Abstract
Incorporating cultural sensitivity into healthcare settings is important to deliver high-quality and equitable care, particularly for marginalized communities who are non-White, non-English speaking, or immigrants. The Clinicians’ Cultural Sensitivity Survey (CCSS) was developed as a patient‐reported survey assessing clinicians’ recognition of cultural factors affecting care quality for older Latino patients; however, this instrument has not been adapted for use in pediatric primary care. Our objective was to examine the validity and reliability of a modified CCSS that was adapted for use with parents of pediatric patients. A convenience sampling approach was used to identify eligible parents during well-child visits at an urban pediatric primary care clinic. Parents were administered the CCSS via electronic tablet in a private location. We first conducted exploratory factor analyses (EFAs) to explore the dimensionality of survey responses in the adapted CCSS, and then conducted a series of confirmatory factor analyses (CFAs) using maximum likelihood estimation based on the results of the EFAs. Exploratory and confirmatory factor analyses (N = 212 parent surveys) supported a three-factor structure assessing racial discrimination (\(\alpha\)=0.96), culturally-affirming practices (\(\alpha\)=0.86), and causal attribution of health problems (\(\alpha\)=0.85). In CFAs, the three-factor model also outperformed other potential factor structures in terms of fit statistics including scaled root mean square error approximation (0.098), Tucker-Lewis Index (0.936), Comparative Fit Index (0.950), and demonstrated adequate fit according to the standardized root mean square residual (0.061). Our findings support the internal consistency, reliability, and construct validity of the adapted CCSS for use in a pediatric population.
Similar content being viewed by others
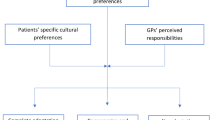
Avoid common mistakes on your manuscript.
Background
Dismantling disparities in pediatric primary care, especially around care access, quality, and delivery is a priority of the National Academy of Medicine and American Academy of Pediatrics [1, 2]. Families of color (those identifying as Black, Latino/a/e, Indigenous, Asian, Pacific Islander, or multi-racial), those living in poverty, and those identifying as immigrants and/or non-English speaking are less likely to receive high-quality healthcare and are less likely to have a medical home [3,4,5]. Eliminating these disparities, which are rooted in intersecting racism, poverty, xenophobia, and language injustice, is critical to promote child health equity and thriving [6].
One important way to promote health equity in pediatric primary care is the provision of culturally sensitive care (CSC). Past research from the adult literature has shown that CSC is an essential part of building trust and rapport in patient-provider communication during medical encounters and has been linked to improvements in patient-reported quality of care, such as improved satisfaction with providers who had received cultural competency training and improved patient-provider communication [7, 8]. However, additional work has indicated that clinicians often lack training in providing CSC to patients [9, 10], which may lead to poor health care quality [11,12,13]. Patients who perceive discrimination or stigma from a healthcare provider may be less likely to adhere to treatment recommendations [14,15,16,17,18].
Less work has focused on the provision of CSC in pediatric settings. The pediatric setting is unique as providers are engaging with patients, parents, and other caregivers. Pediatric visits, particularly annual physical exams or well-child visits, also have different goals than adult settings, as well-child visits are tailored to the age and developmental stage of the patient. Ragavan et al. conducted one of the first studies examining culturally sensitive care within pediatric primary care, finding positive associations between parent-reported receipt of CSC and well-child care quality [19]. Further, a recent systematic review of culturally sensitive interventions in pediatric primary care found preliminary evidence of intervention feasibility, acceptability, and efficacy [6]. Other analyses considering the validity of cross-cultural instruments have focused on specific patient health needs such as food allergies, obsessive–compulsive disorder, and pain but not the concept of cultural sensitivity broadly and were not focused on parents [20,21,22].
To understand how CSC is being translated into pediatric settings, tailored measures are needed. A few measures are available for adults, including the Clinician’s Cultural Sensitivity Survey (CCSS-29). The CCSS-29 is a 29-item patient-reported outcome measure developed by Nápoles and colleagues that assesses cultural sensitivity across 14 domains, including: complementary and alternative medicine (2 items), mind–body connections (2 items), causal attribution of health problems (2 items), preventive care (2 items), family involvement (2 items), modesty (2 items), use of prescription medications (1 item), spirituality (1 item), doctors’ discrimination due to education (2 items), doctors’ discrimination due to race/ethnicity (2 items), staff discrimination due to race/ethnicity (2 items), sensitivity to language needs (3 items), discrimination due to language needs (3 items), and sensitivity to immigrant status (3 items) [23]. The CCSS-29 administers the first 11 domains (20 items) to all patients. Two domains (sensitivity and discrimination due to language needs) are targeted towards non-English speaking patients, and sensitivity to immigrant status is only asked of patients born abroad. The CCSS-29 has been used and validated in Latino/a/e- identifying adults [24]. However, neither the CCSS-29, or, to our knowledge, other CSC measures have been developed specifically for pediatric primary care settings. It is imperative to adapt and psychometrically validate CSC measures for use in pediatric settings so practices have an evidenced-based method to assess the provision of CSC delivered to patients and their families.
In this study, we examined the structural validity of a modified version of the CCSS-29. Since the original survey was designed for self-administration by adult patients who identify as Latino/a/e, it is important to examine the construct validity of the modified instrument which was administered to parents of young children from different racial and ethnic backgrounds. We addressed these gaps by conducting exploratory and confirmatory factor analyses of a modified version of the CCSS-29, the CCSS-Pediatric Primary Care (CCSS-PPC), based on cross-sectional data collected from a sample of parents and caregivers of patients 3–48 months at a large urban safety-net hospital [19].
Methods
Modification of the CCSS-29 for Use in Pediatric Primary Care
To modify the CCSS-29 for a pediatric primary care setting, three pediatricians assessed the relevance of each item and subscale using Brotanek et al.’s (2008) conceptual framework for cultural competency [25]. The Brotanek et al. framework highlights how language sensitivity, clinicians’ practices, parental beliefs, folk illnesses, and normative cultural values (i.e., beliefs and behaviors that a particular cultural group values in interpersonal interactions) intersect to create culturally sensitive environments within pediatric primary care settings. The following subscales were excluded from the modified instrument due to lack of relevance in pediatric primary care: Mind–body connections (e.g., “How often did doctors ask how your health was affecting your life?”), preventive care (e.g., “How often did doctors talk to you about ways to stay healthy?”), modesty (e.g., “How often did doctors take a few moments to put you at ease before examining you?”), and use of prescription medications (e.g., “How often did doctors ask if you might have concerns about taking prescription medicines?”). The ‘sensitivity to immigrant status’ and ‘discrimination due to language needs’ subscales were excluded since they did not apply to all patients; a majority of our patient population was not recent immigrants and preferred to converse in English. The remaining 8 subscales included complementary and alternative medicine, causal attributions, family involvement, spirituality, clinician discrimination due to education, clinician discrimination due to racial and ethnic background, office staff discrimination due to racial and ethnic background, and sensitivity to language needs.
The CCSS-29 survey instructions and items were then modified for use as a parent-reported instrument in a pediatric primary care context. For instance, instead of asking respondents to reflect on their “experiences talking with their doctors at [clinic name] over the past 12 months,” the revised pediatric version instructed parents to reflect on their “experience bringing your child (who is between the ages of 3–48 months) to their well-child visits (which is also called a routine visit or a physical exam).” These adaptations were straightforward; for instance, an item from the ‘causal attribution of health problem’ domain was changed from “How often did doctors seem interested in what you thought might be causing your health problem?” to “How often did your child's doctors seem interested in what you thought might be causing your child's health problem?” The original CCSS-29 included three items regarding sensitivity to language needs; after pilot testing, we dropped two questions regarding interpreter use since a majority of parents preferred to converse in English. No other modifications to the original CCSS-29 item wording or response options were made. Possible responses to each item were ‘Never,’ ‘Rarely,’ ‘Sometimes,’ ‘Usually,’ ‘Always.’ Higher scores may indicate higher or lower cultural sensitivity depending on the specific question. Thus, we recoded all responses from 1 to 5, with 5 being the most culturally sensitive. A copy of the original CCSS-29 and modified instruments (in English, Haitian Créole, and Spanish), which we refer to as the CCSS-PPC throughout this study, are included as Appendices A1-A4 in Supplementary material.
Linguistic Translation and Cultural Adaptation
A bilingual researcher modified the original Spanish CCSS-29 translations to match our adapted English CCSS-PPC. Another bilingual researcher then back-translated the Spanish survey to ensure linguistic and conceptual equivalence. For Haitian-Creole surveys, we sent the English version of the survey to a professional translation service which was then back-translated by a bilingual research assistant. Four bilingual participants piloted the Spanish and Haitian-Creole surveys prior to use. Participants were asked about how easily they understood the questions, their interpretation of the questions, and how well the questions were translated from English to ensure the CCSS-PPC was interpreted as intended.
Study Participants
A convenience sampling approach was used to identify eligible participants at an urban pediatric primary care clinic affiliated with a large, urban safety-net hospital in Boston, MA. Caregivers or parents (18 + years) with at least one child aged 3–48 months were recruited before or after well-child visits. Recruitment occurred from December 2017 to August 2018 [19]. After reviewing a consent form and assenting to participate, caregivers/parents completed the CCSS-PPC via electronic tablet in a private location. Respondents were able to complete the survey in either English, Haitian Creole or Spanish. Respondents were also given the choice to either complete the survey independently or have the survey read to them by a trained research assistant. Participants received a ten-dollar gift card for their time.
Analytic Approach
Our analysis proceeded in three main steps. Surveys were first compiled, reviewed for missing data, and sample descriptive statistics were calculated. Item-level missingness was generally low (0–5%); we excluded 19 respondents (9% of total) that were missing answers to one or more items. In sensitivity analyses, we replaced missing answers using random forests and multiple imputation using chained equations to reduce the potential for non-response bias [26]. Second, we conducted exploratory factor analyses (EFAs) using ordinary least squares estimation and oblimin rotation to explore the dimensionality of the adapted CCSS-PPC in our study sample. We conducted a scree test to explore the optimal number of factors. A scree test involves visual examination of the eigenvalues, which indicate the proportion of variance explained by each additional factor, for a natural breakpoint. We also employed three common statistical approaches to inform the number of factors we would extract in our EFA models; these included parallel analysis, the acceleration factor, and the optimal coordinates index [27].
Third, we conducted a series of confirmatory factor analyses (CFA) using maximum likelihood estimation based on the results of the EFAs. We considered factor loadings of ≥ 0.40 and an adequate fit to the specified CFA model as supportive evidence for the given factor structure. Adequate fit was defined as a scaled root mean square error approximation (RMSEA) ≤ 0.10, a Tucker–Lewis Index (TLI) and Comparative Fit Index (CFI) of ≥ 0.90, and standardized root mean square residual (SRMSR) of ≤ 0.08 [28,29,30,31,32,33]. We also used likelihood ratio tests to compare the fit of alternative factor solutions to the 8-factor model implied by the original CCSS instrument. The internal consistency reliability of each factor was examined by Cronbach’s α, where coefficients \(\ge\) 0.70 were considered adequate for group-level measurement.
Boston University Medical Campus’s Institutional Review Board determined this study to be exempt. All analyses were conducted using R version 4.0.2 and the associated ‘psych’ and ‘lavaan’ packages [34, 35]. Our analytic dataset and R scripts are available upon request.
Results
Of the 299 individuals approached during recruitment, 212 agreed to participate (71% response rate) and their characteristics are contained in Table 1. The majority of participants were between the ages of 25–34 (50%), identified as female (81.1%), and either attended at least some college or completed a college degree (59.4%). A diverse mix of racial and ethnic backgrounds were represented; the largest group identified as Black, African American, or African (46.2%).
Item-level means, standard deviations, and inter-item correlations for the revised CCSS-PPC questionnaire are contained in Table 2. Respondents were most likely to report that doctors inquired if they used any alternative medicines (CAM1, mean 3.21), expressed interest in what they thought may be causing the health problem (CAUSAL1, mean 4.18) or that doctors really seemed to listen to what they thought was causing the health problem (CAUSAL2, mean 4.37). Respondents were least likely to report that staff discriminated against them due to their race/ethnicity (STAFF_DIS_RACE1, mean 1.10), that staff made negative assumptions about them due to their race/ethnicity (STAFF_DIS_RACE2, mean 1.10), or discrimination due to language needs (LANG1, mean 1.12). In general, items within each of the Nápoles et al. domains were highly correlated. However, answers to the discrimination-related questions (comprising three original domains of the CCSS-29) were all highly correlated.
Results of the scree test (Fig. 1), parallel analysis, and optimal coordinates index supported a three-factor model. Results of the acceleration factor supported a two-factor model. Based on these results we decided to test two-, three-, and four-factor models in our EFAs and CFAs. In our four-factor EFA, one of the racial discrimination items (DIS_RACE1) had a higher factor loading with the education-related discrimination items.
The two-factor CFA model demonstrated poor fit across RMSEA, TLI, CFI, and SRMSR. The three-factor CFA model demonstrated poor fit across RMSEA, TLI, and CFI. The four-factor CFA model demonstrated adequate fit according to TLI, CLI, and SRMSR, but RMSEA exceeded the 0.10 threshold. The eight-factor CFA model demonstrated adequate fit according to CFI and SRMSR, but poor fit according to RMSEA and TLI (Table 3). In an effort to capture conceptually broad versus conceptually narrow constructs which often have poor predictive power [36], we tested a modified 3-factor CFA model with all discrimination items loading onto a single factor and with residual correlations between DIS_RACE1, DIS_ED1, and DIS_ED2 items allowed. We found that fit statistics for the three-factor CFA model improved substantially after allowing for residual correlation. The modified three-factor model also generally outperformed the other factor structures in terms of fit statistics; this model with residual correlation minimized RMSEA (0.098), maximized both TLI (0.936) and CFI (0.950), and demonstrated adequate fit according to SRMSR (0.061). Results of the likelihood ratio tests demonstrate that the modified three-factor model minimized both Akaike Information Criteria and Bayesian Information Criteria and thus outperformed all other factor structures that we tested.
Factor loadings for the final three-factor EFA and CFA models are contained in Table 4. Loadings for other models are available as Appendices A5-A8 in Supplementary material. The first factor comprises six items and combines three of the original Nápoles et al. domains: discrimination due to education, discrimination due to race/ethnicity and staff discrimination due to race/ethnicity (Cronbach’s ɑ = 0.96, 95% CI 0.95 to 0.97). The second factor comprises six items and combines four of the original domains: complementary and alternative medicine, family involvement, spirituality, and sensitivity to language needs (Cronbach’s ɑ = 0.86, 95% CI 0.83 to 0.89). The third factor comprises two items and is identical to the original ‘causal attribution of health problem’ domain (Cronbach’s ɑ = 0.85, 95% CI 0.81 to 0.89). Our model suggests three domains centered around 1) discrimination, 2) culturally-affirming practices, and 3) causal attribution of health problems. In sensitivity analyses, we repeated our analyses after imputation of missing data. This produced identical factor loadings (see Appendices A9-A11 in Supplementary material).
Discussion
We evaluated the psychometric validity and reliability of the CCSS-PPC for use as a parent-reported measure of CSC in a pediatric primary care setting. Our analyses suggested a more streamlined conceptual model consisting of a three-factor model for clinician cultural sensitivity, a finding which differs from the eight-domain model implied by the original CCSS-29. Our model suggests three domains centered around 1) discrimination, 2) culturally-affirming practices, and 3) causal attribution of health problems. Fig. 2 shows the full-text of each of the CCSS-PPC questions that fall within associated domains. ‘Discrimination’ consists of a series of questions regarding discrimination experiences, such as negative assumptions and experiences from both doctors and administrative staff made about a parent/caregiver’s level of education, race/ethnicity, and levels of comprehension. ‘Culturally-affirming practices’ incorporates questions relating to traditional medicinal practices, inclusion of extended family members in child health care visits and decision-making, and recognition of spiritual beliefs and language preferences. The last domain, ‘causal attribution of health problems’ consists of two questions asking whether parent/caregiver beliefs regarding the cause of their child’s illness are considered by clinicians.
The strengths of our model include being a simplified and streamlined conceptual framework that has been tested and applied in a safety-net pediatric population. The CCSS-PPC was framed by Brotanek et al.’s (2008) conceptual framework for cultural competency. Notably, our model includes ‘discrimination’ which is not within the Brotanek et al. framework. This highlights a significant gap in the original conceptual framework; namely considering racism as a structural factor relating to cultural sensitivity. The inclusion of ‘discrimination’ as a construct combines multiple domains pertaining to discrimination in the original CCSS-29 model. ‘Discrimination’ as a domain is important to highlight as the questions posed often relate to an individual’s internal beliefs regarding medical interactions as opposed to validation of external discriminatory experiences. For instance, the domains of ‘complementary and alternative medicine, language, and family’ are combined into our second domain, ‘culturally-affirming practices’. Considering the plurality of traditional beliefs and practices that may be relevant for clinical care, the results of our analyses support the validity of culturally-affirming practices as a singular domain strengthening the overall validity of the measure as a whole.
Examining and actively working to dismantle patients’ experiences of discrimination is a critical tenet to the provision of CSC. However, this survey is limited in its focus on interpersonal discrimination; while important, future cultural sensitivity measures should consider strategies to dismantle how structural and institutional factors impact CSC. Examples include recruitment, retention, promotion, and support of underrepresented minority healthcare providers, universal access to and use of interpreter services in healthcare settings, providing social service support for patients living in poverty, increasing access to pediatric primary care services, among many others [37, 38].
Limitations
Our findings should be considered in light of several limitations. While our response rate was high (71%), we were unable to collect demographic information on individuals who declined participation and could not assess the potential for non-response bias. We used a convenience sample at a single large urban safety-net hospital in New England and future research should attempt to replicate our findings in other patient populations. Our sample primarily consisted of respondents who were native-born or long-term U.S. residents; our findings may not generalize to new immigrants. While we aimed to minimize any risk of coercion for our participants, we acknowledge that this risk was not entirely eliminated given the context of a majority non-English-speaking participant population. Additionally, our model was tested and validated in caregivers and parents of a pediatric cohort ages 3–48 months; it should be further validated in additional samples such as parents of older children. Notably, our survey is intended for use with parents; future studies should consider ways to collect perceptions of cultural sensitivity from adolescents directly. We also substantially modified and streamlined the CCSS domains using Brotanek et al.’s conceptual framework for cultural competency, eliminating those questions that were determined to not be too general and not focused on issues of cultural sensitivity. Our findings may thus not apply to users of the original unadulterated survey instrument.
Conclusions
The streamlined CCSS-PPC assesses culturally-sensitive care along three dimensions: discrimination, culturally-affirming practices, and causal attribution of health problems. In this racially and ethnically diverse sample of parents at a large urban academic medical center, we found this simplified three-factor conceptual model outperformed all measured alternatives.
References
Smedley, B. D., Stith, A. Y., & Nelson, A. R. (2003). Institute of Medicine, Committee on Understanding and Eliminating Racial and Ethnic Disparities in Health Care. Unequal treatment: confronting racial and ethnic disparities in healthcare.
Committee on Pediatric Workforce. Ensuring culturally effective pediatric care: implications for education and health policy. Pediatrics. 2004;114(6):1677–85.
Flores G, Committee on Pediatric Research. Racial and ethnic disparities in the health and health care of children. Pediatrics. 2010;125(4):e979–1020.
Cohen AL, Christakis DA. Primary language of parent is associated with disparities in pediatric preventive care. J Pediatr. 2006;148(2):254–8.
Chokshi DA. Income, poverty, and health inequality. JAMA. 2018;319(13):1312–3.
Okoniewski W, Sundaram M, Chaves-Gnecco D, McAnany K, Cowden JD, Ragavan M. Culturally sensitive interventions in pediatric primary care settings: a systematic review. Pediatrics. 2022;149(2):e2021052162.
Castro A, Ruiz R. The effects of nurse practitioner cultural competence on Latina patient satisfaction. J Am Assoc Nurse Pract. 2009;21:278–86.
Alfred M, Ubogaya K, Chen X, Wint D, Worral PS. Effectiveness of culturally focused interventions in increasing the satisfaction of hospitalized Asian patients: a systematic review. JBI Evidence Synthesis. 2016;14(8):219–56.
Ayonrinde O. Importance of cultural sensitivity in therapeutic transactions. Dis Manag Health Out. 2003;11(4):233–48.
Sorensen J, Norredam M, Dogra N, Essink-Bot ML, Suurmond J, Krasnik A. Enhancing cultural competence in medical education. Int J Med Educ. 2017;8:28.
Hausmann LR, Hannon MJ, Kresevic DM, Hanusa BH, Kwoh CK, Ibrahim SA. Impact of perceived discrimination in health care on patient-provider communication. Med Care. 2011;49(7):626.
Nong P, Raj M, Creary M, Kardia SL, Platt JE. Patient-reported experiences of discrimination in the US health care system. JAMA Netw Open. 2020;3(12):e2029650–e2029650.
Cuevas AG, O’Brien K, Saha S. African American experiences in healthcare: “I always feel like I’m getting skipped over.” Health Psychol. 2016;35(9):987.
Beach MC, Price EG, Gary TL, Robinson KA, Gozu A, Palacio A, Cooper LA. Cultural competency: a systematic review of health care provider educational interventions. Med Care. 2005;43(4):356.
Carrillo JE, Green AR, Betancourt JR. Cross-cultural primary care: a patient-based approach. Ann Intern Med. 1999;130(10):829–34.
Day TW. Cross-cultural medicine at home. Minn Med. 1992;75(3):15–7.
Riffenburgh RS. Communication in cross-cultural medical practice. West Med; Med J West. 1966;7(11):320–2.
Brach C, Fraserirector I. Can cultural competency reduce racial and ethnic health disparities? A review and conceptual model. Med Care Res Rev. 2000;57(1_suppl):181–217.
Ragavan MI, Griffith KN, Cowden JD, Colvin JD, Bair-Merritt M. Parental perceptions of culturally sensitive care and well-child visit quality. Acad Pediatr. 2020;20(2):234–40.
DunnGalvin A, De BlokFlokstra BMJ, Burks AW, Dubois AEJ, Hourihane JOB. Food allergy QoL questionnaire for children aged 0–12 years: content, construct, and cross-cultural validity. Clin Exp Allergy. 2008;38(6):977–86.
Yucelen AG, Rodopman-Arman A, Topcuoglu V, Yazgan MY, Fisek G. Interrater reliability and clinical efficacy of Children’s Yale-brown obsessive-compulsive scale in an outpatient setting. Compr Psychiatry. 2006;47(1):48–53.
Finley GA, Kristjánsdóttir Ó, Forgeron PA. Cultural influences on the assessment of children’s pain. Pain Res Manage. 2009;14(1):33–7.
Nápoles-Springer AM, Santoyo J, Houston K, Pérez-Stable EJ, Stewart AL. Patients’ perceptions of cultural factors affecting the quality of their medical encounters. Health Expect. 2005;8(1):4–17.
Nápoles AM, Santoyo-Olsson J, Farren G, Olmstead J, Cabral R, Ross B, Gregorich SE, Stewart AL. The patient-reported Clinicians’ cultural sensitivity survey: a field test among older Latino primary care patients. Health Expect. 2012;15(1):63–77.
Brotanek JM, Seeley CE, Flores G. The importance of cultural competency in general pediatrics. Curr Opin Pediatr. 2008;20(6):711–8.
Shah AD, Bartlett JW, Carpenter J, Nicholas O, Hemingway H. Comparison of random forest and parametric imputation models for imputing missing data using MICE: a CALIBER study. Am J Epidemiol. 2014;179(6):764–74.
Costello AB, Osborne J. Best practices in exploratory factor analysis: four recommendations for getting the most from your analysis. Pract Assess Res Eval. 2005;10(1):7.
Hu LT, Bentler PM. Cutoff criteria for fit indexes in covariance structure analysis: conventional criteria versus new alternatives. Struct Equ Modeling. 1999;6(1):1–55.
Steiger JH. Structural model evaluation and modification: an interval estimation approach. Multivar Behav Res. 1990;25(2):173–80.
Chen F, Curran PJ, Bollen KA, Kirby J, Paxton P. An empirical evaluation of the use of fixed cutoff points in RMSEA test statistic in structural equation models. Sociol Methods Res. 2008;36(4):462–94.
Muthén, B. O. (1997). Robust inference using weighted least squares and quadratic estimating equations in latent variable modeling with categorical and continuous outcomes. Psychometrika.
Satorra A, Bentler PM. A scaled difference chi-square test statistic for moment structure analysis. Psychometrika. 2001;66(4):507–14.
Xia, Y. (2016). Investigating the chi-square-based model-fit indexes for WLSMV and ULSMV estimators (Doctoral dissertation, The Florida State University).
Revelle, W., & Revelle, M. W. (2017). Procedures for psychological, psychometric, and personality. R Package Bpsych^, Version 1 (5).
Rosseel Y. Lavaan: an R package for structural equation modeling and more version 0.5–12 (BETA). J Stat Softw. 2012;48(2):1–36.
Reise SP, Moore TM, Haviland MG. Bifactor models and rotations: exploring the extent to which multidimensional data yield univocal scale scores. J Pers Assess. 2010;92(6):544–59.
Jindal M, Heard-Garris N, Empey A, Perrin EC, Zuckerman KE, Johnson TJ. Getting “Our House” in Order: re-building academic pediatrics by dismantling the anti-black racist foundation. Acad Pediatr. 2020;20(8):1044–50.
Ragavan MI, Cowden JD. The complexities of assessing language and interpreter preferences in pediatrics. Health equity. 2018;2(1):70–3.
Funding
This work was supported by Agency for Healthcare Research and Quality (Grant Numbers T32 HS022242, K12 HS026395, T32 HS022242).
Author information
Authors and Affiliations
Corresponding author
Additional information
Publisher's Note
Springer Nature remains neutral with regard to jurisdictional claims in published maps and institutional affiliations.
Supplementary Information
Below is the link to the electronic supplementary material.
Rights and permissions
Springer Nature or its licensor (e.g. a society or other partner) holds exclusive rights to this article under a publishing agreement with the author(s) or other rightsholder(s); author self-archiving of the accepted manuscript version of this article is solely governed by the terms of such publishing agreement and applicable law.
About this article
Cite this article
Loo, S., Brady, K.J.S., Ragavan, M.I. et al. Validation of the Clinicians’ Cultural Sensitivity Survey for Use in Pediatric Primary Care Settings. J Immigrant Minority Health 25, 790–802 (2023). https://doi.org/10.1007/s10903-023-01469-2
Accepted:
Published:
Issue Date:
DOI: https://doi.org/10.1007/s10903-023-01469-2