Abstract
In this paper, the assessment of seasonal water quality and land use land cover change in the Subarnarekha watershed in Ranchi stretch was analysed. Agricultural runoff along with climate change adds to the pollution risk to the Subarnarekha River of Ranchi stretch in Jharkhand. Water quality indicators, like acidity alkalinity (ALK), total dissolved solids (TDS), hardness (H), dissolved oxygen (DO), biochemical oxygen demand (BOD), chlorides (CL−), electrical conductivity (EC), salinity (SAL), resistivity (RES) and pH, were assessed as per the standard method. During monsoon season, acidity, alkalinity, hardness, chlorides, salinity, pH and DO decreased, whereas EC, TDS, BOD and resistivity increased in comparison to pre-monsoon season. In post-monsoon, chloride problem was observed very high. Hardness was least in monsoon and maximum in post-monsoon season. EC and BOD increased in monsoon season in comparison to other seasons. Statistical analysis like HCA (hierarchical cluster analysis) and PCA (principal component analysis) also confirmed the problem of TDS, EC, chloride and hardness in the area. WQI (water quality index) analysis showed that the water quality was poor to unsuitable on all the sampling points throughout the study area in all seasons. LULC (land use land cover) and NDWI (normalized difference water index) analysis had also concluded that due to high rate of urbanization, the area has undergone a massive change in terms of forest cover and water bodies. The need for afforestation, forest protection and wetland protection can be clearly seen from the result of this study.
Similar content being viewed by others
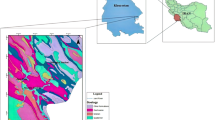
Explore related subjects
Discover the latest articles, news and stories from top researchers in related subjects.Avoid common mistakes on your manuscript.
Introduction
Watershed hydrology is affected by various factors like soil properties, vegetation types, geology, terrain, land use practices, climate change and their spatial pattern of interaction among them (Tsegaye and Bharti 2022; Shaikh et al. 2023). The increase in anthropogenic activities and development is changing the land use and land cover of the global landscape (Baltodano Martínez and van Griensven 2023). These changes adversely affect the water quality of the rivers and lakes (Muhammed et al. 2022). Climate change enhances these problems and also causes the new one. Therefore, it is an urgent need to assess the water quality changes with varying temperatures and precipitations (Madhusudana Rao et al. 2020; Alamdari et al. 2022). The impact of climate change on river or stream flow is also dependent on the season change in the area. Freshwater resources may become vulnerable and highly affected due to climate change. So, it is evident that both climate and land use changes are important driver of changes in watershed hydrology (Baltodano Martínez and van Griensven 2023).
The water quality parameters like pH, dissolved oxygen (DO), pathogens and nutrients are directly influenced by the rainfall and temperature. Excessive runoff in rainy season causes flood which increases water pollution by deteriorating the water quality of surface and ground water resources (Shaikh et al. 2023). The water quality also gets affected by drought. The concentration of contaminants increases because of less availability of water (Tsegaye and Bharti 2022). People are forced to use contaminated water for self-use, crops and animals. This situation leads to various water-borne diseases among plants and animals. Global warming leads to less dissolved oxygen in the water reservoir, and thus, increase in temperature adversely affects the quality of the water (Amanullah et al. 2020). Therefore, the monitoring and assessment of the water quality of surface waterbodies like river has become important to quantify the degradation. At the same time, it will also help in environmental management (Baltodano Martínez and van Griensven 2023).
In water quality assessment, lot of variables are responsible for influencing the water quality. Therefore, Multivariate statistical analysis is an important tool for the water quality assessment as it helps in better understanding of the spatial and temporal dynamics of water quality (de Andrade Costa et al. 2020; Varol et al. 2022). The relationship between the two parameters, its direction and strength can be assessed by the correlation matrix analysis. Through cluster analysis, one can group the data as per their nature. The similar parameters or homogeneous groups fall into the same group or class (Howladar et al. 2018). In Principal component analysis, the data pattern is recognised which help in explaining the variance of a large dataset. It transforms the dataset into a smaller set of independent (uncorrelated) variables (Arora and Keshari 2021).
The water quality index (WQI) is a mathematical tool that significantly minimizes the complex water quality data sets into a single classifying value. This value describes the water quality status of water bodies or degree of pollution (Uddin et al. 2023). This method was introduced through mathematical equations to know the water quality status in the river (Horton 1965). The WQI is a rating, reflecting the combined influence of different water quality parameters on the overall water quality (Gautam et al. 2015; Akhtar et al. 2021; Marselina et al. 2022).
The normalized difference water index (NDWI) has become a prominent and reliable tool in studying the surface water abstraction or availability patterns in land use–related analyses (Guha et al. 2020; Oad et al. 2023). It easily and explicitly differentiates all the land features like water bodies , land, soil and vegetation. The important feature of NDWI is that it removes any hindrance like soil and terrestrial vegetation elements and enlarges the existence of open water features in remotely sensed digital imagery (Guha et al. 2020; Singh and Kansal 2022).
LULC study is a crucial component of global change and sustainable development strategies, as it has major impact on ecosystem and climate change (Santy et al. 2020). It is used to quantify changes in the natural environment and human activity (Tsegaye and Bharti 2022). LULC has greater impact on water quality as most of the surface water bodies get recharged from surface runoff (Wu et al. 2020). Land use causes habitat degradation and poor water quality (Hong et al. 2016). Various activities related to urbanization, agriculture and industries may often involve aggressive land use which ultimately increases runoff (Mandal et al. 2021). The runoff transports pollutants to the rivers which results in surface water degradation. The climate change variables like land surface temperature, precipitation and extreme weather condition will pose a greater threat to the agriculture as more pests and disease outbreaks may occur which will reduce the crop productivity (Shukla et al. 2019).
The better understanding of LULC and climate change dynamics will help in water pollution management and sustainable land-use planning. Therefore, the objectives of the study are (1) to do seasonal water sampling and analysis by APHA (American Public Health Association) standard procedure, (2) to analyse water quality data statistically and WQI calculation, (3) to prepare NDWI for pre- and post-monsoon seasons using ArcGIS software, and (4) to assess the change in LULC (land use land cover). The novelty of the work lies in the study of land use and land cover change on the water quality of Subarnarekha River in a small but representative stretch that lies in the Ranchi district of Jharkhand. As the area is getting urbanized at the increasing rate, changes in LULC are very high. The comparative studies for water quality in all the three seasons like pre-monsoon, monsoon and post-monsoon were done. WQI and NDWI maps help in understanding the impact on water quality and quantity. Thus, the observation of the study and inferences will help the decision makers in identifying major hotspots for environmental management.
Methodology
Study area
The Subarnarekha River basin is the smallest among the 14 major river basins of India. It passes through three states in eastern part of India like Jharkhand, West Bengal and Orissa (Madhusudana Rao et al. 2020). The total stretch of river is approx. 395 km before it finally merges into the Bay of Bengal. The selected area for study is the stretch passing through Ranchi district whose co-ordinates were 23°27′1.27″N to 23°16′52.39″N and 85°39′51.98″E to 85°18′10.00″E. The total length of the river under investigation was 50.7 km. The basin is surrounded by Chota Nagpur Plateau. The origin of the river is near the village Nagri which is situated in Ranchi district of Jharkhand. The elevation of the area is 600 m. Along the river side, there are human settlement and industries. The river receives rainfall in the month of June-September. The river water is also used for irrigation. Twelve distinct locations were selected for sample collection based on major water pollution sources, such as proximity to any industry or any human settlement. The study area is shown in Fig. 1. The list of sampling points and their latitude and longitude is shown in Table 1.
Data acquisition and analysis
The seasonal water quality data for 12 points (S1–S12) was obtained for statistical analysis and WQI calculation. The data is processed to assess the land use land cover change which subsequently leads to water quality deterioration. The source of satellite data which is used for calculation of NDWI and LULC is shown in Table 2.
Water sampling and parametric analysis
The surface water sampling was done in the year 2022–2023 for three seasons like monsoon, post-monsoon and pre-monsoon. The sampling started in the month of August 2022 and completed in April 2023. The water samples were collected in a pre-rinsed polyethylene bottle and brought to the laboratory for analysis. The samples were analysed as per standard APHA methods in the lab. Twelve locations on the river stretch were selected for sample collection. The selection of sampling points was based on major milestones, such as proximity to any industry or any human settlement along 50.7 km of Ranchi stretch of Subarnarekha River. The samples were seasonally collected like in pre-monsoon (March–April), monsoon (August–September) and post-monsoon (November–December). The water quality parameter testing in laboratory was done for 11 parameters such as alkalinity, acidity, TDS (mg/l), hardness, DO (mg/l), BOD (mg/l), chlorides, EC (mS/cm), salinity, pH and resistivity based on the APHA standard method. The statistical analysis (like cluster analysis, PCA and correlation analysis) was done with the help of IBM SPSS Statistics 26 software.
Calculation of water quality index (WQI)
WQI calculated by Brown’s method, which is already used many times for region specific water quality index (Brown et al. 1972; Gorai et al. 2016; Uddin et al. 2021). WQI is a dimensionless unit, which can indicate the water quality ranging from poor to excellent (Uddin et al. 2021). The selected parameter for WQI calculation was alkalinity, total dissolved solids, hardness, BOD, DO, chloride and pH. For the WQI calculation, BIS 10500 standards were used. The Brown formula is given in Eq. 1.
where Wn is the unit weight of nth water quality parameters, and Qn is the quality rating of nth water quality parameter. Quality rating of nth water quality parameters will be calculated using Eq. 2.
where Vn is the actual value which can be found after the parameter analysis. Vs is the standard value of that parameter, and Vi is the ideal value of that parameter
The Vi value is 0 for all parameters except pH = 7 and DO = 14.6 mg/l.
W n is the unit weight, which can be calculated by Eq. 3.
where k is the constant proportionality which can be calculated by Eq. 4.
The obtained index value was matched with the given quality status range. Thus, the water quality can be fixed for a season.
Calculation of normalized difference water index (NDWI)
NDWI is an index, calculated by the satellite data derived in the form of near infrared and shortwave infrared channels (Guha et al. 2020). NDWI helps in assessing water stress in the vegetation and monitoring any change in the water availability. The NDWI value is basically an image ratio. The raster pixel of same functional form is divided by their corresponding values. The result of which gives the information related to change in water content. It was extracted from Landsat 8 satellite data and put them into Eq. 5. The preparation of NDWI maps for seasonal water quality was done with the help of Arc-GIS and Google Earth Pro. This procedure is shown in the flowchart in Fig. 2. NDWI maps help in determining the coverage of water in any region. The NDWI ranges between −1 and 1 (Nikam et al. 2020). The values more than 0.5 represent water body, and very low values represent vegetation. The cloud coverage was kept less than 10%. For NDWI calculation, raster calculator was used (Gebrechorkos et al. 2020).
where NIR is near-infrared (band 5 for Landsat 8), and SWIR is short wave infrared (band 3 for Landsat 8).
NDWI was calculated for pre- and post-monsoon seasons of the year 2022. The NDWI classes were classified in five groups such as impervious surface (−0.18 to −0.06), moderate vegetation (−0.26 to −0.22), healthy vegetation (−1 to −0.26), sparse vegetation (−0.22 to −0.18) and water bodies (−0.06 to 0.2). Healthy vegetation includes forest cover and high plants. Moderate and sparse vegetation means the agriculture and uncultivated lands, respectively. The impervious surface includes hardened soil, road pavement, rocks and urban areas.
Land use and land cover (LULC) maps
LULC change was determined by using satellite data. The study area image was procured for the years 2000, 2010 and 2020 from the USGS earth explorer. Data collection was done from Landsat-8 OLI for the years 2000, 2010 and 2020. The flow chart for making spatial distribution maps ire shown in Fig. 2. The data were pre-processed for radiometric correction. This data set was used for LULC map preparation. The obtained data were converted into a composite band. This data can be viewed with false colour composites (FCC) for the land feature identification. The FCC formed by bands 5, 4 and 3, which can be called composite band image, was classified by the ISO unsupervised classification method in Arc GIS map 10.5.1 (Yu et al. 2021). The five land features can be identified to assess LULC change. They are water bodies, vegetation, built-up area, agriculture and barren land. The unsupervised classification method does not need any prior knowledge of land cover types. Class assignments to each pixel were done by the interpreter (Nordin et al. 2022). Unsupervised classification was developed through different clustering methods such as K-means and interactive self-organization data analysis (ISODATA). This study uses unsupervised classification method for the different land cover classifications with the use of Landsat satellite image and GIS software (Ali et al. 2019).
Results and discussion
Water quality analysis
During monsoon season, acidity, alkalinity, hardness, chlorides, salinity, pH and DO decreased, whereas EC, TDS, BOD and resistivity increased in comparison to pre-monsoon season. The electrical conductivity at S2, S3, S8, S9, S10 and S12 was very high. The highest EC observed was 1057 micro S/cm. at S10. TDS was found very high at many sampling points. The three sampling points like S2, S10 and S12 had recorded TDS beyond permissible level. Sampling points S3, S8, S9 and S11 had recorded TDS beyond 450 mg/l. The pH values were observed in the standard range, i.e. 6.5–8.5, but mostly in between 7 and 8.5.
In post-monsoon, chloride problem was observed very high. The calcium and magnesium chlorides were the main component causing chloride problem in water. Sampling points like S7, S8, S10 and S12 had recorded chloride towards higher value than other points. EC was more at sampling points S1, S2, S3 and S4. At S3, the least pH value, i.e. 6.5 was observed. The rest of the points observed pH values in the standard range, i.e. 6.5–8.5. On an average, TDS values were more than 250 mg/l at all the points. The acidity was least in post-monsoon season. In pre-monsoon, acidity was higher at points S5, S8, S10 and S12. Hardness was found maximum at S12. Chloride problem was observed at sampling points, S3, S4, S7, S8, S10 and S12. The pH values were observed in the standard range, i.e. 6.5–8.5.
Hardness was least in monsoon and maximum in post-monsoon season. EC and BOD increased in monsoon season in comparison to other seasons, although BOD was well within the permissible limit. Dissolved oxygen was comparatively less in monsoon than in other seasons. Chlorides were less in monsoon than in pre-post-monsoon. The presences of chlorides were related with industrial pollution. pH was highly alkaline in nature in post-monsoon. pH always has a very important role in altering the aquatic environment. The pH of natural waters usually remains around 7, but the presence of pollutants causes it to change.
The BOD level varied in both seasons like in monsoon as well as in post-monsoon season. During monsoon, BOD increased, although it was well within permissible limit. These occurred due to the mixing of surface runoff with the river water (Jiménez-Navarro et al. 2021). Occurrence of heavy rainfall can lead to increased runoff, which had carried pollutants into waterways and increased BOD levels. The water quality was worst in pre-monsoon. There was high temperature and least rainfall during pre-monsoon. Changes in temperature can affect the solubility of minerals in water (Giri and Singh 2014; Jain and Singh 2020). As the solubility of minerals increased due to increase of temperature, it had led to higher levels of dissolved calcium and magnesium in water (Gautam et al. 2015).
Water hardness was least in monsoon than pre-post-monsoon season. The hardness of water impacts significantly to the human health, environment and infrastructure. During monsoon season, the temperature of ambient environment decreased; it might be a reason for decreased hardness in water. Water hardness in post-monsoon had also increased because of less rainfall (Gaur et al. 2020). Water hardness got affected by the changes in precipitation patterns. Drought conditions caused increased concentrations of minerals in surface water and groundwater due to reduced dilution. Conversely, heavy rainfall increased dilution and reduced water hardness, acidity, salinity, electrical conductivity and alkalinity. The drop in ambient temperature reduced chloride content in water (Wang et al. 2020). A higher value of hardness referred to more inflow of runoff water in the river. TDS may also contribute to hardness of water. More surface runoff and hardness cause increase in pH.
In monsoon season, the runoff carried pollutants like sediments, nutrients, fertilizers and pesticides from agriculture and industrial areas. The increased concentration of such pollutants resulted in decrease dissolved oxygen levels, pH and increased BOD, turbidity and total suspended solids (TSS). During monsoon season, more runoff and erosion of sediments took place because of excessive rain. Therefore, higher TDS was observed in this season. In pre-monsoon season, higher TDS was observed because the higher temperature increased the rate of chemical reactions which led to increased solubility of minerals in the water. Land use land cover changes also led to increase in TDS. Deforestation and unscientific agricultural practices led to increased erosion and sedimentation in the waterways (Garg et al. 2016).
Figure 3 shows the spatial distribution of total dissolved solids in Subarnarekha River as per the sampling location in pre-monsoon. In pre-monsoon season, temperature is at its peak and the pollutant gets concentrated, so TDS problem becomes more pronounced in comparison to the other. Higher TDS infers to city and industrial releases to the river. TDS changes with more dilution or addition to the natural water. Naturally, the river water temperature changes are attributed to the atmospheric changes.
Statistical analysis
The descriptive statistics of dataset are given in Tables 3, 4 and 5. Among the obtained data in the pre-monsoon season, a strong positive correlation was found between TDS and EC (0.839), salinity and TDS (0.804), and hardness (0.810). A strong negative correlation was found between resistivity and TDS (−0.756) as well as EC (−0.984). The increment in TDS is usually associated with increase in salts in water samples, which also increases the EC of water. The correlation analyses for all the three seasons are given in Table 6, 7 and 8. During summer season, the surface water evaporates and the concentration of salts and ions increase tremendously. In the monsoon season, the strong positive correlation was found between EC and TDS (0.976), salinity and TDS (0.997), as well as salinity and EC (0.959). In monsoon season, the influx of soil fines and agrochemicals in form of runoff is a common phenomenon in the water bodies. A strong negative correlation was found between resistivity with TDS (−0.0981), EC (−0.946) and salinity (−0.983). Similarly, in the post-monsoon season, the strong positive correlation was found between salinity and EC (0.855). A strong negative correlation was between Cl− and TDS (−0.738), salinity and BOD (−0.725), resistivity and TDS (−0.784), EC (−0.760) and pH (−0.900).
Hierarchical cluster analysis (HCA)
Hierarchical cluster diagram is to recognize the source and similarities between the water chemistry factors. HCA is a prevalent data analysis technique that displays the results in the form of a tree diagram known as a dendrogram (Taşan et al. 2022). The parameters can make a cluster based on their origin types. In pre-monsoon, parameters were classified into two major clusters at the mean distance, whereas overall eleven clusters were formed to combine all the parameters (Arora and Keshari 2021).
In pre-monsoon, the cluster-1 composed of parameters at mean distance BOD, DO, RES, pH, ALK, hardness, chloride, acidity, TDS and salinity indicating similarity in parameter variation. Out of all BOD, DO, RES, pH, ALK, hardness, chloride and acidity, these parameters showed the maximum similarity and formed a cluster at the minimum distance. All these parameters were closely correlated and formed a cluster, whereas TDS and salinity showed the lesser distance and more similarity between them. Cluster 2 composed of only one parameter, i.e. electrical conductivity (EC). A cluster, with a single member, showed that the parameter holds a considerable distance from all the other remaining parameters. They have different rates of change than other parameters. In monsoon, also the two clusters are formed and similar results were observed as in pre-monsoon. In post-monsoon, three clusters were observed. The cluster 1 composed of BOD, DO, RES, Acidity, pH, Alkalinity and hardness. Cluster 2 composed of chloride. Cluster 3 composed of TDS, salinity and EC, where TDS and salinity were closely correlated. EC has different rates of change than other parameters. The cluster analysis is shown in Fig. 5 a, b and c.
Principal component analysis
PCA was important to extract the hidden information for the proper understanding of the datasets. A seasonal PCA was done on the obtained data. It used rotation of the components to obtain optimal distribution of variances in among various components. Therefore, varimax rotation was applied to obtain optimal distribution of variances in various components (Arora and Keshari 2021).
In the pre-monsoon months, the first four factors contribute to 84.577%, 89.365% for monsoon season and 86.055% for post-monsoon season with a significance higher than 1. The number of factors (clusters) can now be selected based on principal components showing eigenvalues above one or number of principal components forming the cliff in scree plot (Nguyen and Huynh 2022; Varol et al. 2022). It can be observed from the scree plots (Fig. 4a–c) for three seasons that only four clusters are needed to group the water samples. Using the varimax rotation, it was determined that for pre-monsoon season, the factor 1 consisted of parameters indicating presence of salts, metals and other impurities like TDS, EC, salinity and Cl−. Factor 2 consisted of hardness. Factor 3 included the pH determining components such as acidity and alkalinity. Factor 4 had biological components such as BOD and DO. For monsoon season, the factor 1 consisted of TDS, EC and salinity, factor 2 consisted of DO and Cl− and factor 3 consisted of hardness and pH, while factor 4 consisted of alkalinity. For post-monsoon season, the factor 1 included resistivity, factor 2 consisted of alkalinity and DO, while factor 3 consisted hardness and factor 4 consisted of pH. Therefore, it is clear that the water sample data for three seasons can be clustered into four groups (Fig. 5).
WQI analysis
The WQI is an indicator of health of aquatic bodies as there use for human consumption. Knowledge of the water quality and evaluation of water quality index (WQI) plays a significant role in water quality control and management (Marselina et al. 2022). Water quality can be treated as excellent, good, poor and very poor if WQI lies in the ranges 0–25, 26–50, 51–75 and 76–100, respectively (Syeed et al. 2023). In this study, it was found that the water quality was poor to unsuitable on all the sampling points throughout the study area in all seasons. In the pre-monsoon season, the water had higher pH, EC, chlorides and alkalinity. This lowers the water quality. Increased pH, EC and alkalinity indicate the presence of carbonaceous salts in the water bodies, which get highly concentrated in summers with lowering of water table. In the monsoon season, the WQI further deteriorates to unsuitable category as the surface erosion is common phenomenon in monsoon period (Azhari et al. 2022). This leads to flushing of soil fines and metals in the water bodies making it quite high with TDS and EC. High TDS values make water unfit for drinking. Similarly, in the post-monsoon season, TDS and salinity values are high in water bodies along with hardness and EC. A WQI value in all seasons at every sampling point is shown in Fig. 6.
Analysis of NDWI results
From Fig. 7 a and b, it was seen that in the year 2022, there was 1% reduction in impervious surface (from 452.14 to 392.45 km2) and 13% reduction in sparse vegetation (from 2393. to 1772.66 km2) from pre-monsoon season to monsoon season. This usually occurs as the surface soil moisture drop significantly. The ambient temperature change affects the water availability (Oad et al. 2023). The clayey loam soil (rich in kaolinite and Illite clay) which is dominantly present in Ranchi City becomes quite hardened and appears as impervious surface. In the post-monsoon period, the vegetation cover flourishes as the soil regains its moisture (Jean Milien et al. 2023). Due to low water availability, the agriculture is dependent on rain. Most croplands are left barren in summers. In case of healthy vegetation and moderate vegetation, there was a slight increase, i.e. 4% (from 575.05 to 763.016 km2) for healthy vegetation and 10% (from 1431.62 to 1906.67 km2) for moderate vegetation respectively. Commercial cropping period starts from monsoon months (June–July), and harvesting occurs in post-monsoon months (October–November). Weed growth is high in post-monsoon months and thus increases in the vegetation cover observed. The area of water bodies increased from 30.66 km2 in pre-monsoon to 48.21 km2 in post-monsoon season. Ranchi City has an average rainfall of 1200 to 1400 mm annually. The water level rises in major water bodies, while the rainfed tributaries and ponds are also filled significantly in monsoon and post-monsoon months compared to pre-monsoon months (Deoli et al. 2022).
LULC analysis
The land use land cover pattern played a crucial role in land degradation analysis (Tsegaye and Bharti 2022; Alshehri et al. 2023; Sadhwani et al. 2023). In this study, a two-decade study showed that surface water body increased from 156.01 to 326.71 km2 (2000 to 2010) and then reduced to 267.28 km2 in 2020. This could be due to climate variation, i.e. due to change in precipitation and temperature pattern. The significant reason in the last decade was speedy urbanization (Sadhwani et al. 2023). The construction of residential houses and commercial buildings took place mostly at the cost of agricultural land and water bodies (Hoang et al. 2020). The changes in the LULC are mainly due to anthropogenic activities (Malede et al. 2022). Similar is the case of uncultivated land, which has increased from 617.83 km2 in 2000 to 971.61 km2 in 2020. Lack of rainfall or irrigation water leads to agricultural land left uncultivated. The land is reserved for future construction work. The forest cover and agricultural land have dramatically reduced in past two decades from 1130.967 km2 in 2000 to 551.833 km2 in 2020 and 2188.67 km2 in 2000 to 1969.27 km2 in 2020 respectively. The LULC analysis is shown in Fig. 8 a and b. Forest cover has been deforested mostly for infrastructure development and mining activities in Jharkhand. Loss of agricultural land is attributed to urbanisation solely.
Conclusion
The main aim of this study was to understand the impact of land use land cover changes on the water quality. As the area is under the speedy urbanization, the construction of residential houses and commercial buildings took place mostly at the cost of agricultural land and water bodies. Subarnarekha is the longest river in the north-east region. The human settlement and industries alongside the river pollute the water also. Strong scientific proof supports the anthropogenic origin of climate change, and impacts on natural and human systems have already been detected (IPCC 2018). To analyse the impact on water of Subarnarekha River, 12 sampling points were selected. Samples were taken seasonally and sent to the lab and analysed for 11 water quality parameters. The seasonal data obtained was statically analysed with correlation analyses, HCA and PCA. It was observed that TDS, chloride, EC and hardness were the major problematic parameter. WQI analysis was also done and observed that the water quality was found poor to unsuitable on all the sampling points throughout the study area in all seasons. LULC change rapidly alters the hydrologic process with an increase in urbanization. LULC and NDWI analysis had also concluded that due to high rate of urbanization, the area has undergone a massive change in terms of forest cover and water bodies. Also, due to uncontrolled urbanization, the enormous growth of population and industrialization, most of the city has now been covered with concrete as the housing has become the important issue. Due to impervious nature of the concrete, the infiltration of water has stopped which led to depletion of groundwater table. The analysis of the LULC data also suggested the similar view. It is the utmost demand to manage the environment by planting more trees and protecting forests in the area.
Data availability
All the data and material will be available on responsible request.
References
Akhtar N, Ishak MIS, Ahmad MI, Umar K, Md Yusuff MS, Anees MT, Qadir A, Ali Almanasir YK (2021) Modification of the water quality index (WQI) process for simple calculation using the multi-criteria decision-making (MCDM) method: a review. Water 13(7):905
Alamdari N, Claggett P, Sample DJ, Easton ZM, Yazdi MN (2022) Evaluating the joint effects of climate and land use change on runoff and pollutant loading in a rapidly developing watershed. J Clean Prod 330:129953
Ali FRAKM, Fouad KMA, Abdul-Rahman BA (2019) Evaluation of impact of vegetation decrease on precipitation rates in Baghdad City using remote sensing technique. Eco Env & Cons 25:S44–S50
Alshehri F, Abuamarah BA, Abd El-Hamid HT (2023) Impact of land use dynamics on land surface temperature using optical remote sensing data integrated with statistical analysis in Riyadh, Saudi Arabia. Adv Space Res 72(5):1739–1750. https://doi.org/10.1016/j.asr.2023.04.051
Amanullah et al (2020) Effects of climate change on irrigation water quality. In: Fahad S et al (eds) Environment, climate, plant and vegetation growth. Springer, Cham. https://doi.org/10.1007/978-3-030-49732-3_6
Arora S, Keshari AK (2021) Pattern recognition of water quality variance in Yamuna River (India) using hierarchical agglomerative cluster and principal component analyses. Environ Monit Assess 193:1–18
Azhari HE, Cherif EK, Sarti O, Azzirgue EM, Dakak H, Yachou H, Esteves da Silva JC, Salmoun F (2022) Assessment of surface water quality using the water quality index (WQI), multivariate statistical analysis (MSA) and geographic information system (GIS) in Oued Laou Mediterranean Watershed, Morocco. Water 15(1):130
Baltodano Martínez A, van Griensven A (2023) How remote sensing can identify land cover and climate change impacts on lake water quality: Lake Nicaragua and Lake Titicaca case studies. In: EGU General Assembly Conference Abstracts, EGU23, the 25th EGU General Assembly. EGU, Vienna, Austria. https://doi.org/10.5194/egusphere-egu23-11416
Brown RM, Mccleiland NJ, Deiniger RA, O’Connor MF (1972) Water quality index-crossing the physical barrier. Res Jerusalem 6:787–797
de Andrade Costa D, Soares de Azevedo JP, Dos Santos MA, dos Santos Facchetti Vinhaes Assumpção R (2020) Water quality assessment based on multivariate statistics and water quality index of a strategic river in the Brazilian Atlantic Forest. Sci Rep 10(1):22038
Deoli V, Kumar D, Kuriqi A (2022) Detection of water spread area changes in eutrophic lake using Landsat data. Sensors 22(18):6827
Garg V, Dhumal IR, Nikam BR, Thakur PK, Aggarwal SP, Srivastav SK, Senthil Kumar A (2016) Water resources assessment of Godavari River basin, India. In: Proceedings of ACRS 2016: 37th Asian Conference on Remote Sensing Organized at Colombo, Sri Lanka, pp 17–21
Gaur S, Mittal A, Bandyopadhyay A, Holman I, Singh R (2020) Spatio-temporal analysis of land use and land cover change: a systematic model inter-comparison driven by integrated modelling techniques. Int J Remote Sens 41(23):9229–9255
Gautam SK, Maharana C, Sharma D, Singh AK, Tripathi JK, Singh SK (2015) Evaluation of groundwater quality in the Chotanagpur plateau region of the Subarnarekha River basin, Jharkhand State, India. Sustain Water Qual Ecology 6:57–74
Gebrechorkos SH, Bernhofer C, Hülsmann S (2020) Climate change impact assessment on the hydrology of a large river basin in Ethiopia using a local-scale climate modelling approach. Sci Total Environ 742:140504
Giri S, Singh AK (2014) Assessment of surface water quality using heavy metal pollution index in Subarnarekha River, India. Water Quality, Exposure and Health 5(4):173–182
Gorai AK, Hasni SA, Iqbal J (2016) Prediction of ground water quality index to assess suitability for drinking purposes using fuzzy rule-based approach. Appl Water Sci 6:393–405
Guha S, Govil H, Besoya M (2020) An investigation on seasonal variability between LST and NDWI in an urban environment using Landsat satellite data. Geomat Nat Haz Risk 11(1):1319–1345
Hoang NH, Ishigaki T, Kubota R, Tong TK, Nguyen TT, Nguyen HG, Yamada M, Kawamoto K (2020) Waste generation, composition, and handling in building-related construction and demolition in Hanoi, Vietnam. Waste Manag 117:32–41
Hong C, Xiaode Z, Mengjing G, Wei W (2016) Land use change and its effects on water quality in typical inland lake of arid area in China. J Environ Biol 37(4):603
Horton RK (1965) An index number system for rating water quality. J Water Pollut Control Fed 37:300–306
Howladar MF, Al Numanbakth MA, Faruque MO (2018) An application of Water Quality Index (WQI) and multivariate statistics to evaluate the water quality around Maddhapara Granite Mining Industrial Area, Dinajpur, Bangladesh. Environ Syst Res 6:1–18
Jain CK, Singh S (2020) Impact of climate change on the hydrological dynamics of River Ganga, India. J Water Clim Chang 11(1):274–290
Jean Milien E, Nunes GM, Pierre G, Hamilton SK, Da Cunha CN (2023) Hydrological dynamics of the Pantanal, a large tropical floodplain in Brazil, revealed by analysis of Sentinel-2 satellite imagery. Water 15(12):2180
Jiménez-Navarro IC, Jimeno-Sáez P, López-Ballesteros A, Pérez-Sánchez J, Senent-Aparicio J (2021) Impact of climate change on the hydrology of the forested watershed that drains to Lake Erken in Sweden: an analysis using SWAT+ and CMIP6 scenarios. Forests 12(12):1803
Madhusudana Rao C, Bardhan A, Patra JP (2020) Assessment of hydrological response in Subarnarekha River basin under anticipated climate change scenarios. Global Nest J 22:207–219
Malede DA, Alamirew T, Andualem TG (2022) Integrated and individual impacts of land use land cover and climate changes on hydrological flows over Birr River Watershed, Abbay Basin, Ethiopia. Water 15(1):166
Mandal U, Sena DR, Dhar A, Panda SN, Adhikary PP, Mishra PK (2021) Assessment of climate change and its impact on hydrological regimes and biomass yield of a tropical river basin. Ecol Indic 126:107646
Marselina M, Wibowo F, Mushfiroh A (2022) Water quality index assessment methods for surface water: a case study of the Citarum River in Indonesia. Heliyon 8(7):e09848
Muhammed HH, Nasidi NM, Wayayok A (2022) Impact of climate changes and landuse/land cover changes on water resources in Malaysia. In: Environmental Degradation in Asia (pp 465–483). Springer, Cham. https://doi.org/10.1007/978-3-031-12112-8_21
Nguyen TG, Huynh THN (2022) Assessment of surface water quality and monitoring in southern Vietnam using multicriteria statistical approaches. Sustain Environ Res 32(1):1–12
Nikam BR, Aggarwal SP, Thakur PK, Garg V, Roy S, Chouksey A, Dhote PR, Chauhan P (2020) Assessment of early season agricultural drought using remote sensing. Int Arch Photogramm Remote Sens Spat Inf Sci 43:1691–1695
Nordin NH, Rahman AHA, Wahab NA, Sembok TM b T, Shukran MA b M, Yunus MSFM, Isnan S, Shukur MRAABA (2022) Monitoring of mangroves changes in Pulau Kukup using geographical information system (GIS). IOP Conf Ser Earth Environ Sci 1064(1):012009
Oad VK, Szymkiewicz A, Khan NA, Ashraf S, Nawaz R, Elnashar A, Saad S, Qureshi AH (2023) Time series analysis and impact assessment of the temperature changes on the vegetation and the water availability: a case study of Bakun-Murum Catchment Region in Malaysia. Remote Sens Applications: Society and Environment 29:100915
Sadhwani K, Eldho TI, Karmakar S (2023) Investigating the influence of future landuse and climate change on hydrological regime of a humid tropical river basin. Environ Earth Sci 82:210. https://doi.org/10.1007/s12665-023-10891-6
Santy S, Mujumdar P, Bala G (2020) Potential impacts of climate and land use change on the water quality of Ganga River around the industrialized Kanpur Region. Sci Rep 10:9107. https://doi.org/10.1038/s41598-020-66171-x
Shaikh TA, Adjovu GE, Stephen H, Ahmad S (2023) Impacts of urbanization on watershed hydrology and runoff water quality of a watershed: a review. In: Proceedings of the World Environmental and Water Resources Congress 2023, vol 1. ASCE, Henderson, NV, USA, pp 1271–1283. Available online: https://ascelibrary.org/doi/10.1061/9780784484852.116
Shukla PR, Skea J, Calvo Buendia E, Masson-Delmotte V, Pörtner H-O, Roberts DC, Zhai P, Slade R, Connors S, van Diemen R et al (2019) Climate change and land: an IPCC special report on climate change, desertification, land degradation, sustainable land management, food security, and greenhouse gas fluxes in terrestrial ecosystems; intergovernmental panel on climate change (IPCC): Geneva, Switzerland
Singh S, Kansal ML (2022) Chamoli flash-flood mapping and evaluation with a supervised classifier and NDWI thresholding using Sentinel-2 optical data in Google earth engine. Earth Science Informatics 15(2):1073–1086
Syeed MM, Hossain MS, Karim MR, Uddin MF, Hasan M, Khan RH (2023) Surface water quality profiling using the water quality index, pollution index and statistical methods: a critical review. Environ. Sustain Indicat 18:100247. https://doi.org/10.1016/j.indic.2023.100247
Taşan M, Demir Y, Taşan S (2022) Groundwater quality assessment using principal component analysis and hierarchical cluster analysis in Alaçam, Turkey. Water Supply 22(3):3431–3447
Tsegaye L, Bharti R (2022) The impacts of LULC and climate change scenarios on the hydrology and sediment yield of Rib watershed, Ethiopia. Environ Monit Assess 194(10):717
Uddin MG, Nash S, Olbert AI (2021) A review of water quality index models and their use for assessing surface water quality. Ecol Indic 122:107218
Uddin MG, Nash S, Rahman A, Olbert AI (2023) Performance analysis of the water quality index model for predicting water state using machine learning techniques. Process Saf Environ Prot 169:808–828
Varol M, Karakaya G, Alpaslan K (2022) Water quality assessment of the Karasu River (Turkey) using various indices, multivariate statistics and APCS-MLR model. Chemosphere 308:136415
Wang M, Shao Y, Jiang QO, Xiao L, Yan H, Gao X, Wang L, Liu P (2020) Impacts of climate change and human activity on the runoff changes in the Guishui River Basin. Land 9(9):291
Wu H, Yang W, Yao R, Zhao Y, Zhao Y, Zhang Y, Yuan Q, Lin A (2020) Evaluating surface water quality using water quality index in Beiyun River, China. Environ Sci Pollut Res 27:35449–35458
Yu R, Asif Ikbal M, Rahman A (2021) Improvement of substation monitoring aimed to improve its efficiency with the help of big data analysis. J Intell Syst 30(1):499–510
Acknowledgements
I would like to express my profound gratitude to my project guide, Dr. Neeta Kumari, for her guidance and support during my work. I convey my sincere gratitude to Dr. Bindhu Lal, Head, Dept. of Civil and Environmental Engineering, BIT, Mesra, Ranchi, for providing me various facilities needed to complete my project work. Finally, I must express my very profound gratitude to my parents for providing me with unfailing support and continuous encouragement throughout the years of my study. My apologies and heartful gratitude to all those who have assisted me yet have not been acknowledged by name.
Author information
Authors and Affiliations
Contributions
Kiran Prakash Kadave: concept, design, data analysis, material preparation, data validation and interpretation and drafting the manuscript; Dr. Neeta Kumari: supervising, drafting, correction, concept, processing, analysis and interpretation.
Corresponding author
Ethics declarations
Ethical approval
Ethical approval and informed consent are not applicable for this study.
Consent to participate
Not applicable.
Consent for publication
All the authors have consented to publish the work.
Competing interests
The authors declare no competing interests.
Additional information
Responsible Editor: Philippe Garrigues
Publisher’s Note
Springer Nature remains neutral with regard to jurisdictional claims in published maps and institutional affiliations.
Supplementary information
ESM 1
(DOCX 450 kb)
Rights and permissions
Springer Nature or its licensor (e.g. a society or other partner) holds exclusive rights to this article under a publishing agreement with the author(s) or other rightsholder(s); author self-archiving of the accepted manuscript version of this article is solely governed by the terms of such publishing agreement and applicable law.
About this article
Cite this article
Kadave, K.P., Kumari, N. Assessment of seasonal water quality and land use land cover change in Subarnarekha watershed of Ranchi stretch in Jharkhand. Environ Sci Pollut Res (2023). https://doi.org/10.1007/s11356-023-30979-7
Received:
Accepted:
Published:
DOI: https://doi.org/10.1007/s11356-023-30979-7