Abstract
The cross-association statistical technique is used to correlate major and minor lithofacies and the corresponding facies areas in the widely separated two borehole log profiles of Early Permian succession from the Kaghaznagar and Kothagudem sub-basins of Pranhita–Godavari Graben (PGG) of southeastern India. The one-to-one correspondence of the cross-association of major lithofacies and facies areas is strikingly similar and matches significantly more than expected. It indicates the continuity of single homogenous succession deposited under an identical depositional environment in the widely separated two sub-basins of PGG. However, the dissimilarity between the micro-lithofacies, on the other hand, suggests a different sub-environment through space and time. The significant correlation of major lithofacies and facies areas of the two sub-basins suggests meandering stream depositional facies model of the Early Permian Barakar succession in PGG. It may also provide information regarding the exploration of coal. The dissimilarities of cross-association at the micro-lithofacies level may reflect the differential subsidence through space and time.
Similar content being viewed by others
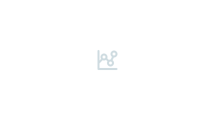
Avoid common mistakes on your manuscript.
1 Introduction
Stratigraphic correlation is the demonstration of equivalency of a stratigraphic unit in space and time, and the corresponding depositional environment (Krumbein and Sloss 1963; Doyle et al. 1994; Miall 2016). Conventional stratigraphic correlation is generally based on litho-, bio- and chrono-stratigraphic criteria. The matter of correlation between different geological sections (or cores) is relatively easier when the vertical rock sequence is of homogeneous lithology and thickness from one place to another. However, in many cases, the lithological correlation is difficult due to lateral changes in bed thickness and lithology, missing of strata by erosion, and tilting of strata. It will then be even difficult to designate the exact stratigraphic position of a rock column when it is compared with the type section. The cross-association statistical analysis should be one of the possible objective approaches to overcome problems of stratigraphic correlation in these cases. It has been successfully applied to correlate vertical succession of micro-facies in two carbonate sections more than 300 km apart, from the Sub-Himalaya of northwest India (Rao et al. 1985) and in detecting the similarity between upper Cretaceous carbonate rock type of Ajlun Group in central and north Jordon (Saqqa and Al-Saleh 2006). The Gondwana sequence of Peninsular India represents a superposition of discrete lithologies of sandstone, shale and coal, which are further divisible into a number of micro-lithofacies on the basis of texture. The coal seams have long been used as marker horizons for litho-stratigraphic correlation. However, this method seems to be inadequate to explain the equivalency of inter-seam micro-lithofacies, particularly in the coal-bearing Barakar Formation. The Early Permian Gondwana sequence represents such an example where the stratigraphic correlation has always been difficult due to lateral pinching and splitting of coal beds. The problem of correlation is further compounded due to great lateral variability in the thickness of lithological units. Khan and Tewari (2012a) have attempted intra-basinal stratigraphic correlation of borehole profiles, about 40 km apart, from the Early Permian Barakar Formation of East Bokaro Gondwana sub-basin of eastern India. An attempt is made to apply a similar technique for correlating inter-basinal vertical succession of major and micro-lithofacies in widely separated sub-basins of Pranhita–Godavari Graben (PGG) of southeastern India using the cross-association statistical analysis. The two sections under study are 300 km apart located in the southeastern (Kothagudem) and northwestern (Kaghaznagar) parts of PGG, respectively (figure 1). The quantitative result so obtained may help in analysing the sedimentation model useful in the exploration of Barakar coal in this basin.
Geological map of Pranhita–Godavari Graben of southeastern India (after Murthy and Rao 1996) showing locations of borehole profiles.
2 Geological setting and lithic-fill composition
The area under study is located in PGG, one of the major NNW–SSE trending linear belts of Gondwana formations which extend over 470 km in length and about 45 km wide in the southeastern part of the Indian peninsula (figure 1). It preserves about 3000 m thick lithic fill sediments deposited in the time span of 180 Ma, i.e., Early Permian to Early Cretaceous and was classified into ‘Talchir diamictite’, ‘Barakar sandstone’, ‘Kamthi sandstone’ of the Lower Gondwana sequence (table 1). The Barakar Formation is the main coal-bearing unit underlain by the Talchir Formation along a gradational contact and overlain by the Barren Measure Formation. More than 200 boreholes have been drilled in the the Barakar Formation by different coal exploration agencies such as Singareni Collieries Company Limited (SCCL), Indian Bureau of Mines (IBM), Geological Survey of India (GSI) and Central Mine Planning and Design Institute Limited (CMPDIL). Among these, two boreholes (figure 1) drilled and logged by GSI have been used in the present study. These litho-logs (figure 2) are not affected by faults and record lithofacies deposited in response to various sub-environments of the fluvial system.
The Gondwana lithic fill of the present area represents a fairly continuous succession comprising Lower and Upper Gondwana. The Barakar Formation covers an area of about 600 km\(^{2}\) and is made up of sandstone (60%), pebbly sandstone (PSD, 17%) and siltstone, shale/clay and coal (23%). It is divisible into two members namely the lower and upper. The lower member is 50–200 m thick and consists of PSD and feldspathic sandstone, siltstone and occasional coal bands. The upper member is 200–300 m thick and is chiefly constituted of fining upward coal-bearing cyclothemic sequence of lithologies such as sandstone, shale and coal whose number varies from 2 to 15. Lithofacies present in each cyclothem range from pebbly/very coarse-grained sandstone (CSD) at the bottom to arenaceous shale/carbonaceous shale to coal at the top (Tewari et al. 2009). The coal seams are constituted of interbanded carbonaceous shale–shaly coal–coal. The workable coal seams vary in number, from two to as many as eight, in certain places, while on an average three to four seams occur in most of these areas (Murthy and Rao 1996). These lithofacies can be categorised under six micro-facies groups namely very coarse/(PSD, CSD), medium-grained sandstone (MSD), fine-grained sandstone (FSD), shale and coal (C) in conformity with borehole record for quantitative stratigraphic correlation. The coarser members (sandstones) compare very closely in texture and sedimentary structure with channel sediments, whereas shale and coal represent flood plain and swamp deposits, respectively (Bogg 2005; Nichols 2009; Miall 2013). The lithology in vertical succession compares well with the sediments laid down by meandering river alluvium described by Miall (2013) and therefore a fluvial meandering stream depositional environment can be assigned to the Barakar succession of the PGG. The pebbly to very coarse sandstone (PSD) represents the channel floor (CSD and MSD) are channel (proximal and distal) deposits, whereas FSD represents the lateral accretion deposit on point bar. The interbedded sandstone–shale and grey shale, on the other hand, characterise proximal (levees) and distal (overbank) flood plain deposits, respectively. The interbanded carbonaceous shale–coal sequence represents the vegetal accumulation in back swamps in the flood plain of meandering streams that received fine clastic during the overflow of the river (Casshyap 1977). The micro-facies, thus, represents the deposit characteristics of channel, levees, and flood plain and back swamp sub-environments of the meandering stream depositional systems.
3 Principle of cross-association
There are different statistical techniques that can be used to analyse the data consisting of a series of lithological states. Since the data are in nominal scale, statistical methods based on the Pearson correlation coefficient cannot be used for the analysis, because these methods require data measured in a ratio scale. Thus, the data can only be analysed using the cross-association method, an approach essentially suitable to compare vertical sequences of nominal data (Davis 1986, p. 234). Cross-association is an index that tends to measure the degree of similarity or correspondence (equivalence) between two sequences.
In the present study, the two sequences represent two geological columnar sections (MK 001-Kaghaznagar and MKD 005-Kothagudem) and taken from two different localities of PGG. The two sequences are of length n and m, respectively, and that the variable of interest has k different nominal values (rock-unit types), coded as 1, 2, 3, ..., k. To assess the degree of similarity between two sequences, the nominal values in a given sequence are moved stepwise past the nominal values of a second sequence. At each step, the matching position, number of comparisons (the length of the overlapped sequences) and the number of matches are recorded. At each match position, the matching ratio (number of matches to number of comparisons) is computed, which is cross-association index (CAI). Assuming that the number of matches at position \(i,\, i\) =1, 2, 3, ..., \(m+n-\)1, then CAI is given by
where \(\Delta \) is the length of the overlapping sequences (number of comparisons), which takes the values 1, 2, 3, ..., minimum (n, m, i) and N is the number of matches. The CAI(i) ranges between zero and one and a large value of CAI(i) indicates the similarity of the two sequences. The significance of CAI is determined by \(\chi ^{2}\) test that involves comparison of a number of matches and mismatches of the overlapping segment with those between two totally random sequences containing the same number of observations in each state as the two strings of data under consideration.
Let us assume that we have two random sequences; each has the same number of observations and are of the same composition. Firstly, we find out the expected total number of matches and at any position, we have a match with probability p or a mismatch with probability \(1{-}p\). Thus, we have a Bernoulli trial (Binomial trail) with a probability of success = p and probability of failure = q. Suppose that the values of the first sequence be \(a_{1}\), \(a_{2}\), \(a_{3}\), ..., \(a_{k}\), where \(a_{i}\) denotes the total number of times that state i occurs, \(i= 1, 2, 3, {\ldots }, k\); \(\sum _{i=1}^k {a_i } =n\), and for the second sequence, the values are denoted by \(b_{1}\), \(b_{2}\), \(b_{3}\), ..., \(b_{k}\); \(\sum _{i=1}^k {b_i } =m.\) Using some counting techniques, the total number of possible ways for filling, at random, any matching position with two identical values of k (one value is for sequence 1 and the second value is for sequence 2) is \(m \times n\). The total number of possible ways for filling any match position with two identical values of k, where a match occurs, is \(\sum _{i=1}^k {a_i } b_i \). Thus, the probability of a match between two sequences at any position is given by
(For more details about counting techniques, see Schervish and DeGroot 2016.) At this point, we can say that the two investigated sequences originate from two populations with unknown p, i.e., \(H_{0}\): \(p \le p^* { vs}. H_{1}\): \(p > p^*\), where \(H_{0 }\) indicates that the two sequences are not similar and \(H_{1}\) the two sequences are similar.
Once the probability of a match \(p^*\) for a random sequence is computed, one can deduce the probability of a mismatch q as:
We can now estimate the number of matches (E) and mismatches (\(E')\) occurring in a random sequence
with n being the length of the compared sequence, E the expected number of matches from a random sequence and \(E^\prime \) the expected number of mismatches from a random sequence.
It should be noted that the number of comparisons ‘n’ expresses the length of the effective compared sequence (overlapped segment) and therefore varies according to the match position.
Let O be the total number of matches for an overlapping sequences of length \(\Delta \). Given the value of \(\Delta \), O is the sum of Bernoulli trials (binomial trails) with a common probability of success \(p^*\). However, these trails are not independent because there are possibilities of a match at position i that will reduce chances of getting a match at position j. In other words,
Thus, O is not a binomial random variable. However, the expected value of O continues to be \(\Delta \)p\(^*\). It can be shown that the conditional probability \(p^{**}\) is given by
where m and n are the lengths of the two sequences and k is a variable, i.e., major and minor lithofacies states.
As is seen in the above formula, \(p^{**}\) gets closer to \(p^*\) as m and n gets larger. Thus, for fairly large values of m and n which are nearly valid in our present study and under some conditions, the approximate distribution of O can be found in the literature under the null hypothesis. Serfling (1975) showed that the distribution of O can be approximated by the Poisson distribution. Saqqa and Al-Saleh (2006) concluded that the probability that O is larger than the given number can be approximated under the null hypothesis and hence the p-value of the test under the Poisson distribution can also be determined as
where O is the total number of matches for a given \(\Delta \), \(\Delta \) is the length of overlapping segments and o is the number of matches, \(H_{0}\) indicates that the two sequences are not similar, \({\mu = E }= \Delta p^{*}, e\) = Euler’s, where \({\mu = E = }\Delta p^*\), e = Euler’s number = 2.71828, and x is the number of occurrences.
The Poisson distribution can be used to provide a reasonable approximation to the binomial distribution if n is large and p is small. This led Serfling (1975) to recommend the \(\chi ^{2}\) test to determine whether the observed number of matches at a given matching position is significantly large so that the hypothesis \(H_{0}\) can be rejected, i.e., the two sequences are comparable. The approximate test statistics is as follows:
where O is the observed number of matches, \(O'\) is the observed number of mismatches, E is the expected number of matches and \(E'\) is the expected number of mismatches. The approximate distribution of \(\chi ^{2}\) under the null hypothesis is the well known \(\chi ^{2}\) distribution with one degree of freedom. The Yates correction is applied to the \(\chi ^{2}\)-statistics when the values of the expected number of matches (\(E'\)) are small, as for the comparison near the end of data sequences. The Yates correction calls for a subtraction of 0.5 from the absolute difference of the observed and expected number of matches (see Davis 1986, 236–237). The modified \(\chi ^{2}\) statistics becomes
Large values of \(\chi ^{2}\) or \(\chi ^{2}_{Y}\) indicate that there is a similarity between the two sequences (i.e., rejection of \(H_{0})\), while small values of \(\chi ^{2}\) or \(\chi ^{2}_{Y}\) indicate that the similarity between the two sequences is just as that of two independent random sequences of the same composition. \(H_{0}\) is rejected if \(\chi ^{2}\) or \(\chi ^{2}_{Y}\) is larger than a critical value taken from the \(\chi ^{2}\) table (e.g., Brase and Brase 2016) for a given level of significant \(\propto \) is 0.05; the corresponding critical value is 3.84.
4 Data analysis
In the present work, the method of cross-association analysis has been applied to the vertical succession of major and micro-lithofacies recorded in two arbitrary selected borehole sections about 300 km apart (figure 1). This distance seems adequate to represent all the homotaxial sediments of various sub-environments of meandering stream depositional environment. The borehole MKD 005 (Kothagudem) in the southeast, penetrating up to 731.0 m records 725.80 m of Barakar strata with 198 major lithofacies and 292 micro-lithofacies states, excluding 6.20 m weathered zone at the top. The borehole MK 001 (Kaghaznagar) in the NW has penetrated up to 332.0 m and represents 162 major lithofacies and 180 micro-lithofacies states in 321.0 m of Barakar strata, excluding 12.0 m weathered zone at the top. The thickness of major and micro-lithofacies states shows wide variation ranging from 0.10 to 14.20 m in the Kaghaznagar borehole MK 001 and 0.15–23.90 m in the Kothagudem borehole MKD 005. In the present study, the data string of borehole MK 001 from the bottom, one state at a time, and the degree of correspondence between the overlapped segments at each position was calculated. The process was repeated till the bottom most state of borehole MKD 005 coincides with the top most state of borehole MK 001. The cross-association analysis has been performed in two versions, one based on the three major lithofacies namely sandstone, shale and coal and the other based on the six minor lithofacies namely PSD, CSD, MSD, FSD, shale and coal. The sole purpose of these two analyses is to assess the significance of increased lithofacies descriptive detail for the resulting stratigraphic correlation. The quantitative results are listed in table 2.
5 Cross-association of major lithofacies
Considering the three major lithofacies of MK 001 and MKD 005 borehole log profiles using the notation of the previous section, we have k = 3, n=164, m = 198. Hence,
With major lithologic states, the maximum match is reached at the 92nd match position. The total number of matches at this position is 46 matches and 38 mismatches in 84 comparisons. The probability of matches under the condition of randomness of the data sequence, involving a similar number of lithologic states, is computed to be 0.404. Therefore, the probability of mismatches under conditions of randomness with the same frequencies of major lithofacies as in observed sequences is 0.596. Therefore, the expected number of matches and mismatches are 34 and 50, respectively. The corresponding computed value of the \(\chi ^{2}\) and \(\chi ^{2}_{Y}\) test function is 7.115 and 6.534, exceeding the critical value of 3.841 for one degree of freedom at 5% significance level (see the \(\chi ^{2}\) table in Davis 1986). Hence, the null hypothesis (\(H_{0})\) is rejected in favour of the alternative hypothesis (\(H_{1})\). At this overlapping position, the total number of the matches is large enough to indicate that the two Barakar sequences, though separated by about 300 km, have an amount of similarity more than the similarity occurring when the two Barakar sequences are two independent randomly selected sequences. To see how strong this conclusion is, one may calculate the p-value of the test, which is \(P_{r}\) (\(\chi ^{2}>\) 6.534), equation (3), the \(p^{**}\) value for this test is found to be 0.0407, we can also approximate the p-value using the Poisson approximation (equation 4) and using MINITAB statistical software the p-value comes out to be 0.121. A low p-value (\(\le \) 0.05) indicates that our sample provides strong evidence against the null hypothesis for the entire population. Similarly, \(p^{**}\) and p-values are the good indications that the evidence against \(H_{0}\) is strong.
The statistical result also implies that the basic cyclic pattern of fluvial sedimentation remained laterally invariant for a distance of at least 100 km as has been independently indicated by the stationary of Markov chain cyclicity model for the sedimentary succession (Tewari et al. 2009). Thus, the vertical sequence of lithologies, in both the borehole profiles, is statistically correlatable and suggests the same depositional model, i.e., meandering stream depositional environment as derived from palaeocurrents and palaeochannel studies (Singh and Tewari 2007; Tewari and Singh 2008).
6 Cross-association of micro-facies
With six micro-facies states of Kaghaznagar borehole MK’001 and Kothagudem borehole MKD 005 logs, using the notation of the previous section, in this case, we have k = 6, \(n =180\), m = 292. Hence, \(p^* = 0.191\). MK 001 vertical section is moved by the MKD 005 vertical section one position at a time. The maximum match is reached at a 112th position that involves 120 comparisons. At this position, the number of matches and mismatches are 27 and 83, respectively. The probability of matches under the condition of randomness of the data sequence, involving a similar number of litho-logic states, is computed to be 0.191. Therefore, the probability of mismatches under conditions of randomness with the same frequencies of micro-lithofacies as in the observed sequences is 0.819. The observed value of \(\chi ^{2}\) and \({\chi ^{2}_Y}\) test function is 2.258 and 1.909, less than the critical value (\(\chi ^{2} = 3.841\)) for one degree of freedom at 5% significance level. Therefore, the null hypothesis (\(H_{0})\) cannot be rejected at 5% level of significance such that the total number of matches is not large enough to indicate that the two micro-lithofacies sections have a degree of similarity over that similarity when the two micro-lithofacies sections are at any two independent randomly selected sections of equivalent composition. Thus, the vertical sequences of micro-facies in two selected borehole sections are not statistically correlatable and perhaps indicate juxtaposition of dissimilar micro-facies which are characteristics of diverse sub-environments. The statistical results assume that these two sequences are relatively not similar, though, in nature this is not the case. The \(p^{**}\)-value of the test (equation 3) in this case is 0.217 which is >0.05 indicating strong evidence against the null hypothesis hence reject the null hypothesis. On the other hand, the Poisson approximation of the p-value is 0.1670, which is also not significant at \(\propto \) = 0.05, i.e., 5% level of significance strengthens the above inference.
This statistical result indicates that the inclusion of internal facies details of sedimentary lithofacies does not improve its lateral stratigraphic correlation and actually have an opposite effect. In other words, the lack of significant correlation at a micro-facies level possibly suggests the deposition of sediment on different sub-environments at different locations during each phase of sedimentation, while channel sediments were deposited at one place, homotaxial levees, flood plain and swamp deposits were laid down simultaneously at other places. This inference strengthens the field observations that the splitting of coal seams and frequent occurrence of laterally restricted carbonaceous sequences too suggest that the environment was not identical everywhere, while coal forming conditions occurred at one place simultaneously sand and silt was being deposited elsewhere. Thus a coal seam, apart from being laterally restricted, may also exhibit diverse roof rocks at different places corroborating principal component and factor analysis of Early Permian Gondwana coal sequences elsewhere in central Peninsular India (Khan and Tewari 2011, 2012b).
7 Conclusions
The cross-association analysis of two widely separated boreholes drilled in the early Permian Barakar Formation of the PGG, Andhra Pradesh, has shown that despite the varying lengths of the sequences of lithofacies states, the two sections represent one and the same lithic assemblages. It would imply that the two sections, although widely spaced, provide evidence of similar depositional environment during the Barakar sedimentation. The depositional sedimentary units, such as channel-fill and over-bank deposits, show considerable lateral variation in both transverse and parallel directions to the channel belt owing to the variability in hydraulic conditions (Singh and Khan 2000; Bogg 2005; Miall 2013; Tewari and Khan 2017). On the other hand, an increase in the descriptive details of sedimentary succession in the criteria of litho-stratigraphic correlation does not improve its stratigraphic correlation and actually have an opposite effect due to differential subsidence of lithofacies in space and time.
References
Bogg S 2005 Principle of Sedimentology and Stratigraphy; 2nd edn, Springer, UK, 688p.
Brase C H and Brase C P 2016 Understanding Basic Statistics; 7th edn, Cengage Learning, USA, 118p.
Casshyap S M 1977 Patterns of sedimentation in Gondwana basins; In: IV International Gondwana Symposium, Calcutta, pp. 1–34.
Davis J C 1986 Statistics and Data Analysis in Geology, John Wiley & Sons, New York, 646p.
Doyle P, Bennett M R and Baxter A N 1994 The Key to Earth History – An Introduction to Stratigraphy, John Wiley & Sons, New York, 244p.
Khan Z A and Tewari R C 2011 R-mode factor analysis of lithologic variables from cyclically deposited Late Paleozoic Barakar sediments in Singrauli Gondwana sub-basin, Peninsular India; J. Asian Earth Sci. 40 144–149.
Khan Z A and Tewari R C 2012a Geostatistical cross association method used for the litho-stratigraphic correlation of coal measures profiles; J. Asian Earth Sci. 45 162–166.
Khan Z A and Tewari R C 2012b Principal component analysis of lithologic variables in Early Permian Barakar coal measures, western Singrauli Gondwana sub-basin of central India; J. Geol. Soc. India 79 404–410.
Krumbein W C and Sloss L L 1963 Stratigraphy and Sedimentation, Freeman & Company, USA, 660p.
Miall A D 2013 The Geology of Fluvial Deposits: Sedimentary Facies, Basin Analysis and Petroleum Geology, Springer Verlag, Berlin, 582p.
Miall A D 2016 Stratigraphy: A Modern Synthesis, Springer Verlag, Berlin, 454p.
Murthy B V R and Rao C M 1996 A new litho-stratigraphic classification of Permian (Lower Gondwana) successions of Pranhita–Godavari basin with special reference to Ramagundan coal belt, Andhra Pradesh, India, Gondwana Nine, Oxford & IBH Publishing Co. Pvt. Ltd., New Delhi, 1 697–712.
Nichols G 2009 Sedimentology and Stratigraphy; 2nd edn, John Wiley & Sons, New York, 452p.
Rao C G, Raj D and Singh S K 1985 Quantitative stratigraphic correlation by cross association analysis of micro-facies successions from two measured carbonate sections from the sub-Himalaya of northwest India; J. Indian Assoc. Sedimentol. 5 84–91.
Saqqa W A and Al-Saleh M F 2006 On the use of geostatistical cross association method for litho-stratigraphic correlation; J. Data Sci. 4 1–20.
Schervish M J and DeGroot M H 2016 Probability and Statistics; 4th edn, Addison-Wesley, USA, 911p.
Serfling R J 1975 A general poisson approximation; Ann. Probab. 3 726–731.
Singh A and Khan A 2000 Lithofacies analysis, architectural elements and depositional models of Yamuna River point bars; J. Indian Assoc. Sedimentol. 19 137–151.
Singh D P and Tewari R C 2007 Paleochannel patterns of early and middle Permian Gondwana streams of Bellampalli–Chinnur area of Andhra Pradesh; Gondwana Geol. Mag. 22 73–78.
Tewari R C and Khan Z A 2017 Structure and sequences in Early Permian fluvial Barakar rocks of peninsular India Gondwana basins; Arab. J. Geosci. 10 1–15.
Tewari R C and Singh D P 2008 Permian Gondwana Paleo-currents in Bellampali calbelt of Godavari valley basin, Andhra Pradesh and Paleogeographic implications; J. Geol. Soc. India 71(2) 266–270.
Tewari R C, Singh D P and Khan Z A 2009 Application of Markov chain and entropy to lithologic succession – An example from the Early Permian Barakar Formation, Bellampalli coalfield, Andhra Pradesh, India; J. Earth Syst. Sci. 118 583–596.
Acknowledgements
The borehole profiles used in this study were collected during an extensive field study of the area between the years 2006 and 2008. We are thankful to Sri Vassava Chari, the then General Manager (Exploration), Singareni Collieries Company Limited (SCCL), Kothagudem, Andhra Pradesh, for the permission to use these borehole profiles for research. Some of these borehole logs have been used in our earlier study of cyclical characters of Early Permian coal measures of Bellampalli sub-basin (JESS 2009). We are grateful to the reviewers for many useful comments and Associate Editor of the Journal of Earth System Science for critical assessment.
Author information
Authors and Affiliations
Corresponding author
Additional information
Corresponding editor: Partha Pratim Chakraborty
Rights and permissions
About this article
Cite this article
Khan, Z.A., Tewari, R.C. Lithofacies correlation in Early Permian fluvial Gondwana stratigraphy of southeastern India using cross-association statistics. J Earth Syst Sci 128, 3 (2019). https://doi.org/10.1007/s12040-018-1029-x
Received:
Revised:
Accepted:
Published:
DOI: https://doi.org/10.1007/s12040-018-1029-x