Abstract
This case study uses several univariate and multivariate statistical techniques to evaluate and interpret a water quality data set obtained from the Klang River basin located within the state of Selangor and the Federal Territory of Kuala Lumpur, Malaysia. The river drains an area of 1,288 km2, from the steep mountain rainforests of the main Central Range along Peninsular Malaysia to the river mouth in Port Klang, into the Straits of Malacca. Water quality was monitored at 20 stations, nine of which are situated along the main river and 11 along six tributaries. Data was collected from 1997 to 2007 for seven parameters used to evaluate the status of the water quality, namely dissolved oxygen, biochemical oxygen demand, chemical oxygen demand, suspended solids, ammoniacal nitrogen, pH, and temperature. The data were first investigated using descriptive statistical tools, followed by two practical multivariate analyses that reduced the data dimensions for better interpretation. The analyses employed were factor analysis and principal component analysis, which explain 60 and 81.6 % of the total variation in the data, respectively. We found that the resulting latent variables from the factor analysis are interpretable and beneficial for describing the water quality in the Klang River. This study presents the usefulness of several statistical methods in evaluating and interpreting water quality data for the purpose of monitoring the effectiveness of water resource management. The results should provide more straightforward data interpretation as well as valuable insight for managers to conceive optimum action plans for controlling pollution in river water.
Similar content being viewed by others
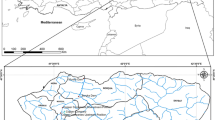
Explore related subjects
Discover the latest articles, news and stories from top researchers in related subjects.Avoid common mistakes on your manuscript.
Introduction
Rivers contribute significantly to the growth of a country’s economy. The benefits of rivers are not limited to the supply of water; they also serve other purposes such as recreation and sport, fishing, navigation, irrigation, generation of hydropower, transportation, waste disposal, and even sand mining. As such, riverside development inevitably impacts river water quality. River problems are normally related to water quality, flash floods, water shortage, sedimentation, and squatters. The main sources of organic water pollution are domestic and industrial sewage and effluent from palm oil mills, rubber factories, and animal husbandry (H. Juahir et al. 2011; Qadir et al. 2008). Mining operations, housing and road development, along with logging and forest clearing, are the major sources of highly concentrated suspended sediment in downstream river stretches. In urban and industrial areas, organic water pollution often results in environmental problems and adversely affects aquatic life as well. In addition to this, pollution loading from non-point sources, such as fertilizers from farming areas among others is usually difficult to estimate because it is a function of rainfall/storm water runoff. Nevertheless, it cannot be ignored due to the impact on environmental values and the intrinsic value of receiving waters.
The quality status of river water is evaluated by a water quality index (WQI), a single dimensional number distilled mathematically from large amounts of water quality data (Nives 1999). The WQI has a value between 0 and 100, with a higher index value representing better water quality (Cude 2001; Pandey and Sundaram 2002). The quality of river water can be evaluated either with individual parameters or a few, select, important parameters. Numerous countries utilize the WQI method to assess overall river status (Swaroop Bhargava 1983). The WQI normally consists of six parameters: biochemical oxygen demand (BOD), dissolved oxygen (DO), suspended solids (SS), chemical oxygen demand (COD), ammoniacal nitrogen (AN), and pH. Another parameter frequently taken into account is the river water temperature (TEMP) (Shuhaimi-Othman et al. 2007; Fulazzaky 2010). Similar measures are also used in Malaysia.
The collection of river water data in Malaysia is largely carried out by the Department of Irrigation and Drainage and the Department of Environment, Malaysia. The Klang River basin in central Peninsular Malaysia in particular has a total of 20 water quality monitoring stations set up along the main Klang River and 11 tributaries, including the Gombak River, Kerayong River, Penchala River, and Damansara River. It is noteworthy that the quality of water along the Klang River has been deteriorating with city expansion and various other industrial activities (Rahman 2007). The lack of awareness, enforcement, and informed strategies all contribute to the worsening of the water quality conditions in Malaysia (Ibarahim and Lee 2004). In 1976, the Klang River was one of 42 rivers categorized as badly polluted, while 16 others were categorized as mildly polluted. The status of the Klang River had not changed by 1990 when a similar study was conducted. According to the 2000 Malaysia Environmental Quality Report, the water of the Klang River was still polluted and raw water from this source necessitated extensive treatment. However, in 2007, 13 out of 20 monitoring stations showed improvement in water quality. There were mostly located at the tributaries and upper stream of the Klang River (Othman et al. 2012). Two of the stations are class II, meaning that the raw water requires standard treatment prior to consumption and is safe for recreational activities. The application of multivariate methods including factor analysis (FA) and principal component analysis (PCA) as statistical tools in the investigation of water quality data is widely found throughout the literature (Kazi et al. 2009; Koklu et al. 2009; Yidana and Yidana 2009; Vialle et al. 2011; Hafizan Juahir et al. 2009; Wang et al. 2013; Lapong et al. 2012; Mustapha et al. 2013; Nduka and Orisakwe 2011; Varol et al. 2013). Both FA and PCA are very powerful techniques whose main objective is to reduce the dimensions of a multivariate data set. This is accomplished by finding new variables in terms of linear combinations of the original variables that are able to retain most of the information present in the data and, more importantly, are interpretable.
Through various agencies, the government has responded to the various water quality findings. Studies on identifying the point and non-point sources of pollution in several rivers have been conducted, resulting in the implementation of different appropriate action plans at diverse locations (Ibarahim and Lee 2004). Such planning can be improved by understanding the variations of river water quality parameters. Hence, to assist the local environmental policy makers in preparing well-informed action plans, it is important to provide the profile of each monitoring station with detailed water quality parameter compositions. However, no studies have been carried out so far on determining the level of contamination contributed by different water quality parameters to the WQI. For this reason, we attempt in this study to evaluate the importance of water quality parameters and identify the potential factors contributing to the variations in the Klang River basin, Malaysia. The first objective of the paper is to describe each parameter considered in the data using appropriate descriptive statistics. In particular, the differences and similarities shared by the parameters of different stations are highlighted by looking at the mean and standard deviation and illustrating them in an error bar plot. The second objective is to analyze the data using multivariate statistical techniques with the focus on reducing the dimensions of data for simpler interpretation. To achieve this objective, factor analysis and principal component analysis are applied to the seven parameters considered in this study (Basilevsky 2009; Johnson and Wichern 2002; Jolliffe 2002). The results are expected to provide insight into the quality of the water and consequently enable managers to form appropriate action plans at the different locations along the river basin.
Material and methods
Study area
The Klang River basin is located within the state of Selangor and the Federal Territory of Kuala Lumpur, Malaysia. It is one of the basins undergoing considerable development and urbanization and is thus subjected to pollution from point and non-point sources. The catchment area is around 1288 km2. The river originates from the steep mountain rainforests of the main Central Range along Peninsular Malaysia and flows through the Federal Territory of Kuala Lumpur (Federal Territory), parts of Gombak, Hulu Langat, Klang, and Petaling districts in Selangor State, and the municipal areas of Ampang Jaya, Petaling Jaya, and Shah Alam culminating at the river mouth in Port Klang, the largest sea port in Malaysia. The Klang Gates and Batu Dams are two reservoirs located in the Klang River basin with capacities of 25,104 and 30,199 million liters, respectively. Two water treatment plants that take raw water from these dams produce a total of 256 million liters of treated water per day. The study area is considered a paradigm of rivers located in a humid, tropical area in Southeast Asia. The study area is depicted in Fig. 1.
Monitored parameters and analytical methods
Figure 2 illustrates the 20 selected monitoring station sites. They are labeled as St. 02–18, St. 23–24, and St. 26. Nine stations are located along the Klang River’s main stem, i.e. St. 02–10. St. 02 is located near the Strait of Malacca. Three stations are along the Damansara River, i.e. St. 11–13, and there is one station each for the Pencala, Rekah, and Ampang Rivers, i.e. St. 14, 15, and 23, respectively. St. 16 and 26 are found along the Kerayong River, while St. 17, 18, and 24 are situated along the Gombak River. Data on the six parameters that constitute the WQI formula were collected from 1997 onwards. Othman et al. (2012) showed that for these stations, the WQI indicates positive improvement starting in the 1998–2005 interval and persisting until 2007. Hence, data from 2005 to 2007 will be used for analysis in this paper. An additional parameter measuring the temperature (TEMP) of river water is also considered.
BOD (biochemical oxygen demand) is defined as the amount of oxygen required by aerobic microorganisms to dissolve organic matter in a sample of water. It is one of the most essential and widely used parameters for measuring pollutants and biodegradable organic compounds in water (Kwok et al. 2005; Simon et al. 2011). The BOD is also indicative of the total DO (defined below) concentration necessary for the oxidation and degradation of some organic matter. DO is defined as the amount of oxygen dissolved in a water body and measures the health of the water and its ability to support a balanced aquatic ecosystem (Snyder 2007). The DO appears as microscopic bubbles of gaseous oxygen which are mixed in water and available to aquatic organisms for respiration (Ji 2008). Total suspended solids (TSS) are the solid matter suspended in water, comprising of organic and inorganic materials, such as plankton, silt, and industrial waste (Ell 2008). The COD is the amount of specified oxidant that reacts with a sample of water under controlled conditions and is expressed in terms of its oxygen equivalence (Camlab 2009). COD is viewed as a useful measure of water quality because its application determines the amount of organic pollutants present in surface water or wastewater (Viessman and Hammer 2005). AN is a measure of the amount of ammonia or toxic pollutants which are usually found in waste products and landfill leachate, such as liquid manure, sewage, and other liquid organic waste products (Mirmohseni and Oladegaragoze 2003).
The pH of surface water is specified for the protection of fish life and the control of undesirable chemical reactions. The pH of any water body surface is defined as a measure of hydrogen ion concentration. In other words, pH is a measure of the alkalinity or acidity of water soluble substances (Covington et al. 1985).
Data treatment and statistical techniques
Different univariate and multivariate techniques can be used to analyze the Klang River water quality data set. It is common practice to initially visualize the data by using a graphical tool, or more specifically in this study, an error bar plot. Further investigation may then be carried out using multivariate techniques, including principal component analysis (PCA) and factor analysis (FA). These techniques are very useful when the objective is to reduce the dimensions of the data in order to enhance the quality of analysis interpretation. All mathematical and statistical computations were done using SPlus 8.1 (TIBCO Spotfire S+® 8.1 2008) software.
Descriptive statistical tool
An error bar plot displays a range of errors around plotted data points. In our case, the middle point of the vertical line represents the mean value with one standard error on each side. More precisely, the part of the error bar above each point (mean value) represents plus one standard error and the section below represents minus one standard error. The error bar is basically a description of the degree of confidence that the mean represents the true parameter value. The longer the error bar, the less confidence we feel about in that particular value. If the error bar of one station overlaps significantly that for another station, there is a much lower likelihood that the values from these two stations differ significantly.
Principal component analysis (PCA)
Investigations involving a large number of observed variables can often be simplified by considering a smaller number of linear combinations of the original variables. With a standardized linear combination (SLC), it is possible to make meaningful comparisons between various choices of linear combinations. PCA finds a set of SLCs, called the principal components (PCs), which taken together can explain all the variance of the original data. PCs are uncorrelated (orthogonal) variables obtained by multiplying the original correlated variables with a list of coefficients (loadings or weightings). There are generally as many PCs as variables. However, because of the way they are calculated, it is usually possible to consider only a few of them which together explain most of the original variation. PCA therefore imparts information on the most important parameters, explaining the whole data set while permitting data reduction with the least amount of loss of original information; it is a potent method for pattern detection that attempts to explain the variance of a huge set of inter-correlated variables and convert them into a smaller set of independent (uncorrelated) variables (PCs) (Singh et al. 2004). Here, PCA is performed on a data correlation matrix, such that the structure of the underlying data set can be described. The correlation coefficient matrix determines how well the variance of each element can be explained by each relationship with each of the others (Liu et al. 2003; Singh et al. 2004).
Factor analysis (FA)
In many scientific fields, quantities that are not directly measurable are frequently of interest. Nonetheless, it is often possible to measure other quantities which reflect an underlying variable of significance. Factor analysis (FA), therefore, is an attempt to explain the correlations between observable variables in terms of underlying factors, which are themselves not directly observable. At first glance, FA closely resembles PCA. Both use linear combinations of variables to explain sets of observations of many variables. In PCA, the observed variables are the quantities of interest. The combination of these variables in the PCs is primarily a tool for simplifying the interpretation of the observed variables. In FA, by contrast, the observed variables are of relatively little intrinsic interest, whereas the underlying factors are the quantities of interest and become subjected to the varimax rotation.
Results and discussion
Descriptive statistical tool
The basic statistics of the 3-year (2005–2007) data set on the Klang River water quality are plotted in the error bar plots in Fig. 3a–g. According to Fig. 3a, the DO levels for St. 10 and 24 are among the highest with means greater than 7 mgL−1. In contrast, Fig. 3b indicates that the BOD readings at these two stations are among the lowest with very small variation of values. Similar behavior is also observed for the COD and AN readings in Fig. 3c, f, respectively. As for temperature, that at St. 24 is the lowest, with the value at St. 10 a close second. These are indicators of good river water quality in these areas. The stations are situated at the most upstream points of the Gombak and Klang Rivers respectively and are surrounded mainly by tropical forest and agricultural as well as animal farming regions (Fig. 4). On the other hand, the DO level for St. 02–05 downstream of the main river, St. 14 at Penchala River, and St. 26 upstream of the Kerayong River are almost the same, having some of the lowest mean values (less than 3 mgL−1). However, the BOD and COD readings are much higher for St. 14 and 26. Figure 4 shows that these stations are situated in urban areas of the Klang Valley and are exposed to different point sources including domestic and industrial sewage. Meanwhile, the mean readings for SS and pH are generally similar for all stations, as portrayed by the overlapping error bar plots for these two variables (Fig. 3d–e). The stable SS readings are possibly due to the fact that this is an already developed urban area. St. 04 shows much larger variations for the SS readings compared to the other stations, and a similar behavior is observed for the COD readings. Such observations may be the result of an outlying observation at St. 04, something that will be elaborated on in Section 3.2. No extreme behavior with respect to the seven variables is noted for the remaining stations situated upstream of the Klang River and those along the tributaries (Damansara and Rekah Rivers).
Multivariate statistical techniques
PCA was applied to the multivariate data consisting of seven parameters as described in Section 2.2. The intention is to identify the principal components (PCs) which are linear combinations of the original parameters and are fewer in number and more interpretable. A scree plot serves to identify the suitable number of PCs to be preserved in order to envision the underlying data structure (Singh et al. 2004) as per Fig. 5. Clearly, the bend is noticeable after the fourth PC, for which reason the first four PCs are considered as they explain 81.6 % of the data variation. Table 1 provides the loadings (or coefficients) of the PCs. The first PC, or PC1, contains a large negative loading on DO and positive loading on the BOD, COD, SS, AN, and temperature. In other words, PC1 shows the inverse relationship between DO and the five other variables, with smaller PC1 values indicating better river water quality. Consequently, we can label PC1 as the overall index for the quality of water at a particular point along the Klang River. Such labeling is unfortunately not feasible for the other three PCs because no clear pattern can be identified from their loadings—it is not possible to identify the relationship between DO and the rest of the variables for these cases. As a result, the FA method has to be used to reduce the data dimensions.
FA was applied to the data to identify new latent factors that influence each other. Based on the formula s = 0.5(p − k)2 − 0.5(p + k), where p is the number of parameters and k is the number of latent factors considered, we look for a value of k yielding the smallest value s > 0. For the present data, three underlying factors seem to be sufficient to explain roughly 60 % of the total variance.
The corresponding factor loadings are given in Table 2. Factor 1, which explains 24.3 % of the total variance, has strong positive loading on COD and moderate positive loading on BOD and SS, which can be interpreted as the index of pollution in river water. A higher value for factor 1 indicates worse water quality at a particular point along the river. On the other hand, factor 2 explains 19.4 % of the total variance and has moderate positive loading on AN and temperature and moderate negative loadings on DO. This factor represents the contrast between good quality and bad quality river water parameters, similar to PC1 described previously. Hence, factor 2 can be regarded as the index of water quality. Again, since the loading on DO is negative, a smaller value of factor 2 indicates better quality. Factor 3 explains 15.4 % of the total variance and is dominated by a strong positive loading on pH. It thus represents the level of river water acidity.
The relation of the factors to both the original variables and original data can additionally be seen in biplots (Gabriel 1971). Biplots are a generalization of the simple two-variable scatterplot. Here, the original variables are represented by arrows according to the loadings under the new latent factors. The arrow length indicates the size of the standard deviation of the original variables, while the angle between the arrows indicates the correlation between the variables. The individuals are displayed as points signifying their respective values with regard to the new latent factors. This is very useful in identifying clusters or outlying individuals.
The biplots of the three latent factors are given in Fig. 6a–c. When the first two factors are considered as in Fig. 6a, the standard deviation of COD is slightly larger than that of SS, BOD, AN, TEMP, and DO while the standard deviation of pH is nearly 0. This is true since the loading for pH is almost zero in factors 1 and 2. In addition, COD is positively correlated with BOD, TEMP, and AN but negatively correlated with SS and DO. Further, no significant separation of data points is observed. Most points are centered around the origin of the biplot, but a single point, number 263, lies far from the cluster. The observation shows a large positive deviation in SS and negative deviation in DO suggesting that the station is more heavily polluted at this particular time point. The observation is a reading at St. 04 on 15 March 2007, with the following parameter values: DO = 1.81 mg/l, BOD = 50 mg/l, COD = 387 mg/l, SS = 5550 mg/l, pH = 7.71, AN = 2.81 mg/l, and TEMP = 27.72 °C. The reading for DO is well below the overall mean value of the variable with DOmean = 4.46, but the readings for the rest are considerably higher than the corresponding overall means, namely, BODmean = 10.04, CODmean = 21.39, SSmean = 110.64, ANmean = 4.13, and TEMPmean = 28.09. These values are indicative of the significant deterioration of the water quality at this specific point in time. From the other two plots b and c in Fig. 6, as expected, the standard deviation of pH is the largest due to the dominant role of pH in factor 3. Again, time point 263 is remote from the rest (Fig. 6b) while the others are more scattered (Fig. 6c).
Figure 7 illustrates the error bar plots of the predicted factor values for every station. It is obvious that with the exception of a few stations, the means of the three factors are mostly close to zero, which explains the data clusters centered at the origins of the biplots in Fig. 6. Note that factor 1 refers to the index of pollution—a higher predicted value means more pollution. As anticipated, St. 04, 14, and 26 with smaller DO values but larger COD and BOD values stand out as the most polluted stations (Fig. 7a). In fact, the values of factor 1 for St. 04 fluctuate the most and contribute to the occurrence of outlying observation number 263 previously described. On the other hand, factor 2 is labeled as the index of water quality such that the water quality is better for a smaller predicted factor value. According to Fig. 7b, the river water at St. 10 and 24 is of better quality compared to that at other stations. As for factor 3, which denotes the river water acidity level, the results are in accord with the initial findings that there is not much difference in the acidity of river water among the stations.
Conclusion
To design a water quality monitoring program, we should first understand the patterns and variations of water quality parameters observed at the monitoring stations. This task is made easier by replacing the large number of parameters by a smaller number of so-called factors that are able to summarize the variation in the original data. In our study, based on the seven main environmental parameters for water quality, we are able to identify three main factors which explain the pollution, quality, and acidity level at 20 monitoring stations in the Klang River basin. These findings will help managers to easily interpret data and hence assist them to make better decisions regarding action plans. For example, it is noted that the water quality at St. 04 and 14 is originally identified to be similar to St. 02 and 03; the factor analysis nevertheless suggests that after taking into account the contribution of each variable, St. 04 and 14 are actually worse. It is also significant to note that St. 10 and 24 seem to have good water quality as per the better DO readings (factor 2) compared to the other stations but have similar pollution index readings (factor 1). St. 10 is situated near the Klang Gates Dam and St. 24 is in the vicinity of recreational areas. It is clear from Fig. 4 that development is gradually approaching the regions next to those involving traditional farming and agricultural activities. For this reason, appropriate plans of action should urgently be implemented at these two rivers due to their importance in providing clean raw water and clean recreational areas to Klang Valley residents. Observations also reveal that although St. 26 is situated upstream of the Kerayong River, it is identified as the most polluted station according to higher pollution index values (factor 1) and a worse water quality index (factor 2).
Again, Fig. 4 indicates that the areas surrounding the station have experienced an abundance of new industrial and residential development in recent years and the results suggest that proper planning to control the pollution sources is immediately required. In general, this study points out the importance of understanding the variations observed in river water environmental parameters in order to adequately plan and ensure that the quality of the water does not continue to deteriorate. Such studies should be periodically conducted on each of the 189 river basins in Malaysia owing to the diversity of land usage in their surroundings. The commitment shown by the government in allocating significant amounts of funding to control river water pollution in the past years should be complemented by efficient action plans following proper studies with fine statistical analysis practice.
References
Basilevsky, A.T. (2009). Statistical factor analysis and related methods: theory and applications: Wiley.
COD or Chemical Oxygen Demand definition (2009). The Laboratory People. http://camblab.info/wp/index.php/272/. Accessed 2013.
Covington, A. K., Whalley, P. D., & Davison, W. (1985). Recommendations for the determination of pH in low ionic strength fresh waters. Pure and Applied Chemistry, 57(6), 877–886.
Cude, C. G. (2001). Oregon water quality index a tool for evaluating water quality management effectiveness. JAWRA Journal of the American Water Resources Association, 37(1), 125–137. doi:10.1111/j.1752-1688.2001.tb05480.x.
Ell, M.J. (2008). Total suspended solids (TSS). In N. D. D. O. Health (Ed.), North Dakotan, USA.
Fulazzaky, M. A. (2010). Water quality evaluation system to assess the status and the suitability of the Citarum river water to different uses. Environmental Monitoring and Assessment, 168(1–4), 669–684. doi:10.1007/s10661-009-1142-z.
Gabriel, K. R. (1971). The biplot graphic display of matrices with application to principal component analysis. Biometrika, 58(3), 453–467. doi:10.1093/biomet/58.3.453.
Ibarahim, R., & Lee, C. M. (2004). Pollution prevention and river water quality improvement programme. Buletin Ingenieur, 23, 6–7.
Ji, Z.G. (2008). Hydrodynamics and water Quality: modeling rivers, lakes, and estuaries: Wiley-Interscience.
Johnson, R.A., & Wichern, D.W. (2002). Applied multivariate statistical analysis. (5th ed., pp. 767): Prentice Hall.
Jolliffe, I. T. (2002). Principal component analysis. New York, USA: Springer.
Juahir, H., Zain, S. M., Aris, A. Z., Yusoff, M. K., & Mokhtar, M. B. (2009). Spatial assessment of Langat River water quality using chemometrics. Journal of Environmental Monitoring, 12(1), 287–295.
Juahir, H., Zain, S. M., Yusoff, M. K., Hanidza, T. I., Armi, A. S., Toriman, M. E., et al. (2011). Spatial water quality assessment of Langat River basin (Malaysia) using environmetric techniques. Environmental Monitoring and Assessment, 173(1–4), 625–641. doi:10.1007/s10661-010-1411-x.
Kazi, T. G., Arain, M. B., Jamali, M. K., Jalbani, N., Afridi, H. I., Sarfraz, R. A., et al. (2009). Assessment of water quality of polluted lake using multivariate statistical techniques: a case study. Ecotoxicology and Environmental Safety, 72(2), 301–309. doi:10.1016/j.ecoenv.2008.02.024.
Koklu, R., Sengorur, B., & Topal, B. (2009). Water quality assessment using multivariate statistical methods—a case study: Melen River system (Turkey). Water Resources Management, 24(5), 959–978. doi:10.1007/s11269-009-9481-7.
Kwok, N.-Y., Dong, S., Lo, W., & Wong, K.-Y. (2005). An optical biosensor for multi-sample determination of biochemical oxygen demand (BOD). Sensors and Actuators B: Chemical, 110(2), 289–298. doi:10.1016/j.snb.2005.02.007.
Lapong, E., Fujihara, M., Izumi, T., Kobayashi, N., Kakihara, T., Hamagami, K. (2012). Water quality index and statistical analysis methods for water quality characterization in agricultural rivers. Paper presented at the Water Quality 2012, Hangzhou, China, 19–21 September
Liu, C.-W., Lin, K.-H., & Kuo, Y.-M. (2003). Application of factor analysis in the assessment of groundwater quality in a blackfoot disease area in Taiwan. Science of the Total Environment, 313(1–3), 77–89. doi:10.1016/s0048-9697(02)00683-6.
Mirmohseni, A., & Oladegaragoze, A. (2003). Construction of a sensor for determination of ammonia and aliphatic amines using polyvinylpyrrolidone coated quartz crystal microbalance. Sensors and Actuators B-Chemical, 89(1–2), 164–172. doi:10.1016/S0925-4005(02)00459-8.
Mustapha, A., Aris, A. Z., Juahir, H., Ramli, M. F., & Kura, N. U. (2013). River water quality assessment using environmentric techniques: case study of Jakara River basin. Environmental Science and Pollution Research International, 20(8), 5630–5644. doi:10.1007/s11356-013-1542-z.
Nduka, J. K., & Orisakwe, O. E. (2011). Water-quality issues in the Niger Delta of Nigeria: a look at heavy metal levels and some physicochemical properties. Environmental Science and Pollution Research International, 18(2), 237–246. doi:10.1007/s11356-010-0366-3.
Nives, S.-G. (1999). Water quality evaluation by index in Dalmatia. Water Research, 33(16), 3423–3440.
Othman, F., Alaa-Eldin, M. E., & Mohamed, I. (2012). Trend analysis of a tropical urban river water quality in Malaysia. Journal of Environmental Monitoring, 14(12), 3164–3173. doi:10.1039/C2EM30676J.
Pandey, M., & Sundaram, S. M. (2002). Trend of water quality of river Ganga at Varanasi using WQI approach. International Journal of Ecology and Environmental Science of the Total Environment, 28, 139–142.
Qadir, A., Malik, R. N., & Husain, S. Z. (2008). Spatio-temporal variations in water quality of Nullah Aik-tributary of the river Chenab, Pakistan. Environmental Monitoring and Assessment, 140(1–3), 43–59. doi:10.1007/s10661-007-9846-4.
Rahman, H. A. (2007). A survey on a river pollution in Malaysia. In Geographic Conference, USPI, Malaysia.
Shuhaimi-Othman, M., Lim, E. C., & Mushrifah, I. (2007). Water quality changes in Chini Lake, Pahang, West Malaysia. Environmental Monitoring and Assessment, 131(1–3), 279–292. doi:10.1007/s10661-006-9475-3.
Simon, F. X., Penru, Y., Guastalli, A. R., Llorens, J., & Baig, S. (2011). Improvement of the analysis of the biochemical oxygen demand (BOD) of Mediterranean seawater by seeding control. Talanta, 85(1), 527–532. doi:10.1016/j.talanta.2011.04.032.
Singh, K. P., Malik, A., Mohan, D., & Sinha, S. (2004). Multivariate statistical techniques for the evaluation of spatial and temporal variations in water quality of Gomti River (India)—a case study. Water Research, 38(18), 3980–3992. doi:10.1016/j.watres.2004.06.011.
Snyder, J. (2007). Dissolved Oxygen. http://www.seagrant.sunysb.edu/oli/Water%20Quality/DissolvedOxygen.html2013.
Swaroop Bhargava, D. (1983). Use of water quality index for river classification and zoning of Ganga River. Environmental Pollution Series B, Chemical and Physical, 6(1), 51–67.
TIBCO Spotfire S+® 8.1 (2008). Guide to Statistics. (Vol. 1, pp. 718): TIBCO Software Inc.
Varol, M., Gokot, B., & Bekleyen, A. (2013). Dissolved heavy metals in the Tigris River (Turkey): spatial and temporal variations. Environmental Science and Pollution Research International, 20(9), 6096–6108. doi:10.1007/s11356-013-1627-8.
Vialle, C., Sablayrolles, C., Lovera, M., Jacob, S., Huau, M. C., & Montrejaud-Vignoles, M. (2011). Monitoring of water quality from roof runoff: interpretation using multivariate analysis. Water Research, 45(12), 3765–3775. doi:10.1016/j.watres.2011.04.029.
Viessman, W., & Hammer, M. J. (2005). Water supply and pollution control (7th edn.). The University of Michigan. USA: Pearson Prentice Hall.
Wang, Y., Wang, P., Bai, Y., Tian, Z., Li, J., Shao, X., et al. (2013). Assessment of surface water quality via multivariate statistical techniques: a case study of the Songhua river Harbin region, china. Journal of Hydro-Environment Research, 7(1), 30–40. doi:10.1016/j.jher.2012.10.003.
Yidana, S. M., & Yidana, A. (2009). Assessing water quality using water quality index and multivariate analysis. Environmental Earth Sciences, 59(7), 1461–1473. doi:10.1007/s12665-009-0132-3.
Acknowledgments
We would like to express our thanks to the Department of Irrigation and Drainage and the Department of Environment, Malaysia for their cooperation when we conducted this study. This research is financially supported by the Research Grant (No: RG244-12AFR, RP009C-13AFR, FL001-13SUS) from the University of Malaya. We are most grateful and would like to thank the reviewers for their valuable suggestions, which led to a substantial improvement of the article.
Author information
Authors and Affiliations
Corresponding author
Rights and permissions
About this article
Cite this article
Mohamed, I., Othman, F., Ibrahim, A.I.N. et al. Assessment of water quality parameters using multivariate analysis for Klang River basin, Malaysia. Environ Monit Assess 187, 4182 (2015). https://doi.org/10.1007/s10661-014-4182-y
Received:
Accepted:
Published:
DOI: https://doi.org/10.1007/s10661-014-4182-y