Abstract
Quantity and quality distribution of surface water and groundwater are changing under the impacts of both climate variability and human activities. The main goal of this paper is to evaluate the abovementioned impacts on the water resources in the Saveh plain, central Iran. To achieve this aim, spatial and temporal changes of the surface and groundwater quality and quantity have been analyzed, using hydrometric and meteorological data. The nonparametric Mann-Kendall test was used to identify trends and change points in the annual rainfall and runoff for the period of 1946 to 2011. In order to analyze the impacts of the Saveh Dam on runoff, the dam operation year, 1994, was considered as a change point. Mann-Kendall test results show that rainfall time series was divided into two parts, namely, 1966–1989 and 1990–2007, and averages of annual rainfall in five stations increase from 10 to 21 %. Also, runoff time series was divided into two parts, namely, 1946–1995 and 1996–2007 and averages of annual runoff in four stations decrease from 8 to 83 %. Results show that rainfall changes in Shahabasi, Razin, Jalayer, Emamabad, and Ahmadabad stations increased from 9 to 33 % before and after 1994. Nevertheless, runoff decreased from 24 to 81 %. The results indicate that the greatest lack of runoff between stations is at Shahabasi station and one important reason for the severe lack is operation of the Saveh Dam in 1994. Highest groundwater level decline, about 168.67 cm, occurred in 1994 that is the operation year of the Saveh Dam. Trend analysis of surface water quality show that electrical conductivity increased 957.34 μmho/cm before and after 1994. Also, the Wilcox water quality classification method has been reduced from C3-S1 to C4-S2. Average groundwater electrical conductivity (EC) during 1999–2003 and 2004–2009 increased to 89.6 μmho/cm. Also, the groundwater quality indices for agricultural usages are classified in four classes including, C4-S2 16, C4-S1 46, C3-S1 30, and C2-S1 8 % of the total aquifer area. In conclusion, in order to have a sustainable development, the effects of water projects on environment and water resources need to be predicted very carefully.
Similar content being viewed by others
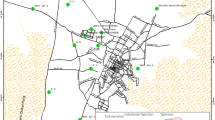
Explore related subjects
Discover the latest articles, news and stories from top researchers in related subjects.Avoid common mistakes on your manuscript.
Introduction
Groundwater is the only source of potable water in many areas. It is also the main source of water for agricultural and industrial sectors in many semi-arid areas. It has been emphasized by Estrela et al. (1996) and Scheidleder et al. (1999) that in semi-arid areas, groundwater is increasingly exploited as a resource for public water supply and irrigation. Therefore, the response of groundwater systems to drought and human activities is ever more important. On the other hand, as stated by Ahmadi and Sedghamiz (2007), Iran is located in an arid to semi-arid region of the world and about 95 % of freshwater is allocated for agriculture, out of which 80 % is supplied through groundwater. Therefore, it is clear that groundwater is the vital component for sustainable agriculture. In recent years, many fertile and agricultural plains, such as Fars, suffered from 0.5 to 15 m water table level drop, in which many wells are now out of use. Serious restrictions and regulations about increasing the number of agricultural wells in problematic areas are established by the government. Dragoni and Sukhija (2008) stated that the use of groundwater can mitigate droughts, because many aquifers have a large storage capacity and are potentially less sensitive to climate change than surface water bodies, which often rely on groundwater discharge to maintain base-flow conditions. However, the ability to use groundwater storage to buffer rainfall deficits that affect surface water resources will be constrained by the need to protect groundwater-dependent environmental systems (Skinner 2008). Drought affects on groundwater recharge, groundwater levels, and groundwater discharge, respectively. Therefore, climate variability affects components of the groundwater systems including recharge, discharge, flow, storage, and surface-subsurface interactions.
Following a review of previous studies in survey impacts of climate variability on groundwater, it was found that most efforts so far have been focused on investigating sensitivity of groundwater levels to climate variability. For instance, Van der Kamp and Maathuis (1991) investigated the annual fluctuation of groundwater levels as a result of loading by surface moisture considering both theoretical aspects of aquifer characteristics and empirical data. They observed that the relatively poor correlation between climatic parameters and groundwater levels was due to the distance of the observation wells from climate stations. Chen et al. (2002) also emphasized in order to link climate variables with groundwater levels, the weather station should exist in the recharge zone of the observation well. Also, Chen et al. (2004) in another study used cross correlation analysis between historical climate records and groundwater levels to examine the relative importance of climate on groundwater level variation. Their results showed that annual rainfall explained the variations in groundwater levels significantly. Eltahir and Yeh (1999), using the crossing theory approach, assessed the asymmetric response of aquifer water level to floods and droughts. They reported that the drought left a significantly more persistent signature in the aquifer water level than the corresponding signature of the flood. Taylor and Alley (2001) commended that the groundwater level data itself provide a direct means of measuring the overall impacts of both natural and anthropogenic changes to groundwater resources. Although the groundwater monitoring networks have existed for several years, very little research has been carried out internationally to interpret the water table and quality trends.
Broers and Grift (2004) studied the groundwater quality trends due to anthropogenic-induced changes in agricultural practices. They employed a combination of Mann-Kendall nonparametric trend analysis of time series at specific depths and time-averaged concentration–depth profiles. By plotting the median and other percentiles over years, Almasri and Kaluarachchi (2004) evaluated that the nitrate pollution trend of groundwater in agriculture dominated watersheds. They combined the concentration data of different wells having few observations to reduce the uncertainty of predictions and conclusions.
On the other hand, as reported by much researches, human interventions affect directly, withdrawal, construction water surface regulation structural, and indirectly, land use and anthropogenic climate change on groundwater resources (Woldeamlak et al. 2007; Brouyère et al. 2004; Scibek and Allen 2006 and Dams et al. 2008). According to Kundzewicz et al. (2007), the IPCC (2007a) stated that a lack of necessary data has made it impossible to determine the magnitude and the direction of groundwater change due solely to climate change. Thus, there is a need to evaluate and understand climatic variability over a long time to better plan and manage groundwater resources well into the future, while taking into consideration the increasing stresses on those resources from population growth and industrial, agricultural, and ecological needs (Warner 2007). Groundwater quantity and quality vary in time depending on natural and anthropogenic drivers. Potential impacts of regional climate patterns and trends on surface water have been studied in some researches, but about groundwater remain effects are poorly understood (Green et al. 2007a, b, and Green et al. 2007a). Also, groundwater response to climate variability coupled with human activities is little known (Holman 2006; Green et al. 2007b; Bovolo et al. 2009). Some of the relevant literatures are mentioned below.
Bao et al. (2012) assessed the attribution of climate variability and human activities for streamflow decrease quantitatively in three catchments located in different parts of the Haihe River Basin. Their results indicated that the decrease of streamflow between the two periods could be attributed to 58.5 % (41.5 %), 40.1 % (59.9 %), and 26.1 % (73.9 %) from climate variability (human activities) in the Taolinkou, Zhangjiafen, and Guantai catchment, respectively. Shanshan et al. (2012) quantified the impacts of climate variability and human activities on streamflow in the water source area of the Baiyangdian Lake, based on analyses of hydrologic changes of the upper Tanghe River catchment (a sub-basin of the Baiyangdian Basin) from 1960 to 2008. Their results showed that the annual streamflow decreased significantly (significance level 0.05) by 1.7 mm, and an abrupt change was identified around the year 1980. The quantification results indicated that climate variations accounted for 38–40 % of decreased streamflow, while human activities accounted for 60–62 %. Therefore, the effect of human activities played a dominant role on the decline of the streamflow in the water source area of the Baiyangdian Lake. Wu and Liu (2012), to evaluate the effects of human activities such as land uses and reservoir operation on the hydrological and nonpoint source (NPS) pollution processes in a highly agricultural area, the Iowa River Basin, used the Soil and Water Assessment Tool (SWAT). Their study highlights the need to further develop a more complete but feasible algorithm to represent the nutrient removal mechanism in a reservoir for a watershed/water quality model. Zhang et al. (2012) have investigated the impacts of climate change and human activates on the runoff for the Huifa River Basin, northeast of China, with the SWAT, which calibrated and verified for the baseline period 1956–1964, and then used to reconstruct the natural runoff from 1965 to 2005. Their results indicate that both climate change and human activities are responsible for the decrease of observed runoff in the Huifa River. However, human activities contributed more to runoff decrease in wet years due to regulation and storage of the water projects.
Ye et al. (2013) analyzed the characteristics of hydro-climatic changes of the Poyang Lake catchment based on the observed data for the period 1960–2007. Their results showed that for the catchment average water balance, quantitative assessment revealed that climate change resulted in an increased annual runoff of 75.3–261.7 mm in the 1970s–2000s for the Poyang Lake catchment, accounting for 105.0–212.1 % of runoff changes relative to the 1960s. However, human activities should be responsible for the decreased annual runoff of 5.4–56.3 mm in the other decades, accounting for −5.0 to −112.1 % of runoff changes.
Chen et al. (2013) used the nonparametric Mann-Kendall test and the Mann-Kendall-Sneyers test to identify trend and step change point in the annual runoff for data from the Kaidu River Basin in the arid region of northwest China during the period of 1960–2009. They employed the hydrologic sensitivity analysis method to evaluate the effects of climate variability and human activities on mean annual runoff for the human-induced period based on precipitation and potential evapotranspiration. Their results indicated that in 1994–2009, climate variability was the main factor that increased runoff with contribution of 90.5 %, while the increasing percentage due to human activities only accounted for 9.5 %, showing that runoff in the Kaidu River Basin is more sensitive to climate variability than human activities. Wu and Chen (2013) used the physically based hydrological/water quality model, Soil and Water Assessment Tool, to investigate the influence of PS and NPS pollution on the water quality of the East River (Dongjiang in Chinese) in southern China. Their results indicate that NPS pollution was the dominant contribution (>94 %) to nutrient loads except for the mineral phosphorus (50 %). Wang et al. (2013) used hydro-climatic data from four catchments including the Luanhe, Chaohe, Hutuo, and Zhanghe River catchments, in the Haihe River basin from 1957 to 2000 to quantitatively attribute the hydrological response (i.e., runoff) to climate change and human activities, separately. They found that human activities were the main driving factors for the decline in annual runoff in the Luanhe River catchment, Chaohe River catchment, and Zhanghe River catchment, accounting for over 50 % of runoff reduction. However, climate variability should be responsible for the decrease in annual runoff in the Hutuo River catchment.
Guo et al. (2014) have conducted an investigation into attributing the runoff response to climate change and human activities in two catchments named Wushan and Shetang, situated in the upper reaches of the Weihe River, in China. The results show that the decline in annual runoff over both catchments can be mainly attributed to the human activities and the reduction percentages due to human activities range from 59 to 77 %. Hu et al. (2014) have investigated a quantitatively analyses of climate fluctuation impacts and human activities on the decreasing streamflow in the semi-arid Huangfuchuan River basin in the middle reaches of the Yellow River basin, from 1985 to 2006. Their results show that the climate fluctuations accounted for a decrease in mean annual streamflow of approximately 10.38 mmyear−1 (51.03 %), whereas human activities, including soil–water conservation measures, water intakes, and man-made water storages, caused a decrease of approximately 9.96 mmyear−1 (48.97 %).
On the other hand, some researches have emphasized on the importance of including the effects of mutually human activities and climate variability on both surface and groundwater resources with respect to the quantity and/or water quality aspects. Generally speaking, McCarthy et al. (2001), Karl and Trenberth (2003), Sophocleous (2004), Barnett et al. (2006), Hennessy et al. (2006), Wentz et al. (2007), and Adger and Coauthors (2007) highlighted that the subsequent effects on global and regional climates are uncertain, especially on groundwater resources. Although, significant development has been made toward assessments of surface water, little is known about how groundwater might respond to climate change and variability. While climate change impacts directly on surface water resources, the relationship between climate variables and groundwater is more complex and difficult to quantify. Groundwater resources are affected by climate variability through direct interaction with surface water resources, such as rivers, and indirectly through the recharge process. Therefore, quantifying the impact of climate change on groundwater resources requires not only reliable understanding of changes in major climatic variables, but also accurate estimation of groundwater recharge. Furthermore, most groundwater systems have already been distorted by human activities that are not necessarily related to climate variability. Thus, there are vital and ongoing needs to address the expected coupled effects of human activities and climate variability on groundwater resources.
As mentioned earlier, the main objective of this paper has been to evaluate impacts of both human activities and climate variability on quantity and quality of water resources, equally surface and groundwater, in the Saveh plain, central Iran, to achieve a wider scientific understanding of all aspects of the phenomena. To achieve this goal, not only changes in rainfall, runoff, and construction of a huge reservoir dam have been studied but exploitation of groundwater and management-induced effects have been also considered. As the above review of the previous studies in this field show, so far, most effort has been focused on individual studies of one or two of the abovementioned phenomena, i.e., surface or groundwater and quantity or quality of them. Hence, the emphasis of this paper has been to study the different impacts of the human activities and climate variability on these phenomena.
Materials and methods
Study area
The study area is the Saveh plain, central Iran, which is located between 34° 45′ to 35° 03′ N and 50° 08′ to 50° 50′ E, with an elevation of about 1100 m above sea level (see Fig. 1). In the study area, the annual long-term mean rainfall is 202 mm, the annual average temperature is 18.2 °C, and the annual average humidity is 39 % with a dry climate. Wheat, barley, vegetables, and some other garden plants are extensively cultivating in this area. The extension of the aquifer is 1260 km2. In the area, because of severe overexploitation, the groundwater extent is divided, by the government, into the forbidden exploitation area and the limited exploitation area (Ministry of Energy, Iran Water Resources Management Company 2005).
Data
In Fig. 1, the locations of gauging and rainfall stations are illustrated. In this paper, monthly and yearly rainfall data of five meteorological stations including Razin, Jalayer, Shahabasi, Ahmadabad, and Emamabad, and data of four hydrometric stations named Razin, Jalayer, Shahabasi, and Asgarabad were used. Shahabasi station is located downstream of the Saveh Dam. Moreover, yearly temperature, groundwater level data (32 piezometric wells), groundwater discharge, and quality data of surface and groundwater have been used. All of used data were collected and recorded by the Iran Water Resources Management Company (IWRM). The used data in the research were presented in Table 1.
Monthly surface water withdrawals data from April 2001 to March 2012 (132 months) were used. Surface water quality data including electrical conductivity (EC) and sodium adsorption ratio (SAR) with annually time step from 1966 to 2005 were used. The surface water quality data were collected in Razin, Jalayer, and Shahabasi stations.
Groundwater data is used including monthly groundwater level in 32 observation wells from March 2003 to February 2007 (48 months), yearly groundwater fluctuations data from 1988 to 2007, and monthly groundwater discharge data from April 2002 to March 2009 (84 months). Also, yearly groundwater quality data including EC, SAR, total dissolved solids (TDS), total hardness (TH), pH, Mg+2, and Ca+2 parameters from 1999 to 2009 were used.
Trend test and change point analysis
The nonparametric Mann-Kendall (MK) trend test is frequently used to assess trends of hydrological time series (Zhang et al. 2012; Chen et al. 2013). For a time series x1,x 2,x3,…,xn, the MK rank statistics is defined as:
The mean and variance of the test statistics (Sk) are:
The test statistics (UFk) is calculated by the following formula:
The values of UFk constitute a forward sequence curve (UF). For the given α significance level and U α (the critical value of the standard normal distribution), if ǀUFk > U αǀ, it means the forward sequence curve (UF) has a trend α significance level. The same equation is then used to the inversed series (xn,…,x3,x 2,x1) for backward sequence values of the statistics UBk (k = n, n-1,…,1) and gets a backward sequence curve (UB). The intersection point of UF and UB within the confidence interval is the time when a change point occurred. More details of MK test are explained in Zhang et al. (2012) and Chen et al. (2013).
According to the analysis results of trend and step change point analysis, the runoff and groundwater series divided into a natural and a human-induced period series (Huo et al. 2008; Jiang et al. 2011; Chen et al. 2013).
Correlation analysis between rainfall and groundwater fluctuation
Rainfall is the most important variable of climate which impacts the groundwater recharge. Therefore, the Standardized Precipitation Index (SPI) was used to investigate the impact of drought on groundwater levels. SPI is computed by dividing the difference between normalized seasonal rainfall and its long-term seasonal mean by the standard deviation to measure rainfall shortage, the index was first proposed by McKee et al. (1993):
Where; X ij is the seasonal rainfall at the ith rain gauge station and the jth observation, X im is the long-term seasonal mean and σ is its standard deviation. Since SPI is equal to the z value of the normal distribution. McKee et al. (1993, 1995) proposed a seven-category classification for SPI (see Table 2).
In this study, in order to determine the appropriate time scale, Standardized Precipitation Index, the correlation of SPI based on time scales of 1, 3, 6, 9, 12, 18, 24, and 48 months with groundwater levels were calculated. Also, to consider time delay impact of precipitation on groundwater levels, correlation between SPI and groundwater level data has been computed for 1, 2, 3, 4, 5, 6, 12, and 24 months. Moreover, the Pearson correlation coefficient has been worked out using Eq. (2) below (Pearson 1895).
Where, x and y are SPI and groundwater level parameters, respectively. S x and S y are standard deviation of variables x and y, and r xy is the covariance between the variables x and y.
According to the initial survey, it was found that in the case study of this paper, runoff not only has been affected by anomaly rainfall but it has also been influenced by the construction of the Saveh Dam. Therefore, in order to recognize such effects, runoff variations have been analyzed.
Water quality analysis
To evaluate water quality analysis, Wilcox water quality classification method is used. In order to avoid the longsome and tedious explanation of used methods, for more details see Wilcox (1955).
Results and discussion
Trend and change point analysis in annual rainfall
Analysis of historical trends in hydrologic processes can help verify the start of the human-induced period. Annual rainfall in five meteorological stations during 1966–2007, annual runoff in four stations during 1946–2007, and groundwater fluctuation in the Saveh plain during 1988–2007 was analyzed using Mann-Kendall test to recognize trends and change point. Figure 2[α] shows both the forward (UFk) and backward (UBk) result of the test at the 0.05 significance level in (a) Shahabasi, (b) Emamabad, (c) Razin, (d) Ahmadabad, and (e) Jalayer stations. The intersection of UFk and UBk curves indicates a changes point in annual rainfall.
The results show that change point occurred in 1980 in Shahabasi station (Fig. 2[α]a), in 1973 and 1990 in Emamabad station (Fig. 2[α]b), in 1980 in Razin stations (Fig. 2[α]c), in 1979 and 1990 in Ahmadabad station (Fig. 2[α]d), and in 1985 in Jalayer station (Fig. 2[α]e). Therefore, we conclude that the abrupt change point in annual rainfall in the Saveh plain occurred around 1980, 1985, and 1990. Based on the Mann-Kendall test, rainfall time series was divided into two parts, namely, 1966–1989 and 1990–2007, which indicate before and after change point. Figure 2[β] shows variation of annual rainfall before and after change points. Different parts of Fig. 2[β] show that averages of annual rainfall in five stations increase from 10 to 21 % (Table 3).
Figure 2[γ] presents yearly SPI in the five mentioned stations. Generally speaking, Fig. 2[γ] shows that all five stations had a situation near normal, (−0.99 < SPI < 0.99), during the studied period. However, as it can be seen in the Fig. 2[γ], an extreme drought occurred in Emamabad station during 1970 and also it experienced severe droughts in 1981 and 1996. On the other hand, an extreme drought occurred in Ahmadabad station during 1978 and 1979. Shahabasi station experienced an extreme drought during 1966 and 1979 and another severe drought in 1996. Also, in Razin and Jalayer stations, extreme droughts occurred in 1997.
Effects of climate variability and human activities on runoff
Effects of climate variability on runoff
Runoff is affected by many factors that can be attributed to climate variability and human activity.
The quantification of the individual impacts is actually difficult because changes in runoff are associated with changes in both climate variability and human activity. In this research, rainfall variability from climate factors and construction of the Saveh Dam and surface water withdrawal from human activities is selected to analyze their impacts on runoff. Figure 3[α] shows the UFk and UBk curves of annual runoff in four (a) Shahabasi, (b) Razin, (c) Jalayer, and (d) Asgarabad stations.
The results show that change point occurred around 1954–1997 in Shahabasi station (Fig. 3[α]a), around 1979–1996 in Razin station (Fig. 3[α]b), around 1985–1996 in Jalayer station (Fig. 3[α]c), and around 1980–1985 in Asgarabad station (Fig. 3[α]d). Therefore, we conclude that the abrupt change point in annual runoff in the Saveh plain occurred around 1996. Based on the Mann-Kendall test, runoff time series was divided into two parts, namely, 1946–1995 and 1996–2007 that indicate before and after change point. Figure 3[β] shows variation of annual runoff before and after change points. Different parts of Fig. 3[β] show that averages of annual runoff in four stations decrease from 8 to 83 % (Table 3).
Trend and change point analysis of annual rainfall and runoff results show that annual rainfall in the studied stations increase from 10 % in Razin station to 33 % in Ahmadabad station. On the other hand, annual runoff decreased from 83 % in Shahabasi station to 5 % in Razin station Although, in some of years, annual runoff increased such as in 1967 in Shahabasi station. Generally, due to increased rainfall and decreased runoff, it can be concluded that human activates impact on runoff. Despite the complexity of the human activities’ impacts on runoff, some of these activities are discussed.
Effects of human activities on runoff
One of the most important factors influencing on runoff is surface water withdrawals. Unfortunately, in the Saveh plain, long-term surface water withdrawals data is not measured. Figure 4 presents variability of monthly surface water withdrawals in the Saveh plain.
Figure 4 shows that in 2006, surface water withdrawals decreased from 6.33 Mm3 to 4.29 Mm3. In other words, surface water withdrawals in 2002–2012 decreased 32 % although the trend of surface water withdrawals (Fig. 4a) in 2005 significantly increased.
Another human activity that affects on runoff is building dams on rivers. Water retaining structures intercept or obstruct the natural flow of water in wet seasons and store water for drier periods. The Saveh Dam is located 25 km west of Saveh city on the Qarachay River (see Fig. 1). The dam, 128 m height, is regulating about 230 million cubic meters of water every year. The Saveh Dam was operated in 1994. In order to analyze the impacts of the Saveh Dam on runoff, the operation year, 1994, was considered a change point, and variability in runoff of three stations, Razin, Jalayer, and Shahabasi has been surveyed. Razin and Jalayer stations and Shahabasi station are located in upstream and downstream of the Saveh Dam, respectively. Therefore, differences between runoff changes in before and after change point, 1994, can indicate effects of dams on runoff. Table 4 shows that rainfall changes in Shahabasi, Razin, Jalayer, Emamabad, and Ahmadabad stations increase from 9 to 33 % before and after 1994. Nevertheless, runoff decreased from 24 to 81 %. The results indicate that greatest lack of runoff between stations is Shahabasi station, and one important reason for the severe lack is operation of the Saveh Dam in 1994.
Also, results of Table 4 show that the runoff decrease in Razin and Jalayer stations are significantly different from the runoff decrease in Shahabasi station. The different decreases between 42 % in Jalayer station and 81 % in Shahabasi station can be seen as a result of the Saveh Dam construction. So, it can be concluded that the contribution of the Saveh Dam in runoff decrease at Shahabasi Station is about 39 %.
Effects of climate variability and human activities on groundwater fluctuation
Figure 5 presents trend and change point analysis of groundwater fluctuations in the Saveh aquifer during 1988–2007.
Results show that significantly decreasing trend in groundwater level started from 1993 and had continued. Table 5 shows the groundwater fluctuation changes before and after change points.
Table 5 shows that highest groundwater level decline, about 168.67 cm, occurred in 1994, that is the operation year of the Saveh Dam. In order to investigate the reasons for the decline in groundwater levels in the aquifer Saveh, climatic and human factors have been studied.
Effects of climate variability on groundwater fluctuation
Table 6 shows the correlation coefficients between SPI and the average groundwater levels for different time scales. In order to maximize the correlation between climatic parameters and groundwater levels, Emamabad and Ahmadabad meteorological stations which are located in the recharge zone of the observation wells were selected to analyze the impacts of drought on groundwater levels. The correlation coefficients between SPI with different time scales of 1, 3, 6, 9, 12, 18, 24, and 48 months and average groundwater levels in the selected areas of Ahmadabad and Emamabad stations were calculated to determine the best time scale for SPI.
As Table 6 reveals, the highest statistically significant correlation coefficient belongs to Ahmadabad station and it is at the scale of 24 months and equal to 0.573. Moreover, the correlation coefficients of 18- and 48-month time scales are significant for the same abovementioned station with values of 0.526 and 0.490, respectively. However, there is only one significant value of the correlation coefficient for Emamabad station, which is equal to 0.750 and at the scale of 48 monthly SPI. As can be seen from the table, the rest of the correlations are not significant at timescales less than 18 months.
It is well known that rainfall affects groundwater levels with a delay time. This part focuses on finding out the effects of rainfall on groundwater levels in the study area. To achieve this aim, according to the results which are presented in Table 6, 24-month time-scaled SPI values for Ahmadabad and 48-month time-scaled SPI values for Emamabad stations have been chosen to investigate the delay time effects of rainfall on groundwater fluctuations. Then, the correlation coefficients between the abovementioned SPI values and monthly average groundwater levels with different time delays of 1, 2, 3, 4, 5, 6, 12, and 24 months were calculated and the results are presented in Table 7, below.
Table 7 indicates that for Ahmadabad and Emamabad stations, the highest correlation coefficient values are with 4- and 5-month delay and are equal to 0.812 and 0.762, respectively. Moreover, the other relevant values of 2-, 3-, 4-, 6-, and 12-month delays are significant at the 0.01 level, for both mentioned stations. Changes of SPI in contrast with average groundwater levels in Ahmadabad station (24-month time-scaled SPI with 4-month delay) and Emamabad station (48-month time-scaled SPI with 5-month delay) are shown in Fig. 6a, b, below.
As Fig. 6a shows, the lack of rainfall from 2 November to 3 December has decreased the groundwater level for a total of 2.058 m from 3 April to 3 November. Drought which occurred during 3 September to 3 November has caused a drop in groundwater level during 4 April to 4 August, equal to 1.275 m. Also, there is another groundwater level drop equal to 2.079 m in November2004, which was due to overexploiting of aquifer to the autumn irrigation usages. From October 2004 to March 2006, the groundwater level fluctuations showed to be highly correlated with the Standardized Precipitation Index values.
Figure 6b shows 48 monthly SPI changes with the average of groundwater level fluctuations for Emamabad station. Average drop in groundwater levels in Emamabad station area from 3 July to 3 November has been 0.925 m, due to drought which occurred through 3 March to 3 July. Drought from 4 March to 4 October had decreased 1.098 m in groundwater levels during 4 April to 4 August. The main drought had occurred during 5 September to 6 July and caused a loss of total 2.251 m in 6 February to 6 October.
However, in order to more accurately assess the impact of rainfall on groundwater level, it is better that characteristics such as intensity, duration, and amount should be investigated, but the results show that the intra-annual rainfall distribution affects groundwater levels with a time delay of about 5 months.
Effects of human activities on groundwater fluctuation
The impact of withdrawals on groundwater fluctuations seems simple, but expected trend may be delayed or obscured by the occurrence of long transient periods after significant water balance changes, or by interferences between several abstraction wells (Custodio 2002). Mann-Kendall test for monthly variation of groundwater discharge in the Saveh aquifer shows there is no significant increase in groundwater discharge during 2001–2011. In 2003, groundwater discharge, about 0.05 Mm3 (4 %), increased. Due to increase in rainfall after 1994 (see Table 5), it can be concluded that the decrease in groundwater level in the Saveh aquifer has been more due to human activities such as dam construction on Saveh, not the result of climate change.
Analysis of surface water and groundwater quality
Analysis of surface water quality
Temporal analysis of quality changes in the Qarachay and Mazlaqan Rivers show an increasing trend in their quality values.
Electrical conductivity in Razin, Jalayer, and Shahabasi stations had shown an increased trend. Electrical conductivity values in Shahabasi station are more than the values of Jalayer station, and the reason can be that Shahabasi station is located downstream of Jalayer station. In order to more accurately evaluate surface water quality changes in the Saveh plain, EC variation in Shahabasi station has been statistically analyzed. Figure 7 presents Mann-Kendall test (a) and yearly variation of EC in Shahabasi station (b) during 1966–2004.
Figure 7a shows that increased trend of EC has begun in 1992. Figure 7b shows that two change points occurred in 1972 and 1992. Average EC during 1966–1971, 1972–1991, and 1992–2004 are equal to 1863.17, 1379.77, and 2230.87 μmho/cm, respectively. According to the Wilcox method, the river water quality had degredated from C3-S1 to C4-S2 class.
Analysis of groundwater quality
To evaluate temporal changes of groundwater quality in the Saveh aquifer, the annual average of water quality parameters from 2002 to 2008 were analyzed.
Results indicate high levels of calcium (Ca2+), electrical conductivity (EC), and total dissolve solutes (TDS) in Saveh groundwater during 2002 to 2008. Comparison of surface and groundwater quality criteria in the Saveh plain show that greater values of electrical conductivity and sodium adsorption ratio belong to the surface waters.
In order to more accurately evaluate groundwater quality changes in the Saveh aquifer, EC variation has been statistically analyzed during 1999–2009. Result of MK test for yearly EC shows that trend EC is not significant. Average EC during 1999–2003 and 2004–2009 are equal to 3234.5 and 3324.1 μmho/cm, respectively. Groundwater quality to agricultural usages in the Saveh aquifer (2008) classified using Wilcox method in four classes including 16 % class C4-S2, 46 % class C4-S1, 30 % Class C3-S1, and 8 % in Class C2-S1. According to the results, the construction of the Saveh Dam has been the main reason of groundwater drops in the Saveh aquifer.
Previous investigations have also highlighted similar results to the current study. For instance, degradation of groundwater quality as a result of human activities is reported by Broers and Grift (2004), and also effects of rainfall and runoff variation on groundwater quantity are emphasized by Chen et al. (2002, 2004). It is worth noting that Zhang et al. (2012) indicate that both climate change and human activities are responsible for the decrease of observed runoff in the Huifa River. However, human activities contributed more to runoff decrease in wet years due to regulation and storage of the water projects. Also, Wang et al. (2013) found that human activities were the main driving factors for the decline in annual runoff in the Luanhe River catchment, Chaohe River catchment, and Zhanghe River catchment, accounting for over 50 % of runoff reduction. However, climate variability should be responsible for the decrease in annual runoff in the Hutuo River catchment. The results of the current paper correspond with the results reported by Zhang et al. (2012) and Wang et al. (2013). Also, Guo et al. (2014) found that the decline in annual runoff over both catchments can be mainly attributed to the human activities; the reduction percentages due to human activities range from 59 to 77 %. While the results of the current paper show lesser contribution of human activities in reduction of the annual runoff, about 39 %. Hu et al. (2014) showed that climate fluctuations accounted for a decrease in mean annual streamflow of approximately 10.38 mm/year (51.03 %), whereas human activities (including soil–water conservation measures, artificial water intakes, and man-made water storage infrastructure) caused a decrease of approximately 9.96 mm/year (48.97 %). The current paper results in comparison with Hu et al. (2014) indicate a greater contribution of climate change in reduction of mean annual streamflow in the study area.
Generally speaking, human activities played a dominant role on the variation of groundwater quality and quantity in contrast with climate variability in the Saveh plain. Similar results are reported by Ye et al. (2013) and Shanshan et al. (2012). However, it should be noted that results of this field depend on various factors such as climatic conditions of case study that should be considered in comparison of the results.
Conclusions
It is well documented and obvious that runoff not only is affected by anomaly rainfall but it also is affected by human interventions, such as withdrawals and surface water regulation. On the other hand, distinguishing between impacts of climate variability and human activities on runoff is complicated and controversial. Construction of the Saveh Dam, and following that, decreasing runoff at downstream of the dam, has been the main reason of the groundwater drops. The results show that the abrupt change point in annual rainfall in the Saveh plain occurred around 1980, 1985, and 1990. Based on the Mann-Kendall test, rainfall time series was divided into two parts, namely, 1966–1989 and 1990–2007, which indicate before and after change point. Also, results show that averages of annual rainfall in five stations increase from 10 to 21 %. Abrupt change point in annual runoff in the Saveh plain occurred around 1996. Based on the Mann-Kendall test, runoff time series was divided into two parts, namely, 1946–1995 and 1996–2007, which indicate before and after change point. Results show that averages of annual runoff in four stations decrease from 8 to 83 %. Trend and change point analysis of annual rainfall and runoff results show that annual rainfall in the studied stations increase from 10 % in Razin station to 33 % in Ahmadabad station. On the other hand, annual runoff decreased from 83 % in Shahabasi station to 5 % in Razin station. In order to analyze the impacts of the Saveh Dam on runoff, the operation year of the dam, 1994, was considered as a change point. The difference decreases of runoff between 42 % in Jalayer station and 81 % in Shahabasi station can be seen as a result of the Saveh Dam construction. Highest groundwater level decline, about 168.67 cm, occurred in 1994, which is the operation year of the Saveh Dam. In Ahmadabad and Emamabad stations, the highest correlation coefficients between SPI and groundwater fluctuations with 4- and 5-month time delays are equal to 0.812 and 0.762, respectively. Average surface water EC during 1966–1971, 1972–1991, and 1992–2004 are equal to 1863.17, 1379.77, and 2230.87 μmho/cm, respectively. According to the Wilcox method, the river water quality had degredated from C3-S1 to C4-S2. Average groundwater EC during 1999–2003 and 2004–2009 are equal to 3234.5 and 3324.1 μmho/cm, respectively. Groundwater quality for agricultural usages in the Saveh aquifer can be classified into four classes including: 16 % class C4-S2, 46 % class C4-S1, 30 % Class C3-S1, and 8 % in Class C2-S1.
References
Adger, N., & Coauthors. (2007). Summary for policy makers. Climate change 2007: impacts, adaptation and vulnerability. In M. L. Parry et al. (Eds.), Cambridge University Press, 7–22.
Ahmadi, S. H., & Sedghamiz, A. (2007). Geostatistical analysis of spatial and temporal variations of groundwater level. Environmental Monitoring and Assessment, 129, 277–294.
Almasri, M. N., & Kaluarachchi, J. J. (2004). Assessment and management of long-term nitrate pollution of groundwater in agriculture-dominated watersheds. Journal of Hydrology, 295, 225–245.
Bao, Z., Zhang, J., Wang, G., Fu, G., He, R., Jin, J., Liu, Y., Zhang, A., & Yan, X. (2012). Attribution for decreasing streamflow of the Haihe River basin, northern China: climate variability or human activities. Journal of Hydrology, 460–461, 117–129.
Barnett, J., Webber, M., Wang, M., Finlayson, B., & Dickinson, D. (2006). Ten key questions about the management of water in the yellow river basin. Environmental Management, 38, 179–188.
Bovolo, C. I., Parkin, G., & Sophocleous, M. (2009). Groundwater resources, climate and vulnerability. Environmental Research Letters, 4(3), 1–4.
Broers, H. P., & Grift, B. (2004). Regional monitoring of temporal changes in groundwater quality. Journal of Hydrology, 296, 192–220.
Brouyère, S., Carabin, G., & Dassargues, A. (2004). Climate change impacts on groundwater resources: modelled deficits in a chalky aquifer, Geer basin. Belgium Hydrogeology Journal, 12, 123–134.
Chen, Z., Grasby, S. E., & Osadetz, K. G. (2002). Predicting average annual groundwater levels from climatic variables: an empirical model. Journal of Hydrology, 260, 102–117.
Chen, Z., Grasby, S. E., & Osadetz, K. G. (2004). Relation between climate variability and groundwater levels in the upper carbonate aquifer, southern Manitoba, Canada. Journal of Hydrology, 290, 43–62.
Chen, Z., Chen, Y., & Li, B. (2013). Quantifying the effects of climate variability and human activities on runoff for Kaidu River Basin in arid region of northwest China. Theoretical and Applied Climatology, 111, 537–545.
Custodio, E. (2002). Aquifer overexploitation: what does it mean? Hydrogeology Journal, 10, 254–277.
Dams, J., Woldeamlak, S. T., & Batelaan, O. (2008). Predicting land-use change and its impact on the groundwater system of the KleineNete catchment, Belgium. Hydrology and Earth System Sciences, 12, 1369–1385.
Dragoni, W., Sukhija, B.S. (2008). Climate Change and groundwater: a short review. Geological Society Special Publication, 1–12.
Eltahir, E. A. B., & Yeh, P. J. F. (1999). On the asymmetric response of aquifer water level to floods and droughts in Illinois. Water Resource Research Journal, 35, 1199–1217.
Estrela, T., Marcuello, C., Iglesias, A., (1996). Water resources problems in Southern Europe. European Environmental Agency.
Green, T. R., Bates, B. C., Charles, S. P., & Fleming, P. M. (2007a). Physically based simulation of potential effects of carbon dioxide—altered climates on groundwater recharge. Vadose Zone Journal, 3, 597–609.
Green, T. R., Taniguchi, M., & Kooi, H. (2007b). Potential impacts of climate change and human activity on subsurface water resources. Vadose Zone Journal, 3, 531–532.
Guo, Y., Li, Z., Amo-Boateng, M., Deng, P., & Huang, P. (2014). Quantitative assessment of the impact of climate variability and human activities on runoff changes for the upper reaches of Weihe River. Stochastic Environmental Research and Risk Assessment, 28, 333–346.
Hennessy, K., Page, C., Durack, P., Bathols, J. (2006). Climate change projections for Victoria. CSIRO Marine and Atmospheric Research, Aspendale, Victoria 3195.
Holman, I. P. (2006). Climate change impacts on groundwater recharge-uncertainty, shortcomings, and the way forward. Hydrogeology Journal, 14, 637–647.
Hu, Z., Wang, L., Wang, Z., Hong, Y., & Zheng, H. (2014). Quantitative assessment of climate and human impacts on surface water resources in a typical semi-arid watershed in the middle reaches of the Yellow River from 1985 to 2006. International Journal of Climatology. doi:10.1002/joc.3965.
IPCC, et al. (2007). Climate change 2007: the physical science basis. In S. Solomon (Ed.), Contribution of working group I to the fourth assessment report of the intergovernmental panel on climate change (p. 996). Cambridge: Cambridge University Press.
Karl, & Trenberth, K. E. (2003). Modern global climate change. Science, 302, 1719–1723.
Kundzewicz, Z. W., Mata, L. J., Arnell, N. W., Doll, P., Kabat, P., Jimenez, B., Miller, K. A., Oki, T., Sen, Z., & Shiklomanov, I. A. (2007). Freshwater resources and their management. In M. L. Parry, O. F. Canziani, J. P. Palutikof, P. J. van der Linden, & C. E. Hanson (Eds.), Climate change 2007: impacts, adaptation and vulnerability (pp. 173–210). Cambridge: Cambridge University Press.
McCarthy, J. J., Canziani, O. F., Leary, N. A., Dokken, D. J., & White, K. S. (Eds.). (2001). Climate change 2001: impacts, adaptation, and vulnerability. Cambridge: Cambridge University Press.
McKee, T. B., Doesken, N. J., & Kleist, J. (1993). The relationship of drought frequency and duration to time scales, paper presented at 8th conference on applied climatology. Anaheim: American Meteorological Society.
McKee, T. B., Doesken, N. J., & Kleist, J. (1995). Drought monitoring with multiple time scales, paper presented at 9th conference on applied climatology. Dallas: American Meteorological Society.
Ministry of Energy of Iran, Iran Water Resource Management Company. (2005). Status evaluation of groundwater resources of Iran. Tehran, Iran (available in Persian).
Pearson, K. (1895). Mathematical contributions to the theory of evolution, II: skew variation in homogeneous material. Philosophical Transactions Royal Society Proceedings, 58, 241.
Scheidleder, A., Grath, J., Winkler, G., Stark, U., Koreimann, C., Gmeiner, C., Nixon, S., Casillas, J., Gravesen, P., Leonard, J., Elvira, M. (1999). Groundwater quality and quantity in Europe. European environment agency.
Scibek, J., & Allen, D. M. (2006). Comparing modelled responses of two highpermeability, unconfined aquifers to predicted climate change. Global and Planetary Change, 50, 50–62.
Shanshan, H. U., Changming, L., Hongxing, Z., Zhonggen, W., & Jingjie, Y. (2012). Assessing the impacts of climate variability and human activities on stream flow in the water source area of Baiyangdian Lake. Journal of Geographical Sciences, 22, 895–905.
Skinner, A. C. (2008). Groundwater: still out of sight but less out of mind. Quarterly Journal of Engineering Geology and Hydrogeology, 41, 5–19.
Sophocleous, M. A. (2004). Global and regional water availability and demand: prospects for the future. Natural Resources Research, 13, 61–75.
Taylor, C.J., Alley, W.M. (2001). Groundwater level monitoring and the importance of long-term water level data. U.S. Geological Survey, Circular 1217.
Van der Kamp, G., & Maathuis, H. (1991). Annual fluctuations of groundwater levels as a result of loading by surface moisture. Journal of Hydrology, 127, 137–152.
Wang, W., Shao, Q., Yang, T., Peng, S., Xing, W., Sun, F., & Luo, Y. (2013). Quantitative assessment of the impact of climate variability and human activities on runoff changes: a case study in four catchments of the Haihe River basin. China Hydrological Process, 27, 1158–1174.
Warner, S. D. (2007). Climate change, sustainability, and ground water remediation: the connection. Groundwater Monitoring and Remediation, 27, 50–52.
Wentz, L., Ricciardulli, K. H., & Mears, C. (2007). How much more rain will global warming bring? Science, 317, 233–235.
Wilcox, L. V. (1955). Classification and use of irrigation waters, United States salinity laboratory, circular no. 969 (p. 9). Washington, D. C.: USDA.
Woldeamlak, S. T., Batelaan, O., & De Smedt, F. (2007). Effects of climate change on the groundwater system in the Grote-Nete catchment. Belgium Hydrogeology Journal, 15, 891–901.
Wu, Y., & Chen, J. (2013). Investigating the effects of point source andnonpoint source pollution on the water quality of the East River (Dongjiang) in South China. Ecological Indicators, 32, 294–304.
Wu, Y., & Liu, S. (2012). Modeling of land use and reservoir effects on nonpoint source pollution in the Iowa River Basin. Journal of Environmental Monitoring, 14, 2350–2361.
Ye, X., Zhang, Q., Liu, J., Li, X., & Xu, C. (2013). Distinguishing the relative impacts of climate change and human activities on variation of stream flow in the Poyang Lake catchment. China Journal of Hydrology, 494, 83–95.
Zhang, A., Zhang, C., Fu, G., Wang, B., Bao, Z., & Zheng, H. (2012). Assessments of impacts of climate change and human activities on runoff with SWAT for the Huifa River Basin. Northeast China Water Resource Management, 26, 2199–2217.
Acknowledgments
This work has been supported by the University of Tehran. The authors are grateful to the university and also to the Iran Water Resources Management Company for providing required data.
Author information
Authors and Affiliations
Corresponding author
Rights and permissions
About this article
Cite this article
Mohammadi Ghaleni, M., Ebrahimi, K. Effects of human activities and climate variability on water resources in the Saveh plain, Iran. Environ Monit Assess 187, 35 (2015). https://doi.org/10.1007/s10661-014-4243-2
Received:
Accepted:
Published:
DOI: https://doi.org/10.1007/s10661-014-4243-2