enterprise agility in insurance industry employing TISM and their interrelationships by MICMAC analysis. Panigrahi et al. (2023) elaborated the effects of agility in supply chain on operations performance measurement systems in Indian manufacturing firm using PLS-SEM approach. Yadav et al. (2020) mentioned that LSS and I4.0 is now available to all the industries of almost in all the countries, but their adoption and implementation will differentiate their performance and will be a key factor for improving competitiveness. Muller et al. (2018) discussed that strategic, operational, as well as environmental and social opportunities are positive success factors of I4.0, whereas Nafchi and Mohelska (2020) mentioned that culture, size and type of organization affects the implementation.
Though, many authors have reported critical success factors of LSS (Yadav and Desai 2017; Lande et al. 2016; Naslund 2013; Manville et al. 2012) and I4.0 (Luthra et al. 2020; Devi et al. 2020; Moeuf et al. 2019) individually/separately for various types of industries, but research on CSFs of Lean Six Sigma in perspective of I4.0 is hardly portrayed or at its infancy. This integration approach will be helpful for many manufacturing companies by adopting strategies for two concepts of operational excellence initiatives at combined level while avoiding separate planning in isolation or at individual level. Therefore an attempt is made to undertake a study to explore a set of CSFs and establish a connection among them for Indian manufacturing industries.
To derive model of CSFs different practices have been adopted by various researchers; moreover, the selection of the practice is subject to the feasibility, suitability, effectiveness, desired form of consequence required by the practitioners. Interpretive Structural Modeling (ISM), Analytic Network Process (ANP) and Analytical Hierarchy Process (AHP) are the popular mutli-criteria decision making techniques (Drohomeretski et al. 2014).
The basic objectives of conducting this study are:
-
To identify the critical success factors to Lean Six Sigma implementation in Indian Manufacturing industries in perspective to Industry 4.0.
-
To portray levels of partitions and the hierarchical model to establish contextual relationships among selected factors.
-
To categorize CSFs on the basis of driving-dependency power into four constructs.
The study focuses to excogitate the prominent CSFs of LSS and how they influence the effective implementation of LSS in I4.0 systems. The outcomes of this study will definitely help practitioners of Indian manufacturing industries to plan the strategies of right implementation of LSS in view of current manufacturing scenario.
2 Literature review
The papers from recognized journals selected using keyword ‘critical success factors for LSS’, ‘Enablers for LSS implementation’, ‘success factors of Industry 4.0’, ‘LSS critical factor for implementation in manufacturing industries.
As shown in Fig. 1, PRISMA method of systematic literature review has been followed, the authors have searched papers from available databases i.e. Scopus, google scholar, science-direct, Web of Science etc. and total 124 articles were identified at initial stage, after initial examination, 23 articles out of 124, were omitted which do not exhibited relevant information regarding the designated refrain of critical success factors. After the screening stage, the articles showing the interlink or interdependency between LSS and Industry 4.0, were more focused for study so that major finding can be extracted from the pool. 49 articles were included for the further consideration that showed interlinking between LSS and Industry 4.0 and finally, 20 critical success factors were extracted from the screened articles.
2.1 Integration of LSS and industry 4.0
Now a days, the complexity of data volumes is the major challenge before LSS practitioners and this can be diminished by integrating I4.0 technologies with DMAIC projects (Tanawadee et al. 2023). The Lean six sigma methodologies will not become outdated but will get more values with advance technologies of fourth generation of manufacturing to align the companies with competitiveness (Skalli et al. 2022). Manufacturing firms are focusing to increase their operational performance and to achieve excellence, lean practices are integrated with advanced technologies that have contributed a lot in this direction in developing countries like India (Swarnakar et al. 2020; Yadav et al. 2020). The policies and initiatives taken by the Government will boost the manufacturing sector in India and help them to become a preferred site in international manufacturing arena around the world. In manufacturing segments, the firms require to alter their production facilities, quality improvement techniques as per present emerging drifts in these areas. Lean and six sigma end results can be attained in more significant means using practices of new cutting-edge technologies i.e. Industry 4.0 and Internet of Things. The said approach generated a lot of opportunities to remain competitive for their survival. Lean, six sigma and Industry 4.0 needs to be integrated to achieve full manufacturing potential and operational excellence to face global competition (Yadav et al. 2020).
Kamble et al. (2020) has examined the effect of I4.0 and lean practices in Indian manufacturing industries through survey at Mumbai, Pune, Bangalore, and Chennai and has observed that it leads to enhancement of sustainable organisational performance. They also observed that implementation of I4.0 technologies are still in premature stage, but slowly getting momentum. Rossin et al. (2019) studied the influence of I4.0 and lean manufacturing to achieve operational excellence through survey of 108 European manufacturers. Similarly, Chiarini and Kumar (2020) also mentioned LSS can provide foundation for to achieve effective outcome/maximizing the impact of I4.0 technologies by interviewing manufacturing managers in in ten Brazilian manufacturing case organizations in which integrated approach of LSS and I4.0 was used. Bhat et al. (2020) has leveraged LSS for I4.0 to the process industries, and realized an annual savings of around 20% in production and overhead costs, which shows that LSS can be applied in the advent of I4.0. Yadav et al. (2018) applied hybrid framework to facilitate lean six sigma implementation in a manufacturing industry but they limit their study up to LSS only. A new methodology of LSS 4.0 was introduced by Arcidiacono and Pieroni (2018), and has proved its efficiency when applied to the healthcare sector. This methodology is able to optimise the services supply process, reduced (human and/or material wastage and improved the Quality of Experience (QoE) of the patients. In existing literature there are various researchers who had explored different CSFs of LSS and I4.0 as standalone. In this study, extent literature review has been carried out for identification of LSS CSFs in perspective to I4.0 in Indian manufacturing industries to ensure the better performance outputs while implementing the concepts of LSS and I4.0. From the review, it is observed that in existing research (Thomas et al. 2016; Andersson et al. 2014; Luthra et al. 2020), CSFs of LSS and I4.0 are portrayed as standalone but there is no such study has been carried out which can explore these factors fusing with I4.0 technologies in context of Indian manufacturing as such. Therefore, the present study covers CSFs by taking effects of I4.0 technologies on LSS implementation in Indian context.
2.2 Critical success factors
‘Critical Success Factor’ is termed as most significant enabler to attain organizational objectives for effective quality management and performance (Psomas 2016; Chiarini 2013; Lande et al. 2016). Yadav and Desai (2017) identified twelve enablers through literature review and expert’s opinion, and applied ISM-Fuzzy MICMAC for analyzing them. Abu Bakar et al. (2015) reviewed critical success factors of LSS deployment and revealed that integrated approaches of Lean and Six Sigma are less portrayed yet. Brun (2011) conducted survey and interviews to identify CSFs for Six Sigma implementation in Italian companies. Various authors have studied LSS success factors in context to manufacturing (Kashyap et al. 2023; Thomas et al. 2016; Andersson et al. 2014), service (Antony et al. 2014; Nicoletti and Vergata 2013) and healthcare sectors (Rathi et al. 2023; Gijo and Antony 2014). Luthra et al. (2020) applied ISM for structuring the CSFs for Industry 4.0. Lande et al. (2016) identified CSFs of LSS implementation in Indian SME’S through exploratory study. Zhang et al. (2019) adopted Fuzzy-DEMATEL for analysis of Industry 4.0 enablers. Soti et al. (2010) developed a model of LSS enabler using ISM-MICMAC technique in Indian industries.
Table 1 depicts analysis of extensive work on LSS and I4.0 critical success factors or enablers. From analysis it has observed that the authors (Devi et al. 2020;Yadav and Desai 2017; Kamble et al. 2018) have applied ISM-MICMAC approach for modeling of CSFs of LSS or Industry 4.0 separately without considering interlinking between two. While Rathi et al. (2023) have applied ISM-MICMAC in developing a model of success factors employing green LSS in Indian healthcare facility Lande et al. (2016) and Moeuf et al. (2019) described LSS CSFs in context of SMEs. Lin et al. (2018) used ANNOVA for analysis of I4.0 CSFs with reference to automotive sector. Kashyap et al. (2023) formed a structured model of CSFs for implementation of lean Industry 4.0 in manufacturing supply chain. Machado et al. (2019) elaborated I4.0 enablers through interview and a case study in manufacturing company. Therefore, from Table 1, it is clear that the authors have analyzed LSS and I4.0 critical success factors using different modeling techniques in context of various domains but rarely have studied these in integrated forms in perspective to Industry 4.0. Hence, there is still scope to analyze LSS critical factors combined with present manufacturing shift.
Table 2 represents 20 CSFs extracted from comprehensive literature search on LSS implementation in view of Industry 4.0. These factors are reflecting fusion of LSS with I4.0.
3 Methodology
The procedure carried out in conducting current study is represented in Fig. 2. In this paper, empirical research with the questionnaire method is carried out to identify the factors for LSS in context of Industry 4.0.
The study is oriented towards identification of LSS CSFs and their contextual relationships with I4.0. As current manufacturing sector, is demanding changes towards customized production and for that the companies need to align existing quality programs with cutting edge quality improvement techniques. The identified CSF for application in Indian manufacturing industries is mentioned in Table 2. The findings may help out the policy makers and management by providing an insight in developing strategies for integrated approach of LSS and I4.0 for sustainable development in India.
The suitability of selected CSFs with respect to Indian manufacturing companies was verified after discussion with eight experts. Who have vast experience in manufacturing domain. Table 3 shows details about experts including their experiences and expertise. All of the experts have working at senior most positions with the experiences in LSS, Industry 4.0.
One expert was quality manager from automobile manufacturing industry having adequate skills in six sigma projects and second expert was from the field of project management working with aerodynamic industry. Third expert was a consultant from recognized firm and having more than 17 years of experience in providing Six Sigma black belt and green belt training to industry professionals. Fourth expert was production manager at reputed fastener manufacturing company having expertise in LSS as well as in Industry 4.0, and fifth and sixth one belonged to academic background from recognized technical university having research experience in LSS, I4.0 and Life Cycle Assessment. Seventh expert was head of Research and Development cell in manufacturing company and last one was quality manager at project based Indian industry. Eighth expert is quality control manager in manufacturing firm having 12 years of experience in working on Industry 4.0 techniques implementation. All the experts were consulted sharing the list of all twenty CSFs identified from literature search and requested to give inputs to improve the formulation of survey questionnaire to ensure effective of data collected during the responses. The experts were requested to check identified CSFs in context of Indian manufacturing industries and give their amendments without considering any biasness. The list was reviewed as per their suggestions. The experts from industry suggested some modifications in the name of CSFs and these were incorporated to finalize the list.
3.1 Design of survey questionnaire and data collection
A survey questionnaire was developed after incorporating the inputs from the experts. The questionnaire was designed to know the current position of LSS employment in view of I4.0 in manufacturing companies of India. To get the response from the companies, each question of the questionnaire to be rated on 5-point Likert scale where 1 stands for ‘Not critical’, 2 for ‘Less critical’, 3 signifies ‘Critical’, 4 indicates ‘Most critical’ and 5 means ‘Extremely critical’. The survey questionnaire was sent to 137 industries of manufacturing sector. In duration of 08 months, after making straight efforts, total 61 responses were got. Out of 61 replies, 04 responses were not considered as they were not filled with complete information and seem not relevant to research. Further investigation concludes that out of 57, 44 were found appropriate and comprehensive for the study. It was noticed that response rate was observed 41.60% and the respondents from the companies, were having vast experiences in LSS and I4.0.
3.2 Testing reliability of survey data
Prior to the development of hierarchical model employing ISM, reliability test was carried out using SPSS 25.0. Cronbach’s alpha (α) and item-total correlations or corrected item-total correlations (CITC) were estimated after feeding responses to SPSS 25.0. The values of Cronbach’s alpha (α) and CITC were compared with threshold levels to determine the consistency and reliability of survey responses (As shown in Table 4).
According to Black and Porter (1996), the items having Cronbach’s alpha (α) value above 0.7, are consistent and reliable. If CITC value exceeds 0.3, signifies association of an item with the compound score of all the objects appearing the same set (Churchill and Iacobucci 2002). In this test, four CSFs (highlighted in Table 4) were found having CITC value less than 0.3 (highlighted in bold), means these items (CSF5- HRM policies and reward & recognition system, CSF9- Mechanism for projects tracking & review, CSF10- Appropriate project team selection and CSF18- Work procedures standardization) were not showing the similar construct with all other 16 items. Therefore, four CSFs were removed from former identified CSFs in Table 2. Finally, total 16 CSFs (revised codes C1 to C16) were screened for further study and analysis (shown in Table 5).
4 Interpretive structural modeling and MICMAC analysis
ISM is well-known interactive technique used to establish contextual relationship between complex of item or key issues (Yadav and Desai 2017; Mohammed et al. 2008). ISM proposed by Warfield (1974) and it is an interpretive method as contextual relationships between the items affecting the system under consideration is based judgments collected from experts. There are different MCDM techniques found in literature i.e. DEMATEL, VIKOR, AHP, ANP, TOPSIS, etc. However, these practices have limited room to develop structural model for the parameters in perspective of the demand of the industries to execute new policies for quality (Yadav and Desai 2016) but the use of these techniques depends upon the suitability of applications. ISM has the capability to discover better solution for the above said problem. This technique enables researchers and practitioners to map better relationship among key items in complex situations. To classify the selected parameters into four different clusters, the researchers have smashed ISM with MICMAC analysis to achieve uttermost results which shows independencies on each other parameter (Tripathy et al. 2013).
4.1 Stepwise procedure to interpretive structural modeling
Interpretive Structural Modeling is adopted to establish contextual mapping among the factors those are finally screened as CSFs (shown in Table 5). A comprehensive model of selected factors is structured using ISM analysis. Many authors have used ISM-MICMAC analysis in their work (Yadav and Desai 2017; Luthra et al. 2020; Devi et al. 2020).
ISM comprised of following stages in development of model;
-
In initial phase, factors were identified from existing literature and the same were verified from experts group. Reliability of selected factors was also checked.
-
In the next stage, pairwise relationship is developed with the opinion of selected experts. It leads development of SSIM. Thereafter, Initial Reachability Matric (IRM) was formatted.
-
Transitivity analysis was performed in IRM and Final Reachability Matric (FRM) was formed. Later, factors were classified into reachability; antecedent and intersection sets and using these sets, level partitions were developed.
-
According to level partitions, ISM model was developed.
5 Results
5.1 Formation of SSIM
Experts from industry as well as academics were consulted to provide their inputs for identifying the connection between two factors or CSFs (i and j) and directions of connection.
The experts also have validated the identified factors. V, A, X and O are four signs which are used for indicating different relationships.
[V]—Factor i will influence factor j
[A]—Factor j will influence factor i
[X]—Factor i and j will influence each other
[O]—Factor i and j are unrelated or will not influence each other
Based on connections between factors and its directions, SSIM is formed by assigning symbols shown in Table 6.
5.2 Construction of IRM
To get IRM, four alphabetic symbols (V, A, X and O) are transformed into binary digits 0 and 1 according to certain rules.
-
If (i, j) entry in SSIM is ‘V’ then ‘1’ is assigned in IRM and it will be assigned as ‘0’ at corresponding (j, i) entry.
-
If (i, j) entry in SSIM is ‘A’ then ‘0’ is assigned while at (j, i) entry, ‘1’ is assigned.
-
If (i, j) entry is ‘X’ in SSIM then place (i, j) and (j, i) both as ‘1’.
-
If (i, j) entry is ‘O’ in SSIM then put (i, j) and (j, i) both entries as ‘0’.
On the basis of mentioned rules, IRM is constructed as shown in Table 7.
5.3 Construction of final reachability matrix (FRM)
Final Reachability Matrix Transitivity is formed after performing transitivity analysis in IRM. Transitivity denotes that if a factor ‘x’ effects ‘y’ and factor ‘y’ is effecting further another factor’z’ then it’s clear that factor ‘x’ will certainly effect factor ‘z’. As per this rule FRM matrix (*denotes transitivity) is deduced as shown in Table 8, whereas highlighted value shows driving power, dependency power and ranks.
5.4 Panelizing the levels
Reachability, antecedent and interaction sets are three main arrays to derive level partitions. Intersection sets are deduced taking common factors out of reachability and antecedent sets. The reachability set contains the CSF and others those are driven by it. On other way, antecedent set has CSF and others those on which it depends. The row having common factors in reachability and interaction sets (highlighted in bold) is assigned first level. In the next iteration, the CSFs assigned first level will be discarded from process and other levels are assigned following the same procedure. The identified levels (highlighted in bold) are shown in Tables 9, 10, 11, 12 and 13.
5.5 ISM model formation
ISM model is derived according the levels assigned in level partitioning process in Sects. 5.4. Five levels were assigned to the factors and accordingly model was structured as shown in Fig. 3. It is clear from Fig. 3 that ‘Organizational culture & belief (C1)’, ‘Effective top management commitment & attitude (C5)’, and ‘Motivated and skilled manpower (C10)’ are the most significant CSFs to LSS implementation in perspective to I4.0 in manufacturing organizations. The successful implementation will be possible with the support of top management and skilled manpower. ‘Organizational culture and belief’ is the major CSF in proper adoption of quality improvement methods.
It is evident from the model that ‘Comprehensive training and education for employees (C3)’, ‘Effective leadership & advisory committee (C9)’ and ‘Readiness to change (C12)’ are the three CSFs which will be influenced by ‘Organizational culture & belief (C1)’, ‘Effective top management commitment & attitude (C5)’, and ‘Motivated and skilled manpower (C10)’. ‘Comprehensive training and education for employees (C3)’, ‘Effective leadership & advisory committee (C9)’ and ‘Readiness to change (C12)’ may lead to ‘Enhance supply chain coordination (C2)’ and ‘Effective communication at all levels (C13)’. These two CSFs further driving ‘Linking LSS/I4.0 with business strategy/goals, supplier and customer (C7)’, ‘Precise selection of ‘LSS/smart manufacturing tools/ I4.0 technologies (C8)’ and ‘Resources availability/utilization (C14)’ as these all three CSFs occupy the same level in model and finally, the authors drive ‘Linking LSS with smart technologies/I4.0 industry paradigm (C4)’, ‘Appropriate bench marking system (C6)’, ‘Data collection, measurement & analysis tools (11)’, ‘Updating of technological advancement (C15)’ and ‘Adoption of Continuous Improvement (CI) philosophy (C16)’. These five CSFs are positioned at top level of the ISM model and may be driven by other eleven CSFs.
5.6 MICMAC analysis
Driving power of a CSF is calculated after adding all 1’s in corresponding row while dependency power is addition of all 1’s in corresponding column in final reachability matrix. These driving and dependency powers are used for classifying CSFs into four constructs. The driving power of a factor depicts that how many factors are driven by particular CSF i.e. in Table 8, CSF1 is having sum of 1 s in row 16; it means the CSF1 drives or influences all other CSFs. The dependence power of a CSF justifies that the CSF depends on how many other CSFs i.e. in Table 8, CSF1 is depending on 9 other CSFs. However, MICMAC analysis categorizes the CSFs in four clusters, i.e. autonomous, dependent, linkage and driving CSFs. Figure 4 is derived from driving and dependency power of CSFs.
-
The first cluster or lower left quadrant contains ‘autonomous CSFs’ having weak dependence and driving power. No such CSF is there in current analysis. All the factors influencing each other and no such factor which shows independency. Therefore, there is no any CSF in first quadrant
-
The second cluster or lower right quadrant indicates ‘dependent CSFs’ which shows weak driving, but strong dependence power. As per Fig. 4, two CSFs i.e. ‘LSS/smart manufacturing tools/ I4.0 technologies (C8)’ and ‘Data collection, measurement & analysis tools (C11)’ fall under this category. It is obvious that effectiveness of data collection and measurement depend on LSS/smart manufacturing tools/I4.0 technologies. Hence, both the factors showing in second quadrant.
-
The third cluster or upper right quadrant signifies ‘linkage CSFs’ having high driving and dependence power both. There are eleven CSFs fall in this quadrant i.e. ‘Organizational culture & belief (C1)’, ‘Enhance supply chain coordination (C2)’, Comprehensive training and education for employees (C3)’, ‘Linking LSS with smart technologies/I4.0 industry paradigm (C4)’, ‘Appropriate bench marking system (C6)’, ‘Linking LSS/I4.0 with business strategy/goals, supplier and customer (C7)’, ‘Effective leadership & advisory committee (C9)’, ‘Readiness to change (C12)’, ‘Effective communication at all levels (C13)’, ‘Updating technological advancement (C15)’ and ‘Adoption of Continuous Improvement (CI) philosophy (C16)’. All these are linked to each other. The factors falling in this quadrant have the capability to drive and depend on each other and implementation of one will influence the way to implement other.
-
The fourth cluster or upper left quadrant contains ‘driving CSFs’ having strong driving power but weak dependence power. In this cluster two CSFs fall i.e. ‘Effective top management commitment & attitude (C5)’, and ‘Motivated and skilled manpower (C10)’. These two factors are having adequate capability to drive other factors. That’s why falling in this cluster.
-
‘Resources availability/utilization (C14)’ is only one CSF which comes between ‘linkage CSFs’ and ‘dependent CSFs’ types of cluster. Resource availability is the major factor which is linked with the success of others.
-
In this analysis, no any factor falls in category of ‘autonomous CSFs’ as all the factors are influencing each other and all have importance on their place with respect to other.
-
This analysis helps us to understand the extent of level of interaction of these critical success factors within Indian Manufacturing industries.
6 Discussion
The analysis and ranking of critical factors for successful implementation of quality improvement methods in context to current shifting of manufacturing is major concern for establishing the firm in competitive market. Complexity and increasing volume of data are the challenges faced by LSS practitioners. These challenges can be mitigated by mapping and supporting DMAIC phases of six sigma method with I4.0 technologies (Tanawadee et al. 2023).
In the past, several studies have covered CSFs of LSS and Industry 4.0 standalone. Authors have identified CSFs from literatures and expert’s opinions but, have not mentioned about reliability test for final screening of the factors before applying modeling techniques (Bag et al. 2018; Yadav and Desai 2017; Mittal et al. 2019; Machado et al. 2019).
In the present work, twenty CSFs were identified from existing literature and finalized for Indian manufacturing context after discussion with experts, further checking the consistency using reliability test. The reliability test results revealed a good consistency with Cronbach’s alpha value greater than 0.8. ISM model is developed to establish the contextual relationship among the CSFs.
The ISM model shows that ‘organizational culture and belief (C1)’, ‘effective top management commitment and attitudes (C2)’, and ‘motivated and skilled manpower (C10)’ are the most significant success factors positioning at bottom in constructed model. The result also maps with the study carried out by Samanta et al. (2023), the authors explored top management commitment as topmost critical success factor regarding integration of LSS to Industry 4.0 for attainment of organizational excellence in context of manufacturing industries. The successful adoption of any quality improvement strategy depends on existing culture and belief in the organization (Moeuf et al. 2019) and positive attitude of the top management (Devi et al. 2020). Also, the manpower must be skilled and motivated in emerging technologies. Therefore, the success of LSS implementation in view of Industry 4.0 will be realized by innovating and learning culture inbuilt in the organization having clear vision. The top management has to ensure bright environment of continuous improvement by providing required facilities for accepting competitive quality improvement methodologies. This attitude of the management will create transformation culture within the employees and reinforce their self-confidence with mitigation of fear failure. C1, C2 and C10 are the common CSFs and these have much importance for successful implementation of any initiative. Organization’s culture, commitments from top management side and skilled manpower all three factors have vital role for right adoption of new strategies in manufacturing as well as in other sectors. Indian manufacturing industries need to work for developing smart culture to skill their manpower as according to current manufacturing changes.
The model revealed that ‘comprehensive training and education for employees (C3)’, ‘effective leadership and advisory committee (C9)’ and ‘resistance to change (C12)’ are also the major influencing factors in implementation of LSS in perspective Industry 4.0. Indian manufacturing organization need to provide adequate training for employees to enrich their skills about advanced technologies required to stand with current competitive scenario (Devi et al. 2020; Mittal et al. 2019). As the LSS is data driven methodology relying on analytical tools, therefore, the employees must be trained in advanced big data analytics techniques. The leadership and advisory committee of the company should comprise technical person having vast knowledge about current trends in manufacturing so that they can guide the employees towards right path (Zhang et al. 2019). These practices will build the confidence in the employees and they will always be ready to adopt new things. ‘Enhance supply chain coordination (C2)’, and ‘effective communication at all levels (C13)’ contribute in proper implementation of LSS with advanced technologies. The organization has to develop strong relationship among different supply chain partners and remove all the barriers of good communication between inter-departments within as well as outside of the company (Thomas et al. 2016; Machado et al. 2019; Mittal et al. 2019).
The other factors as ‘linking LSS and Industry 4.0 with business strategy/goal, supplier, customers (C7)’, ‘precise selection of LSS and smart manufacturing tools and Industry 4.0 technologies (C8)’ and ‘resource availability and utilization (C14)’ are also responsible for successful implementation of LSS project in the manufacturing organization. The Industry 4.0 technologies i.e. IOT, enhance the power of LSS tools to analyze and validate the root causes of the pinpoints in more efficient and effective way (Skalli et al. 2022). The LSS projects must be aligned with business strategy or goal of the organization (Titmarsh et al. 2020; Yadav et al. 2020; Zwetsloot et al. 2018). The tools used in the projects must be selected precisely and the organization should ensure availability of required financial and non-financial resources with proper monitoring of utilization of these.
Further, ‘linking LSS with smart technologies/Industry 4.0 paradigm (C4)’, ‘appropriate benchmarking system (C6)’, ‘data collection, measurement and analysis tools (C11)’, ‘updating of technological advancement (C15)’ and ‘adoption of continuous improvement philosophy (C16)’ are also having important role in LSS implementation in look of Industry 4.0. There is ample need to link existing LSS system with current smart manufacturing system (Titmarsh et al. 2020) to get benefits from cutthroat market of dynamics demands. The organization has to decide appropriate benchmarking system. Engineers, managers and employees must be aware about advance tools of data collection, measurement and analysis. (Zwetsloot et al. 2018; Soti et al. 2010). Manufacturing industries have to ensure successful implementation of LSS with advanced data analytics to provide the products as per the voice of the customers.
For the realization of LSS implementation in context of Industry 4.0, the manufacturing industries need to give full concern to the above ranked critical factors and adopt potential practices in their facilities as a business strategy.
7 Theoretical and practical implications of the study
This study provides potential insights as theoretical outcomes for practitioners and researchers in the domain of LSS and Industry 4.0. Critical factors ranked through ISM approach were confirmed with the experts from manufacturing domain. The results of this will enhance the knowledge base about the factors responsible for successful implementation of LSS in context to Industry 4.0. The researchers will learn about sequential execution of ISM-MICMAC analysis approach applied for prioritization of the factors. Moreover, the analysis explores awareness about mapping of LSS approach with Industry 4.0 concepts. Further, theoretical base of the presented work will provide a strong platform to the practitioners regarding proper adoption of LSS practices with Industry 4.0 technologies in manufacturing organization and will open the path of transformation.
The outcomes of the work carried out provide better insights as practical implications for Indian manufacturing companies. The hierarchical model developed depicts a roadmap for the practitioners to implement better LSS practices in view of Industry 4.0. The results revealed that organizational culture, commitments of top management and skills of manpower are main responsible factors to be considered in drafting policies or business strategies to achieve competitiveness. The integration of LSS approach with Industry 4.0 practices will bring a new edge to existing manufacturing scenario and will develop capabilities to produce customized products to address dynamic demands of customers. The Government of India has launched schemes like ‘Make in India’, ‘Skill India’ and ‘Digital India’ to fuse Industry 4.0 concepts in the exiting manufacturing facilities. The Indian manufacturing industries need to facilitate adequate training to their employees to enhance technical knowledge about advanced manufacturing practices. This study also reveals that linking of LSS practices with smart tools will give big height to quality methods. The framework constructed in this study could be a base for judicious selection of LSS practices with I4.0 to get maximum benefits from integrating both the approaches.
In reference to practical facets, the present study had conducted by adopting proved precise method of modeling and experts from Indian industries have contacted for the inputs. Therefore, the study would help the manufactures to develop their strategic plan of implementing LSS with taking considerations of discussed success factors in current competitive market.
This study offers in-depth understandings of critical success factors and interdependencies of the factors using ISM technique that could create effective platform for practitioners to employ emerging technologies of Industry 4.0 with LSS implementation in more efficient way. In present study 16 key factors have been recognized and examined, in future studies; the researchers may include more potential enablers. The authors may use other effective analysis techniques to unlock more findings regarding this concept.
8 Conclusion
In present research work, the critical factors to successful implementation of LSS in context to Industry 4.0 in Indian manufacturing industries are explored and modeled using interpretative structure modeling process. The identification of CSFs was done through extent literature search and considering inputs from domain experts. Structured questionnaire based on identified CSFs were administered to Indian manufacturing industries for getting responses regarding these factors. The reliability test was carried out for checking consistency and final shortlisting of CSFs. These screened CSFs were analyzed and modeled applying ISM and outcomes showed that organization’s culture, top management commitment and skills manpower are the main factors influencing adequate adoption of LSS practices in view of Industry 4.0. It signifies that the culture of an organization and role of management reflect positive impacts on adoption of advanced technologies. Also, the organization must facilitate adequate training to the employees by arranging expert sessions regarding current digital technologies. Academic institutions must also include these new edge technologies in their curriculum so that proper fusion of these may take place in exiting manufacturing facilities. The interlinking of exiting quality improvement tools like LSS with advanced data analytics techniques would provide greater strength to these tools and would result more business performance. The development and analysis of CSFs in this study contributes in designing the proper strategies to map LSS with Industry 4.0 technologies for getting better benefits. The manufacturers can prioritize their plans to get the advantages of integrating LSS and Industry 4.0. The study provides a strong foundation for deciding the right path of successful adoption of LSS in context of Industry 4.0.
The study elaborated in this paper, is exploratory type and may contain biasness on the inputs provided by the judgment group. The factors analyzed in this study are derived for Indian manufacturing industries but this may be different in perspective to other countries or domain. In future, these factors can be derived for other industry sectors like healthcare, projects, electronics, service and engineering. The ISM methodology discussed in this study may be applied for ranking of other factors of different domain.
References
Abu Bakar FA, Subari K, Mohd Daril MA (2015) Critical success factors of Lean Six Sigma deployment: a current review. Int J Lean Six Sigma 6(4):339–348
Andersson R, Hilletofth P, Manfredsson P, Hilmola OP (2014) Lean Six Sigma strategy in telecom manufacturing. Ind Manag Data Syst 114(6):904–921
Antony J, Setijono D, Dahlgaard JJ (2014) lean six sigma and innovation—an exploratory study among UK organizations. Total Qual Manag Bus Excell 27:124–140
Assarlind M, Aaboen L (2013) Forces affecting one lean six sigma adoption process. Int J Lean Six Sigma 5(3):324–340
Bag S, Telukdarie A, Pretorius JHC, Gupta S (2018) Industry 4.0 and supply chain sustainability: framework and future research directions. Benchmarking Int J. https://doi.org/10.1108/BIJ-03-2018-056/full/html
Bertola P, Teunissen J (2018) Fashion 4.0. Innovating fashion industry through digital transformation. Res J Text Appar 22(4):352–369
Bhamu J, Sangwan KS (2014) Lean manufacturing: literature review and research issues. Int J Oper Prod Manag 34(7):876–940
Brun A (2011) Critical success factors of six sigma implementations in Italian companies. Int J Prod Econ 131(1):158–164
Chiarini A (2013) Differences between six sigma applications in manufacturing and the service industry. Int J Prod Qual Manag 12(3):345–360
Chiarini A, Kumar M (2020) Lean six sigma and industry 4.0 integration for operational excellence: evidence from Italian manufacturing companies. Prod Plan Control 32(13):1–18
Churchill G, Iacobucci D (2002) Marketing Research, Methodological Foundations, 8th edn. Harcourt Publishing, London
Devi KS, Paranitharan KP, Agniveesh AI (2021) Interpretive framework by analysing the enablers for implementation of Industry 4.0: an ISM approach. Total Qual Manag Bus Excell 32(13–14):1494–514
Dewangan DK, Agrawal R, Sharma V (2015) Enablers for competitiveness of indian manufacturing sector: an ISM-Fuzzy MICMAC analysis. Proc Soc Behav Sci 189:416–32
Drohomeretski E, Gouvea da Costa SE, Pinheiro de Lima E, da Garbuio PA (2014) Lean, six sigma and lean six sigma: an analysis based on operations strategy. Int J Prod Res 52(3):804–824
Franchetti M, Barnala P (2013) Lean six sigma at a material recovery facility: a case study. Int J Lean Six Sigma 4(3):251–264
Gijo EV, Antony J (2014) Reducing patient waiting time in outpatient department using lean six sigma methodology. Qual Reliab Eng Int 30(8):1481–1491
Gunasekharan S, Elangovan D, Parthiban P (2014) A Comprehensive study to evaluate the critical success factors affecting lean concept in Indian manufacturing industries. Appl Mech Mater 592:2569–2576
Jayaraman K, Kee TL, Soh KL (2012) The perceptions and perspectives of Lean Six Sigma (LSS) practitioners. TQM J 24(5):433–446
Kashyap A, Yadav AK, Vatsa ON, Chandaka TN, Shukla OJ (2023) Investigation of the critical success factors in the implementation of the lean industry 4.0 in manufacturing supply chain: an ISM approach. Manag Environ Qual 34(4):981–996. https://doi.org/10.1108/MEQ-04-2022-0109
Lande M, Shrivastava RL, Seth D (2016) Critical success factors for lean six sigma in SMEs (small and medium enterprises). TQM J 28(4):613–635
Lin D, Lee CKM, Lau H, Yang Y (2018) Strategic response to Industry 4.0: an empirical investigation on the Chinese automotive industry. Ind Manag Data Syst 118(3):589–605
Luthra S, Yadav G, Kumar A, Anosike A, Mangla SK, Garg D (2020) Study of key enablers of industry 4.0 practices implementation using ISM-Fuzzy MICMAC Approach, In: Proceedings of the International Conference on Industrial Engineering and Operations Management Dubai, UAE, March 10–12, 2020., IEOM Society International, pp. 241–251.
Machado CG, Winroth MP, Carlsson D, Almstrom P, Centerholt V, Hallin M (2019) Industry 4.0 readiness in manufacturing companies: challenges and enablers towards increased digitalization. Proc CIRP 81:1113–1118
Manville G, Greatbanks R, Krishnasamy R, Parker DW (2012) Critical success factors for Lean Six Sigma programmes: a view from middle management. Int J Qual Reliab Manag 29(1):7–20
Mittal S, Khan MA, Romero D, Wuest T (2019) Smart manufacturing : characteristics, technologies and enabling factors. J Eng Manuf Smart Manuf Digit Fact 233(5):1342–1361
Moeuf A, Lamouri S, Pellerin R, Tamayo-Giraldo S, Tobon-Valencia E, Eburdy R (2019) Identification of critical success factors, risks and opportunities of Industry 4.0 in SMEs. Int J Prod Res 58(5):1–17
Mohammed IR, Shankar R, Banwet DK (2008) Creating flex-lean-agile value chain by outsourcing: an ISM-based interventional roadmap. Bus Process Manag J 14(3):338–389
Naslund D (2013) Lean and six sigma—critical success factors revisited. Int J Qual Serv Sci 5(1):86–100
Nicoletti B, Vergata T (2013) Lean Six Sigma and digitize procurement. Int J Lean Six Sigma 4(2):184–203
Panigrahi RR, Jena D, Mishra PC (2022) Inventory automation practices and productivity: a study on steel manufacturing firms. Int J Appl Syst Stud 9(3):195–213
Panigrahi RR, Jena D, Meher JR, Shrivastava AK (2023) Assessing the impact of supply chain agility on operational performances-a PLS-SEM approach. Meas Bus Excell 27(1):1–24
Panizzolo R, Garengo P, Sharma ML, Gore A (2012) Lean manufacturing in developing countries: evidence from Indian SMEs. Prod Plan Control Manag Oper 23(10/11):769–788
Pongboonchai-Empl T, Antony J, Garza-Reyes JA, Komkowski T, Tortorella GL (2023) Integration of Industry 4.0 technologies into Lean Six Sigma DMAIC: a systematic review. Prod Plan Control. https://doi.org/10.1080/09537287.2023.2188496
Psomas E (2016) The underlying factorial structure and significance of the Six Sigma difficulties and critical success factors. TQM J 28(4):530–546
Rathi R, Kaswan MS, Antony J, Cross J, Garza-Reyes JA, Furterer SL (2023) Success factors for the adoption of green lean six sigma in healthcare facility: an ISM-MICMAC study. Int J Lean Six Sigma 14(4):864–897
Samanta M, Virmani N, Singh RK, Haque SN, Jamshed M (2023) Analysis of critical success factors for successful integration of lean six sigma and Industry 4.0 for organizational excellence. TQM J. https://doi.org/10.1108/TQM-07-2022-0215
Shamsi MA, Alam A (2018) Exploring lean six sigma implementation barriers in information technology industry. Int J Lean Six Sigma 9(4):523–542
Singh N, Panigrahi R, Panigrahi RR, Shrivastava AK (2024) An integrated total interpretive structural modeling and MICMAC model for uncovering enterprise agility barriers in the insurance industry. Decis Anal J 10(100421):1–11
Skalli D, Charkaouli A, Cherrafi A (2022) Assessing interactions between lean six-sigma, circular economy and industry 4.0: toward an integrated perspective. IFAC PapersOnLine 55(10):3112–3117
Snee RD (2010) Lean Six Sigma-getting better all the time. Int J Lean Six Sigma 1(1):9–29
Soti A, Shankar R, Kaushal OP (2010) Modeling the enablers of Six Sigma using interpreting structural modeling. J Model Manag 5(2):124–141
Swarnakar V, Tiwari AK, Singh AR (2020) Evaluating critical failure factors for implementing sustainable lean six sigma framework in manufacturing organization: a case experience. Int J Lean Six Sigma 11(6):1069–1104
Thomas AJ, Francis M, Fisher R, Byard P (2016) Implementing Lean Six Sigma to overcome the production challenges in an aerospace company. Prod Plan Control 27(7/8):591–603
Titmarsh R, Assad F, Harrison R (2020) Contributions of lean six sigma to sustainable manufacturing requirements: an Industry 4.0 perspective. Proc CIRP 90:589–593
Tripathy S, Sahu S, Ray PK (2013) Interpretive structural modelling for critical success factors of R&D performance in Indian manufacturing firms. J Model Manag 8(2):212–240
Vaidya S, Ambad P, Bhosle S (2018) Industry 4.0—a glimpse proceedings of second international conference on materials manufacturing and design engineering. Proc Manuf 20:233–238
Warfield JW (1974) Developing interconnected matrices in structural modeling institute of electrical and electronics engineering transactions on systems. Man Cybern 4(1):51–81
Yadav G, Desai TN (2016) Lean Six Sigma: a categorized review of the literature. Int J Lean Six Sigma 7(1):2–24
Yadav G, Desai TN (2017) Analyzing lean six sigma enablers: a hybrid ISM- Fuzzy MICMAC approach. TQM J 29(3):488–511
Yadav G, Seth D, Desai TN (2018) Application of hybrid framework to facilitate lean six sigma implementation: a manufacturing company case experience. Prod Plan Control 29(3):185–201
Yadav N, Shankar R, Singh SP (2020) Impact of Industry 4.0/ICTs, Lean Six Sigma and quality management systems on organizational performance. TQM J 32(4):815–835
Zhang A, Venkatesh VG, Wang JX, Venkatesh M, Wan M, Qu T (2019) Drivers of Industry 4.0-enabled smart waste management in supply chain operations: a circular economy perspective in China. Prod Plan Control 34(10):1–51
Zwetsloot IM, Kuiper A, Akkerhuis TS, de Koning H (2018) Lean Six Sigma meets data science: integrating two approaches based on three case- studies. Qual Eng 30(3):419–431
Kamble S, Gunasekaran A, Dhone NC (2020) Industry 4 0 and lean manufacturing practices for sustainable organizational performance in Indian manufacturing companies. Int J of Prod Res 58(5):1319–1337
Nafchi MZ, Mohelska H (2020) Organizational culture as an indication of readiness to implement industry4.0. Information 11(174):1–11
Muller JM, Kiel D, Voigt KI (2018) What drives the implementation of industry 4.0? The role of opportunities and challenges in the context of sustainability. Sustainability 10(247):1–24
Rossin F, Forget P, Lamouri S, Pellerin R (2019) Impacts of industry 4.0 technologies on Lean principles. Int J Prod Res. 50(6):1644–1661
Bhat A, Patil S, Belur J (2020) ISM fuzzy technique for management decision-making to reduce employee turnover: a study of Indian manufacturing industry. J Commerce Management 9(2):16–39
Arcidiacono G, Pieroni A (2018) The revolution Lean six sigma 4.0. Int J Adv Sci Eng Inform Tech. 8(1):141–149
Kamble SS, Gunasekaran A, Sharma R (2018) Analysis of the driving and dependence power of barriers to adopt industry 4.0 in Indian manufacturing industry. Comput Ind 101:107–119
Black SA, Porter LJ (1996) Identification of the critical factors of TQM. Decis Sci 27(1):1–21
Funding
The authors did not receive any funding from any organization for the submitted work.
Author information
Authors and Affiliations
Corresponding author
Ethics declarations
Conflict of interest
The authors have no potential conflicts of interest to declare that are relevant to the content of this article.
Human and animal rights
Authors do not involve any human/animal participants in research.
Informed consent
Research is conducted on data collected.
Additional information
Publisher's Note
Springer Nature remains neutral with regard to jurisdictional claims in published maps and institutional affiliations.
Appendix A
Appendix A
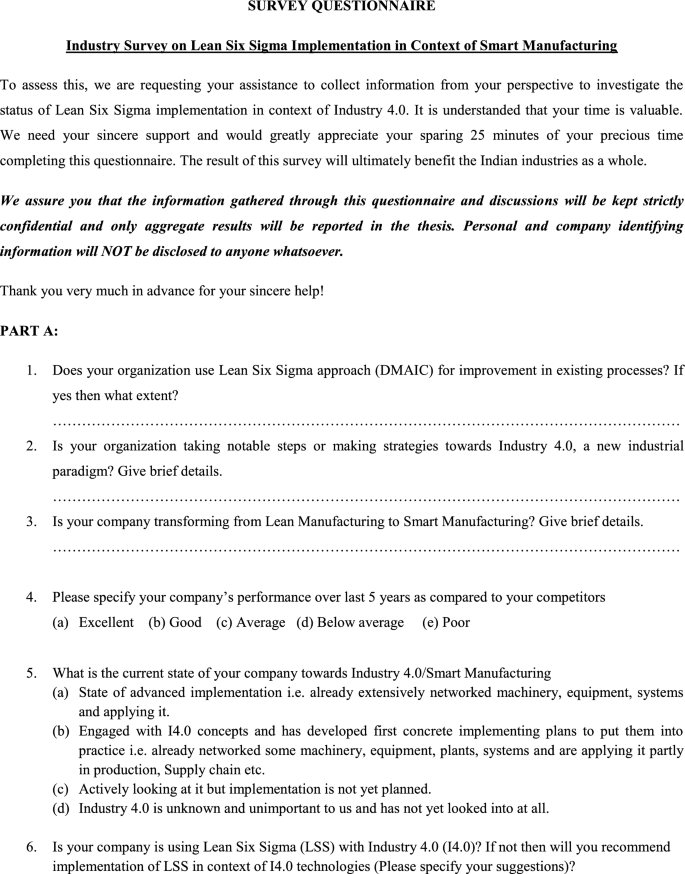


Rights and permissions
Springer Nature or its licensor (e.g. a society or other partner) holds exclusive rights to this article under a publishing agreement with the author(s) or other rightsholder(s); author self-archiving of the accepted manuscript version of this article is solely governed by the terms of such publishing agreement and applicable law.
About this article
Cite this article
Kumar, P., Bhamu, J., Goel, S. et al. Interpretive structural modeling of lean six sigma critical success factors in perspective of industry 4.0 for Indian manufacturing industries. Int J Syst Assur Eng Manag 15, 3776–3793 (2024). https://doi.org/10.1007/s13198-024-02375-y
Received:
Revised:
Accepted:
Published:
Issue Date:
DOI: https://doi.org/10.1007/s13198-024-02375-y