Abstract
From four Korean pine (Pinus koraiensis) orchards, 60 clones were selected and analyzed for the fatty acid and amino acid components of the seeds to reveal the variations and correlations of the seed characteristics among the clonal source orchards and clones. The nutritional components of the seeds of the P. koraiensis trees exhibited rich genetic variation; the variation coefficient of the fatty acids was 2.24–66.83 %, while the variation coefficient of the amino acids was 14.70–38.88 %. Relatively high genetic-improvement potential exists for the nutritional components of the seeds. The phenotypic differentiation of the fatty acid and amino acid components reveals that variation within the population (85.18 %) was the primary source for the variation of the fatty acid components; variation among the orchards (63.08 %) was the primary source of the variation of the amino acid components. Data drawn from various clonal source orchards all showed that the seed characteristics were highly controlled by heritability (h2 > 80 %), and the seed characteristics of the P. koraiensis trees exhibited a similar genetic gain trend. The principal components were analyzed to obtain the comprehensive principal component value for each clonal seed orchard. Twelve clones were selected based on a clonal selection rate of 20 %. Correlation and multiple stepwise-regression analyses were conducted, considering different location conditions, to reveal the stable correlations between the seed characteristics to facilitate improvements of the seed yield of P. koraiensis trees and the clonal selection. Species of real characteristics in P. koraiensis were controlled by higher heritability. Genetic gain was obtained by selecting of superior clones.
Similar content being viewed by others
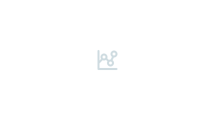
Avoid common mistakes on your manuscript.
Introduction
The tree species Pinus koraiensis, commonly called Korean pine, is in the genus Pinus in the family Pinaceae. Korean pine is a grade II national key preserved wild plant of China and is a native tree species in the Northeast China forest region (Lim 2012). P. koraiensis is a good source of timber, paper, and pine oil. In addition, P. koraiensis nuts are famous for their medical uses and nutritional values. P. koraiensis seeds are the most popular among current pine-seed products.
Pine nuts have very high nutritional value (Yoon et al. 1989; Wolff et al. 2000; Nergiz and Donmez 2004), containing large amounts of crude protein, crude fat, polysaccharide, and crude fiber as well as vitamins, minerals, and trace elements (calcium, phosphorous, manganese, cobalt, copper, and zinc) (Nergiz and Donmez 2004; Zadernowski et al. 2009). The internal quality is a key criterion on which the fruit marketability and practicability of woody plants that are used for edible oil are determined. The content of the nutritional components in pine nuts, such as fat and protein, is equivalent to the fat content in walnuts, which is significantly higher than that in hazelnuts, wild apricots, yellow horns and oil palms. The unsaturated fatty acid components in pine nuts have an enhancing and protecting effect on cytokines. The range of amino acids in pine nuts is relatively complete and the essential amino acid content is abundant (Mensink and Katan 1990; Willett and Ascherio 1994; Savage 2001; Ferramosca et al. 2008).
Studies of the variety and nutritional quality of edible nuts, including walnuts, chestnuts and almonds, have already been conducted (Savage 2001; Nasri et al. 2005; Venkatachalam and Sathe 2006; Sathe et al. 2008; Fernandes et al. 2010; Sharma et al. 2010). P. koraiensis has been studied regarding its flowering and fruiting, seed orchard management and nutritional value; however, there have been very few reports dedicated to the assessment of P. koraiensis’s germplasm resources or comprehensive evaluations of the nutritional components of P. koraiensis nuts among different clones (Kang and Lindgren 1998; Mutke et al. 2005). Therefore, in the present study, we elucidate the nutritional values of P. koraiensis nuts through the analysis of the nutritional components of pine nuts from different seed orchards; discuss the variations of the nutritional components of P. koraiensis among clonal source orchards and among clones within the same population; identify closely related economic indices based on the correlation analysis; select high-quality clones using a multi-character combined selection strategy; provide theories and excellent germplasm resources for the selection of P. koraiensis; and lay the foundation for the construction of seed orchards and advanced-generation seed orchards.
Materials and methods
Materials
Experimental materials were collected from four P. koraiensis seed orchards, which were located in Weihe (WH) Township in Shangzhi City, Linkou (LK) County in Mudanjiang City, Tieli (TL) City and Hegang (HG) City in Heilongjiang Province, China. Fifteen clones were selected from each seed orchard (Table 1). For each clone, clones from three standard trees were randomly selected. The clones collected from each tree were treated for seed production and were then used for sample preparation. The clones were collected in the fall of 2011 and were stored in the refrigerator (5 °C) in the experimental forest of the Northeast Forestry University for further use. The locations of the samples are shown in Fig. 1. The maternal plants in all four of the seed orchards were established after grafting in 1979. The parental origins for the seed orchards were the following: parent tree of Hebei (Weihe); parent tree of Wuying (Hegang); parent tree of the local plantation (Qingshan, Linkou); and parent tree of Wuying (Tieli).
Methods
The selected P. koraiensis seeds were first weighed following harvesting and then re-weighed after the shells were removed. The pine seed oil was extracted as follows. The pine nuts were ground into a fine powder and extracted using the Soxhlet extraction method at 70 °C for 8 h. This step was repeated three times. The solvent was dried in a rotary drier, and the oil was obtained, which was stored at −20 °C for further use. The extracted oil was treated using the simple methyl esterification method (Wolff et al. 1997) (KOH-CH3) and was analyzed using an HP6890/HP5973 gas chromatograph-mass spectrometer (GC–MS) (Agilent, USA) under the following conditions. A DB-5 (60 m × 0.25 mm × 0.20 μm) chromatographic column was used, and the temperature of the injection port was 250 °C. The initial temperature was 140 °C, which was maintained for 5 min, then increased to 240 °C at 2.5 °C/min, and then maintained at 240 °C for 5 min: the total analysis time was 50 min. The injection volume was 1.0 µL. Helium (He) was used as the carrier gas, delivered at 1.0 mL/min. The diversion ratio was 20:1. The temperature of the transmission line was 250 °C. Electron ionization (EI) was used as the ionization mode. The temperature of the ion source was 230 °C. The multiplier voltage was 1.4 kV. The electron energy was 70 eV. The molecular-weight scanning range was 50–550 amu. The scanning time was 0.5 s in the full-scan mode using the spectra library DATABASE/NIST98.L.
The defatted powder of the pine nuts was extracted as follows: 50 mL of water was added to 0.5 g of powder for extraction. Ultrasonic-assisted extraction (60 °C, 500 W) was used for 2 h. After suction filtration, the constant volume of the extract was set at 50 mL. The extract was used to determine the protein content. The Bradford method was used for protein-content determination (Shen et al. 2008), with bovine serum albumin as the standard. The standard curve was Y = 7.3943x + 0.0288, where Y represents absorbance (λ = 595 nm), and the unit of x is mg/mL. The obtained protein amino acid sample from defatting the pine nuts was pre-treated using the hydrochloric acid-hydrolysis method (Bond et al. 2005). A Hitachi L-8800 amino acid analyzer was used for testing.
Data processing and analysis
The ArcGIS software was used to construct the geographic coordinates for the sample collection. Data Processing System 14.01 and Predictive Analytics Software 18.0 were used for the analysis of quantitative traits based on genetic parameters. The experimental design used was hierarchical classification; the linear model was X ijk = μ + S i + T j(i) + ε ijk , where each observed value, X ijk , represents the kth observed value of the jth clone in the ith orchards; µ represents the orchards mean; the treatment effect among the seed orchards (fixed) is S i = (μI−μ); the clonal effect within the seed orchard (random) is T j(I) = (μij−μ); and the random error is ε ijk = (X ijk− μij). The sum of squares of the total variation (\({\text{SS}}_{\text{T}} = \sum\limits_{i = 1}^{a} {\sum\limits_{j = 1}^{m} {\sum\limits_{k = 1}^{n} {\left( {xijk - \bar{x}} \right)^{2} } } }\)) consists of sum of squares among the seed orchards (\({\text{SS}}_{t} = {\text{mn}}\sum\limits_{i = 1}^{a} {(\bar{x}_{i} - } \bar{x})^{2}\)), the sum of squares among the clones within the orchards (\({\text{SS}}_{\text{d}} = {\text{n}} \sum\limits_{i = 1}^{a} {\sum\limits_{j = 1}^{m} {\left( {\bar{x}_{ij} - \bar{x}_{i} } \right)^{2} } }\)), and the sum of squares of the testing error (\({\text{SS}}_{\text{e}} = \sum\limits_{i = 1}^{a} {\sum\limits_{j = 1}^{m} {\sum\limits_{k = 1}^{n} {\left( {x_{ijk} - \bar{x}_{ij} } \right)^{2} } } }\)). The α represents that there are numbers of seed orchards, m represents there are numbers of m clones in each seed orchard, and n represents there are numbers of observations in each clone.
The phenotypic differentiation coefficient (Vst) was used to reflect the phenotypic differentiation among the seed orchards:
where \(\delta_{{{\text{t}}/{\text{s}}}}^{2}\) represents the variance component among the seed orchards; \(\delta_{\text{s}}^{2}\) represents the variance component within the seed orchards.
A linear model, X ij = μ + S i + ε ij , was used to estimate the repeatability (R) of the clone in each orchard using the following formula: R = VC/(VC + VE/N), where VC represents the variance of the clone, VE represents the variance of the environment, and N represents the number of individuals measured for each clone. R was used as the upper limit of the estimate of the heritability (h 2). One standard deviation (σ A)—by which the clonal mean surpassed the total mean of all the seed orchards—was used as the selection standard to estimate the genetic gain. The following characteristics are critical to model selection: genetic gain, \(\varDelta G\left( \% \right) = (h^{2} \times {\text{S}}/\bar{x}) \; \times \; 100\;\% ,\;\,i = {\text{S}}/\sigma_{\text{A}} , \, \varDelta G = (ih^{2} \sigma_{\text{A}} /\bar{x})\; \times \; 100\;\% ,\,\;\bar{x}\) represents the mean of the characteristic; h 2 represents the clonal heritability; i represents the selection intensity; S represents the selection differential of the characteristic’s value; and σ A represents the standard deviation of the selection characteristic.
A Pearson correlation is used for the methods of correlation analysis. The following equation (Eq. 1) is used for measuring the relevance degree in traits, when data meets the normal distribution.
The meaning of \(\bar{x}\) and \(\bar{y}\) represent x and y, respectively. Meanwhile, the meaning of x i and y i represent x and y. The method of multiple stepwise regression analysis is established by the optimal combination of the multiple independent variable x. The regression equation can predict the regression analysis of dependent variable y. The regression equation with y = b 0 + b 1 x 1 + b 2 x 2 + b 3 x 3 +…+ b n x n. b 0 is the absolute term. b1, b1 and bn are partial regression coefficient of x1, x2 and xn. The multiple correlation coefficient is r, which represents the linear relationship between the independent variable (xi) and the dependent variable (y).
To evaluate the information contained in our experimental data, principal component analysis (PCA) was applied. This is a chemometric method to visualize information contained in experimental data and to find the true dimensions of a dataset (Miloun et al. 1992). The variables measured for each sample describe each sample in a variables space. PCA generates a set of new orthogonal variables (linear combinations of the original ones) so that the maximal amount of variance contained in the dataset (information) is concentrated in the first principal components. The loadings are the coefficients of the original variables defining each principal component.
Results
Variation of the nutritional components of Korean pine seeds
Korean pine nuts are the product of an edible woody oil tree species with oil content as high as 56.76 %. There are a variety of fatty acids in pine nuts. Gas Chromatograph-Mass Spectrometer-computer (GC–MS) was used to analyze the fat content of pine nuts from four different seed orchards (using a total of 60 clones). Table 2 shows that there were 11 types of fatty acids detected, including five saturated fatty acids—myristic acid (C14:0); palmitic acid (C16:0); margaric acid (C17:0); stearic acid (C18:0) and arachidic acid (C20:0)—as well as six unsaturated fatty acids—palmitoleic acid (C16:1∆9c); oleic acid (C18:1∆9c); gadoleic acid (C20:1∆9c); linoleic acid (C18:2∆9c,12c); α-linolenic acid (C18:3∆9c,12c,15c) and eicosadienoic acid (C20:2∆11c,14c). The mean level of saturated fatty acids (∑SFA) was 12.05 %, and C16:0 had the highest content, with a mean of 7.12 %. The total content of unsaturated fatty acids that are beneficial to humans was 84.67 %. The unsaturated fatty acids present included monounsaturated fatty acids (∑MUFA) (24.97 %) and polyunsaturated fatty acids (∑PUFA) (59.70 %). Among the unsaturated fatty acids, linoleic acid (C18:2∆9c, 12c) had the highest content (42.02 %), followed by oleic acid (C18:1∆9c), α-linolenic acid (C18:3∆9c,12c,15c), gadoleic acid (C20:1∆9c), eicosadienoic acid (C20:2∆11c,14c), and palmitoleic acid (C16:1∆9c).
The fat content and fatty acid components of Korean pine nuts from different seed orchards and the multiple comparison between them (Table 2) show that—except for the C14:0, C16:0, C18:3, and C20:2 seeds—all the other indices significantly differed among different seed orchards. The genetic-variation coefficient reflects the genetic-variation capacity of the characteristic. Of the fatty acid components, C14:0 had the highest variation coefficient (66.83 %), and C18:2 had the lowest variation coefficient (2.24 %), indicating that there was significant variation between different fatty acid components. Therefore, it is imperative to collect as many fine tree species as possible and expand breeding orchards as much as possible when constructing advanced-generation seed orchards. The potential of using fat content from the following seeds—C14:0, C17:0, C18:0, C20:0, C16:1, and C20:2—as indices for improvement was relatively high. Stability was the best when using C16:0, C18:1, C20:1, C18:2, C18:3, ∑SFA, ∑MUFA, ∑PUFA, and ∑USFA as the selection standards.
Variation in the level of fatty acids in Korean pine seeds was shown in Table 2. The variation coefficient indicates the dispersing characteristics of the character value, and correspondingly, a larger variation coefficient indicating greater dispersal of the character value. Korean pine seeds have rich inherent genetic variations. In addition, environmental heterogeneity among different seed orchards enhances differences in the seeds. Performing breeding selection based on characteristics with a relatively high variation coefficient from all the seed orchards can provide excellent propagative materials for improving germplasm resources of Korean pine.
Variation of the protein and amino acid components in pine nuts
Amino acids are the component units for protein. We should take the content and ratio of different amino acid components (especially essential amino acids) into consideration in the evaluation of the nutritional values of pine nuts. In the present study, the hydrochloric acid-hydrolysis method was used (tryptophan was damaged during the test; therefore, it was not detected). A total of 17 amino acids were detected. The mass fraction mean of the total amount of acids (TAA) was 40.433 %. There were seven essential amino acids; the mass fraction mean of essential amino acids (EAA) was 9.79 %. The percentage of essential amino acids relative to total amino acids was 24.21 %. Glutamate (Glu) had the highest content among all the amino acid components of pine nuts, with a mass fraction percentage of 8.46 %. Leucine (Leu) had the highest content among all the essential amino acids, with a mass fraction percentage of 2.7828 %. Except for methionine, there were significant differences among all the other amino acid components from different seed orchards (Table 3). The variation coefficient of proline (Pro) (38.88 %) was the highest among the amino acid components, while the variation coefficient of methionine (Met) (14.70 %) was the lowest. The amino acid components had relatively high potential for genetic improvement.
Comparing the amino-acid components of pine nuts from different seed orchards, the protein content and the variation coefficients of aspartic (Asp) and glycine (Gly) were the highest in the seed orchard in Hegang. The variation coefficients of cysteine (Cys), Pro, Met, and phenylalanine (Phe) were the highest in the seed orchard in Tieli. The variation coefficients of serine (Ser), Glu, alanine (Ala), tyrosine (Tyr), histidine (His), arginine (Arg), threonine (Thr), valine (Val), isoleucine (Ile), leucine (Leu), lysine (Lys), TAA, and EAA were the highest in the seed orchard in Weihe.
The protein content and all the amino acid components (except for methionine) from the seed orchard in Linkou were higher than the mean levels of the seeds from the other seed orchards, indicating that the breeding population of the seed orchard in Linkou was more suitable for the breeding of protein character-related propagative materials. The result may be associate with the annual precipitation and altitude of local seed orchard. The annual rainfall of linkou seed orchard is 520 mm, which is the lowest in the four locations. In addition, the elevation of linkou seed orchard is 400 m, which is the highest in the four locations. The different environment maybe effect the content of protein in tree. So future research should study the cause of this phenomenon.
Phenotypic differentiation among the fatty acid components and amino acid components
Nested analysis of variance was used to decompose the phenotypic variation. The percentages of variance components relative to the total variation among orchards and the variation within the population were calculated. The mean phenotypic variance of the fatty acid components among the orchards was 14.28 % of the total variation (Table 4); the mean phenotypic variance of the fatty acid components within the orchards was 82.86 % of the total variation. The phenotypic differentiation coefficient of the fatty acid components among the orchards was 0.15–37.71 %.
The variation among the orchards (14.82 %) was far smaller than the variation within a given population (85.18 %). The variation within a population was the primary source of the variation of fatty acid components. Table 5 shows that the mean phenotypic variance of the amino acid components among orchards was 62.80 % of the total variation; the mean phenotypic variance of the amino acid components within orchards was 36.73 % of the total variation. The phenotypic variance coefficients of all the amino acid components were higher than 50 %, except for Met, with a relatively low phenotypic variance coefficient (5.10 %). The variation among the orchards (63.08 %) was greater than the variation within the orchards (36.92 %). The variation among the orchards was the primary source of the variation of amino acid components.
Evaluation of the clones within the population and parameter estimation
A single-factor, randomized linear model was used to analyze the genetic parameters of the source population of each clone. The upper limit of heritability that was estimated from clonal heritability was above 80 % (Table 6), indicating that the differences among the clones were primarily caused by genetic effects. The fatty acid and amino acid components were strongly controlled by genetics, and character selection could yield relative high genetic gains.
The selection standard considered was a difference of one standard deviation by which the clonal mean surpassed the total mean of the population character of all the seed orchards (to analyze unsaturated fatty acid components with the desired economic characteristics and essential amino acid components in pine nuts). The component index selection had the expected genetic gains. Among the fatty acid components, C16:1, C18:1, C20:1, C18:3, C20:2, ∑MUFA, and ∑SFA from all four of the seed orchards achieved over 5 % genetic gains. Among the amino acid components, Thr, Val, Leu, Phe, TAA, and EAA had the most prominent selection effects, and all achieved over 10 % genetic gains.
Among the fatty acid components, C20:2 had the best genetic gains in all four of the seed orchards, with a genetic gain of variable amplitude (ΔG) of 21.56 % to 55.77 %. Among the amino acid components, Met had the highest genetic gains in the seed orchard in Hegang (18.69 %); the genetic gains of Phe were the greatest in the seed orchards in Linkou (29.5 %) and Tieli (48.36 %); and the genetic gain of Ile was the highest in the seed orchard in Weihe (43.38 %).
Correlation between seed characteristics
Because different orchards have different genetic backgrounds and different location conditions, the clonal orchards were analyzed separately for characteristic indices. The linear relationships between the protein content and oil content of the seeds from the four different locations were investigated. The correlations for the locations were as follows: Pearson (HG) = 0.604, Pearson (LK) = 0.226, Pearson (TL) = 0.227, and Pearson (WH) = 0.435. It is clear that the correlations were not consistent. Therefore, linear correlation analysis was performed separately on the fatty acid components and amino acid components.
Tables 7 and 8 list the analysis of the correlations among fatty acid components. In all four of the seed orchards, ∑SFA exhibited a positive correlation with C16:0, C18:0, and C20:0, and a negative correlation with C18:1 and ∑MUFA. ∑MUFA exhibited a negative correlation with C20:0, C20:1, C18:3, and ∑PUFA and a positive correlation with C18:1 and ∑USFA. ∑PUFA exhibited a positive correlation with C20:1 and C18:3; C18:1 exhibited a negative correlation with C20:0, C20:1, C18:3 and ∑PUFA; and ∑USFA exhibited a negative correlation with C16:0. Therefore, a combined selection of characteristics can be carried out between ∑MUFA and C18:1/∑USFA as well as between ∑PUFA and C20:1/C18:3.
Tables 9 and 10 list the correlations among the amino acid components. In the four different seed orchards, TAA exhibited a positive correlation with Thr, Ser, Glu, Gly, Val, Met, Leu, Tyr, Phe, Lys, His, and Arg. EAA exhibited a positive correlation with Thr, Ser, Glu, Gly, Val, Met, Ile, Leu, Tyr, Phe, Lys, Arg, and TAA. Arg exhibited a positive correlation with Ser, Glu, Leu, Tyr, Phe, Lys and His. Thr exhibited a positive correlation with Ser, Val and Tyr. Phe exhibited a positive correlation with Glu, Gly, Leu, and Tyr. Val exhibited a positive correlation with Ser and Val, and Gly exhibited a positive correlation with Ile and Leu. Therefore, combined selection of characteristics can be carried out among TAA, EAA, Thr, Ser, Phe, Arg, and Val.
Under different location conditions, correlations among the fatty acid components and the amino acid components of Korean pine nuts were very stable, indicating that the inherent genetic bases of these characteristics were closely related, and the characteristics exhibited broad adaptability to the heterogeneity of the environment. All the other characteristics performed differently under different location conditions, indicating that different location conditions altered the allele frequencies of specific sites through natural selection, and these sites controlled the adaptability-related characteristics.
Multiple stepwise-regression analysis
Multiple stepwise-regression analysis of fatty acid components on the oil content of seeds
We investigated the fatty acid components that affected oil content. The fatty acid components of Korean pine trees from all four of the seed orchards were used as independent variables: (X), X1 (C14:0), X2(C16:0), X3(C17:0), X4(C18:0), X5(C20:0), X6(∑SFA), X7(C16:1), X8(C18:1), X9(C20:1), X10(∑MUFA), X11(C18:2), X12(C18:3), X13(C20:2), X14(∑PUFA), X15(∑USFA), and X16 (∑), while the oil content of the seeds was used as the dependent variable (Y). The dependent variable Y was normally distributed, and therefore, multiple stepwise-regression analysis was performed. After rejecting the independent variables with an insignificant regression coefficient, the best regression equations were as follows:
Even though the oil content (Y) was affected by different fatty acid components in the four seed orchards, C14:0(X 1), C18:0 (X 4) and C20:2 (X 13) were the most stable and had a linear effect with the oil content (Y) in more than three of the seed orchards.
Multiple stepwise-regression analysis of amino acid components with regard to the protein content
We investigated the important factors of amino acid components that affected the protein content. The amino acid components of the Korean pines from all four of the seed orchards were used as independent variables: (Z), Z1(Asp), Z2(Thr), Z3(Ser), Z4(Glu), Z5(Gly), Z6(Ala), Z7(Cys), Z8(Val), Z9(Met), Z10(Ile), Z11(Leu), Z12(Tyr), Z13(Phe), Z14(Lys), Z15(His), Z16(Arg), Z17(Pro), Z18(TAA), and Z19(EAA). The protein content of the seeds was used as the dependent variable (Y), which was normally distributed. Therefore, multiple stepwise-regression analysis was performed and the best regression equations were as follows:
Thr(Z 2), Ser(Z 3), Glu(Z 4), Ala(Z 6), Cys(Z 7), Val(Z 8), Met(Z 9), Leu(Z 11), Tyr(Z 12), His(Z 15), Arg(Z 16) and Pro(Z 17) were the most stable and had a significant linear relation to the protein content (Y).
Multi-characteristic selection
The clones were from four different orchards and were therefore analyzed separately for their principal components. There are ten fatty acids indicators in oil content, including C16:1, C18:1, C20:1, C18:2, C20:2, C18:3, ∑MUFA, ∑PUFA, and ∑USFA. Ten indicators were selected as the variable factors for the principle components. We chose the protein content from protein indicators, including Thr, Val, Met, Ile, Leu, Phe, Lys, TAA, and EAA. The ten indicators were selected as the variable factors for the principle components (Table 11). The factors with an initial eigenvalue greater than 1 were extracted from each population, and the cumulative variance for each population was 89.08 % for HG, 83.39 % for LK, 84.99 % for TL and 91.38 % for WH.
The extracted principal components included most of the information of the quality characteristics of the seeds. The ratio of the eigenvalue associated with each principle component to the sum of the eigenvalues of the total extracted principle components was used as the weight to obtain a comprehensive model for the principle components. The comprehensive principle component values for all the orchards were calculated and sorted.
A selection rate of 20 % was used for each population to select clones. HG6, HG8, and HG39 were selected from the seed orchard in Hegang; LK79-36, LK15 and LK32 were selected from the seed orchard in Linkou; TL3101, TL1194, and TL1131 were selected from the seed orchard in Tieli; and WH28, WH19, and WH117 were selected from the seed orchard in Weihe. The nutritional components of the selected clones had higher values than the clonal source population for all the seed orchards (Table 11). The selected clones had good development values for seeds, and the selected clones could also provide excellent propagative materials for the construction of clonal seed orchards.
Discussion
Pine nuts are woody oil plants with high nutritional value. The content of oil and protein in pine nuts are equaled with that in walnut, armeniaca sibirica and chestnut (Chen et al. 2000; Hao et al. 2002; Mahesh and Shridhar 2006; Seeram et al. 2008; Li et al. 2009). In the present study, GC–MS was used to determine the fatty acid content of pine seed oil. In total, 11 fatty acids were detected, and the unsaturated fatty acid content (∑USFA) was 84.67 %; 17 amino acids were detected in the pine nuts, 7 of which were essential amino acids for humans. The essential amino acid content was 24.03 % of the total amino acid content.
The phenotypic characteristics of the plants reflect the adaptability of genotypes to environmental change. Irreversible changes occur to phenotypic characteristics under long-term selective pressure. New phenotypes are generated after stable inheritance. Therefore, phenotypic variations are very important for adaptability and evolution (LePage 2001; Pigliucci et al. 2006). Genetic variation is a product of gradual adaptation to the environment and continuous evolution of organisms and is an important characteristic of genetic information.
Phenotypic variation of seeds not only determines the dispersity of the species and the distribution pattern of the population but is also an important economic characteristic that can be used for development. It also is very important for the selection of varieties of forest trees (Greipsson and Davy 1995). Currently, genetic analysis is the focus of the study of the variation of seed characteristics of plants, especially the study of important economic characteristics. Seeking genetic materials with high-quality characteristics lays the foundation for genetic improvement.
The nutritional components of the Korean pine nuts contained rich genetic variation; the variation coefficient of fatty acids was 2.24–66.83 %, while the variation coefficient of amino acid components was 14.70–38.88 %, indicating that there was relatively large potential for genetic improvement among the nutritional components of Korean pine nuts.
The phenotypic differentiation among the fatty acid and amino acid components of pine nuts reveals that the variation within the population (85.18 %) was the primary source of the variation of the fatty acid components; the variation among the orchards (63.08 %) was the primary source of the variation of the amino acid components. The amino acid and fatty acid components had a negative or insignificant Pearson correlation, which might explain the inconsistent results of the phenotypic differentiation of the amino acid and fatty acid components. In addition, the heterogeneity of different seed orchards enhances the variations of seed characteristics. Korean pine trees are monoecious and cross-pollinated and are naturally pollinated in heterozygous seed orchards for a very long time. Thus, these trees have formed extensive hybrid orchards (Politov et al. 1999; Goroshkevich 2004), which provide advantageous conditions for the selection of germplasm resources of Korean pine. Genetic markers and seeding tests are useful for further revealing the genetic differences among the orchards and exploring the variation patterns (Zheng et al. 2009; Sharma et al. 2010; Gomes et al. 2014).
Under the same condition as other factors, the greater standard deviation yields greater gain in traits expected. Low phenotypic variation means that there is little opportunity to find trees much bigger than the population average. When selecting strength equals with heritability in characters, a greater gain is obtained in the greater variability characteristics of groups. Traits with bigger heritability can obtain greater genetic gain, with different characters with different genetic rates.
We studied the characteristics of Korean pine seeds from different seed orchards and revealed the breeding potential of the germplasm resource. We also estimated the genetic parameters and the expected genetic gains for the Korean pine seed characteristics. The seed characteristics were all highly controlled by heritability (h2 > 80 %) in the different clonal source orchards. The Korean pine seed characteristics all exhibited a similar trend for genetic gains; the fatty acid components (C16:1, C18:1, C20:1, C18:3, C20:2, ∑MUFA, and ∑SFA) achieved more than 5 % genetic gains in the four seed orchards, and the amino acid components (Thr, Val, Leu, Phe, TAA, and EAA) all achieved more than 10 % genetic gains in the orchards, which provided a basis for the quality selection of seed characteristics. Through the analysis of the principal components, the comprehensive principal component values for all the seed orchards were calculated and sorted. A clonal selection rate of 20 % was used as the standard, and 12 clones were selected. The desired characteristics of the selected clones were generally higher than the means of the clonal orchards, indicating that the clones could provide good clonal propagative materials.
The level of variation was bigger in the seed orchards due to the different sampling-site conditions and fewer hits, which caused the different between phenotypic and genetic variation (Sun et al. 2005). The differences inevitably leaded to different correlation between real properties in different seed orchards. In comparison, the habitat differences between individuals were smaller than the differences between seed orchards. The proportion of differences was extremely small in terms of environmental impact, which was more likely determined by genetic factors of plant. In order to fully reveal the real cause of phenotypic variation in seed orchards, we need to conduct further research into internal patterns of genetic variation, spatial distribution patterns, and the relationship in real properties.
Correlation analysis was conducted on clonal orchards separately. There was no significant correlation between the oil and protein contents of the pine nuts. Therefore, correlation analyses were conducted separately among the fatty acid and amino acid components. Under different location conditions, the correlation between the partial characteristics of the fatty acid and amino acid components was very stable; the inherent genetic bases of the characteristics were closely related and had a broad adaptability to the heterogeneity of the environment. Furthermore, based on the multiple stepwise-regression analysis, the factors that affected the oil and protein contents provided a basis for improving the seed yield and clonal selection of Korean pine trees.
In the present study, we conducted an initial evaluation of the germplasm resources of the Korean pine trees. However, we only used annual variation data from 1 year for analysis. Thus, the analysis of annual variation data from multiple years is necessary. Further studies will be focused on the management of field seedling cultivation and the morphology, phenophase, and patterns of physiological and environmental habitat factors.
Conclusion
Through the study of the seed characteristics of four clonal seed orchards of Korean pine, we reveal that the nutritional components of the Korean pine nuts contained rich genetic variation. Through selection, expected genetic gains could be achieved among the nutritional components of the pine nuts, and high-quality clones could be selected as superior clones, including HG6, HG8, HG39, LK79-36, LK15, LK32, TL3101, TL1194, TL1131, WH28, WH19, and WH117. The composition of fatty acid and amino acid in superior clones was significantly higher than the average level of clonal seed orchards. We also studied the phenotypic differentiation of clonal orchards under different locations and the correlations between population characteristics. The results reveal the stability among the seed characteristics, which provides a basis for improving the seed yield of Korean pine trees and the clonal selection.
References
Bond B, Fernandez DR, VanderJagt DJ, Williams M, Huang YS, Chuang LT, Millson M, Andrews R, Glew RH (2005) Fatty acid, amino acid and trace mineral analysis of three complementary foods from Jos, Nigeria. J Food Compos Anal 18:675–690
Chen ZX, Lei ZX, Liu HN, Wu GY, Wang XB (2000) Analysis of chestnut ingredients and its integrated quality evaluation. J Fruit Sci 17(4):286–289 (In Chinese)
Fernandes DC, Freitas JB, Czeder LP, Naves MMV (2010) Nutritional composition and protein value of the baru (Dipteryx alata Vog.) almond from the Brazilian Savanna. J Sci Food Agri 90:1650–1655
Ferramosca A, Savy V, Einerhand AWC, Zara V (2008) Pinus koraiensis seed oil (PinnoThin (TM)) supplementation reduces body weight gain and lipid concentration in liver and plasma of mice. J Animal Feed Sci 17:621–630
Gomes RA Jr, Gurgel FdL, Peixoto LdA, Bhering LL, da Cunha RNV, Lopes R, Pina AJdA, Veiga AS (2014) Evaluation of interspecific hybrids of palm oil reveals great genetic variability and potential selection gain. Ind Crops Prod 52:512–518
Goroshkevich SN (2004) Natural Hybridization between Russian Stone Pine (Pinus siberica) and Japanese Stone Pine (Pinus pumila), USDA Forest Service Proceedings pp. 169–171
Greipsson S, Davy AJ (1995) Seed mass and germination behavior in orchards of the dune-building grass Leymus-Arenarius. Ann Bot 76:493–501
Hao YB, Wang KJ, Wang SL (2002) analysis of fatty acid composition in several early fruit Walnut protein. Food Sci 23(10):123–125 (In Chinese)
Kang KS, Lindgren D (1998) Fertility variation and its effect on the relatedness of seeds in Pinus densiflora, Pinus thunbergii and Pinus koraiensis clonal seed orchards. Silvae Genetica 47:196–201
LePage BA (2001) New species of Picea A. Dietrich (Pinaceae) from the middle Eocene of Axel Heiberg Island, Arctic Canada. Bot J Linn Soc 135:137–167
Li M, Liu Y, Sun C, Meng YN, Yang KQ, Hou LQ, Wang JY (2009) Research advance about nutrients and medicinal value of Walnut. J Chinese Cereals Oils Assoc 24(6):166–169 (In Chinese)
Lim TK (2012) Pinus koraiensis. Edible Medicinal and Non-Medicinal Plants. pp 297–303
Mahesh V, Shridhar KS (2006) Chemical composition of selected edible nut seeds. Food Feed Chem 54(13):4705–4714
Mensink RP, Katan MB (1990) Effect of dietary trans-fatty-acids on high-density and low-density-lipoprotein cholesterol levels in healthy-subjects. N Engl J Med 323:439–445
Miloun M, Militky J, Forina M (1992) Chemometric for analytical chemistry: PC-aided statistical data analysis, 1. Ellis Harwood New York
Mutke S, Gordo J, Gil L (2005) Variability of Mediterranean stone pine cone production: yield loss as response to climate change. Agric For Meteorol 132:263–272
Nasri N, Khaldi A, Fady B, Triki S (2005) Fatty acids from seeds of Pinus pinea L.: composition and population profiling. Phytochemistry 66:1729–1735
Nergiz C, Donmez I (2004) Chemical composition and nutritive value of Pinus pinea L. seeds. Food Chem 86:365–368
Pigliucci M, Murren CJ, Schlichting CD (2006) Phenotypic plasticity and evolution by genetic assimilation. J Exp Biol 209:2362–2367
Politov DV, Belokon MM, Maluchenko OP, Belokon YS, Molozhnikov VN, Mejnartowicz LE, Krutovskii KV (1999) Genetic evidence of natural hybridization between siberian stone pine, Pinus Sibirica du tour, and dwarf Siberian pine, P, Pumila (Pall.) Regel. Forest Genetics pp 41–48
Sathe SK, Seeram NP, Kshirsagar HH, Heber D, Lapsley KA (2008) Fatty acid composition of California grown almonds. J Food Sci 73:607–614
Savage GP (2001) Chemical composition of walnuts (Juglans regia L.) grown in New Zealand. Plant Foods Hum Nutr 56:75–82
Seeram NP, Kshirsagar HH, Heber D, Lapsley KA (2008) Fatty acid composition of California grown Almonds. J Food Sci 73(9):604–607
Sharma OC, Murkute AA, Kanwar MS (2010) Assessing genetic divergence in seedling trees of Persian walnut (Juglans regia). Indian J Agric Sci 80:360–363
Shen L, Wang X, Wang Z, Wu Y, Chen J (2008) Studies on tea protein extraction using alkaline and enzyme methods. Food Chem 107:929–938
Sun HQ, Li A, Ban W (2005) Morphological variation and its adaptive significance for Changnienia amoena, an endangered orchid. Biodivers Sci 13(5):376–386 (In Chinese)
Venkatachalam M, Sathe SK (2006) Chemical composition of selected edible nut seeds. J Agri Food Chem 54:4705–4714
Willett WC, Ascherio A (1994) Trans-fatty-acids—are the effects only marginal. Am J Public Health 84:722–724
Wolff RL, Deluc LG, Marpeau AM, Comps B (1997) Chemotaxonomic differentiation of conifer families and genera based on the seed oil fatty acid compositions: multivariate analyses. Trees Struct Funct 12:57–65
Wolff RL, Pedrono F, Pasquier E, Marpeau AM (2000) General characteristics of Pinus spp. seed fatty acid compositions, and importance of Delta 5-olefinic acids in the taxonomy and phylogeny of the genus. Lipids 35:1–22
Yoon TH, Im KJ, Koh ET, Ju JS (1989) Fatty-acid compositions of Pinus Koraiensis seed. Nutr Res 9:357–361
Zadernowski R, Naczk M, Czaplicki S (2009) Chemical composition of Pinus sibirica nut oils. Eur J Lipid Sci Technol 111:698–704
Zheng YL, Sun WB, Zhou Y, Coombs D (2009) Variation in seed and seedling traits among natural orchards of Trigonobalanus doichangensis (A. Camus) Forman (Fagaceae), a rare and endangered plant in southwest China. New Forest 37:285–294
Author information
Authors and Affiliations
Corresponding author
Additional information
Project funding: This work was supported by the Special Research of Public Welfare Forestry Industry for Financial Support for “Korean pine Improved Type Selection and Directive Breeding Technology Research (201204320)” and the Fundamental Research Funds for the Central Universities (2572014AA23).
The online version is available at http://www.springerlink.com
Corresponding editor: Hu Yanbo
Rights and permissions
About this article
Cite this article
Zhang, Z., Zhang, H., Yang, C. et al. Clonal variations in nutritional components of Pinus koreansis seeds collected from seed orchards in Northeastern China. J. For. Res. 27, 295–311 (2016). https://doi.org/10.1007/s11676-015-0154-5
Received:
Accepted:
Published:
Issue Date:
DOI: https://doi.org/10.1007/s11676-015-0154-5