Abstract
This study investigates the impact of information and communication technology (ICT) on carbon dioxide emissions for a panel of 91 countries over the period 1990 to 2017. The study constructs an ICT index through principal component analysis and tests for the presence of cross-sectional dependence (CSD) in the data. The study employs pooled ordinary least squares, fixed-effects model, and system-generalized method of moments estimation techniques with panel-corrected standard errors (PCSE) to tackle the issues of CSD in the data. The findings of the study show that ICT reduces CO2 emissions for the full sample of countries. However, the comparative study of developed and developing countries depicts that ICT encourages environmental sustainability in developed countries whereas opposite results are found for developing countries. Moreover, presence of the environmental Kuznets curve is confirmed for the full sample as well as for developed and developing countries. It suggests that with higher levels of development of a country, it would be possible to contribute towards environmental sustainability along with ICT diffusion. Therefore, the outcome of this study may be helpful for policymaker and policies may be designed to encourage ICT investments in developing countries, as ICT will take care of environmental sustainability with higher levels of development.
Similar content being viewed by others
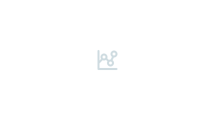
Explore related subjects
Discover the latest articles, news and stories from top researchers in related subjects.Avoid common mistakes on your manuscript.
Introduction
Over the past three decades, significant diffusion of information and communication technology (ICT) has boosted productivity and enhanced energy efficiency in the greater parts of the economies. The research has largely documented these potential benefits of ICT around the world. However, little attention has been paid to the environmental consequences of ICT. And so, the relationship between ICT and environmental sustainability is still inconclusive (Khan 2019). Some studies found that rapid growth of ICT has positively affected environment by mitigating carbon dioxide (CO2) emissions, whereas some other studies provided the evidence that greater usage of ICT appliances has caused environmental deterioration. Also, the existing literature shows heterogeneous consequences of ICT for CO2 emissions in the developed and developing countries (Al-Mulali et al. 2015; Higon et al. 2017). Since, environment is an important global issue, therefore, this study conducts a comparative analysis of the developed and developing countries at a global level to understand the relationship between ICT and CO2 emissions.
The term ICT includes all those appliances and communication devices (for instance, radio, television, mobile phone, computer, and satellite system) which assist the users to access, transmit, or store information (Salahuddin et al. 2016b; Pradhan et al. 2018). Thus, ICT users have much better access to the information than before in terms of speed, scale, and scope (Erdmann and Hilty 2010). It is believed that ICT infrastructure improves service delivery, promotes transparency, and encourages interaction between government and citizens (Sabri et al. 2012; Lu 2018). Likewise, ICT diffusion has substantially reduced production costs, enhanced the efficiency of resources allocation, and stimulated much greater investment in different sectors of the economies (Pradhan et al. 2018).
However, ICT infrastructure has led to higher electricity consumption which is one of the key reasons of global CO2 emissions. It has been estimated that ICT sector has caused higher electricity consumption globally from 3.9% in 2007 to 4.6% in 2012 (Salahuddin et al. 2016b; Ejemeyovwi et al. 2019). Usually, electricity is generated by the combustion of fossil fuels whereas production, transportation, and combustion of fossil fuels (oil, natural gas, and coal) have substantial environmental impacts. Notably, CO2 emissions from the use of fossil fuels have increased about 1.9% per year over the last three decades (International Energy Agency 2009). Therefore, it is argued that rapid growth of ICT sector may have caused environmental degradation in terms of higher CO2 emissions (Lee and Brahmasrene 2014).
Conversely, the United Nations Climate Change Conference (2015) stated that ICT could diminish 20% of CO2 pollution yearly by 2030 because of efficiency in production (Lu 2018). It is believed that ICT can reduce CO2 emissions by developing efficient transportation systems, building smarter cities, and introducing industrial processes (Akande et al. 2019). Similarly, ICT can improve environmental quality by introducing different approaches such as dematerialization and demobilization. Therefore, a casual examination may suggest that ICT improves energy efficiency, and thus contributes to the reduction of CO2 emissions (Haseeb et al. 2019).
It suggests that the relationship between ICT and environment is complicated, as it has negative as well as positive effects on the quality of environment (Houghton 2010). Although, several empirical works have been conducted to investigate this relationship yet, the net impact of ICT on environmental sustainability is ambiguous as the results of the existing studies typically depend on the choice of time period, sample of the countries analyzed, and the econometric approach adopted.
The existing ICT literature mostly focuses on developed countries and largely ignores developing countries (Salahuddin et al. 2016b; Akande et al. 2019). Moreover, in the previous studies, ICT is generally measured with a single dimension (i.e., internet usage and mobile phones) which cannot be generalized for other dimensions of ICT (Al-Mulali et al. 2015; Salahuddin et al. 2016a). Furthermore, the empirical studies usually ignore the potential problem of endogeneity of the variables (Higon et al. 2017). Lastly, ICT literature typically does not pay attention to the existence of cross-sectional dependence (CSD) in the data (Dabbous 2018; Ng et al. 2020). However, ignoring CSD may cause loss of efficiency in the estimator and may provide biased estimates. Therefore, it is argued that results of those studies which are based on the assumptions of no endogeneity and cross-sectional independence should be interpreted with caution.
These gaps in the existing ICT literature provide us the motivation of this study. The study conducts a comparative analysis of developed and developing countries to investigate the impact of ICT on CO2 emissions at a global level. The study includes all those countries in the analysis for which data is available over the period 1990 to 2017.The study constructs an ICT index through principal component analysis (PCA) and tests for the existence of cross-sectional dependence in the data. The study takes into account CSD problem and employs pooled ordinary least squares (POLS), fixed-effects model (FEM), and system-generalized method of moments (SGMM) estimation techniques with panel-corrected standard errors (PCSE) to tackle the issues of CSD in the data (Beck and Katz 1995; Driscoll and Kraay 1998; Hoechle 2007; Reed and Ye 2009). It is assumed that PCSE estimator can tackle the issue of CSD and produce robust estimation results.
This study contributes to the emerging literature on ICT and environmental sustainability conundrum by conducting a comparative analysis of the impact of ICT on CO2 emissions for a large number of developed and developing countries. In addition, the study constructs an ICT index through principal component analysis rather than confining the analysis to a single indicator of ICT. Furthermore, the study addresses the cross-sectional dependence problem and employs estimators with Driscoll and Kraay standard errors (PCSE) which are assumed to address cross-sectional dependence and deliver heteroskedasticity and autocorrelation-consistent standard errors with unbiased estimates.
The outcome of this study would be helpful for policymaker and they can design ICT investment policies according to the findings of this study. The findings of this study would suggest whether all economies can take the advantage of “greening through ICT” or for some countries protection is needed from the adverse environmental consequences of ICT. Specifically, findings of this study would be helpful in determining environmental consequences of ICT for developed and developing countries.
The structure of the study is as follows: the “Literature review” section reviews literature on the relationship between ICT and environmental sustainability while the “Methodology” section provides the methodological framework for the analysis. The “Data and variable description” section describes the data and variables while the “Empirical results and discussion” section discusses the estimation results of the study. Finally, the “Conclusion” section concludes the study and suggests some policy implications.
Literature review
The higher levels of CO2 emissions are the main sustainability challenge and risk to our welfare and existence nowadays (Ben-Jebli et al. 2016). It is argued that while ICT revolution has led to a new era of economic growth among countries, nonetheless, it has contributed to today’s well-known phenomenon of global warming caused by higher CO2 emissions. According to the International Energy Agency (IEA 2009), ICT sector annually contributes to global CO2 emission by 2% while ICT products roughly consume 15% of worldwide private power utilization. Hence, ICT diffusion is an important factor while focusing on the quality of environment.
However, in this area, the work is typically done as an extension of the environmental Kuznets curve (EKC). In the early 1990s, Grossman and Krueger (1991) found the evidence that environmental quality is an inverted U-shaped function of per capita income. It suggests that environmental quality degrades in the early stages of economic growth, but beyond some higher per capita income level, the trend reverses.
There are three explanatory reasons behind the shape of EKC (Grossman and Krueger 1991; Panayotou 1997; Dinda 2004). It includes scale effect, composition effect, and technique effect. The scale effect explains that economic growth negatively affects quality of environment because larger scale of economic activities needs more energy and produces higher levels of emissions. Secondly, composition effect explains that economic growth improves quality of environment because economy transforms from manufacturing to services-based economy where the share of cleaner activities increases. Lastly, technique effect lets the countries to grow and substitute the old and dirty technology with new and environment-friendly technology. If technology leads to efficient allocation of resources, then pollution decreases even if the output of the nation increases. Accordingly, an inverted U-shaped EKC is witnessed as a combination of composition and technique effects overcoming the scale effect.
The EKC hypothesis is one of the most widely tested hypotheses since the 2000s. According to the web of science statistics, there are more than three thousand published articles and almost eighty thousand citations for EKC hypothesis (Dogan et al. 2020). Nonetheless, EKC literature provides inconsistent evidence about the existence of EKC. Ben-Jebli et al. (2016) proved the existence of inverted U-shaped EKC hypothesis for 25 OECD countries over the period 1980 to 2010. In contrast, Nuroglu and Kunst (2018) revealed that their data did not support EKC hypothesis for developing countries for the time span of 1960 to 2011, while Ng et al. (2020) lent moderate support to the EKC hypothesis for a panel of 76 countries over the period 1971to 2014.
In EKC literature, CO2 emissions are typically employed as a proxy for environmental degradation; however, different proxies are available for environmental degradation. For example, Almeida et al. (2017) reinvestigated EKC hypothesis for a panel of 152 countries; however, they used a composite index of ecological indicators, instead of CO2 emissions, to measure environmental degradation. The study could not prove EKC hypothesis and concluded that economic growth alone is not enough to improve environmental quality. On the other hand, Ulucak and Bilgili (2018) employed ecological footprint (EF) variable and verified inverted U-shaped relationship between economic growth and EF for a panel of 45 countries.Footnote 1 In a recent study, Fernández-Amador et al. (2020) analyzed the impacts of economic growth on per capita methane emissions for a sample of 66 countries. The study concluded that economic growth has substantially increased per capita methane emission in their sample.
The literature focusing on EKC hypothesis provides contradictory evidence about the existence of EKC (Dinda 2004; Farhani and Ozturk 2015; Ben-Jebli et al. 2016; Ng et al. 2020). In view of that, Halicioglu (2009) emphasized that a complex relationship exists between per capita income and environmental degradation and therefore some additional factor besides per capita income may have an important role in the occurrence of the downward sloping part of the EKC. It is usually argued that ICT may be included as a potential variable to explain the existence of EKC phenomenon.
The ICT sector is a power drainer sector and its carbon emissions were equal to 830MtCO2e in 2007. Notably, this amount of carbon emissions is just equal to the carbon emissions emitted by the air industry in 2007 (Uddin 2011). In addition, carbon emissions of ICT sector are expected to increase on an average of 6% each year up to 2020 (Salahuddin et al. 2016b). In this situation, aggregation of different ecological impacts (i.e., composite index of ecological indicators and ecological footprint) may simplify the impact of carbon emissions (Moffatt 2000). Therefore, domain-specific indicators are usually suggested to focus on various ecosystem components, separately (Galli 2015). Accordingly, CO2 is generally used as a proxy for environmental deterioration in the ICT literature.
Following this line of argument, Lee and Brahmasrene (2014) examined the relationship between ICT and CO2 for a panel of Association of South East Asian Nations (ASEAN). The study established that ICT significantly increased CO2 emissions for the selected sample over the period 1991 to 2009. Likewise, Haseeb et al. (2019) using panel data of BRICS economies over the period 1994 to 2014 found that internet usage and mobile cellular subscriptions have adversely impacted CO2 emissions; however, electricity consumption has positively affected CO2 emissions in the BRICS economies.
On the other hand, Al-Mulali et al. (2015) investigated the influence of internet retailing on CO2 emissions for a sample of 77 countries and found that internet retailing significantly impacted CO2 emissions only in the developed countries. Similarly, Higon et al. (2017) concluded that ICT expansion negatively affects carbon emissions in the developed countries, while the opposite is true for the developing countries. Ozcan and Apergis (2018), and Tsaurai and Chimbo (2019) suggested that ICT investments contributed to the reduction in air pollution in the emerging markets.
It follows from the discussion that ICT has both positive and negative implications for sustainability (Higon et al. 2017; Dabbous 2018; Haseeb et al. 2019). The growth of ICT sector is believed to mitigate CO2 emission as ICT optimizes production processes, improves energy efficiency, reduces emission intensity, develops transportation systems, and builds smarter cities (Akande et al. 2019). It suggests positive role of ICT infrastructure while leading to economic growth (Salahuddin et al. 2016b; Ejemeyovwi et al. 2019). However, as the economy develops, demand for and supply of ICT products increases and exerts pressure on energy requirement (Dabbous 2018). Therefore, electricity consumption and production increases which is one of the main causes of CO2 emissions. It suggests that ICT production, use, and disposal have negative environmental consequences while leading to economic growth.
In this context, a three-order typology has been widely discussed in the ICT literature that clearly identifies the impact mechanism of ICT for CO2 emissions (Erdmann and Hilty 2010; Houghton 2010; Khan 2019). It includes first-order (direct use effects), second-order (indirect substitution effects), and third-order (rebound effects) impacts of ICT (Fig. 1).
Impact mechanism of ICT for CO2 emissions. Source: Adapted from Erdmann and Hilty (2010). Dotted arrows refer to the influence of economic, political, and cultural variables while thin solid arrows show first-order impacts of ICT. Likewise, medium solid arrows indicate second-order impacts of ICT whereas thick arrows signify third-order impacts of ICT
The first-order use impacts of ICT signify that energy consumption intensifies during the production, processing, distribution, and installation of ICT infrastructure. These effects are related with the life cycle of ICT products which spans over production, delivery, transportation, usage, and disposal of ICT products. A recent assessment suggests that the direct impacts of ICT infrastructure are expected to be around 2 to 3% of global CO2 emissions (Khan 2019).
The second-order substitution effects facilitate transition towards sustainable patterns of production and consumption. These effects include dematerialization, demobilization, and finally decarbonization. Dematerialization replaces physical books with e-books, post mail with e-mail, and newspaper to electronic paper to minimize waste materials (Akande et al. 2019). Likewise, demobilization reduces outdoor activities and saves fossil fuel consumed in the vehicles and results in fewer carbon emissions. It encourages video conferences rather than traveling and supports working at home instead of going to the workplaces. The substitution effects assist in optimizing production processes, improving energy efficiency and realizing decarbonization. It is further assumed that the indirect impacts of ICT are greater than the direct impacts of ICT and therefore, there are net positive impacts of ICT (Houghton 2010).
The third-order rebound impacts of ICT refer to the effects where additional demand is stimulated for the resources which are being used efficiently (i.e., greater use of more energy efficient transport system). A standard third-order rebound effect (enabled by the direct or indirect use of ICT) represents the situation where an increase in efficiency (substitution effect) is likely to reduce environmental effects of an activity, but additional demand compensates for the reduction. These potential rebound impacts are realized through the impacts on life style, value system, and cultural framework. It is argued that rebound effects are likely to be greater in developing countries where energy demand is usually unsatisfied.
From the above discussion, it can be concluded that ICT has heterogeneous environmental consequences. Therefore, Houghton (2009) suggests that the role of ICT in the mitigation of environment is not easy to cope with and a detailed investigation is needed to know exactly about the relationship between ICT and CO2 emissions.
Methodology
Following Grossman and Krueger (1991), we model the environmental Kuznets curve (EKC) as follows:
Here, CO2 shows carbon dioxide emissions and it is used as a proxy for environmental degradation. The GDPC is GDP per capita while GDPC2 shows square of GDP per capita. In Eq. (1) i stands for country and t is time period; lastly, εit is an error term. In EKC framework, it is assumed that environmental degradation depends on the level of GDP per capita and it exhibits inverted U-shaped pattern. However, some other factors may also play important roles in environmental degradation, for example, ICT. Hence, the relationship between environmental degradation and GDP per capita is extended as follows:
According to the literature, ICT causes diverse effects on the quality of environment. It is submitted that investment in ICT infrastructure can be environment-friendly if ICT increases energy efficiency but if it increases energy use, it can deteriorate the quality of the environment (Dabbous 2018; Haseeb et al. 2019). Therefore, ICT may positively as well as negatively affect the quality of environment depending on energy consumption (EC). Therefore, we include energy consumption in our EKC model.
Similarly, another important variable is trade openness which involves the transfer of goods manufactured from one country to another either for consumption or for further handling. It suggests that pollution may also be created in the production of goods which are exported to other countries. Wyckoff and Roop (1994) concluded that 13% of the overall CO2 emissions of the six largest OECD countries are related to their imports of industrial goods. Hence, trade openness (TO) is also related with CO2 emissions as follows:
Equation (4) explains the specified model for the panel data analysis. In this study, all the variables, except information and communication technology index, are converted into their natural logarithmic to obtain direct elasticities (Shahbaz et al. 2013).
The expected sign of β1 is positive which shows that carbon dioxide emissions increase with increase in per capita income due to scale effect. In contrast, the expected sign of β2 is negative which shows that after a threshold level, further increase in per capita income leads to a decline in CO2 emissions because of environmental friendly technological advancement and composition effects. In that case, the other two effects are stronger than scale effect (Grossman and Krueger 1991). However, environmental impacts of ICT are not clear a priori and the sign of the coefficient of ICT (β3) may be positive or negative (Haseeb et al. 2019). The expected coefficient sign of control variable energy use (β4) is positive; however, the expected sign of β5 (coefficient of trade openness) may be positive or negative as trade openness has different impacts for developed and developing countries (Guo et al. 2018).
In this study, panel data are to be analyzed due to its flexible nature in the estimation process. However, the study tests for cross-sectional dependence (CSD) and employs pooled ordinary least squares (POLS), fixed-effects model (FEM), and system-generalized method of moments (SGMM) estimation techniques with panel-corrected standard errors (Driscoll & Kraay standard errors) to tackle the issue of cross-sectional dependence in the data (Beck and Katz 1995; Driscoll and Kraay 1998; Hoechle 2007; Reed and Ye 2009). The POLS and FEM/REM are the most widely used estimation techniques in EKC literature while SGMM addresses the endogeneity issue, whereas the estimators with panel-corrected standard errors handle cross-sectional dependence in the data and yield heteroskedasticity as well as autocorrelation-consistent standard errors. Moreover, estimation techniques with PCSE provide robust estimation results.
The study includes a large number of countries with different income levels; therefore, FEM has been employed as it estimates the influence of variables that changes over time. FEM deals with the differences in the intercept so that intercept changes for each cross-section but slope remains the same. Moreover, the fixed-effect model introduces fixed dummies to capture the differences among countries. However, in the presences of cross-sectional dependence, FEM estimator is although consistent but not efficient. Moreover, the estimated standard errors are biased. Therefore, applying FEM under cross-sectional dependence of errors may perhaps deliver inconsistent estimates and misleading information.
Cross-sectional dependence has recently received significant attention. The existence of CSD cannot be denied in the modern economy due to higher economic, financial, and trade integration. Particularly, CSD cannot be ignored in the backdrop of shared global shocks (i.e., financial and oil crises), shared global institutions (i.e., International Monetary Fund), and shared global spillover impacts among the countries. Usually, all these factors are not included into our regression models and consequently cross-section interdependence may display in the residuals.
It is argued that CSD is an important problem in panel data and ignoring CSD in the estimation may cause loss of efficiency in the estimator and may provide invalid test statistics. Nonetheless, most common panel data estimators are based on the assumption of cross-sectional independence and are unable to handle this serious issue.Footnote 2 However, the assumption of cross-sectional independence may be considered inappropriate for panel data analysis (Lu 2018; Ng et al. 2020).
For that reason, cross-sectional dependence tests have been suggested to be able to determine the presence of CSD in the data. Our study conducts Breusch and Pagan Lagrange multiplier (LM); Pesaran-scaled LM and Pesaran CD (2004) tests for each model (Dabbous 2018; Ulucak and Bilgili 2018; Lu 2018; Ng et al. 2020). If the null hypothesis of no cross-section dependence is rejected for a model, it suggests that the model is cross-sectionally dependent.
In the presence of CSD, one option could be feasible generalized least squares (FGLS) estimate but it underestimates standard errors in the finite samples (Parks 1967; Reed and Ye 2009). Moreover, FGLS can be applied when the number of time periods (T) is greater than or equal to the number of cross-sections (N). Instead, when number of cross-sections is greater than the number of time periods (N > T), then panel-corrected standard errors (PCSE) estimation performs significantly better than FGLS estimator (Reed and Ye 2009). The PCSE estimator is widely recognized in handling CSD problem and it has opened up a variety of choices for panel data estimation (Hoechle 2007).
Therefore, the present study employs POLS, FEM, and SGMM estimation techniques with panel-corrected standard errors to obtain robust results. The standard errors estimated as panel-corrected standard errors resolve that the covariance matrix estimator is consistent, independent of the cross-sectional dimension (Hoechle 2007). Moreover, robust estimation results are produced.
Data and variable description
This study investigates the impact of ICT on carbon dioxide emissions for a panel of 91 countries over the period 1990 to 2017.The primary objective of this study is to conduct a comparative analysis of environmental impacts of ICT for the developed and developing countries. Therefore, the full sample is further categorized into 30 developed and 61 developing countries according to the World Bank classification (Higon et al. 2017; WDI 2018).
The threshold variable selected for the categorization of developed and developing countries is gross national income per capita (GNIP) in current US dollars in 2017.The World Bank annually estimates the size of economies in terms of gross national income per capita and classifies countries into four groups. The low-income countries are those with a GNIP of 995 US dollars or less in 2017. Likewise, lower middle-income and upper middle-income countries are those countries having GNIP between 996 to 3895 US dollars and between 3896 to 12,055 US dollars, respectively. The low- and middle-income groups taken together are usually referred to as developing countries (LDCs) while the countries having GNIP greater than 12,055 US dollars are categorized as high-income or developed countries (DCs).These income groups are associated with an annually updated threshold level of GNIP provided by the World Bank. The study has included 91 countries in its empirical analysis on the basis of data availability for the given time period.
Table 1 provides the description, data sources, and definitions of the variables which are being used in our present analysis.
The data for all the variables have been taken from the World Development Indicators (WDI 2018). However, an index of ICT is constructed from four sub-components of ICT through principal component analysis (PCA) to avoid multicollinearity problem (Dabbous 2018), whereas the sub-components of ICT are internet users, mobile subscriptions, telephone subscriptions, and fixed broadband subscriptions (Lee and Brahmasrene 2014; Lu 2018).
Principal component analysis is a technique (based on a strong statistical basis) that reduces the dimensionality of a dataset, increases its interpretability, but at the same time minimizes information loss. The PCA is performed by creating new uncorrelated variables, yet, holding the variation in the data up to maximum level. It is based on the assumption that the data is standardized and linear relationships exist between the variables. PCA is considered an appropriate method and it has been adopted widely in the past ICT studies (Dabbous 2018; Akande et al. 2019).
Before performing PCA, we tested the linear relationship between all the variables using Pearson correlation coefficients. And then we computed eigenvectors and eigenvalues for the identification of principal component. After identification of principal component, we constructed an ICT index from four indicators of ICT. Table 8 in the appendix shows computed eigenvectors for the four ICT variables.
The study also tested for the sampling adequacy of our dataset using the Kaiser-Meyer-Olkin (KMO) measure of sampling adequacy (Akande et al. 2019). The overall value of KMO for the dataset is 0.69, which indicates that our PCA leads to consistent results. Moreover, we performed Bartlett’s test of sphericity to test the suitability of our data for reduction (Cruz-Jesus et al. 2017). This test gave us a p value less than 0.05 making it significant. Table 9 in the appendix provides details of these tests.
Empirical results and discussion
The study conducts variance inflation factor (VIF) tests for multicollinearity and reports results in Table 2. The results shows that average VIF is less than 5 implying that there is no threat of multicollinearity in our models (Salahuddin et al. 2016a; Majeed and Khan 2019).
Likewise, the study tests for cross-sectional dependence and conducts Breusch and Pagan Lagrange multiplier (LM) test, Pesaran-scaled LM, and Pesaran CD (2004) tests for developed countries, developing countries, and for the full sample of 91 countries (Ulucak and Bilgili 2018; Ng et al. 2020). Table 3 tabulates CSD tests results.
The CSD test reported in Table 3 strongly rejects the null hypothesis of no cross-sectional dependence (Lu 2018). It suggests the existence of cross-sectional dependence in our panel data. So CSD problem should be handled to obtain robust estimation results.
The study also applies Hausman test to select whether REM or FEM is more appropriate. Hausman (1978) introduced a test which specified the best model between REM and FEM. The results of Hausman tests are reported in Table 4.
According to Hausman test, if the p value of test is less than 0.05, then we reject the null hypothesis and accept the alternate hypothesis that fixed-effect model is more suitable and applicable. Therefore, on the basis of Hausman test results, the choice has been made between REM and FEM for the developed countries, developing countries, and for the full sample and FEM is preferred in all our models.
The study employs POLS, FEM, and SGMM panel estimation techniques; however, it conducts estimations with panel-corrected standard errors to handle CSD in our data. Table 5 reports the panel estimation results for the developed countries (column 1 to 3), developing countries (column 4 to 6), and for the full sample (column 7 to 9), respectively.
Table 5 reports that GDP per capita (GDPC) significantly and positively affects CO2 emissions in developed countries, developing countries, and in the full sample of 91 countries. Although, GDPC reflects the ability of a nation to produce more goods and services, it accelerates emissions of CO2 and causes environmental degradation. It can be explained with the help of scale effect that shows that larger scale of economic activities needs more energy and produces higher levels of emissions that negatively affect quality of environment (Grossman and Krueger 1991; Dinda 2004). Moreover, this result possibly signifies that production of more goods is possible with the growth of industrial sector that leads to the use of more fossil fuels and emissions of CO2 (Sohag et al. 2017). It means growth of industrial sector may result in significant environmental unsustainability due to air pollution. So the general idea about the negative environmental effect of growth is clearly confirmed across countries. These findings suggest that of GDPC contributes to environmental degradation (Ben-Jebli et al. 2016; Lu 2018).
The coefficient of GDP per capita is positive while its squared term is significantly negative for all income groups. It suggests that an economy emits low levels of CO2 after achieving certain threshold level of GDP per capita (Grossman and Krueger 1991). It may be because of the technique effect that shows that adoption of advanced eco-friendly technologies leads to the production of higher levels of output with lower levels of CO2 emissions. If the technology leads to efficient allocation of resources, then pollution decreases even if the output of a nation increases (Dinda 2004).
Moreover, it may be because of sectoral transformation. The economies usually shift from the primary agricultural sector to the energy-led industrial sector in the initial stages of growth and emit higher levels of CO2. But with higher levels of development, the economies generally shift towards service sector which produces less pollution as compared with the industrial sector that is more energy intensive (Sohag et al. 2017).
Nonetheless, presence of the environmental Kuznets curve is confirmed for all income groups in our sample (Grossman and Krueger 1991; Panayotou 1997; Dinda 2004; Ben-Jebli et al. 2016; Ng et al. 2020).
It is evident from Table 5 that coefficient of ICT is negatively significant for the developed countries with alternative estimation techniques (column 1 to 3). If diffusion of ICT leads to efficiency in the production, then environmental sustainability can be maintained even if output of a nation increases (Ozcan and Apergis 2018). This negative impact of ICT for CO2 emissions is witnessed as a combination of output, input, and technology effects of ICT overcoming the scale effect of ICT (Higon et al. 2017; Khan 2019). Hence, ICT contributes to the reduction of CO2 emissions and positively impacts environmental quality in developed countries. It also suggests that ICT is capable of decoupling growth from environmental deterioration (Plepys 2002).
In contrast, ICT has not taken such a status in developing countries to cope with environmental pollution (Al-Mulali et al. 2015). Likewise, when we take care of endogeneity issue by employing (SGMM), the results show that ICT has positive but insignificant impact on CO2 emissions.Footnote 3 This result possibly signifies that technological advancement is not enough in developing countries to decline CO2 emissions through ICT. This finding also infers that scale effect of ICT has an important role in explaining environmental degradation in the developing countries (Higon et al. 2017). Furthermore, it implies that ICT production, use, and disposal have negative environmental consequences through increased CO2 emissions in the developing countries.
However, the results also show that ICT declines CO2 emissions for the full sample of 91 countries (column 7 to 9). Moreover, an inverted U-shaped EKC in the presence of ICT illustrates that composition and technology effects are the leading effects in our analysis (Grossman and Krueger 1991). Furthermore, the result obtained from SGMM is consistent with the result obtained from the fixed-effect model. It implies that with an increase in ICT infrastructure, the energy becomes more efficient and produces less emission as advanced technology is usually eco-friendly and ICT plays a significant role in mitigating CO2 emissions (Salahuddin et al. 2016a; Lu 2018).
Since the industrial revolution, the worldwide economies have been built on fossil fuels, which are burnt to fuel economic growth. Consequently, the combustion of fossil fuels has also become the main cause of greenhouse gas emissions and it has been the view that higher energy consumption causes higher environmental deprivation. Table 5 reports positive significant relationship between energy consumption and CO2 emissions (Shahbaz et al. 2013). Similarly, Guo et al. (2018) also revealed that some key industries in China not only consumed a lot of fossil energy and released a lot of carbon dioxide, but also stimulated energy consumption and CO2 emissions in other industries in China.
The energy consumptions contribute to emissions in both DCs and LDCs but the value of contributions in emission is small for DCs as compared with LDCs. This is due to the efficiency enhanced by the developed countries through advanced technology which increases energy efficiency and less amount of energy is used as input in the production processes. Moreover, in the developed nation, the energy-intensive industries are changing into less energy-intensive sectors like service sectors. Furthermore, policies regarding energy are strong enough to protect the environment; hence, energy causes the least amount of emissions in developed countries. Whereas the developing countries are engaged in old and worn-out processes of productions that need more energy and hence cause deprivation of environment. To understand the level of emission of CO2, it is important to consider, for example, the size of economy, state of industrialization, distances for freight transportation, domestic and export markets, and dependence on coal to generate electricity (Leal et al. 2019).
Finally, the role of trade openness in CO2 emission could not be established as trade openness exhibits heterogeneous results for developed and developing countries. The study finds a positive and statistically significant relationship between trade openness and emissions of CO2 for developing countries; however, it is negative for developed countries. The literature focusing on the relationship between environmental quality and trade openness highlights the idea that developing countries have a lower degree of specialization in physical and human capital. Therefore, trade openness may result in increased pollution in LDCs due to the increased production of emission-intensive goods for export to developed nations (Nguyen et al. 2017).
In addition, it may be because of dirty technologies in LDCs which may lead to an increase in environmental degradation. Farhani and Ozturk (2015) highlights environmental effects of trade openness. It includes scale effect (size of the economy), technique effect (the use of environment friendly techniques of production), and composition effects (specialization and comparative advantage of the country).The study finds positive relationship between trade openness and pollution emissions for the developing countries. The study discusses that due to dirty techniques of production under weaker environmental regulations in developing countries, higher trade openness leads to higher levels of pollution emissions. Furthermore, LDCs mostly suffer from unstable intuitions and weak policy implementations; therefore, international trade harms the environment in developing countries.
However, trade activities are less emission intensive in developed countries due to the fact that developed economies have strong policies and regulatory authorities regarding trade. Therefore, trade openness is beneficial for the environment in these countries (Antweiler et al. 2001; Ben-Jebli et al. 2016).
This discussion suggests that trade openness has different impact for different countries and trade openness depends on the country regulatory authority. These finding suggests that the contribution of trade openness in CO2 emission is not conclusive across all sub-samples.
The results also indicate that our instruments are valid as in the test of over-identification; p value is greater than 0.05. Hence, we accept the null hypothesis of the Hansen test that instruments used are exogenous.
Conclusion
This study aims to investigate the impact of ICT on the environmental sustainability. The importance of ICT is very much understandable in the present age of industrial revolution and technological advancement. Likewise, the environmental sustainability is a global issue and ICT has an important role in this environmental sustainability.
This study investigates the impact of ICT on carbon dioxide emissions for a global panel of 91 countries over the period 1990 to 2017. Moreover, this study conducts a comparative analysis of developed and developing countries to investigate the impact of ICT on CO2 emissions. For this purpose, an ICT index has been constructed from four different indicators of ICT. The study conducts cross-sectional dependence tests and employs POLS, FEM, and SGMM estimation techniques with panel-corrected standard errors to handle CSD in our data.
In this study, ICT has negative impact on carbon dioxide emissions for a panel of 91 countries while energy consumption shows positive impact on CO2 emissions as expected. The results also suggest that at the initial level of income, environmental degradation increases but after a threshold level, further increase in income leads to a decline in CO2 emissions. Based on these findings, it can be concluded that EKC exists for all income groups in our analysis. Moreover, the decline in CO2 emissions after the threshold level indicates the technological advancement and efficient use of energy. Furthermore, a comparative study of developed and developing countries has been conducted. The results show that in developed countries, ICT plays a significant role in mitigating emissions while in developing countries, the technological advancement is not enough to decline emissions through ICT. However, existence of environmental Kuznets curve in the presence of ICT suggests that it would be possible to contribute towards environmental sustainability in the latter stages of development even with diffusion of ICT.
The study has following recommendations on the basis of these findings. The path to sustainable environment may be linked to the development of ICT sector in all countries, as with higher GDP per capita more efficient techniques will be used which will take care of the environment. Moreover, policies should be designed to encourage structural changes in the economy which stimulates shift from the manufacturing sector to services sector (less energy-intensive). Furthermore, energy consumption plays an important role in environmental degradation; therefore, some substitute sources of energy like renewable energy (solar energy) should be pushed in the production process.
The study has the following limitations. The analysis has been conducted for a shorter period of time and for a limited number of countries due to data unavailability for ICT indicators; however, it can be extended to all countries. Moreover, the study has used only CO2 as a proxy for environmental degradation but other indicators of environmental degradation (ecological footprint) may also be added for further analysis.
Notes
Ecological footprint is a quantitative measure for environmental impacts and it shows how much of environment is demanded by the individuals. Moreover, it documents the extent to which human activities are within the regenerative capacity of the biosphere (Ulucak and Bilgili 2018).
The employment of POLS to the data with CSD delivers inefficient coefficient estimates and corresponding standard error estimates are also biased.
Our panel causality test results suggest that there is bidirectional causality between CO2 emissions and ICT.
References
Akande A, Cabral P, Casteleyn S (2019) Assessing the gap between technology and the environmental sustainability of European cities. Inf Syst Front 21(3):581–604
Almeida T, Cruz L, Barata E, Sánchez I (2017) Economic growth and environmental impacts: an analysis based on a composite index of environmental damage. Ecol Indic 76:119–130
Al-Mulali U, Sheau-Ting L, Ozturk I (2015) The global move toward internet shopping and its influence on pollution: an empirical analysis. Environ Sci Pollut Res 22:9717–9727
Antweiler W, Copeland BR, Taylor MS (2001) Is free trade good for the environment? Am Econ Rev 91(4):877–908
Beck N, Katz JN (1995) What to do (and not to do) with time-series cross-section data. American Political Science Review 89:634–647
Ben-Jebli M, Youssef S, Ozturk I (2016) Testing environmental Kuznets curve hypothesis: the role of renewable and non-renewable energy consumption and trade in OECD countries. Ecol Indic 60:824–831
Cruz-Jesus F, Oliveira T, Bacao F, Irani Z (2017) Assessing the pattern between economic and digital development of countries. Inform Syst Front 19(4):835–854
Dabbous A (2018) The impact of information and communication technology and financial development on energy consumption: a dynamic heterogeneous panel analysis for MENA countries. Int J Energy Econ Policy 8(4):70–76
Dinda S (2004) Environmental Kuznets curve hypothesis: a survey. Ecol Econ 49(4):431–455
Dogan E, Ulucak R, Kocak E, Isik C (2020) The use of ecological footprint in estimating the environmental Kuznets curve hypothesis for BRICST by considering cross-section dependence and heterogeneity. Sci Total Environ 723(25):138063
Driscoll J, Kraay AC (1998) Consistent covariance matrix estimation with spatially dependent data. Rev Econ Stat 80:549–560
Ejemeyovwi J, Adiat Q, Ekong E (2019) Energy usage, internet usage and human development in selected western African countries. Int J Energy Econ Policy 9(5):316–321
Erdmann L, Hilty LM (2010) Scenario analysis: exploring the macroeconomic impacts of information and communication technologies on greenhouse gas emissions. J Ind Ecol 14(5):826–843
Farhani S, Ozturk I (2015) Causal relationship between CO2 emissions, real GDP, energyconsumption, financial development, trade openness, and urbanization in Tunisia. Environ Sci Pollut Res 22:15663–15676
Fernández-Amador O, Francois J, Oberdabernig D, Tomberger P (2020) Economic growth, sectoral structures, and environmental methane footprints. Appl Econ 52(13):1460–1475
Galli A (2015) On the rationale and policy usefulness of ecological footprint accounting: the case of Morocco. Environ Sci Policy 48:210–224
Grossman GM, Krueger AB (1991) Environmental impacts of a North American free trade agreement (No. W3914). National Bureau of Economic Research
Guo J, Zhang Y, Zhang K (2018) The key sectors for energy conservation and carbon emissions reduction in China: evidence from the input-output method. J Clean Prod 179:180–190
Halicioglu F (2009) An econometric study of CO2 emissions, energy consumption, income and foreign trade in Turkey. Energy Policy 37(3):1156–1164
Haseeb A, Xia E, Saud S, Ahmad A, Khurshid H (2019) Does information and communication technologies improve environmental quality in the era of globalization? An empirical analysis. Environ Sci Pollut Res 26:8594–8608
Hausman, J. A. (1978). Specification tests in econometrics. Econometrica: Journal of The Econometric Society, 1251–1271
Higon DA, Gholami R, Shirazi F (2017) ICT and environmental sustainability: a global perspective. Telematics Inform 34(4):85–95
Hoechle D (2007) Robust standard errors for panel regressions with cross-sectional dependence. Stata J 7(3):281–312
Houghton JW (2009) ICT and the environment in developing countries: an overview of opportunities and developments. Commun Strateg 1(76):39–60
Houghton JW (2010) ICT and the environment in developing countries: a review of opportunities and developments. In: Berleur J, Hercheui MD, Hilty LM (eds) What kind of information society? Governance, virtuality, surveillance, sustainability, resilience. HCC 2010, CIP 2010. IFIP Advances in Information and Communication Technology, vol 328. Springer, Berlin, Heidelbeg, pp 236–247
International Energy Agency (2009). International Energy Agency (IEA): Paris, France
Khan D (2019) Effects of information and communication technology real income, and CO2 emissions: the experience of countries along belt and road. Telematics Inform 45:101300
Leal P, Marques A, Fuinhas J (2019) Decoupling economic growth from GHG emissions: decomposition analysis by sectoral factors for Australia. Economic Analysis and Policy 62:12–26
Lee JW, Brahmasrene T (2014) ICT, CO2 emissions and economic growth: evidence from a panel of ASEAN. Glob Econ Rev 43(2):93–109
Lu WC (2018) The impacts of information and communication technology, energy consumption, financial development, and economic growth on carbon dioxide emissions in 12 Asian countries. Mitig Adapt Strateg Glob Chang 23(8):1351–1365
Majeed MT, Khan FN (2019) Do information and communication technologies (ICTs) contribute to health outcomes? An empirical analysis. Qual Quant 53(1):183–206
Moffatt I (2000) Ecological footprints and sustainable development. Ecol Econ 32:359–362
Ng C, Choong C, Lau L (2020) Environmental Kuznets curve hypothesis: asymmetry analysis and robust estimation under cross-section dependence. Environ Sci Pollut Res 27:18685–18698
Nguyen D, Sévi B, Sjö B, Salah-Uddin G (2017) The role of trade openness and investment in examining the energy-growth-pollution nexus: empirical evidence for China and India. Appl Econ 49(40):4083–4098
Nuroglu E, Kunst RM (2018) Kuznets and environmental Kuznets curves for developing countries. In: Yülek M (ed) Industrial policy and sustainable Growth. Sustainable Development, Springer, Singapore
Ozcan B, Apergis N (2018) The impact of internet use on air pollution: evidence from emerging countries. Environ Sci Pollut Res 25(5):4174–4189
Panayotou T (1997) Demystifying the environmental Kuznets curve: turning a black box into a policy tool. Environ Dev Econ 2(4):465–484
Parks R (1967) Efficient estimation of a system of regression equations when disturbances are both serially and contemporaneously correlated. J Am Stat Assoc 62:500–509
Pesaran, M. H. (2004). General diagnostic tests for cross section dependence in panels. Cambridge Working Papers in Economics No. 0435, Faculty of Economics, University of Cambridge
Plepys A (2002) The grey side of ICT. Environ Impact Assess Rev 22(5):509–523
Pradhan RP, Mallik G, Bagchi TP (2018) Information communication technology (ICT) infrastructure and economic growth: a causality evinced by cross-country panel data. IIMB Manag Rev 30(1):91–103
Reed W, Ye H (2009) Which panel data estimator should I use? Appl Econ 43(8):985–1000
Sabri A, Sabri O, Al-Shargabi B (2012) A cultural e-government readiness model. Intell Inf Manag 4:212–216
Salahuddin M, Alam K, Ozturk I (2016a) The effects of internet usage and economic growth on CO2 emissions in OECD countries: a panel investigation. Renew Sust Energ Rev 62:1226–1235
Salahuddin M, Alam K, Ozturk I (2016b) Is rapid growth in internet usage environmentally sustainable for Australia? An empirical investigation. Environ Sci Pollut Res 23:4700–4713
Shahbaz M, Hye QMA, Tiwari AK, Leitão NC (2013) Economic growth, energy consumption, financial development, international trade and CO2 emissions in Indonesia. Renew Sust Energ Rev 25:109–121
Sohag K, Mamun M, Salah-Uddin G, Ahmed M (2017) Sectoral output, energy use, and CO2 emission in middle-income countries. Environ Sci Pollut Res 24:9754–9764
Tsaurai K, Chimbo B (2019) The impact of information and communication technology on carbon emissions in emerging markets. Int J Energy Econ Policy 9(4):320–326
Uddin M, Rahman A, Memon J (2011) Carbon sustainability framework to reduce CO2 emissions in data centers. International Journal of Green Economics 5(4):353–369
Ulucak R, Bilgili F (2018) A reinvestigation of EKC model by ecological footprint measurement for high, middle and low income countries. J Clean Prod 88:144–157
United Nations Climate Change Conference (2015). COP 21 or CMP 11, 30 November to 12 December: Paris, France
WDI (2018) World development indicators. World Bank, Washington, DC
Wyckoff AW, Roop JM (1994) The embodiment of carbon in imports of manufactured products: implications for international agreements on greenhouse gas emissions. Energy Policy 22(3):187–194
Author information
Authors and Affiliations
Corresponding author
Additional information
Responsible Editor: Philippe Garrigues
Publisher’s note
Springer Nature remains neutral with regard to jurisdictional claims in published maps and institutional affiliations.
Appendix
Appendix
Rights and permissions
About this article
Cite this article
Khan, F.N., Sana, A. & Arif, U. Information and communication technology (ICT) and environmental sustainability: a panel data analysis. Environ Sci Pollut Res 27, 36718–36731 (2020). https://doi.org/10.1007/s11356-020-09704-1
Received:
Accepted:
Published:
Issue Date:
DOI: https://doi.org/10.1007/s11356-020-09704-1