Abstract
Stormwater particles washed from road-deposited sediments (RDS) are traditionally characterized as either turbidity or total suspended solids (TSS). Although these parameters are influenced by particle sizes, neither of them characterizes the particle size distribution (PSD), which is of great importance in pollutant entrainment and treatment performance. Therefore, the ratio of turbidity to TSS (Tur/TSS) is proposed and validated as a potential surrogate for the bulk PSD and quality of stormwater runoff. The results show an increasing trend of Tur/TSS with finer sizes of both RDS and stormwater runoff. Taking heavy metals (HMs, including Cu, Pb, Zn, Cr, and Ni) as typical pollutants in stormwater runoff, the concentrations (mg/kg) were found to vary significantly during rainfall events and tended to increase significantly with Tur/TSS. Therefore, Tur/TSS is a valid parameter to characterize the PSD and quality of stormwater. The high negative correlations between Tur/TSS and rainfall intensity demonstrate that stormwater with higher Tur/TSS generates under low intensity and, thus, characterizes small volume, finer sizes, weak settleability, greater mobility, and bioavailability. Conversely, stormwater with lower Tur/TSS generates under high intensity and, thus, characterizes large volume, coarser sizes, good settleability, low mobility, and bioavailability. These results highlight the need to control stormwater with high Tur/TSS. Moreover, Tur/TSS can aid the selection of stormwater control measures with appropriate detention storage, pollution loading, and removal effectiveness of particles.
Similar content being viewed by others
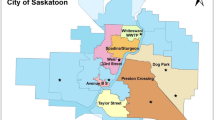
Explore related subjects
Discover the latest articles, news and stories from top researchers in related subjects.Avoid common mistakes on your manuscript.
Introduction
Stormwater particles, derived from the wash-off of road-deposited sediments (RDS) during rainfall events, are potentially toxic mediums containing pollutants such as nitrogen, phosphorus, and heavy metals (HMs) (Beck and Birch 2012; El-Mufleh et al. 2014; Zhang et al. 2013). These particles and associated pollutants are generated from a wide range of natural and anthropogenic sources including wet and dry deposition, vehicle exhausts, tyre wear, road wear, brake pad lining wear, abrasion of construction materials, and soil erosion, among others (Bian et al. 2015; Soltani et al. 2015). Once they enter into urban waterways without treatment, these particles may not only lead to the clogging of waterway beds, refugia loss, reduction of the exchange capacity between benthic and water column zones, reduced food quality, smothering of biota, respiratory damage, and light attenuation but also pose potential toxicity to aquatic systems due to the associated toxicants (i.e., HMs) (Blecken et al. 2012; Charters et al. 2015). Therefore, the stormwater particles should always be the primary focus of stormwater control measures (SCMs) to preserve waterways (Fassman 2012; Fulazzaky et al. 2013; Maniquiz-Redillas et al. 2014; Yahyapour et al. 2014).
Knowledge of the particle properties particularly concentrations and particle size distribution (PSD) during rainfall events facilitates the effective management of stormwater pollution. Characterized as total suspended solids (TSS), traditionally, stormwater particles have been thoroughly investigated in terms of concentrations, loads, intra- and inter-event variability, and their relationships with other pollutants (Chow and Yusop 2014; Herngren et al. 2005; Peng et al. 2016; Zhang et al. 2013). Nonetheless, as TSS in water causes the scattering of light, turbidity has also been investigated as a promising surrogate for TSS (Rügner et al. 2013). However, neither TSS nor turbidity characterizes the bulk PSD of stormwater particles, which determines the characteristics of particles such as mobility, settleability, pollutant entrainment, and removal efficiency of SCMs (Ying and Sansalone 2010; Yun et al. 2010). Generally, fine particles have higher mobility and weak settleability and transport more pollutants. Researchers report a general trend of decreasing HM accumulation with increasing particle sizes (McKenzie et al. 2008). In addition, the removal performance of TSS by SCMs also decreases with finer PSD. Ferreira and Stenstrom (2013) found that the removal percentages of < 8, 8~20, 20~100, and > 100 μm particles by a dry detention basin were 57~75%, 84~91%, 84~95%, and 100%, respectively. These highlight the importance of the granulometry of stormwater particles in stormwater pollution, as well as in the optimal selection and design of SCMs, to enhance treatment performance.
The hetero-disperse PSDs and the preferential accumulation of HMs in fine particles have been consistently reported for stormwater runoff. Nevertheless, these relations, to date, have usually been examined for grab samples, event mean samples, or intra-event samples (first flush and steady state samples) rather than consecutive samples (i.e., time series samples) (Béchet et al. 2009; Kim and Sansalone 2008). Consequently, the intra-event variations of PSDs and HM accumulation during rainfall events are overshadowed. These deficiencies, to a great extent, can be ascribed to the laborious and inconvenient processes of PSD characterization (by laser diffraction) and wet sieving process. However, the paucity can be complemented by introducing a pragmatic and convenient surrogate to evaluate bulk PSD directly, without having to carry out resource intensive laboratory experiments.
For turbidity measurement, light scattering method is applied so that turbidity, in fact, characterizes the optical property of the particles in water. In contrast, TSS measures the absolute weight of particles suspended in a unit volume of water. Under a condition that all particles are of a uniform size, and therefore possess the same light scattering property, there should be a proportionality relationship defined as follows:
where the coefficient m can be taken as a constant. However, solid particles may not be of the same size but have variable PSDs under different situations. For water samples of the same TSS concentration, the measured turbidities are much influenced by particle sizes (Ying and Sansalone 2010). Generally, turbidity is more sensitive to fine particles due to their large specific surface areas, whereas TSS is more sensitive to coarser particles due to their higher weight. Therefore, the variations of PSD will determine the magnitude of the coefficient m from site to site, from event to event, and even from moment to moment. For example, Ying and Sansalone (2010) reported an m value of 2.83 during mass-limited rainfall events and an m value of 1.23 during flow-limited rainfall events for runoff of I-10 Bridge at City Park Lake in Baton Rouge, Louisiana. Similarly, Wang et al. (2017) reported that the m value of road runoff ranged from 0.26 to 2.56 during multiple rainfall events in Yixing, China. We can, thus, deduce that the coefficient m in Eq. (1), or its reciprocal 1/m, is closely related with the PSD of the water sample.
Considering that TSS and turbidity can be measured relatively, rapidly, and easily in contrast to the tedious procedure of PSD analysis, a new indicator Tur/TSS (the ratio of turbidity to TSS) is proposed, in this study, as a potential surrogate of the bulk PSD of stormwater runoff. By laboratory and field experiments, the characteristics of Tur/TSS variation during rainfall events were investigated. Furthermore, the correlative relationship between Tur/TSS and the runoff water quality was evaluated by taking heavy metals as typical pollutants. The results provide validation of the applicability of Tur/TSS as an indicator for assisting runoff pollution control.
Material and methods
Study area and sampling site
The study area is located in Yixing, a typical city with large drainage density in the Taihu Lake Basin, East China. The population is approximately 1.24 million and the total area is 1996.6 km2. Yixing City features a subtropical monsoon climate. The average annual temperature, rainfall, number of rainy days, and evaporation are 15.7 °C, 1177 mm, 136.6 days, and 849 mm, respectively. The sampling site is located at Lvyuan road in the industrial park for Environmental Science and Technology (Fig. 1a) where many environmental protection industries, machinery factories, steel mills, cable factories, and electron processing manufacture factories are distributed. Lvyuan road is a two-way six-lane road with an asphalt surface and has an annual average daily traffic of 12,000 vehicles including trucks (2.3%), buses (1.7%), cars (65.7%), motorcycles (8.6%), pedicabs (4.4%), motorcars (16.8%), and bicycles (0.6%). Lvyuan road is swept daily with both manual and mechanical methods, and debris is seldom observed on road surface.
Sample collection and preparation
RDS samples
Overall, five RDS samples were collected with clean brushes and dustpans from the curbs of Lvyuan road (Fig. 1b) during March 2016 to March 2017. Regardless of the high efficiency of collecting fine particles, the wet and dry vacuum systems were not adopted in this study to prevent the loss of pollutants resulting from dissolution and desorption to aqueous phases during wet sampling and sieving (Zhang and Krebs 2013). The antecedent dry days (ADD) ranged from 2 to 8.5 days. Due to the dominance of < 150 μm RDS in stormwater, the RDS samples were firstly dry-sieved into six fractions with five fractions (< 22, 22~38.5, 38.5~76, 76~105, and 105~150 μm) within < 150 μm and one fraction within 150~2000 μm. Subsequently, all the subsamples were oven-dried at 25 °C for 5 days and stored in a vacuum dryer until analysis.
Stormwater samples
Stormwater samples were collected from the inlet grating at the roadside of Lvyuan road (Fig. 1c) over November 2015 to November 2016. The catchment area is about 230 m2. A total of nine rainfall events were monitored, and 178 samples were obtained. Stormwater samples were collected at 5-min intervals following runoff generation. Polyethylene bottles with 500 ml volume were used and were acid-washed prior to sampling. If the runoff volume was too little to obtain a 500-ml sample, the sampling time was extended to collect sufficient runoff. Furthermore, if the runoff period was over 4 h, sampling intervals were extended to 1 or 2 h. On the other hand, the sampling intervals were shortened to 1~2 min during thunderstorm events. Samples were transported to the laboratory immediately after sampling, pretreated, and analyzed. Rainfall was measured by a tipping-bucket rain gauge installed near the sampling sites, and the data was available as a time series with a 1-min time step. The characteristics of rainfall events monitored are detailed in Table 1.
Laboratory experiments and testing
Batch experiments of RDS samples
A batch of experiments were carried out with RDS fractions of < 22, 22~38.5, 38.5~76, 76~105, 105~150, and 150~2000 μm to evaluate their contributions to turbidity and Tur/TSS. Solutions were formulated with one solvent (ultrapure water), thirty solutes (five RDS samples with six size fractions), and at least three TSS concentrations (500, 1000, 2000, 5000, or 10,000 mg/L). The TSS concentrations selected herein are higher than that in typical stormwater runoff due to two primary considerations. Firstly, very small amount of RDS subsample is needed to formulate a solution with lower TSS concentration (i.e., < 500 mg/L), especially for coarse RDS fractions. It will thus cause higher laboratory error. Moreover, the high TSS concentration can represent the total solids found on road surfaces. The solutions were mixed on a vapor-bathing constant temperature vibrator (CHA-SA) at 25 °C and 150 rpm for 8 h to simulate the wash-off of rainfall. Then, the corresponding turbidity was measured to calculate the ratio of Tur/TSS.
Laboratory testing
Besides the turbidity measurement of the batch experiments, HM concentrations (mg/kg) were also analyzed for < 22, 22~38.5, 38.5~150, and 150~2000 μm RDS. Turbidity, TSS, total, and dissolved heavy metal concentration (mg/L) were analyzed for stormwater samples. Furthermore, to observe the variations of particle sizes with Tur/TSS, morphological analysis was carried out for six representative RDS and stormwater particles, the Tur/TSS of which ranged from 0.001 to 1.74. PSDs were analyzed for 21 representative stormwater samples, the turbidity, TSS and Tur/TSS of which ranged between 41.6–530 NTU, 70–1036 mg/L, and 0.23–1.52 NTU·L/mg, respectively. The concentrations (mg/L) of particulate HMs were calculated as the difference between total and dissolved HMs. The accumulation of HMs (mg/kg) in stormwater particles was calculated as the ratio of particulate HM concentrations and the corresponding TSS.
Turbidity was measured by using a HACH 2100N laboratory turbidimeter. TSS was measured according to the gravimetric method. Surface morphology of particles was observed with a scanning electron microscope (SEM, Hitachi S-3400 N II, Japan). PSD was analyzed via Mastersizer 2000 (Malvern Instruments Ltd.), and the results are presented as the volume proportions of 100 size intervals (from 0.01 to 3500 μm), DV(10), DV(50), and DV(90) (the size at which 10, 50, and 90% particles pass, respectively). The bulk stormwater samples and filtrates through 0.45-μm membranes were used to analyze total and dissolved HMs and were preserved with trace grade nitric acid to lower the pH to ≤ 2. The concentrations of five HMs, including Cu, Pb, Zn, Cr, and Ni, were measured by inductively coupled plasma-optical emission spectrometry (ICP-OES) according to the national standard method (HJ 776-2015) for stormwater samples and according to EPA 6010C:2007 for RDS samples. Quality assurance protocols included blanks, standards, duplicates (10% samples), and spiked samples (5% samples). The recoveries within the range of 70~120% were recorded. Additionally, instrument calibration was conducted after each batch analysis and per the QA/QC procedures detailed in sampling and analysis methods (e.g., HJ 776-2015).
Statistical analyses
To compare the differences of HM accumulation in stormwater particles and RDS, one-way analysis of variance (one-way ANOVA) was employed. All data were firstly examined for normality and homogeneity of variance. Then, least significant difference (LSD) method was used for post-ANOVA pairwise comparisons. Furthermore, principal component analyses (PCA) were carried out to explore the correlations of stormwater particles (turbidity, TSS, and Tur/TSS) with PSDs, HMs, and rainfall characteristics, respectively. PCA is a multivariate statistical data analysis technique capable of identifying correlations between objects and variables by reducing a set of raw data into a number of principal components which retain the most variance within the original data (Miguntanna et al. 2013). In PCA, variables that exhibit similar variances have similar PCA scores forming a cluster when plotted on a biplot. On the biplot, strongly correlated variables have the same orientation whereas uncorrelated variables are orthogonal to each other. Finally, the Pearson correlation analyses were performed to validate the outcomes of PCA analyses. All statistical analyses were carried out using IBM SPSS Statistics 19.0 software (IBM Corporation, Armonk, NY, USA).
Results
Validation of Tur/TSS as a surrogate for PSD
RDS samples
The contribution of RDS to Tur/TSS decreased significantly with the coarse size fractions (Fig. 2). The average Tur/TSS contributed by the < 22, 22~38.5, 38.5~76, 76~105, 105~150, and 150~2000 μm RDS were 0.22 ± 0.05, 0.16 ± 0.02, 0.05 ± 0.008, 0.011 ± 0.004, 0.007 ± 0.003, and 0.004 ± 0.003 NTU·L/mg, respectively. Overall, the Tur/TSS was relatively stable for specific size fractions regardless of the variability in TSS concentrations. This demonstrates that Tur/TSS is decisively influenced by PSD other than the quantity of particles. Compared with the < 38.5 μm RDS, the contributions of the > 38.5 μm RDS to Tur/TSS were very low, demonstrating that the < 38.5 μm RDS were the main contributors to Tur/TSS in stormwater runoff.
Stormwater samples
During the nine rainfall events, the Tur/TSS of stormwater samples ranged from 0.14 to 2.04 NTU·L/mg, with an average of 0.75 ± 0.46 NTU·L/mg. Although the formulated TSS concentrations were several orders of magnitude higher than that typically occurring in stormwater, the Tur/TSS of the formulated solutions were significantly lower than that recorded in stormwater. Only the Tur/TSS of the < 22 and 22~38.5 μm RDS approached the lower limit of stormwater. This finding indicates that the PSD of stormwater may be much finer than < 38.5 μm RDS.
Generally, the PSD of stormwater particles was finer with higher Tur/TSS (Supplementary material: Fig. S1). PCA analysis was carried out to ascertain the detailed relationships between Tur/TSS and PSD of stormwater runoff. The objects for the PCA were stormwater samples from multiple rainfall events, while the variables consisted of the volume proportions of 100 particle size intervals (from 0.01 to 3500 μm), DV(10), DV(50), DV(90), turbidity, TSS, and Tur/TSS. Seven principal components were identified, which explained 97.76% of the total variances. However, the Tur/TSS primarily contributed to PC1, and thus, the PCA biplot was plotted with PC1 and PC2 (Fig. 3). Overall, Tur/TSS and volume proportions of 22 particle size intervals from 0.314 to 5.21 μm (group 1) abutted against the positive PC1 axis and formed acute angles with each other. Moreover, the angles increased with increasing particle sizes. This highlights their strong positive correlations, and that Tur/TSS increases with higher volume proportions of these very fine particles. On the other hand, DV(10) and volume proportions of 9 size intervals from 14.5 to 45.6 μm (group 2) abutted against the negative PC1 axis, and formed obtuse angles with Tur/TSS. This indicates the strong and negative correlations between Tur/TSS and DV(10), as well as the volume proportions of 14.5~45.6 μm particles. Tur/TSS decreased with the increase of these larger particles. These results were further confirmed by the Pearson linear correlation analysis, whereby significant correlations were observed among these parameters (Supplementary material: Table S1). Therefore, Tur/TSS is a particle parameter closely associated with the PSD of stormwater runoff and increases with the proportions of very fine (nano- and submicron-scale) particles.
Principal component analysis of Tur/TSS and PSD of stormwater runoff. Scatter plots of the treatments and loading variables on the PC1 and PC2 axes. The first and second principle components are given. Percentage of variance explained by each component is indicated within the parentheses on each axis
SEM micrographs
The SEM micrographs of RDS (Fig. 4A1–A3) and stormwater particles (Fig. 4B1–B3) with increasing Tur/TSS show that the particle sizes for all RDS samples were evidently larger than stormwater particles, supporting the finding that the PSD of stormwater was much smaller than < 38.5 μm RDS. The particle sizes decrease significantly with the increase of Tur/TSS. More nano- and submicron-scale particles were observed for samples with higher Tur/TSS. It is noteworthy that some small particles were observed to be attached on the surfaces of coarse RDS (Fig. 4A1–A3). Thus, it could be assumed that their detachment is the actual contributors to the Tur/TSS of coarse RDS, as demonstrated by Borris et al. (2016).
Influence of PSD on heavy metal pollution in stormwater
Heavy metal accumulation in RDS and stormwater particles
The accumulation of HMs in RDS with four size fractions and stormwater particles is presented and compared in Table 2. The highest concentrations of Cu, Cr, and Ni in RDS were recorded in the 38.5~150 μm fraction whereas that for Pb and Zn were recorded in the 22~38.5 μm fraction. Although particle sizes play critical roles in the accumulation of HMs, the highest concentration was not observed in the finest fraction. This result is consistent with that reported by Gunawardana et al. (2014) who also detected the highest concentrations of Cu and Zn in the 75~150 μm RDS rather than < 75 μm RDS in Gold Coast, Australia. Furthermore, statistically significant differences were only detected for Cu between 38.5~150 and 150~2000 μm RDS and for Pb between < 150 and 150~2000 μm RDS.
In stormwater runoff, the HMs were dominant in particulate phase (Table 2), and 27.2~100% of HMs in the dissolved phase were below the limit of detection. The HMs (mg/kg) in stormwater particles varied significantly between rainfall events and ranged from 55.8~1577.0 for Cu, 87.4~1913.3 for Pb, 350.0~14,119.0 for Zn, 40.6~1080.0 for Cr, and 19.8~413.9 for Ni. The maximum values were as high as 20.9~40.3 times of the minimum values. Moreover, the average concentrations of Cu, Pb, Zn, Cr, and Ni in stormwater particles were 1.5~3.7, 1.6~4.3, 4.8~9.7, 0.7~1.2, and 0.7~1.2 times of that in RDS. Statistically, the Cu concentration of stormwater particles was significantly higher than that of the < 22, 22~38.5, and 150~2000 μm RDS. Similarly, the Pb concentration was significantly higher than that of the 38.5~150 and 150~2000 μm RDS, and the Zn concentration was significantly higher than that of all four RDS fractions. With respect to Cr and Ni, the concentrations were significantly lower than that of the 38.5~150 μm RDS. The differences were related to the build-up and wash-off pattern of RDS and associated HMs. Most of the stormwater particles washed off from road surfaces are within the very fine sizes, and only few coarse particles are transported into stormwater runoff (Zhao and Li 2013). Therefore, the concentrations of stormwater particles will be higher for HMs (such as Pb and Zn), which accumulated more in fine RDS, but lower for HMs (such as Cr and Ni), which accumulated more in larger RDS. The significantly high HM concentrations and variations in stormwater particles highlight the need to control stormwater runoff with higher HM concentrations.
Relationship between Tur/TSS and HM accumulation
To examine the effectiveness of Tur/TSS as a parameter for characterizing the HMs accumulation, the relationships between HMs and the Tur/TSS were evaluated by PCA. The objects for the PCA were the stormwater samples from multiple rainfall events, while the variables consisted of three particle parameters (turbidity, TSS, and Tur/TSS), volume concentration of total and particulate HMs, and mass concentration of the total and particulate HMs. To be simplified, the volume concentration of total and particulate HM was denoted by THM and PHM, while the mass concentration of total and particulate HM was denoted by THM(m) and PHM(m). Four principal components were identified, which explained 90.70% of the total variances. However, most of the variables contributed to the first two principal components and explained 73.55% of the total variances. Therefore, PCA biplot was plotted with PC1 and PC2 (Fig. 5). Two outstanding clusters were formed for HMs as follows: the mass concentration group (group 1) and the volume concentration group (group 2). The group 1 principally projected on the positive PC2 axis including TCu(m)/PCu(m), TPb(m)/PPb(m), TZn(m)/PZn(m), TCr(m), and Tur/TSS, while group 2 projected on the positive PC1 axis including TCu/PCu, TPb/PPb, TZn/PZn, TCr/PCr, TNi/PNi, turbidity, and TSS. The small angles of group 1 demonstrate the strong and positive correlations between Tur/TSS and the mass concentration of HMs (except TCr(m)/PCr(m) and PNi(m)). Thus, more HMs accumulated in stormwater with higher Tur/TSS and finer PSDs. These results were further confirmed by the Pearson linear correlation analyses, whereby significant correlations were observed among these parameters (Supplementary material: Table S2). The weak correlations between Tur/TSS and the mass concentrations of total and particulate Cr can be attributed to the preferential accumulation of Cr in coarser particles (highest in 38.5~150 μm RDS, Table 2). Moreover, the strong correlation between Tur/TSS and TNi(m) and weak correlation between Tur/TSS and PNi(m) may result from the high dissolvability of Ni (Table 2). Therefore, as an effective surrogate for bulk PSD, Tur/TSS is indicative of the HM accumulation in stormwater particles. Furthermore, the acute angles of group 2 confirm the strong and positive correlations among TSS, turbidity, and volume concentrations of total and particulate HMs, which have been reported by previous researchers (German and Svensson 2002; Hallberg et al. 2006; Helmreich et al. 2010).
PCA biplot of stormwater particles and HM concentrations. The first and second principle components are given. Percentage of variance explained by each component is indicated within the parentheses on each axis. Both the volume concentration (mg/L) and mass concentration (mg/kg) of total and particulate HMs were analyzed. THM and PHM denote the volume concentration of total and particulate HM, while THM(m) and PHM(m) denote the mass concentration of total and particulate HM
Variability of Tur/TSS during rainfall events
Due to the close relationships among Tur/TSS, PSDs, and HM accumulation, the variations of Tur/TSS can be indicative of the variations of PSDs and HM accumulation during rainfall events. The intra-event variations of Tur/TSS and influential factors were analyzed in the following subsections.
Intra-event variations of Tur/TSS
The turbidity, TSS, and Tur/TSS varied greatly during multiple rainfall events and ranged from 9.9 to 746 NTU, 8 to 1728 mg/L, and 0.14 to 2.04 NTU·L/mg, respectively. The variations mostly originated from the rainfall characteristics during wet weather and build-up of RDS during dry weather (Huang et al. 2011; Wijesiri et al. 2015; Zhang et al. 2013). To investigate the temporal variations of turbidity, TSS, and Tur/TSS, four representative rainfall events were selected and illustrated in Fig. 6. Based on the hyetographs, the rain type for March 8 and May 2 could be identified as “middle peak,” whereas that for May 31 is “preceding peak” and that for September 14 is “back peak.” The variations of Tur/TSS were different for all rainfall types. For the “middle peak” precipitation, Tur/TSS varied concavely, namely, decreased firstly and increased subsequently. For the “preceding peak” precipitation, Tur/TSS remained low but increased during the later period, while for the “back peak” precipitation, Tur/TSS varied convexly. However, turbidity and TSS, in general, showed decreasing trends accompanied with a few fluctuations for rainfall events without intermittence. The peak values of turbidity and TSS generally occurred during the initial period of rainfall or when rainfall intensity increased abruptly. The intra-event variations of turbidity and TSS were consistent with findings from previous studies (Kim et al. 2013; Metadier and Bertrand-Krajewski 2012; Zhang et al. 2013).
Factors influencing intra-event variations of stormwater particles
PCA was performed to determine the influence of rainfall characteristics on the intra-event variations of stormwater particles. The objects for the PCA were the stormwater samples from multiple rainfall events, while the variables consisted of four rainfall parameters (runoff time, rainfall intensity, rainfall depth, and cumulative rainfall) and three particle parameters (turbidity, TSS, and Tur/TSS). Two principal components were identified, which explained 72.14% of the total variances. The PCA biplot (Fig. 7) shows that Tur/TSS, rainfall intensity, and rainfall depth primarily projected on the PC2 axis and distributed in absolutely opposite directions, indicating the negative influence of rainfall intensity on Tur/TSS. The increase of rainfall intensity will lead to the decrease of Tur/TSS. This is somewhat expectable since rainfall with higher intensity is capable of transporting larger RDS and results in coarser PSDs and lower Tur/TSS (Zhao et al. 2016). Furthermore, turbidity and TSS mainly projected on the PC1 axis in the opposite direction of cumulative rainfall and runoff time. This result demonstrates the overall decreasing trend of turbidity and TSS during rainfall events, ascribing to the depletion of washable particles on the road surface. This is consistent with the results presented in Fig. 6 and that of other previous studies (Bach et al. 2010; Zhang et al. 2013). Furthermore, these results were also confirmed by the Pearson linear correlation analyses, and significant correlations were observed among these parameters (Supplementary material: Table S3).
PCA biplot of stormwater particles and rainfall characteristics. Rainfall intensity and rainfall depth denote the average rainfall intensity and rainfall depth during the time interval △T of sampling process. Cumulative rainfall denotes the total rainfall depth from rainfall occurrence to sample collection. Runoff time denotes the duration from runoff generation to the sample collection
Discussions
Applicability of Tur/TSS in PSD and stormwater quality characterization
Based on the definitions of turbidity and TSS, Tur/TSS (NTU·L/mg) should be a direct measure of the optical property per unit TSS. For turbidity measurement, the light scattered by the sample is compared with the light scattered by the standard reference suspension of formazin polymer. The measurement is much influenced by the size of solid particles in the sample. Therefore, for particles of finer sizes, the turbidity is high in comparison with particles of larger sizes (Everett 1988). Accordingly, an increasing Tur/TSS value was observed for RDS and stormwater samples with finer sizes.
Overall, the PSDs of RDS and stormwater particles were expressed qualitatively by Tur/TSS. Nonetheless, the PSD curves cannot be plotted via Tur/TSS values. Therefore, the utility of Tur/TSS relates to the strong correlations with DV(10), as well as HM accumulation. The DV(10) of particles and HM accumulation in stormwater can, thus, be predicted according to these relationships. These should be considered in further studies. Another limitation of this study is that Tur/TSS was validated for one specific catchment area. The results may be slightly different in other catchments owing to the differences in the build-up and wash-off processes of RDS and associated HMs. For example, as the relationship between turbidity and TSS is also influenced by other factors (i.e., particle shape, organic content, and degree of water coloring), the relationships of Tur/TSS with PSDs may be influenced by the sources of particles (Gunawardana et al. 2012; Ying and Sansalone 2010). Also, the relationships between Tur/TSS and rainfall intensity may be influenced by road slope and roughness, catchment area, disturbance by passing vehicles, etc. (Liu et al. 2015; Zhang et al. 2013). Therefore, further studies may be needed in other catchments to determine the differences and, thus, to manage the local stormwater runoff better.
Implications to the optimization of stormwater runoff control
PSD is closely associated with the mobility, settleability, pollutant entrainment, and treatment performance of stormwater particles (Selbig et al. 2016). Therefore, the adoption of Tur/TSS as a surrogate for PSD of stormwater runoff is of great significance to stormwater runoff control. The positive correlations of Tur/TSS with HM accumulation indicate that stormwater with higher Tur/TSS will pose significantly higher toxicity to urban waterways than stormwater with lower Tur/TSS. Moreover, stormwater with higher Tur/TSS tends to exhibit weaker settleability and, thus, low treatment performance of particles due to the finer PSDs. Therefore, it is highly suggested to control the stormwater with high Tur/TSS to preserve waterways.
The relationships between stormwater particles and rainfall characteristics are favorable for the identification of stormwater runoff with different pollution conditions. The negative correlations of turbidity and TSS with runoff time indicate the high pollution potential of stormwater runoff in the initial periods and low pollution potential in the middle and later periods. However, the negative relationship between Tur/TSS and rainfall intensity indicates that stormwater runoff with high Tur/TSS generates under low rainfall intensity and, thus, has a much smaller volume, while stormwater runoff with low Tur/TSS generates under high rainfall intensity and has a relatively larger volume. Therefore, the intra-event variations of Tur/TSS vary with rain types. Following this, the characteristics of stormwater are classified based on the particle properties and presented in Table 3. The corresponding SCMs are also suggested with the consideration of detention storage, pollution loading, and control effectiveness of particles. As presented by Jia et al. (2013), sedimentation and filtration are the most effective particle treatment processes, followed by infiltration. Hence, for stormwater with high Tur/TSS, TSS, and turbidity, SCMs such as infiltration trench and constructed wetland are suggested, because they are tolerant to high pollution loading and capable of removing particles effectively by sedimentation and filtration. For stormwater with high Tur/TSS, low turbidity, and TSS, SCMs suggested include sand filter, infiltration trench, and bioretention. These systems with low detention storage are tolerant to low pollution loading and capable of removing particles effectively by filtration. For stormwater with low Tur/TSS, high turbidity, and TSS, SCMs suggested include wet detention basin, infiltration basin, and dry detention basin. These systems with high detention storage are tolerant to high pollution loading and capable of removing particles effectively by sedimentation. For stormwater with low Tur/TSS, low turbidity, and TSS, SCMs suggested include bioretention and grass swale. These systems with medium detention storage are tolerant to low pollutant loading and capable of removing particles by sedimentation.
Conclusions
The quotient of Tur/TSS is proposed and validated, for the first time, as an effective surrogate for bulk PSD and quality of stormwater runoff. The results show that Tur/TSS increases significantly with finer sizes of both RDS and stormwater runoff. For stormwater particles, Tur/TSS positively correlates with the very fine (0.314~5.21 μm) particles, while it negatively correlates with coarser (14.5~45.6 μm) particles. Moreover, the concentrations of HMs in stormwater particles vary significantly during rainfall events and show significant increases with Tur/TSS. These results confirm the effectiveness of Tur/TSS as a particle parameter for characterizing the PSD and quality of stormwater runoff. Rainfall intensity predominantly influenced the intra-event variations of Tur/TSS. Hence, stormwater runoff with higher Tur/TSS generates under low intensity and, thus, characterizes small volume, weak settleability, higher mobility, and bioavailability. Conversely, stormwater runoff with lower Tur/TSS generates under high intensity and, thus, characterizes large volume, good settleability, low mobility, and bioavailability. Therefore, Tur/TSS can aid the optimal selection and design of appropriate SCMs for effective control of stormwater particles and pollutant transport.
References
Béchet B, Durin B, Legret M, Le Cloirec PL (2009) Size fractionation of heavy metals in highway runoff waters. Highway and Urban Environment 17: 235–244
Bach PM, Mccarthy DT, Deletic A (2010) Redefining the stormwater first flush phenomenon. Water Res 44(8):2487–2498. https://doi.org/10.1016/j.watres.2010.01.022
Beck HJ, Birch GF (2012) Metals, nutrients and total suspended solids discharged during different flow conditions in highly urbanised catchments. Environ Monit Assess 184(2):637–653. https://doi.org/10.1007/s10661-011-1992-z
Bian B, Lin C, Wu H (2015) Contamination and risk assessment of metals in road-deposited sediments in a medium-sized city of China. Ecotox Environ Safe 112:87–95. https://doi.org/10.1016/j.ecoenv.2014.10.030
Blecken G-T, Rentz R, Malmgren C, Öhlander B, Viklander M (2012) Stormwater impact on urban waterways in a cold climate: variations in sediment metal concentrations due to untreated snowmelt discharge. J Soils Sediments 12(5):758–773. https://doi.org/10.1007/s11368-012-0484-2
Borris M, Osterlund H, Marsalek J, Viklander M (2016) Contribution of coarse particles from road surfaces to dissolved and particle-bound heavy metal loads in runoff: a laboratory leaching study with synthetic stormwater. Sci Total Environ 573:212–221. https://doi.org/10.1016/j.scitotenv.2016.08.062
Charters FJ, Cochrane TA, O'Sullivan AD (2015) Particle size distribution variance in untreated urban runoff and its implication on treatment selection. Water Res 85:337–345. https://doi.org/10.1016/j.watres.2015.08.029
Chow MF, Yusop Z (2014) Sizing first flush pollutant loading of stormwater runoff in tropical urban catchments. Environ Earth Sci 72(10):4047–4058. https://doi.org/10.1007/s12665-014-3294-6
El-Mufleh A, Bechet B, Basile-Doelsch I, Geffroy-Rodier C, Gaudin A, Ruban V (2014) Distribution of PAHs and trace metals in urban stormwater sediments: combination of density fractionation, mineralogy and microanalysis. Environ Sci Pollut Res Int 21(16):9764–9776. https://doi.org/10.1007/s11356-014-2850-7
Everett DH (1988) Basic principles of colloid science. Royal Society of Chemistry
Fassman E (2012) Stormwater BMP treatment performance variability for sediment and heavy metals. Sep Purif Technol 84:95–103. https://doi.org/10.1016/j.seppur.2011.06.033
Ferreira M, Stenstrom MK (2013) The importance of particle characterization in stormwater runoff. Water Environ Res 85(9):833–842. https://doi.org/10.2175/106143013X13736496909103
Fulazzaky MA, Khamidun MH, Yusof B (2013) Sediment traps from synthetic construction site stormwater runoff by grassed filter strip. J Hydrol 502:53–61. https://doi.org/10.1016/j.jhydrol.2013.08.019
German J, Svensson G (2002) Metal content and particle size distribution of street sediments and street sweeping waste. Water Sci Technol 46:191
Gunawardana C, Egodawatta P, Goonetilleke A (2014) Role of particle size and composition in metal adsorption by solids deposited on urban road surfaces. Environ Pollut 184:44–53. https://doi.org/10.1016/j.envpol.2013.08.010
Gunawardana C, Goonetilleke A, Egodawatta P, Dawes L, Kokot S (2012) Source characterisation of road dust based on chemical and mineralogical composition. Chemosphere 87(2):163–170. https://doi.org/10.1016/j.chemosphere.2011.12.012
Hallberg M, Renman G, Lundbom T (2006) Seasonal variations of ten metals in highway runoff and their partition between dissolved and particulate matter. Water Air Soil Pollut 181:183–191
Helmreich B, Hilliges R, Schriewer A, Horn H (2010) Runoff pollutants of a highly trafficked urban road—correlation analysis and seasonal influences. Chemosphere 80(9):991–997. https://doi.org/10.1016/j.chemosphere.2010.05.037
Herngren L, Goonetilleke A, Ayoko GA (2005) Understanding heavy metal and suspended solids relationships in urban stormwater using simulated rainfall. J Environ Manag 76(2):149–158. https://doi.org/10.1016/j.jenvman.2005.01.013
Huang J, Tu Z, Du P, Li Q, Lin J (2011) Analysis of rainfall runoff characteristics from a subtropical urban lawn catchment in South-east China. Front Env Sci Eng 6: 531–539
Jia H, Yao H, Tang Y, SL Y, Zhen JX, Lu Y (2013) Development of a multi-criteria index ranking system for urban runoff best management practices (BMPs) selection. Environ Monit Assess 185(9):7915–7933. https://doi.org/10.1007/s10661-013-3144-0
Kim JY, Sansalone JJ (2008) Event-based size distributions of particulate matter transported during urban rainfall-runoff events. Water Res 42(10-11):2756–2768. https://doi.org/10.1016/j.watres.2008.02.005
Kim S-W, Park J-S, Kim D, Oh J-M (2013) Runoff characteristics of non-point pollutants caused by different land uses and a spatial overlay analysis with spatial distribution of industrial cluster: a case study of the Lake Sihwa watershed. Environ Earth Sci 71:483–496
Liu A, Liu L, Li D, Guan Y (2015) Characterizing heavy metal build-up on urban road surfaces: implication for stormwater reuse. Sci Total Environ 515-516:20–29. https://doi.org/10.1016/j.scitotenv.2015.02.026
Maniquiz-Redillas MC, Geronimo FK, Kim LH (2014) Investigation on the effectiveness of pretreatment in stormwater management technologies. J Environ Sci (China) 26(9):1824–1830. https://doi.org/10.1016/j.jes.2014.06.018
McKenzie ER, Wong CM, Green PG, Kayhanian M, Young TM (2008) Size dependent elemental composition of road-associated particles. Sci Total Environ 398(1-3):145–153. https://doi.org/10.1016/j.scitotenv.2008.02.052
Metadier M, Bertrand-Krajewski JL (2012) The use of long-term on-line turbidity measurements for the calculation of urban stormwater pollutant concentrations, loads, pollutographs and intra-event fluxes. Water Res 46(20):6836–6856. https://doi.org/10.1016/j.watres.2011.12.030
Miguntanna NP, Liu A, Egodawatta P, Goonetilleke A (2013) Characterising nutrients wash-off for effective urban stormwater treatment design. J Environ Manag 120:61–67. https://doi.org/10.1016/j.jenvman.2013.02.027
Peng HQ, Liu Y, Wang HW, Gao XL, Ma LM (2016) Event mean concentration and first flush effect from different drainage systems and functional areas during storms. Environ Sci Pollut Res Int 23(6):5390–5398. https://doi.org/10.1007/s11356-015-5657-2
Rügner H, Schwientek M, Beckingham B, Kuch B, Grathwohl P (2013) Turbidity as a proxy for total suspended solids (TSS) and particle facilitated pollutant transport in catchments. Environ Earth Sci 69(2):373–380. https://doi.org/10.1007/s12665-013-2307-1
Selbig W, Fienen M, Horwatich J, Bannerman R (2016) The effect of particle size distribution on the design of urban stormwater control measures. Water 8(1):17. https://doi.org/10.3390/w8010017
Soltani N, Keshavarzi B, Moore F, Tavakol T, Lahijanzadeh AR, Jaafarzadeh N, Kermani M (2015) Ecological and human health hazards of heavy metals and polycyclic aromatic hydrocarbons (PAHs) in road dust of Isfahan metropolis, Iran. Sci Total Environ 505:712–723. https://doi.org/10.1016/j.scitotenv.2014.09.097
Wang Q, Zhang Q, Wu Y, Wang XC (2017) Physicochemical conditions and properties of particles in urban runoff and rivers: implications for runoff pollution. Chemosphere 173:318–325. https://doi.org/10.1016/j.chemosphere.2017.01.066
Wijesiri B, Egodawatta P, McGree J, Goonetilleke A (2015) Influence of pollutant build-up on variability in wash-off from urban road surfaces. Sci Total Environ 527-528:344–350. https://doi.org/10.1016/j.scitotenv.2015.04.093
Yahyapour S, Golshan A, Ghazali AH (2014) Removal of total suspended solids and turbidity within experimental vegetated channel: optimization through response surface methodology. J Hydro-Environ Res 8(3):260–269. https://doi.org/10.1016/j.jher.2013.03.004
Ying G, Sansalone J (2010) Particulate matter and metals partitioning in highway rainfall-runoff. Front Env Sci Eng 4: 35–46
Yun Y, Park H, Kim L, Ko S (2010) Size distributions and settling velocities of suspended particles from road and highway. KSCE J Civ Eng 14(4):481–488. https://doi.org/10.1007/s12205-010-0481-1
Zhang J, Krebs P (2013) Comments on “Characterising metal build-up on urban road surfaces” by . Environmental Pollution 176, 87–91. Environ Pollut 182: 500–502, DOI: https://doi.org/10.1016/j.envpol.2013.07.009
Zhang Q, Wang X, Hou P, Wan W, Ren Y, Ouyang Z, Yang L (2013) The temporal changes in road stormwater runoff quality and the implications to first flush control in Chongqing, China. Environ Monit Assess 185(12):9763–9775. https://doi.org/10.1007/s10661-013-3289-x
Zhao H, Li X (2013) Understanding the relationship between heavy metals in road-deposited sediments and washoff particles in urban stormwater using simulated rainfall. J Hazard Mater 246-247:267–276. https://doi.org/10.1016/j.jhazmat.2012.12.035
Zhao H, Chen X, Hao S, Jiang Y, Zhao J, Zou C, Xie W (2016) Is the wash-off process of road-deposited sediment source limited or transported limited? Sci Total Environ 563-564:62–70. https://doi.org/10.1016/j.scitotenv.2016.04.123
Funding
This study is supported by the National Natural Science Foundation of China (Grant No.51508447), the National Program of Water Pollution Control in China (Grant No. 2014ZX07305-002), the National Key Technology Support Program (Grant No.2014BAC13B06), and Program for Innovative Research Team in Shaanxi (PIRT) (Grant No. 2013KCT-13).
Author information
Authors and Affiliations
Corresponding author
Additional information
Responsible editor: Philippe Garrigues
Electronic supplementary material
ESM 1
(DOCX 332 kb)
Rights and permissions
About this article
Cite this article
Wang, Q., Zhang, Q., Dzakpasu, M. et al. Development of an indicator for characterizing particle size distribution and quality of stormwater runoff. Environ Sci Pollut Res 25, 7991–8001 (2018). https://doi.org/10.1007/s11356-017-1074-z
Received:
Accepted:
Published:
Issue Date:
DOI: https://doi.org/10.1007/s11356-017-1074-z