Abstract
The past 100 years have witnessed an evolution of the meaning of validity and validation within the fields of education and psychology. Validity was once viewed as a property of tests and scales, but is now viewed as the extent to which theory and evidence support proposed interpretations and uses of test scores. Uncertainty about what types of validity evidence were needed motivated the current “argument-based” approach, as reflected in the 2014 Standards for Educational and Psychological Testing. According to this approach, investigators should delineate the assumptions required in order for a proposed interpretation or use to be plausible and then seek evidence that supports or refutes those assumptions. Though validation practices within the field of patient-reported outcome measurement have implicitly included many elements of the argument-based approach, the approach has yet to be explicitly adopted. To facilitate adoption, this article proposes an initial set of assumptions that might be included in most arguments for research-related interpretations and uses of scores from patient-reported outcome measures. The article also includes brief descriptions of the types of evidence that would be best suited for evaluating each assumption. It is hoped that these generic assumptions will stimulate further discussion and debate among quality of life researchers regarding how best to adopt modern validity theory to patient-reported outcome measures.
Similar content being viewed by others
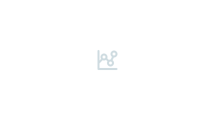
Avoid common mistakes on your manuscript.
Plain language summary
Patient-reported Outcome Measurements (PROMs) are a way to measure information provided directly by the patient. In order for PROMs to be used in a way that helps researchers understand how a patient’s health and feelings have changed in response to a treatment, the PROMS must be suitable for use in a study. This article describes ideas about how to make sure a PROM is suitable for use. These ideas have already been used in psychology and education.
The ideas are:
-
1.
The PROM should reflect all of what is being studied, not just part.
-
2.
The patient should understand the questions and the possible responses.
-
3.
The responses should not be unduly affected by other things, like cultural backgrounds or reading level. (The word “unduly” is included here to mean “in a major way”, because many things may affect how a person responds.)
-
4.
Responses are usually given a score, for example, “very likely” might equal 5. All responses are added and turned into a score. This score should make sense.
-
5.
The score should describe how patients actually feel or function in their daily lives.
-
6.
The scores should be “sensitive enough,” meaning they are actually able to show differences in patients who receive a certain treatment over time.
The goal of this manuscript is to convince other researchers to think deeply about validity and to inspire them to consider new approaches.
Introduction
Any published article that describes the development and evaluation of a new patient-reported outcome measure (PROM) will contain multiple references to validity. However, there are few, if any, articles in the field of PROMs that discuss the theory of validity being used [1]. Ideas about what validity is and how one evaluates it have undergone debate and change within the fields of education and psychology—fields from which PROM researchers imported notions of validity decades ago. This evolution has been described in detail elsewhere [2] including an excellent article in this journal by Edwards et al. [3].
In education and psychology, the current framework for validity was codified in the 2014 Standards for Psychological and Educational Testing [4] (hereafter referred to as “the Standards”). The Standards defines validity as “the degree to which evidence and theory support the interpretations of test scores for proposed uses of tests” [4]. This means that validity is not a property of a measure itself, but rather of empirically supported interpretations and uses of a measure’s scores. Validation is thus “a process of constructing and evaluating arguments for and against the intended interpretation of test scores and their relevance to the proposed use” [4]. Note the use of the word “argument.” The premise is that validity starts with a logical argument regarding the intended interpretation and/or use of scores. [2, 5] The argument consists of important assumptions that underlie a proposed interpretation/use of scores from some measure. Within this framework, the purpose of collecting evidence is to evaluate the plausibility of the argument and, hence, the proposed interpretation/use of scores.
This approach was developed in response to confusion about what types and levels of evidence are needed in validity analyses. In the health outcomes field, this confusion has led to a checklist-based approach to validation that often resembles, as Zumbo et al. [1] called it, “stamp collecting.” Researchers may conduct as many kinds of validity analyses as they can to “cover all the bases.” The motivating question seems to be, “Can I develop a broad base of evidence that supports the validity of this measure?” The challenges with this approach are twofold: First, it is unclear how each validation analysis contributes specifically to validity, aside from the sense that “more is better.” Because of this, there is a greater risk of failing to collect the most relevant type of evidence in a specific situation or of spending time and resources collecting validity evidence that does not lead to a significant incremental improvement in overall validity. In contrast, validity analyses in the argument-based approach are precisely targeted toward evaluating the plausibility of the key assumptions underlying a proposed interpretation/use of PROM scores. The motivating question is, “What are the biggest threats to the reasonableness of my interpretation/use?” The articulation of assumptions and threats guides the validity analyses plan, and the results of those analyses modulate our comfort with the assumptions.
While there are examples of the argument-based approach within the field of education [6,7,8,9], only Hawkins et al. [8] has applied this framework to PROMs to illustrate how one might create and support an argument for the validity of an interpretation/use of scores from a translation of a health literacy measure. More generally, Edwards et al. [3] called for greater consideration of how the argument-based approach can be incorporated into regulatory decision-making regarding PROMs. The argument-based framework has not yet been adopted widely by PROMs researchers, perhaps because of lack of awareness of this approach (of which I have been guilty) and/or lack of knowledge regarding how to apply it to PROMs. In this article, I briefly introduce the major elements of the argument-based approach to validity and then offer a set of assumptions that could be included in arguments for the interpretation/use of PROM scores in research settings. I hope this will promote further discussion among PROM researchers about how to best craft validity arguments and provide insights for developing a compelling rationale for the interpretation/use of PROM scores.
The argument-based approach to validity is an evolution, not a revolution. Most of the older ideas and empirical strategies remain, but are reframed to promote greater clarity and efficiency in validation practices. Therefore, a turn toward the argument-based approach does not require a rejection of the strong qualitative and quantitative validity work that has been done in the past.
Overview of the argument-based approach to validity
The argument-based approach begins with a clear statement of the proposed interpretation/use of scores. For example, one might propose that scores on a specific PROM can be interpreted as the level of some symptom or function, where the symptom or function will be referred to as the concept. The same score could be interpreted in different ways. For example, a score computed from multiple items that ask about how far and how easily one can walk could be interpreted as an indicator of ease and extent of walking, of lower mobility, or of physical functioning. Note that the first interpretation follows straightforwardly from the items themselves, whereas the second and third would require further assumptions and justification in order to be plausible. One might further propose that the scores on the PROM may be used, for example, to make decisions about who has and has not responded positively to treatment, to compare average levels of functioning between experimental groups, to select patients who are eligible to participate in a clinical trial, etc. The argument-based approach requires explicitly stating the use(s) and/or interpretations that will be under investigation. Different arguments might be required for each of these uses of a score to be reasonable.
By explicating the argument underlying the proposed interpretation/use of PROM scores, one crafts what the Standards call the rationale and what Kane [2] refers to as the Interpretation/Use Argument (IUA). An example of a rationale from the Standards involves using scores on a Mathematics Achievement Test to assess students’ readiness for an advanced course (Table 1). Once the rationale has been described, one can evaluate each assumption of the rationale by collecting empirical evidence, conducting literature reviews, obtaining expert consensus, and/or conducting a logical/conceptual analysis. The Standards describe different types of evidence used in this process. The chief types of evidence are those based on test content, response processes, internal structure, and relations to other variables (convergent, discriminant, and test-criterion). Note that the older framework’s “types of validity” (e.g., content validity, convergent validity, discriminant validity) has been recast as “types of evidence.” But whereas in the older framework it was unclear which “types of validity” were relevant, in the argument-based approach, whether collecting a given “type of evidence” is desirable depends solely on whether it could inform the plausibility of the argument being made.
As Kane states, “if the IUA is coherent and complete and if all of its inferences and assumptions are plausible given the evidence, the proposed interpretations and uses can be considered valid. If the IUA is incomplete or if some of its inferences or assumptions are shaky, the validity argument is inadequate [2].”
Note that an additional type of evidence discussed in modern validity theory concerns the potential positive or negative consequences of applying a decision rule based on a test score. In the context of PROMs, one such rule might involve selecting participants for inclusion in a clinical trial of a new medical product based on their scores on some PROM. If there is substantial differential item functioning (DIF) between demographic groups, then some groups might be unnecessarily excluded from participation, raising concerns about justice.
Common assumptions for an argument-based approach for the interpretation/use of prom scores in research setting
In the context of educational and psychological testing, Kane [2] proposed that many IUAs would contain the same basic assumptions. In this section, I adapt and extend Kane’s list of assumptions to PROMs in research settings. As described above, PROM scores could be interpreted and used in different ways; thus no single argument can be offered that fits perfectly with every proposed interpretation/use. However, it is possible to describe several assumptions that are likely to be a part of most arguments for a wide range of interpretations and uses of PROM scores in research contexts. Identifying these common assumptions may be useful for researchers who are constructing appropriate arguments for their own situations.
There are three key considerations for reviewing the common assumptions that follow. First, the concept being assessed should be a feeling or function that patients care about [10]. Second, while the validity argument is an argument for interpreting/using PROM scores in a particular way, the assumptions that make up the argument and the supporting evidence will involve multiple aspects of the PROM aside from the scoring (e.g., the measure’s content and the patient’s interpretations and responses). Third, an argument for the interpretation/use of PROM scores may be expressed in different ways (e.g., different terms or phrasing). With experience, our scientific community could become more adept at expressing these arguments in an efficient and effective way. In this spirit, the following assumptions should be considered as a starting point for discussion and debate (see Table 2 for summary).
Assumption A: The PROM’s item content reflects all of the important aspects of the concept.
The content of the PROM refers to the substance of the items and response options that make up the measure. In order for scores on a PROM to be interpreted as indicators of a patient’s status with respect to the concept, the content of the PROM must reflect the entirety of the concept. Failure to satisfy this assumption is known as construct underrepresentation in the Standards.
Evaluating the plausibility of this assumption necessitates clearly specifying the meaning of the concept, including its scope and all relevant aspects, and conducting a logical analysis to ensure alignment between the item content and the full scope of the concept. Ideally such an analysis would incorporate multiple perspectives (e.g., clinicians, measurement experts, patients, and caregivers) through some consensus process. The results of the analysis can be expressed as a mapping of specific items to specific aspects of the concept.
Assumption B: Patients understand the items and response options as intended
Assumption A above concerns the semantic meaning of the items and response options. However, patients’ understanding of the items and their intentions in providing their responses might differ from the intentions of the measure developer [11]. If this is true, the patients’ responses are not truly reports of the concept. Though it is sometimes challenging to obtain, support for this assumption could come directly from cognitive interviews [12, 13].
Assumption C: Scores on the PROM are not unduly influenced by factors that are not part of the concept
This assumption corresponds to what the Standards refer to as construct contamination. Many factors can influence a patient’s response to a PROM besides the patient’s underlying status with respect to the concept, including faulty memory, cultural variations in the understanding of the items, different agendas and intentions, the patient’s current mood, etc. A patient’s response to a PROM is complex discursive production [11, 14, 15]. Therefore, it is naïve to believe a response is solely a true indication of the person’s status on the concept. This is why “unduly” is used in expressing this assumption: factors external to the concept should not overwhelm the PROM scores such that they no longer serve their intended purpose. To evaluate this assumption, one should consider the most likely influences on patients’ responses to the items and assess the presence and strength of those influences. Thus, Assumption C serves as a general label for a larger collection of specific assumptions concerning particular sources of influence that might be relevant for different interpretations/uses of PROMs, including the following:
Assumption C.1: The PROM's item content does not include issues beyond the concept
The PROM’s item content might address all of the important aspects of the concept (Assumption A), but it is still possible that the PROM contains items that are querying patients about issues other than the concept of interest. For example, a researcher might propose to use a PROM to assess a person’s ability with respect to activities of daily living. The PROM might include items that query how well the person can do the activities of interest (e.g., toileting, bathing), but might also include an item about how satisfied the person feels with their abilities. The content of this satisfaction item is not consistent with the concept of interest, which is the ability to do activities of daily living. Including such items in the computation of PROM scores could result in a contamination of the scores, making it less likely that the scores can be interpreted as reflecting the concept. To evaluate whether items that are irrelevant to the concept are included in the PROM, one can examine the logical alignment between the item content and the full scope of the concept, as described under Assumption A.
Assumption C.2: Differences in linguistic/cultural backgrounds do not lead to substantially different interpretations of the items
Evidence in support of this assumption could take several forms. The process of language translation and/or cultural adaptation (including cognitive interviews) could be described to support the quality of the resulting translation/adaptation. When appropriate, one could also present evidence of measurement invariance, which might include statistical tests for DIF.
Assumption C.3: Differences in patients’ literacy or educational attainment do not lead to substantially different interpretations of the items
Supporting evidence could include readability diagnostics of the instructions and items and/or cognitive interviews that include participants with a range of education and literacy levels. When appropriate, one could present evidence of measurement invariance (including DIF testing) across literacy/education groups.
Assumption C.4: Errors of recollection do not unduly influence assessment of the concept (for measures that use a recall period)
Sources of evidence for this assumption could include cognitive interviews to explore memory retrieval processes, studies of recall accuracy, or literature reviews of the accuracy of recall for similar concepts and items.
Assumption C.5: Different modes of assessment do not lead to substantially different scores on the PROM
Sources of evidence for this assumption could include empirical comparisons of PROM scores and measurement properties across different modes of administration (including testing DIF), as well as literature reviews or meta-analyses of mode effects for similar concepts and items [16, 17].
Assumption C.6: The patient’s status on related, but separate, health domains does not unduly influence scores on the PROM
For some measures there might be a concern that the scores are contaminated by some other concept. For example, in educational testing, scores thought to assess analogical reasoning might be contaminated by the respondents’ vocabulary level. In health status assessment, an example might be the worry that chemotherapy-induced fatigue is influencing patients’ scores on a PROM designed to measure depression. If this is a concern, sponsors/tool developers can evaluate the empirical relationship between scores on the PROM and the patients’ status on the domain that might interfere, which would be an example of discriminant evidence. Note that this type of evidence is only needed when there is a concern about the interfering influence of some factor that is similar to, but different from, the concept of interest. In many cases, we might not have this concern. For example, few would worry that there existed some closely related but distinct health experience that would somehow influence scores on a measure of itchiness. The closest concepts might be pain or burning, but it is unlikely that someone’s ratings of itchiness are really reflecting pain and/or burning.
Assumption D: The method of scoring responses to the item(s) of the PROM is appropriate for assessing the concept
This assumption entails two different inferences—the scoring inference and the scaling inference.
-
(1)
Scoring inference. The scoring inference “specifies the rules by which particular respondent behaviors are coded” (p.1717) into item-level scores [3]. For most PROMs, the patient’s behavior is to select a response option (e.g., “3” or “somewhat likely”), which, if not already a number, is assigned a corresponding value (e.g., “very likely” = 5). The plausibility of the scoring inference requires that the patient’s experience can be meaningfully mapped onto the response options provided for an item. Support for this assumption could come from cognitive interviews, quantitative investigations of floor or ceiling effects at the item level, and/or item-response theory (IRT)-based item characteristic curves to evaluate the possibility of redundant or overlapping response categories.
-
(2)
Scaling inference. When multiple items are used to measure a PROM concept, the scaling inference specifies the rules by which responses to multiple items are combined to arrive at a score. The approach for combining responses to multiple items is often expressed as a measurement model that relates responses to particular items to the concept(s) being measured. The chief types of measurement models are: (a) the reflective [18] or effect indicator model in which inferences about the underlying attribute of the patient (e.g., fatigue) are based on multiple items thought to be the causal effects or reflections of the underlying attribute; (b) the causal indicator model [18, 19] in which inferences about the underlying status of the patient are based on multiple items that measure different causes of the patient’s status; and (c) the composite indicator [20] model in which multiple items are combined to define a composite variable (e.g., Activities of Daily Living). The rationale and justification for combining items will depend upon the particular measurement model chosen for the PROM. Specific assumptions associated with scaling inference could include:
-
a.
The measurement model makes conceptual sense for the concept and the items that are indicators of the concept.
-
b.
In the case of a reflective or causal indicator model, the model provides acceptable fit to the response data.
-
c.
Interpretation of scores is not unduly compromised by deviations from any statistical assumptions of the model.
-
d.
The scoring rule does not create bias with respect to one group of patients versus another.
-
a.
Assumption E: Scores from the PROM correspond to how patients actually feel and/or function in their daily lives
Regardless of the specific purpose of PROMs in a research study, the scores should reflect how the patient feels and/or functions in their daily lives during the period of interest (e.g., past 7 days). As Walton et al. [10] observe, PROMs provide a relatively direct assessment of a patient’s actual health experience, whereas some other types of clinical outcome assessments (e.g., performance-based measures) have a less direct relationship. If care has been taken to justify the preceding assumptions A through D of the rationale, then the PROM scores probably tell us about what is happening in the patient’s life. Another way of looking at this assumption is through viewing PROM-based assessment as a kind of standardized conversation with patients in which we ask them directly about how they feel or function [11]. In the less formal conversations about people’s health that occur every day, we accept as true what people tell us about some aspect of their health so long as we are confident that (a) we share an understanding of relevant health-related language with our interlocutor, (b) we have faith that the person is not trying to mislead us [21], and (c) that the person has the cognitive capacity to render a reasonably accurate report of their experience. To the extent that these conditions are met, we have greater confidence in the truth of assumption E.
However, if there remains doubt the PROM corresponds to the way patients feel and/or function in their daily lives, evidence about the relationship between the PROM scores and the actual experiences of the patients can be collected. One might seek convergent evidence in the form of relationships between scores on the PROM and values of other variables that are expected to be associated with the real health experience(s) of interest. The other variables may include measures of the concept that use alternative methods and/or sources (e.g., observer report or performance tests) or any demographic or clinical variables known to be related to the aspect of health under study.
Assumption F: Scores from the PROM are sensitive enough to reflect differences between patients and/or within patients over time in levels of the concept being measured
Scores on the PROM might reflect the real health experiences of patients (Assumption E), but the assessments might not have sufficient sensitivity to detect important differences between patients or within patients over time. This could be due to measurement error and/or a lack of sufficient granularity. Thus, a key element of any argument for the interpretation/use of PROM scores in research is that the scores are sensitive enough to detect differences of interest. A PROM could be used in a study to assess differences between groups of patients or within a group of patients over time. An investigator would need to be clear about which type of difference is in question and seek support that demonstrates sensitivity to the differences of interest. There are two general approaches for evaluating evidence related to this assumption—direct and indirect.
Direct evidence for sensitivity to differences
The direct approach assesses whether consequential differences between patients or within-patients over time can be assessed by the PROM. Direct evidence for between-person sensitivity could come from empirical investigations of how well scores on the PROM can differentiate between patients who are known to vary with respect to the concept of interest. For example, an investigator might determine how well scores on the PROM can discriminate among patients in each of four categories of disease severity (i.e., using known groups evidence). Direct evidence for sensitivity to within-person change (i.e., responsiveness) could come from a demonstration that scores on the PROM show change over time in a patient group known to change (e.g., in response to a treatment with known efficacy). Alternatively, one could examine the relationship between changes in individual’s PROM scores and changes in some other established indicator(s) of disease severity. These evaluations should demonstrate that the PROM detects a difference that is as small as or smaller than the type of differences the investigators wish to detect.
Indirect evidence for sensitivity to differences
Indirect evidence can be obtained from evaluations of reliability/precision of PROM scores to determine whether the PROM has the requisite sensitivity to detect differences if differences exist. The assumption being investigated is that there is negligible variance in PROM scores due to inconsistency across independent replications of the assessment procedure [4]. What might be the “independent replications” for a PROM? The most straightforward example is two or more assessments on the same group of clinically stable patients over time, i.e., test–retest reliability. Variation in scores of stable patients over time would be interpreted as score inconsistency. Modern testing emphasizes the need to identify the type of replications over which scores are expected to be consistent and to compute an estimate of reliability based on that type of replication. (Note that IRT models can also provide an estimate of error as the inverse of the information function, though this estimate is more challenging to conceptualize in terms of replications [22]).
Conclusion
Developments in validity theory within education and psychology have led to an argument-based approach. In this approach, the intended interpretation/use of a score is explicitly stated, as are the assumptions required for that interpretation/use to be reasonable. This clarifies the type and amount of evidence that is needed to support those assumptions. To date, this approach has not been widely applied to PROMs. The intent of this article has been to offer general assumptions that might comprise an argument for a particular interpretation/use of PROM scores in research settings. It is hoped that these assumptions will be helpful to researchers as they apply the argument-based approach to validation efforts with PROMs. I also hope that this will inspire others to refine, correct, and/or add to this set of assumptions so that our field can implement this approach to validity in the most effective way.
References
Zumbo, B. C. E. (2014). Validity and validation in social, behavioral, and health sciences social indicators research series. Cham: Springer.
Kane, M. T. (2013). Validating the interpretations and uses of test scores. Journal of Educational Measurement, 50(1), 1–73. https://doi.org/10.1111/jedm.12000.
Edwards, M. C., Slagle, A., Rubright, J. D., & Wirth, R. J. (2017). Fit for purpose and modern validity theory in clinical outcomes assessment. Quality of Life Research: An International Journal of Quality of Life Aspects of Treatment, Care and Rehabilitation, 14(2), 1–10. https://doi.org/10.1007/s11136-017-1644-z.
Association, A. E. R., Association, A. P., & Education, N. C. o. M. i. Standards for educational and psychological testing (American Educational Research Association): American Educational Research Association.
Kane, M. T. (2013). Validation as a pragmatic, scientific activity. Journal of Educational Measurement, 50(1), 115–122. https://doi.org/10.1111/jedm.12007.
Cook, D. A., Brydges, R., Ginsburg, S., & Hatala, R. (2015). A contemporary approach to validity arguments: A practical guide to Kane’s framework. Medical Education, 49(6), 560–575. https://doi.org/10.1111/medu.12678.
Hatala, R., Cook, D. A., Brydges, R., & Hawkins, R. (2015). Constructing a validity argument for the objective structured assessment of technical skills (OSATS): A systematic review of validity evidence. Advances in Health Sciences Education Theory and Practice, 20(5), 1149–1175. https://doi.org/10.1007/s10459-015-9593-1.
Hawkins, M. (2018). Application of validity theory and methodology to patient-reported outcome measures (PROMs): Building an argument for validity. Quality of Life Research : An International Journal of Quality of Life Aspects of Treatment, Care and Rehabilitation, 27(7), 1695–1710. https://doi.org/10.1007/s11136-018-1815-6.
Reeves, T. D., & Marbach-Ad, G. (2016). Contemporary test validity in theory and practice: A primer for discipline-based education researchers. CBE Life Sciences Education, 15(1), 11. https://doi.org/10.1187/cbe.15-08-0183.
Walton, M. K., Powers, J. H., Hobart, J., Patrick, D., Marquis, P., Vamvakas, S., et al. (2015). Clinical outcome assessments: Conceptual foundation—report of the ISPOR clinical outcomes assessment—emerging good practices for outcomes research task force. Value in Health, 18, 741–752.
Weinfurt, K. P. (2019). Viewing assessments of patient-reported heath status as conversations: Implications for developing and evaluating patient-reported outcome measures. Quality of Life Research : An International Journal of Quality of Life Aspects of Treatment, Care and Rehabilitation, 13(1), 1–7. https://doi.org/10.1007/s11136-019-02285-8.
Willis, G. B. (2005). Cognitive interviewing: A tool for improving questionnaire design. Thousand Oaks: Sage.
Willis, G. B. (2015). Analysis of the cognitive interview in questionnaire design. Oxford: Oxford University Press.
McClimans, L. (2010). Towards self-determination in quality of life research: A dialogic approach. Medicine, Health Care, and Philosophy, 13(1), 67–76. https://doi.org/10.1007/s11019-009-9195-x.
McClimans, L. (2010). A theoretical framework for patient-reported outcome measures. Theoretical Medicine and Bioethics, 31(3), 225–240. https://doi.org/10.1007/s11017-010-9142-0.
Byrom, B., Gwaltney, C., Slagle, A., Gnanasakthy, A., & Muehlhausen, W. (2019). Measurement equivalence of patient-reported outcome measures migrated to electronic formats: A review of evidence and recommendations for clinical trials and bring your own device. Therapeutic Innovation & Regulatory Science, 53(4), 426–430. https://doi.org/10.1177/2168479018793369.
Eremenco, S., Coons, S. J., Paty, J., Coyne, K., Bennett, A. V., McEntegart, D., et al. (2014). PRO data collection in clinical trials using mixed modes: Report of the ISPOR PRO mixed modes good research practices task force. Value in Health, 17(5), 501–516. https://doi.org/10.1016/j.jval.2014.06.005.
Fayers, P. M., & Machin, D. (2016). Quality of life (3rd ed.). Blackwell: Wiley.
Costa, D. S. J. (2015). Reflective, causal, and composite indicators of quality of life: A conceptual or an empirical distinction? Quality of Life Research, 24(9), 1–9. https://doi.org/10.1007/s11136-015-0954-2.
Bollen, K. A., & Bauldry, S. (2011). Three Cs in measurement models: Causal indicators, composite indicators, and covariates. Psychological Methods, 16(3), 265–284. https://doi.org/10.1037/a0024448.
Gobo, G., & Mauceri, S. (2014). Constructing survey data. Thousand Oaks: Sage.
Brennan, R. L. (2001). An essay on the history and future of reliability from the perspective of replications. Journal of Educational Measurement, 38(4), 295–317. https://doi.org/10.1111/j.1745-3984.2001.tb01129.x.
Acknowledgements
I am grateful to my colleagues—Karon Cook, PhD, Kathryn Flynn, PhD, and Bryce Reeve, PhD, and Arthur Stone, PhD—who provided invaluable feedback on an earlier draft of this paper, helping me to understand what I was trying to say and in many cases providing better ways to say it. The manuscript was much improved thanks to suggestions from two anonymous reviewers. I am also indebted to Karen Staman, MS, who provided invaluable editorial support.
Funding
The author reports no funding for the current work.
Author information
Authors and Affiliations
Contributions
KW, Conceptualization and drafting of final manuscript. The author wrote the manuscript and is responsible for the ideas presented herein.
Corresponding author
Ethics declarations
Conflict of interest
The author declares that he has no conflict of interest.
Ethical approval
This article does not contain any studies with human participants performed by any of the authors.
Additional information
Publisher's Note
Springer Nature remains neutral with regard to jurisdictional claims in published maps and institutional affiliations.
Rights and permissions
About this article
Cite this article
Weinfurt, K.P. Constructing arguments for the interpretation and use of patient-reported outcome measures in research: an application of modern validity theory. Qual Life Res 30, 1715–1722 (2021). https://doi.org/10.1007/s11136-021-02776-7
Accepted:
Published:
Issue Date:
DOI: https://doi.org/10.1007/s11136-021-02776-7