Abstract
The current special issue of Prevention Science indicates that momentum in using individual participant data (IPD) and integrative data analysis (IDA) to combine and synthesize findings in prevention science has accelerated over the past decade. In this commentary, we focus on two general themes involving methods for harmonizing measures and findings of effect heterogeneity. We describe methods for harmonization as retrospective psychometrics, requiring that we attend to the assumptions necessary for accurate measurement, but adjust our methods given the constraints of working with existing datasets that often involve different measures in different studies. We point to novel approaches for increasing confidence that semantic matching and empirical modeling used in these studies will yield accurate and valid measurements that can be combined in IDA. We also review findings about effect heterogeneity, emphasizing the importance of using etiologic and action theories to identify and evaluate sources of such effects. We note that all of the papers in this issue deserve careful attention, as they illustrate how prevention scientists are approaching the complexities of IDA and exploring novel methods for overcoming its challenges.
Similar content being viewed by others
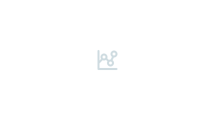
Explore related subjects
Discover the latest articles, news and stories from top researchers in related subjects.Avoid common mistakes on your manuscript.
Investigators began using individual participant data (IPD) to improve meta-analysis over 30 years ago (WHO, 1991), with Stewart and Parmar (1993) suggesting that analysis of individual patient data provided the least biased and most reliable basis for synthesizing data from clinical trials. Interest in using IPD for combining data from multiple studies in the social and psychological sciences accelerated following the seminal development of novel methods for harmonizing and analyzing IPD datasets by Curran, Bauer, Hussong, and their colleagues who introduced these methods under the general rubric of integrative data analysis (IDA) in a special issue of Psychological Methods (Bauer & Hussong, 2009; Curran, 2009; Curran & Hussong, 2009). In the journal of Prevention Science, Brown et al. (2013) recommended its use for studying moderation in the impact of prevention trials, and in 2018 Prevention Science published a supplemental issue (Howe et al., 2018) that included applications to trials studying the prevention of youth depression (Brown et al., 2018) and related methodology (Brincks et al., 2018; Siddique et al., 2018). More recent work in this journal dealt with potential biases with IDA when combining data from trials using group-level interventions (Brown et al., 2022).
The current special issue of Prevention Science indicates that momentum in using IPD and IDA to combine and synthesize findings in prevention science has not only continued but accelerated. This special issue contains 18 papers that apply IDA to study etiology, the effectiveness of prevention programs, and how the impact of prevention programs may vary across participants, times, and contexts. Papers also include tutorials on the use of new methods for harmonizing measures across studies, including semantic harmonization (McDaniel et al., 2023) and empirical methods employing moderated nonlinear factor analysis (MNLFA: Cole et al., 2023; Zhao et al., 2023). Other papers present advances in these techniques that broaden their application to count outcomes that include substantial zeroes (Mun et al., 2023; Saavedra et al., 2023), a common occurrence in studies of substance use; in the analysis of intensive longitudinal data in adaptive intervention studies (Potter et al., 2023); and in strengthening causal inference through applying propensity score matching when combining trial data with epidemiological data that includes intervention status (Saavedra et al., 2023), or dealing with systematic bias when testing differences in intervention impact across different populations or contexts (Barker et al., 2023).
The breadth and innovation of these works is exhilarating. In this commentary, we focus on two general themes involving methods for harmonizing measures and findings involving effect heterogeneity. But we emphasize that all of the papers in this issue deserve careful attention and illustrate how prevention scientists are approaching the complexities of IDA as applied to the field of prevention and exploring novel methods for overcoming its challenges.
Retrospective Psychometrics
As McDaniel et al. (2023) note, measure harmonization for IDA involves retrospective psychometrics. The field of psychometrics, broadly speaking, has built conceptual frameworks and a set of strategies for constructing and validating new measures as a means of increasing our confidence that those measures accurately and consistently represent phenomena of interest. We might describe this field as prospective psychometrics as measures are selected or generated to optimize their inferential capabilities in future studies. In contrast, harmonization for IDA is constrained by the data available, although it can contribute to refinement of measures for future research, including multi-trial follow-up designs (Brown et al., 2007). Harmonization methods require a conceptual framework that can provide guidance in how to combine existing data from multiple studies, often based on different measures of the same construct, to insure both accuracy and consistency across those studies. The papers in this special issue clarify that conceptual frameworks underlying standard psychometrics can provide a starting point for this work, but must be adapted in several ways.
We lean toward a causalist framework for evaluating measurement accuracy and consistency (Bollen & Lennox, 1991; Borsboom, 2008; Borsboom et al., 2003; van Bork et al., 2022), consistent with most of the work described in these papers. This framework presents several assumptions necessary for accurate measurement of construct states. All of these papers focus on outcomes measured as reflective constructs, assuming that construct states are latent and unobservable but can be inferred through their causal impact on observable indicators (Bollen & Lennox, 1991). Accurate measurement using reflective models requires clear and explicit definition of the construct of interest (McDaniel et al., 2023) and measurement methods that lead to data that meets several assumptions, including common cause, causal invariance, nonconfounding, and nomological validity (Howe et al., 2019). Prospective psychometrics has developed a set of strategies for constructing measurements that meet these requirements and has applied quantitative methods such as item response theory (IRT) or confirmatory factor analysis (CFA) modeling. These methods allow us to evaluate whether data are consistent with these assumptions, refine measures that fail to meet assumptions, and adjust estimates of construct states to reduce or eliminate violations. These methods have provided a foundation for the retrospective psychometrics employed in these papers, but have also required extensions to deal with the challenges of IDA datasets.
Common Cause
The common cause assumption holds that all observable indicators of a construct will be empirically associated because they are all influenced by that construct. This provides the justification for using IRT or CFA to evaluate whether a set of indicators are all associated through estimating factor loadings for each indicator. We typically include in our final measurement models only indicators with significant loadings. This can be directly applied to IDA datasets when all studies in an IDA have used identical measures, as in the work of Dong et al. (2023), who combined data from eight randomized teacher training programs that employed the same measure of teacher observations of students’ classroom adaptation.
In many other IDA projects, evidence for empirical associations among many indicators is limited or absent, as different studies employ different measures of the same construct, or change to new measures for later follow-ups, and we have no data to estimate associations among many items across those measures. This was true for outcome constructs used in all the other IDA studies reported in this special issue (Berry et al., 2023; Connell et al., 2023; Hensums et al., 2023; Magee et al., 2023; Mun et al., 2023; Musci et al., 2023; Russell et al., 2023; Saavedra et al., 2023; Seidman et al., 2023; Tiberio et al., 2023; Zhao et al., 2023). In these papers and in the broader literature, we have found three methods employed for combining different measures of the same constructs.
Standard meta-analysis often ignores this issue by using summary statistics based on summed scores and standardizing across studies by converting those to effect sizes, tacitly assuming that different measures are equally accurate representations of the construct of interest and have identical error distributions. As several papers in the special issue point out, this can ignore important sources of measurement bias, and item-level data available with individual participant datasets allows for evaluation of and adjustment for such bias in overall effectiveness and precision. Meta-analyses can increase confidence in construct equivalence by combining only those subsets of trials having similar measures of specific constructs. As an example, Schweer-Collins et al. (2023) combined data from 29 trials of brief interventions for alcohol use, but conduct analyses separately for subsets of trials that measured outcomes such as binge drinking, quantity of alcohol use, or frequency of use. IDA datasets often have studies that employ more than one measure of a construct. In these cases, there is evidence of strength of association among measures that can contribute to harmonization using empirical evidence. For example, Connell et al. (2023) used data from trials of the Family Checkup program where participants completed both the CBCL and the CDI to harmonize indicators of suicidality. When all measures have at least some indirect empirical association with every other measure, standard IRT models can be used to estimate scores for a common factor across all measures (see Howe et al., 2019 for an example). This requires more time-intensive estimation algorithms such as numerical integration or Markov Chain Monte Carlo procedures (Muthén & Muthén, 1998–2017) and requires that measures can be ignorably missing across studies (Little & Rubin, 2002). None of the studies in the special issue directly employs this method of empirical harmonization, although we suspect that many of the IPD datasets used in these studies include sets of measures for which there is empirical evidence of direct or indirect association. The latter occurs when two measures are never administered together, but each is administered together with a third measure. Although measurement models are estimable with such data, it is unclear whether they are biased when applied to datasets with more indirect associations. The field would benefit from simulation studies that explore whether common patterns of more indirect associations can bias harmonization results.
Most of the IDA studies in the special issue employ a third method to establish associations among indicators of different measures that Cole et al. (2023) refer to as logical harmonization. We prefer the term semantic harmonization, for several reasons. Investigators compare the semantic content of each pair of indicators based on the judgments of the research team and develop rules for determining whether two indicators can be judged to have the same meaning, even when couched in different words. This assumes that items matched by the research team will be experienced by study participants as having identical or very similar meanings. Semantic harmonization is used to select only those items that have semantic matches with other items and to combine matched items into a single item. Final empirical harmonization then conducts IRT factor analyses with these semantically harmonized items.
In one of its simplest forms, semantic harmonization can involve assumptions that respondents use the same “count semantics” even when asked to provide counts of behaviors in different ways. For example, Mun et al. (2023) combined items that asked participants to describe drinking behavior with different wording and scales. One measure asked participants to provide an estimate of the total number of drinks they had across a typical week. A second measure used in other studies asked participants to estimate the average number of drinks they had for each day of the week. The research team added these daily responses together to get an estimate of weekly drinking rates. This assumes that participant recall provides an accurate count regardless of item wording. We understand the rationale, but suggest that it may be important to test this assumption when possible, given evidence that memory for prior events can be fickle and influenced by the passage of time, the current measurement context, or developmental age of the respondent (Marini et al., 2023).
Other studies describe more complex forms of semantic harmonization that require attention to both indicator content and scaling. To harmonize outcome measures, Saavedra et al. (2023) combined data on diagnosed anxiety disorders from studies that employed two different interview schedules assumed to provide the same categorization. Other studies used content review by the research team to match questionnaire item content across measures originally developed to measure the same constructs, including depression (Magee et al., 2023; Tiberio et al., 2023; Zhao et al., 2023), anxiety (Tiberio et al., 2023), bullying and victimization (Magee et al., 2023), and sexual activity (Vasilenko et al., 2023). Some studies used similar methods to harmonize constructs that might act as moderators of intervention impact, including elements of socio-economic status (Berry et al., 2023) and individual- or school-level demographics (Vasilenko et al., 2023).
Semantic harmonization also requires attention to the relative meaning of timing cues and scaling systems that can vary across measures. For example, Connell et al. (2023) combined items from three measures with different timing cues: the CBCL, which asks participants to rate whether a behavior or feeling occurred over the past 6 months; the CDI, which focuses on the past 2 weeks; and the BSI, which focuses on the last week. These measures also utilize different rating scales with different numbers of options and different anchor languages. The investigators chose to collapse all items to dichotomies, as did those in several other studies. Cole et al. (2023) provide a thoughtful discussion of the various choices that are commonly required in semantic harmonization, including whether to dichotomize or to map ordinal categories onto each other.
Semantic harmonization also assumes that item wordings will not be interpreted in different ways by different groups of participants, in different contexts, or at different times. We see a key challenge of semantic harmonization reflected in the question, “Whose semantics?” All studies in the special issue used research teams, including content experts, to make the determinations of semantic equivalence. At the least, this assumes that all study participants with reasonable facility in the language of the questionnaire or interview would experience these different items as having the same meaning. More than this, it assumes that item meanings as experienced by content experts are equivalent to content meanings as experienced by non-expert participants. These assumptions may be reasonable for the indicators some constructs (such as age) but not for others (such as experience of fatigue or sadness).
We suggest that the field would benefit from employing systematic methods for rating semantic equivalence and testing its assumptions. Rigorous methods for content analysis are available for using a team of raters to evaluate equivalence based on explicit criteria rather than expert intuition (Bakeman & Gottman, 1997; Krippendorff, 2019). This can require developing coding manuals, training staff naïve to the content area, and assessing inter-rater agreement. Rating systems can also ask raters to indicate how similar they perceive the meaning of two indicators to be. Cole et al. (2023) raise this issue and suggest that procedures for determining item equivalence also include such ratings, distinguishing items of questionable equivalence and testing whether including or excluding such items has any impact on results.
In most of the papers in the special issue, semantic harmonization methods collapse pairs of matched items into single indicator variables. This assumes that indicators matched on content would be perfectly correlated if we were able to administer both of them to a sample of participants. This assumption can be tested in IDA datasets by using moderated factor analysis to assess whether indicator loadings and thresholds vary depending on which measure the item was taken from. None of the papers in the special issue describe such analyses, although some tested whether these parameters varied across studies, and used measurement models that adjusted for such variability when found (Magee et al., 2023; Tiberio et al., 2023). This can help to account for such variability when different studies use different measures.
There may also be empirical or theoretical reasons to think that participant semantics differ from that of the research team. We suspect that differences in developmental age, cultural context, expert status, and observer perspective will be very relevant here. For example, Mlynarski (2018) found that the factor structure of a measure of acculturation differed substantially for youth and parents in Latinex immigrant families, suggesting that adults and youth were interpreting the items very differently. As another example, Seifer et al. (1994) found little concordance between ratings of infant behavior made immediately following a mother-infant interaction by mothers and trained raters using the same rating system. In these cases, it will be important to employ raters similar to those in the population of interest when determining semantic equivalence, rather than expert raters.
In some cases, semantic harmonization is used to match pairs of items that were both administered to participants in one or more studies. In this situation Zhao et al. (2023) decided to use an “OR” rule, creating a single variable that rated a symptom as present if it occurred in any of the matched items. We would suggest using available empirical data to evaluate item equivalence in these cases by studying item associations directly and using methods that incorporate estimates of measurement error when items being paired do not correlate very highly. Bridging studies that are not part of the IDA sample may also provide empirical evidence concerning equivalence. It can be worthwhile to search for existing studies that have employed both measures with a population similar to that involved in the studies being included in the IDA project. It can also be useful to conduct new bridging studies, although this is usually beyond the resources of IDA research teams.
Semantic harmonization can reduce the number of indicators available for measurement modeling. For example, Seidman et al. (this issue) identified 17 symptoms of youth depression, based on five measures that contained a number of items they were unable to match semantically. Bollen and Lennox (1991) note that, in theory, this should have little impact on measurement of a reflective construct, assuming that enough indicators with strong loadings remain. In practice, this will depend on the number and quality of indicators available for matching. Reducing the number of indicators can increase unsystematic measurement error and also increase risk of systematic measurement error or measure contamination. We suggest comparing scores based on semantic harmonization of indicators from each measure with scores based on a measurement model including all indicator scores from that measure, as a means of checking the impact of semantic harmonization.
All studies in the special issue used factor analyses with categorical indicators (IRT models) to model variation in construct states, consistent with the reflective model of measurement. Summary scale scores created by adding item scores together assume tau-equivalence, where the construct has equally strong impact on all of its indicators (Brown, 2015). It is common for the impact of construct states to vary across indicators, leading to different factor loadings for different indicators within the same measure. This violates the tau-equivalent assumption and biases summary scale scores. Factor analytic methods used in these projects that allow factor loadings to vary across items eliminate such bias, leading to more accurate estimates of construct states. Factor analyses using IDA datasets may also be less prone to biases driven by range restrictions when data on indicators are combined across multiple studies with somewhat different populations that together cover a greater range of construct states.
With one exception, studies appeared to follow standard practice of dropping indicators with non-significant factor loadings that are inconsistent with the reflective measurement model. Connell et al. (2023) found that parent and youth reports of youth suicidality loaded on a single factor, but teacher reports did not, yet decided to keep teacher reports in the model to enhance data coverage across samples. A more convincing rationale would require defining the suicidality construct as context-specific, such that reports in different contexts reflect accurate estimates of construct state as it manifests differently (or becomes more easily observable) within each context. This is particularly salient in this example as teachers and educational systems are generally unaware of students’ suicide risk (Brown et al., 2006). This rationale has been applied to modeling of youth externalizing behavior, given that reports by different observers in different contexts (children, parents, teachers) are only very weakly correlated (De Los Reyes et al., 2023).
Causal Invariance
The reflective measurement model also assumes causal invariance: the impact of construct state on observable indicators is constant across all participants, times, and contexts. In the IRT tradition, one way causal invariance is violated is when there is evidence of differential item functioning across one or more variables within these domains. Violations of causal invariance may reflect two different mechanisms. The construct may have different effects on an indicator for different people, across different contexts, or at different times. For example, depression may have a stronger impact on suicide attempts in some groups.
Causal invariance will also be violated if observations of an indicator are less accurate for some participants, places, or times. This may be of particular relevance for semantic harmonization strategies, where semantic similarity of items may vary by population, context, or over development. Such violations can be tested during semantic harmonization through employing raters who vary on relevant characteristics such as developmental age.
The seminal work of Curran, Bauer, Hussong, and their colleagues has led to the development and refinement of moderated nonlinear factor analysis (MNLFA) as a major extension of factor analytic methods employed in retrospective psychometrics to evaluate and adjust for such violations (Bauer, 2016; Bauer & Hussong, 2009; Curran et al., 2014). Gottfredson et al. (2019) released an automated system that facilitates the use of this method, and most of the papers in the special issue employed MLNFA. This acronym has come to reference both the quantitative modeling employed and the more complex set of strategies that integrate semantic and empirical harmonization methods. Zhao et al. (2023) provided a thoughtful tutorial on how to use this strategy, and Cole et al. (2023) described a number of strategic decisions it requires and the potential impact of various branching pathways through these decision points. Musci et al. (2023) developed an extension of MLNFA by integrating it with growth mixture modeling to identify different patterns of change in psychotic-like experiences reported by youth over extended periods following participation in prevention programs.
Most of the IDA projects reported in the special issue employed MNLFA strategies and models to produce factor scores for IDA analyses. They selected two sets of potential moderators: design variables including trial or study, intervention condition, or time since baseline and general demographic factors including age, sex, and ethnicity. Some projects evaluated more complex patterns by including interactions among potential moderators (Connell et al., 2023; Tiberio et al., 2023).
Although not always explicated, the rationale for studying design variables as potential moderators seems well justified. It is conceivable that Intervention conditions may alter how participants interpret questionnaire items, leading to measurement differences across experimental and control groups. Examining whether the underlying measurement model is the same by intervention condition provides a useful test of this issue. Studies may vary in which measures are used and how they are administered, and sample from different populations or contexts. Measurement models may shift across multiple administrations as participants have more exposure to the same measures, and measurement models may evolve over developmental time. Demographic factors such as sexual or gender minority status (Mustanski et al., 2021) can be proxies for variability across a wide range of historical exposures or cultural contexts.
Beyond this, our science provides little guidance as yet in identifying plausible sources of differential item functioning that need attention during harmonization. Most of the papers in this special issue highlight the importance of IDA for identifying sources of heterogeneity in intervention effects, and as we discuss later, this work can benefit from more attention to theoretically derived factors that lead to such heterogeneity. As a common sense rule, we would suggest that any potential moderator of intervention effect be included as a potential moderator in analyses of DIF used in measure harmonization.
There is some disagreement among the papers on whether DIF analyses can provide substantive information about measurement (Magee et al., this issue) or whether findings of DIF should be treated as nuisance effects to be adjusted for but not interpreted (Cole et al., this issue). Most of the papers implicitly follow the latter position, using MNLFA to identify plausible DIF and adjusting factor scores using models that take DIF into account. Those papers that interpret DIF substantively focus mostly on developmental explanations. For example, Magee et al. (2023) found that age at assessment moderated both thresholds and loadings for depression items, suggesting that symptoms such as anhedonia and appetite disturbance were more strongly influenced by depression as children grew older.
We take a middle ground here concerning whether DIF should be treated substantively or as a nuisance factor. The preponderance of significant findings across these studies involved evidence for systematic variation in item thresholds, as compared to item loadings. Loadings reflect the overall sensitivity of an indicator to variation in construct state, while thresholds provide information about the relative range of sensitivity across the levels of the construct state. Given the same loading, lower thresholds reflect sensitivity to lower levels of the construct but often less sensitivity to variation at the higher end, while higher thresholds reflect the opposite. We suggest that loading DIF can reflect more substantive differences, as it can reflect both variations due to reporting differences and those due to different causal impact of the construct on an indicator. For example, separation anxiety may become less common as children grow older, and so differences in its loading on a measure of broader anxiety disorders may be due to substantive developmental differences. This raises an important substantive question as to whether the construct of broad anxiety disorder means the same thing at different points in development. As another example, Patterson (1993) used the metaphor of a chimera (a mythological beast that begins with a simpler body and then evolves by growing the head of a lion and the tail of a snake) as a description of how longitudinal data indicate that conduct disorder evolves from early oppositional behavior to later deviancy, requiring different measurement models at different stages of development. Here, developmental change does not reflect increases or decreases in the same construct, but rather a dynamic reorganization of that construct over development. Forgatch et al. (2016) found that parenting interventions altered this developmental progression.
On the other hand, threshold DIF in the absence of loading DIF may be driven more by variations in reporting. Here, the effects of the construct on the item are the same, but item variation is associated with a different range of construct states. This can occur if unsystematic measurement error is stronger for some populations, contexts, or times of measurement. If we think of unsystematic error as nuisance variance, then it makes sense to model variations in threshold DIF and adjust factor scores accordingly. Threshold DIF can bias estimates of group means, supporting the strategy employed here of adjusting for it within the measurement model when studying mean differences, as is always the case in trials research.
Nonconfounding
Measures can be contaminated if item responses are shaped in part by systematic factors such as participants’ need to manage impressions or their general evaluative stance (seeing things in terms of good/bad distinctions). When more than one item is systematically contaminated, this will violate the conditional independence assumption. When item values are combined through simple summation, this violates the congeneric assumption of measurement (that items reflect only one construct). Scores based on factor models are less prone to contamination compared to simple summary scores as they partition common and unique indicator variance, as long as the effects of contaminating factors are independent of the construct of interest. However, this partitioning fails to account for contamination when all items in a measure are shaped by a contaminant, as the common variance due to the construct cannot be disentangled from that due to the contaminant, absent other information. This can lead to confounding if causes of the construct, including intervention condition, impact the contaminant rather than the construct. This is an issue in psychotherapy research, where alliance with a therapist can lead participants to report more positive outcomes.
Harmonization methods employed by the projects in the special issue have not yet attended to the possibility of contamination and potential confounding of this sort. It can be addressed in two ways, either through including and controlling for measures of possible contaminants such as inclination to respond in socially desirable ways or through bifactor modeling that includes secondary factors for each measure (Howe et al., 2019). The latter method may be more applicable to retrospective psychometric analyses; in our experience, few studies include measurement of potential contaminants and different studies often focus on different factors. In principle, such bifactor models can be applied to semantically harmonized data, although we are not aware of examples in the literature.
Nomological Validity
Nomological validity refers to accumulating evidence that constructs observed with a measure are associated as predicted with their causes and outcomes. Many of the measures employed in these IDA projects have a substantial history of application and a sizeable body of empirical evidence supporting their nomological validity. This evidence increases our confidence in accurate measurement when an IDA project includes studies that all use the same measure, as in the case of Dong et al. (2023), who provide a detailed summary of that evidence for the Teacher Observation of Classroom Adaptation measure. Other studies used measures that were administered in different ways or with different subsets of items in different studies (Hensums et al., 2023) or selected subsets of items from a set of different measures based on semantic similarity (Connell et al., 2023; Seidman et al., 2023; Tiberio et al., 2023).
We suggest that prior evidence for nomological validity becomes more ambiguous as the number of indicators from an established measure decreases. As a simple check, IDA investigators can evaluate whether harmonization might challenge the application of prior evidence of nomological validity by comparing factor scores after harmonization to scores based on each full measure used in their construction. Close concordance would increase confidence in nomological validity based on prior use; weak concordance would challenge it.
Effect Heterogeneity
Many of the papers in the special issue emphasize the utility of IDA for studying variation in intervention impact, also known as effect heterogeneity, given substantially greater power to detect moderation compared to meta-regression (Dagne et al., 2016). Six of the 18 papers used IDA to evaluate effect heterogeneity in intervention trials (Connell et al., 2023; Dong et al., 2023; Hensums et al., 2023; Russell et al., 2023; Tiberio et al., 2023; Vasilenko et al., 2023) and one evaluated whether several aspects of socioeconomic status moderated variation in parent engagement with interventions (Berry et al., 2023). Schweer-Collins et al. (2023) used a two-step procedure to evaluate heterogeneity, estimating interaction effects using individual participant data within each trial, but combining those effect estimates across trials with standard meta-analytic methods.
With one exception, none of the studies found variation in impact across broader sociodemographic variables including age at accrual, sex, race, ethnicity, or economic or social disadvantage. These included trials testing the impact of the Family Checkup program on suicidality (Connell et al., 2023), teacher-based interventions on racial disparities in classroom adaptation (Dong et al., 2023), school-based interventions to reduce bullying (Hensums et al., 2023), crossover impact on depression of youth and parent-focused interventions for youth in foster care (Tiberio et al., 2023), and the effects of teen pregnancy prevention programs on sexual activity (Vasilenko et al., 2023). IDA moderation analyses of effects of the Incredible Years program implemented in European countries on conduct disorder (Berry et al., 2023) have been reported elsewhere, and these also failed to find evidence of moderation by ethnicity and economic or social disadvantage (Gardner et al., 2019). As the exception, Schweer-Collins et al. (2023) found that brief interventions for alcohol use had slightly stronger effects on alcohol use outcomes for women and for one outcome variable on those with less than a high school education, although all effect sizes were modest and no effects were found in any follow-up lasting more than three months.
Two of these projects tested for and found moderation of impact by baseline levels of outcomes. Hensums et al. (2023) found that anti-bullying interventions were more effective for those youth who reported higher levels of victimization at baseline. Russell et al. (2023) found that peer network counseling programs reduced cannabis use at one to 3 months follow-up for those who reported higher levels of cannabis use at baseline and reduced use of other drugs at post-test for those who reported higher levels of those drugs at baseline.
Only one project tested moderation by presence of more specific risk or protective factors measured at baseline. Vasilenko et al. (2023) used multilevel latent class analysis to identify four risk profiles at both the individual and school level based on baseline indicators of primary language, nativity, grades, alcohol use, family structure, and whether a student was in a relationship with someone older or younger than them. They found no moderation effects for individual-level profiles, but did find that pregnancy prevention programs were less effective in reducing sexual behavior in schools with students who were mostly English-only speakers, born in the USA, and more likely to be in single parent households and use alcohol, compared to schools with more second-generation immigrants who were less likely to use alcohol and more likely to reside in two parent households.
What to make of these findings? We suggest that while it will always be important to assess whether the impact of prevention programs may vary across broad demographic characteristics or general types of risk or protective factors, it will be necessary to pay closer attention to early indicators of specific risk for physical and mental health challenges and to the specific proximal targets that interventions are designed to shape. Almost all prevention trials assess baseline levels of the long-term outcomes they hope to prevent, and so tests of baseline outcome moderation should be standard. Most prevention trials also assess baseline levels of at least some of the more specific targets they hope to change, based on the etiologic theories they use to build their interventions. Investigators are increasingly reporting tests of baseline target moderation, or more complete tests of baseline target moderated mediation, as reflected in a recent Prevention Science special section on this topic (Howe & Leijten, 2022). In our experience, harmonization of baseline target measures is currently quite challenging, given that prevention science does not have strong norms for using more standard measures of these targets, and different trials investigators will often select or even create new measures for this purpose. We suggest that the field would greatly benefit from efforts to identify or create and validate measures of common targets and to urge investigators to employ them in tests of moderation and mediation, perhaps through some sort of Consort system (Gardner et al., 2013). More consistent attention to and measurement of moderators will also provide greater opportunities for evaluating how well-combined datasets meet assumptions for causal inference of heterogeneous impacts, as Barker et al. (2023) discuss.
Conclusions
Taken as a whole, the papers of this special issue indicate that our field has a strong and growing interest in combining and harmonizing individual participant data from multiple studies and using recent advances in IDA quantitative methods to more rigorously evaluate both theories of etiology and the effects of prevention programs built on them. They demonstrate substantial progress along a number of fronts, including the complex business of harmonizing measures to establish, evaluate, and adjust models to achieve construct equivalence across studies. In this commentary, we have provided a number of suggestions for how this work might be improved, but want to acknowledge and honor the work of the special issue editors and the authors in moving this field forward, confronting its complexities, and exploring novel and often groundbreaking new methods for achieving its goals.
References
Bakeman, R., & Gottman, J. M. (1997). Observing interaction: An introduction to sequential analysis, 2nd ed. Cambridge University Press. https://doi.org/10.1017/CBO9780511527685
Barker, D. H., Bie, R., & Steingrimsson, J. A. (2023). Addressing systematic missing data in the context of causally interpretable meta-analysis. Prevention Science : THe Official Journal of the Society for Prevention Research. https://doi.org/10.1007/s11121-023-01586-2
Bauer, D. J. (2016). A more general model for testing measurement invariance and differential item functioning. Psychological Methods. https://doi.org/10.1037/met0000077
Bauer, D. J., & Hussong, A. M. (2009). Psychometric approaches for developing commensurate measures across independent studies: Traditional and new models. Psychological Methods, 14(2), 101–125. https://doi.org/10.1037/a0015583(Multi-StudyMethodsforBuildingaCumulativePsychologicalScience)
Berry, V., Melendez-Torres, G. J., Axford, N., Axberg, U., de Castro, B. O., Gardner, F., Gaspar, M. F., Handegård, B. H., Hutchings, J., Menting, A., McGilloway, S., Scott, S., & Leijten, P. (2023). Does social and economic disadvantage predict lower engagement with parenting interventions? An integrative analysis using individual participant data. Prevention Science. https://doi.org/10.1007/s11121-022-01404-1
Bollen, K. A., & Lennox, R. (1991). Conventional wisdom on measurement: A structural equation perspective. Psychological Bulletin, 110(2), 305–314. https://doi.org/10.1037//0033-2909.110.2.305
Borsboom, D. (2008). Latent variable theory [Article]. Measurement, 6(1/2), 25–53. https://doi.org/10.1080/15366360802035497
Borsboom, D., Mellenbergh, G. J., Heerden, J., & v. (2003). The theoretical status of latent variables [Article]. Psychological Review, 110(2), 203–219. https://doi.org/10.1037/0033-295X.110.2.203
Brincks, A., Montag, S., Howe, G. W., Shi, H., Siddique, J., Soyeon, A., Sandler, I. N., Pantin, H., Hendricks Brown, C., Huang, S., Ahn, S., & Brown, C. H. (2018). Addressing methodologic challenges and minimizing threats to validity in synthesizing findings from individual-level data across longitudinal randomized trials [journal article]. Prevention Science, 19, S60–S73. https://doi.org/10.1007/s11121-017-0769-1
Brown, C. H., Brincks, A., Shi, H., Perrino, T., Cruden, G., Pantin, H., Howe, G., Young, J. F., Beardslee, W., Montag, S., Sandler, I., Brown, C. H., & Huang, S. (2018). Two-year impact of prevention programs on adolescent depression: An integrative data analysis approach [Article]. Prevention Science, 19, S74–S94. https://doi.org/10.1007/s11121-016-0737-1
Brown, C. H., Hedeker, D., Gibbons, R. D., Duan, N., Almirall, D., Gallo, C., Burnett-Zeigler, I., Prado, G., Young, S. D., Valido, A., & Wyman, P. A. (2022). Accounting for context in randomized trials after assignment. Prevention Science. https://doi.org/10.1007/s11121-022-01426-9
Brown, C. H., Sloboda, Z., Faggiano, F., Teasdale, B., Keller, F., Burkhart, G., Vigna-Taglianti, F., Howe, G., Masyn, K., Wang, W., Muthén, B., Stephens, P., Grey, S., & Perrino, T. (2013). Methods for synthesizing findings on moderation effects across multiple randomized trials. Prevention Science, 14(2), 144–156. https://doi.org/10.1007/s11121-011-0207-8
Brown, C. H., Wyman, P. A., Brinales, J. M., & Gibbons, R. D. (2007). The role of randomized trials in testing interventions for the prevention of youth suicide. International Review of Psychiatry, 19(6), 617–631. https://doi.org/10.1080/09540260701797779
Brown, C. H., Wyman, P. A., Guo, J., & Peña, J. (2006). Dynamic wait-listed designs for randomized trials: New designs for prevention of youth suicide. Clinical Trials (london, England), 3(3), 259–271. https://doi.org/10.1191/1740774506cn152oa
Brown, T. A. (2015). Confirmatory factor analysis for applied research (2nd ed.). Guilford.
Cole, V. T., Hussong, A. M., Gottfredson, N. C., Bauer, D. J., & Curran, P. J. (2023). Informing harmonization decisions in integrative data analysis: Exploring the measurement multiverse. Prevention Science. https://doi.org/10.1007/s11121-022-01466-1
Connell, A. M., Seidman, S., Ha, T., Stormshak, E., Westling, E., Wilson, M., & Shaw, D. (2023). Long-term effects of the family check-up on suicidality in childhood and adolescence: Integrative data analysis of three randomized trials. Prevention Science. https://doi.org/10.1007/s11121-022-01370-8
Curran, P. J. (2009). The seemingly quixotic pursuit of a cumulative psychological science: Introduction to the special issue. Psychological Methods, 14(2), 77–80. https://doi.org/10.1037/a0015972(Multi-StudyMethodsforBuildingaCumulativePsychologicalScience)
Curran, P. J., & Hussong, A. M. (2009). Integrative data analysis: The simultaneous analysis of multiple data sets. Psychological Methods, 14(2), 81–100. https://doi.org/10.1037/a0015914
Curran, P. J., McGinley, J. S., Bauer, D. J., Hussong, A. M., Burns, A., Chassin, L., Sher, K., & Zucker, R. (2014). A moderated nonlinear factor model for the development of commensurate measures in integrative data analysis. Multivariate Behavioral Research, 49(3), 214–231. https://doi.org/10.1080/00273171.2014.889594
Dagne, G. A., Brown, C. H., Howe, G., Kellam, S. G., & Liu, L. (2016). Testing moderation in network meta-analysis with individual participant data. Statistics in Medicine, 35(15), 2485–2502. https://doi.org/10.1002/sim.6883
De Los Reyes, A., Wang, M., Lerner, M. D., Makol, B. A., Fitzpatrick, O. M., & Weisz, J. R. (2023). The operations triad model and youth mental health assessments: Catalyzing a paradigm shift in measurement validation [Article]. Journal of Clinical Child & Adolescent Psychology, 52(1), 19–54. https://doi.org/10.1080/15374416.2022.2111684
Dong, N., Herman, K. C., Reinke, W. M., Wilson, S. J., & Bradshaw, C. P. (2023). Gender, racial, and socioeconomic disparities on social and behavioral skills for k-8 students with and without interventions: An integrative data analysis of eight cluster randomized trials. Prevention Science. https://doi.org/10.1007/s11121-022-01425-w
Forgatch, M. S., Snyder, J. J., Patterson, G. R., Pauldine, M. R., Chaw, Y., Elish, K., Harris, J. B., & Richardson, E. B. (2016). Resurrecting the chimera: Progressions in parenting and peer processes. Development and Psychopathology, 28(3), 689–706. https://doi.org/10.1017/S0954579416000250
Gardner, F., Leijten, P., Harris, V., Mann, J., Hutchings, J., Beecham, J., Bonin, E.-M., Berry, V., McGilloway, S., Gaspar, M., João Seabra-Santos, M., Orobio de Castro, B., Menting, A., Williams, M., Axberg, U., Morch, W.-T., Scott, S., & Landau, S. (2019). Equity effects of parenting interventions for child conduct problems: A pan-European individual participant data meta-analysis. The Lancet Psychiatry, 6(6), 518–527. https://doi.org/10.1016/S2215-0366(19)30162-2
Gardner, F., Mayo-Wilson, E., Montgomery, P., Hopewell, S., Macdonald, G., Moher, D., & Grant, S. (2013). Editorial perspective: The need for new guidelines to improve the reporting of trials in child and adolescent mental health. Journal of Child Psychology and Psychiatry, 54(7), 810–812. https://doi.org/10.1111/jcpp.12106
Gottfredson, N. C., Cole, V. T., Giordano, M. L., Bauer, D. J., Hussong, A. M., & Ennett, S. T. (2019). Simplifying the implementation of modern scale scoring methods with an automated R package: Automated moderated nonlinear factor analysis (aMNLFA). Addictive Behaviors, 94, 65–73. https://doi.org/10.1016/j.addbeh.2018.10.031
Hensums, M., de Mooij, B., Kuijper, S. C., Fekkes, M., Overbeek, G., Cross, D., DeSmet, A., Garandeau, C. F., Joronen, K., Leadbeater, B., Menesini, E., Palladino, B. E., Salmivalli, C., Solomontos-Kountouri, O., & Veenstra, R. (2023). What works for whom in school-based anti-bullying interventions? An individual participant data meta-analysis. Prevention Science. https://doi.org/10.1007/s11121-022-01387-z
Howe, G., & Leijten, P. (2022). When is it time to revise or adapt our prevention programs? Introduction to special issue on using baseline target moderation to assess variation in prevention impact. Prevention Science. https://doi.org/10.1007/s11121-022-01456-3
Howe, G. W., Dagne, G. A., Brown, C. H., Brincks, A. M., Beardslee, W., Perrino, T., & Pantin, H. (2019). Evaluating construct equivalence of youth depression measures across multiple measures and multiple studies [Article]. Psychological Assessment, 31(9), 1154–1167. https://doi.org/10.1037/pas0000737
Howe, G. W., Pantin, H., & Perrino, T. (2018). Programs for preventing depression in adolescence: Who benefits and who does not? An introduction to the supplemental Issue [editorial]. Prevention Science, 19, S1–S5. https://doi.org/10.1007/s11121-018-0870-0
Krippendorff, K. (2019). Content analysis : an introduction to its methodology (4th edition. ed.). SAGE Publications, Inc.
Little, R. J. A., & Rubin, D. B. (2002). Statistical analysis with missing data (2nd ed.). John Wiley & Sons.
Magee, K. E., Connell, A., Hipwell, A. E., Shaw, D., Westling, E., Keenan, K., Stormshak, E., Ha, T., & Stepp, S. (2023). Developmental models of depression, externalizing problems, and self-regulatory processes: Integrated data analysis across four longitudinal studies of youth. Prevention Science. https://doi.org/10.1007/s11121-022-01441-w
Marini, C., Northover, N. S., Gold, N. D., Rogers, U. K., O'Donnell, K. C., Tofighi, B., Ross, S., & Bogenschutz, M. P. (2023). A systematic approach to standardizing drinking outcomes from timeline followback data. Substance Abuse: Research and Treatment, 17. https://doi.org/10.1177/11782218231157558
McDaniel, H. L., Saavedra, L. M., Morgan-López, A. A., Bradshaw, C. P., Lochman, J. E., Kaihoi, C. A., Powell, N. P., Qu, L., & Yaros, A. C. (2023). Harmonizing social, emotional, and behavioral constructs in prevention science: Digging into the weeds of aligning disparate measures. Prevention Science. https://doi.org/10.1007/s11121-022-01467-0
Mlynarski, L. K. (2018). Do acculturation gaps between Latino parents and adolescents influence family connection and depression? ProQuest Information & Learning]. Dissertation Abstracts International: Section B: The Sciences and Engineering.
Mun, E.-Y., Zhou, Z., Huh, D., Tan, L., Li, D., Tanner-Smith, E. E., Walters, S. T., & Larimer, M. E. (2023). Brief alcohol interventions are effective through 6 months: Findings from marginalized zero-inflated Poisson and negative binomial models in a two-step IPD meta-analysis. Prevention Science. https://doi.org/10.1007/s11121-022-01420-1
Musci, R. J., Kush, J. M., Masyn, K. E., Esmaeili, M. A., Susukida, R., Goulter, N., McMahon, R., Eddy, J. M., Ialongo, N. S., Tolan, P., Godwin, J., Wilcox, H. C., Bierman, K. L., Coie, J. D., Crowley, D. M., Dodge, K. A., Greenberg, M. T., Lochman, J. E., McMahon, R. J., & Pinderhughes, E. E. (2023). Psychosis symptom trajectories across childhood and adolescence in three longitudinal studies: An integrative data analysis with mixture modeling. Prevention Science. https://doi.org/10.1007/s11121-023-01581-7
Mustanski, B., Whitton, S. W., Newcomb, M. E., Clifford, A., Ryan, D. T., & Gibbons, R. D. (2021). Predicting suicidality using a computer adaptive test: Two longitudinal studies of sexual and gender minority youth. Journal of Consulting and Clinical Psychology, 89(3), 166–175. https://doi.org/10.1037/ccp0000531
Muthén, L. K., & Muthén, B. O. (1998–2017). Mplus user’s guide. Eighth Edition. (6th ed.). Muthén & Muthén: Los Angeles, CA.
Patterson, G. R. (1993). Orderly change in a stable world: The antisocial trait as a chimera. Journal of Consulting and Clinical Psychology, 61(6), 911–919. https://doi.org/10.1037/0022-006X.61.6.911(TheAnalysisofChange)
Potter, L. N., Yap, J., Dempsey, W., Wetter, D. W., & Nahum-Shani, I. (2023). Integrating intensive longitudinal data (ILD) to inform the development of dynamic theories of behavior change and intervention design: A case study of scientific and practical considerations. Prevention Science. https://doi.org/10.1007/s11121-023-01495-4
Russell, M. A., Coatsworth, J. D., Brown, A., Zaharakis, N., Mennis, J., Rodriguez, G. C., & Mason, M. J. (2023). Peer network counseling effects on substance use: An individual participant data meta-analysis integrating three randomized controlled trials. Prevention Science. https://doi.org/10.1007/s11121-022-01468-z
Saavedra, L. M., Morgan-López, A. A., West, S. G., Alegría, M., & Silverman, W. K. (2023). Mitigating multiple sources of bias in a quasi-experimental integrative data analysis: Does treating childhood anxiety prevent substance use disorders in late adolescence/young adulthood? Prevention Science. https://doi.org/10.1007/s11121-022-01422-z
Schweer-Collins, M. L., Parr, N. J., Saitz, R., & Tanner-Smith, E. E. (2023). Investigating for whom brief substance use interventions are most effective: An individual participant data meta-analysis. Prevention Science. https://doi.org/10.1007/s11121-023-01525-1
Seidman, S., Connell, A., Stormshak, E., Westling, E., Ha, T., & Shaw, D. (2023). Disrupting maternal transmission of depression: Using integrative data analysis (IDA) to examine indirect effects of the family check-up (FCU) across three randomized trials. Prevention Science. https://doi.org/10.1007/s11121-022-01471-4
Seifer, R., Sameroff, A. J., Barrett, L. C., & Krafchuk, E. (1994). Infant temperament measured by multiple observations and mother report. Child Development, 65(5), 1478–1490.
Siddique, J., de Chavez, P. J., Howe, G., Cruden, G., Hendricks Brown, C., & Brown, C. H. (2018). Limitations in using multiple imputation to harmonize individual participant data for meta-analysis [journal article]. Prevention Science, 19, S95–S108. https://doi.org/10.1007/s11121-017-0760-x
Stewart, L. A., & Parmar, M. K. (1993). Meta-analysis of the literature or of individual patient data: Is there a difference? Lancet (london, England), 341(8842), 418–422.
Tiberio, S. S., Pears, K. C., Buchanan, R., Chamberlain, P., Leve, L. D., Price, J. M., & Hussong, A. M. (2023). An integrative data analysis of main and moderated crossover effects of parent-mediated interventions on depression and anxiety symptoms in youth in foster care. Prevention Science. https://doi.org/10.1007/s11121-023-01524-2
van Bork, R., Rhemtulla, M., Sijtsma, K., & Borsboom, D. (2022). A causal theory of error scores. Psychological Methods. https://doi.org/10.1037/met0000521
Vasilenko, S. A., Odejimi, O. A., Glassman, J. R., Potter, S. C., Drake, P. M., Coyle, K. K., Markham, C., Emery, S. T., Peskin, M. F., Shegog, R., Addy, R. C., & Clark, L. F. (2023). Who benefits from school-based teen pregnancy prevention programs? Examining multidimensional moderators of program effectiveness across four studies. Prevention Science. https://doi.org/10.1007/s11121-022-01423-y
WHO. (1991). Impact of glycine-containing ORS solutions on stool output and duration of diarrhoea: A meta-analysis of seven clinical trials. The International Study Group on Improved ORS. Bulletin of the World Health Organization, 69(5), 541–548.
Zhao, X., Coxe, S., Sibley, M. H., Zulauf-McCurdy, C., & Pettit, J. W. (2023). Harmonizing depression measures across studies: A tutorial for data harmonization. Prevention Science. https://doi.org/10.1007/s11121-022-01381-5
Funding
This study is funded by NIH/NIMH (1R01MH117598, Brown, PI; Howe, Co-I); NIDA and the NIH Office of Disease Prevention (P30DA027828, P30DA027828-09S3, P30DA027828-10S1, Brown PI), NIH/NIMH (R01MH117598, Brown PI), and NIH/NIMH (R01MH124718 Prado and Brown MPI).
Author information
Authors and Affiliations
Corresponding author
Ethics declarations
Ethics Approval
Not applicable.
Consent to Participate
Not applicable.
Conflict of Interest
The authors declare no competing interests.
Additional information
Publisher's Note
Springer Nature remains neutral with regard to jurisdictional claims in published maps and institutional affiliations.
Rights and permissions
Springer Nature or its licensor (e.g. a society or other partner) holds exclusive rights to this article under a publishing agreement with the author(s) or other rightsholder(s); author self-archiving of the accepted manuscript version of this article is solely governed by the terms of such publishing agreement and applicable law.
About this article
Cite this article
Howe, G.W., Brown, C.H. Retrospective Psychometrics and Effect Heterogeneity in Integrated Data Analysis: Commentary on the Special Issue. Prev Sci 24, 1672–1681 (2023). https://doi.org/10.1007/s11121-023-01592-4
Accepted:
Published:
Issue Date:
DOI: https://doi.org/10.1007/s11121-023-01592-4