Abstract
Brown eye spot is one of the most common and important diseases of coffee in Brazil. Using geostatistics, this study evaluated the spatio-temporal aspects of the disease and how they relate to plant nutrition and soil fertility in plots irrigated by center pivot and drip irrigation. The experiments were conducted in Carmo do Rio Claro City, in southern Minas Gerais state, southeast Brazil. The sampling grid was georeferenced with 50 points in 17 ha and 52 points in 11 ha for center pivot and drip irrigation, respectively. Disease incidence was assessed at 60-day intervals from August 2012 to March 2015. Yield, plant mineral nutrition, and soil fertility were evaluated at each point annually. Weather data were obtained from climatological stations with sensors for temperature, relative humidity, wind velocity, leaf wetness duration, and total precipitation that were located inside and outside the coffee canopy in both experimental plots. Climate data were correlated with disease incidence. Disease progress curves were plotted, and semivariogram models were fitted for assessments with a high disease incidence over time. Then, the data were interpolated by ordinary kriging, and maps of disease, yield, and leaf nutrients (B, P, and K) were constructed. The average temperatures and accumulated rainfall rates were lower in periods of higher incidence, but relative humidity was high. All variables exhibited space-time variation in both plots. There was a correlation (p < 0.01) between disease incidence and leaf nutrients (B, K and P). The disease incidence in the center pivot and drip irrigation plots ranged from 0 to 23% and 0–25%, respectively, and varied over space, showing spatial dependence and the presence of disease foci with outward gradients. Areas with high intensity changed over planting years along with yield and nutrient availability.
Similar content being viewed by others
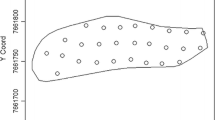
Explore related subjects
Discover the latest articles, news and stories from top researchers in related subjects.Avoid common mistakes on your manuscript.
Introduction
Coffee is an important commodity, and is consumed in several countries. Brazil is the world’s largest producer and exporter of Arabica coffee (Coffea arabica L.) and of coffee in general. Brazil’s coffee production has increased significantly in recent decades, reaching 50.32 million 60 kg coffee green bags in 2016 (FAOSTAT 2018). Approximately 25% of all production comes from irrigated groves, which make up only 10% of the total planted area. Irrigation and fertigation can significantly increase production by supplying water and nutrients to plants. The use of drip and sprinkler irrigation systems in coffee crops has grown in recent years, mainly due to climate change in many coffee growing regions in Brazil (Pozza and Alves 2008). While irrigation has advantages, it can influence the intensity of some diseases (Huber and Gillespie 1992), including Cercospora leaf spot also known as brown eye spot (BES). BES is caused by Cercospora coffeicola Berk. & Cooke: it occurs in all production regions and is associated with quantitative and qualitative losses to plants, from nursery to production (Pozza et al. 2010; Lima et al. 2012).
Water supplied by irrigation, especially sprinkler systems, modifies crop microclimates by changing the environment, extending the period of leaf wetness, increasing the relative humidity of the air and reducing canopy temperature, and thus fostering the germination of fungi and fungal infection processes, increasing the rate of disease progress (Rotem and Palti 1969). Drip systems have the advantages of saving water and energy and contributing to a reduction in disease intensity because they do not increase the duration of leaf wetness (Subbarao et al. 1997; Xiao and Subbarao 2000; Xiao et al. 1998). However, disease intensity does not vary only with water supply; several other factors are involved, such as the expression of disease resistance genes, yield, plant nutrition and soil fertility, which can change over time and space (Alves et al. 2009).
An adequate nutrient supply makes it possible for plants to form resistance barriers (Datnoff et al. 2007), such as a waxy cuticles or cell walls, thus reducing disease intensity (Taiz and Zeiger 2013). Studies have demonstrated the interaction of K, N and Ca in a nutrient solution with BES disease intensity (Pozza et al. 2001; Garcia Junior et al. 2003). Additionally, Belan et al. (2015) reported the movement of Ca in and K out of lesions caused by necrotrophic pathogens of coffee, such as Phoma spp. and C. coffeicola. Although the relationship between plant nutrition and disease intensity over space and time has been studied in controlled environments, few studies exist that define this relationship in a field environment. Information about disease distribution and its spatial dependence on nutrients and microclimate factors along the planting area can inform disease management practices, allowing specialized fertilization and spraying systems and specific planting designs. Thus, this management practice will improve the environmental sustainability.
Geostatistics and spatio-temporal analysis are tools that have been used in the study of the spatial dependence between variables of interest in coffee growing, aiding management and decision-making, from single plots to large field areas. In a non-irrigated coffee plantation, Alves et al. (2009) found spatial variation in BES and rust, depending on their spread from outbreak centres. The authors found a negative correlation between disease and the levels of leaf-S and Ca and Mg in the soil, but a positive correlation between disease and phosphate, N, and K levels shown in ordinary kriging maps. Such knowledge can help farmers to efficiently manage disease with a proper balance of nutrients and a consequent reduction in fungicide application. Furthermore, Musoli et al. (2008) and Pinard et al. (2016) studied the spatial distribution of coffee wilt disease (Fusarium Xylarioides) and Mouen Bedimo et al. (2007) evaluated the spatio-temporal dynamics of coffee berry disease.
This study evaluated the relationship between the spatio-temporal progress of BES and soil fertility and mineral nutrition in coffee plant crops grown with center pivot or drip irrigation.
Material and methods
Location of experimental plots and georeferencing
The studies were conducted at two farms in Carmo do Rio Claro City, south Minas Gerais state, southeast Brazil. The coffee plantations with center pivot and drip irrigation systems are located at latitudes 20°59′55″ and 46°02′52” S and longitudes 21°00′28″ and 46°01′30” W, respectively; both are at 850 m elevation and lack shade or intercrops. The assay was evaluated from August 2012 to March 2015. This time interval was necessary due to the biennial nature of coffee production, i.e., high yields alternate with low yields year by year (Pereira et al. 2011; Sakyiama 2015), which is a physiological characteristic of coffee plants.
The plot irrigated by center pivot system was 17 ha of coffee (Coffea arabica L.) cultivar Acaiá 474/19, which is susceptible to BES. Ten-year-old plants were at a density of 4.0 × 0.5/ha (equivalent to 5000 plants), a slope of 10% and had an average output of 30 60-kg coffee green bags/ha from 2009 to 2012. Irrigation by center pivot started on June 2nd, 2012, with 30 mm applied every 10 days, watered to a monthly minimum depth of 90 mm, as monitored by a rain gauge.
The study plot irrigated by a drip system had 11 ha of coffee (Coffea arabica L.) cultivar Acaiá 474/19. Eighteen-month-old plants were spaced at 3.6 m between rows and 0.7 m between plants, totalling 3968 plants/ha, at a slope of 7%. Production started in 2013, during the experiment, and crops were irrigated throughout the year, based on readings from groups of tensiometers. Both experimental plots were not irrigated during harvest, from May to June.
Sample grids in both plots were georeferenced with a GPS TRIMBLE 4600 LS® and a Leica TC600 Total Station®. The sample grids for the center pivot and drip irrigation plots comprised 50 and 52 sample points spaced 50 × 50 m and 40 × 40 m, respectively (Fig. 1).
Areas with georeferenced sampling points (yellow) (a) plot irrigated by center pivot (left) (b), and plot irrigated by drip irrigation system (right) (c). Relief map with UTM coordinates and height of center pivot plot (d) and drip irrigation plot (e) in Carmo do Rio Claro City, Minas Gerais state (http://earth.google.com)
During the experiment, when necessary, leaf miner and berry borer were chemically controlled, weeds were controlled by mowing. The coffee crops were fertilized according to the recommendations for managing soil fertility in the Minas Gerais state, Brazil, as proposed by Guimarães et al. (1999).
Assessment of incidence of brown eye spot over time
Five plants were assessed at each georeferenced point: three on the row, and one on each side of the central tree, perpendicular to the row. At each sample point and time, 12 leaves from each of five plants were assessed non-destructively. The BES incidence on the leaves was assessed evenly across the middle third of the canopy on arbitrarily selected branches, from the third or fourth pair of leaves in a branch chosen at random (Huerta 1963), totaling 60 leaves at each sample point per evaluation date. Disease assessments occurred at 60-day intervals from August 2012 to March 2015, for 16 assessments in all. Incidence (I) was calculated according to the following equation:
Where:
- I:
-
incidence (%)
- NFD:
-
number of diseased leaves in the five plants at each point;
- NFT:
-
total number of leaves per georeferenced point (60 leaves).
The disease progress curves were plotted over time using the average incidence data from all sampling points during the 32 evaluation months.
Yield
The coffee berries from five plants at each georeferenced sampling point were collected manually in May of 2013 and 2014. The average yield was calculated for five plants at 50 and 52 points in the center pivot and drip irrigation plots, respectively. These data were transformed into units of 60-kg coffee green bags/ha by multiplying the average production of each point by the amount of plants in 1 ha.
Nutritional analysis of plants and soil fertility
To determine foliar nutrient content for all points, sampling was performed in October 2012, 2013, and 2014. Five leaves were collected from the five plants on each side of the planting line, in an east-west direction, for each sampling point. Sampled leaves were from the third or fourth pair of leaves, counted from the end of the plagiotropic branch, in the middle third of the plant. Sampling for soil analysis occurred in the same period. A compound soil sample was collected at each sampling point on the grid. This sample was composed of five soil subsamples collected from the canopy projection area of the plants at each georeferenced point, mixed in a bucket. The samples were sent to the laboratory to determine the levels of leaf and soil nutrients according to the method proposed by Malavolta et al. (1997) and Guimarães et al. (1999).
The leaf contents of each of the following nutrients were determined: N, P, K, Ca, Mg, S, Zn, Fe, Mg, Cu and B. Soil analysis measured hydrogen potential (pH), K, P, Na, Ca, Mg, Al, potential acidity (H + Al), the sum of bases (SB), effective cation exchange capacity (t), cation exchange capacity at pH 7.0 (T), percentage of base saturation (V), percentage of aluminium saturation (m), organic matter (OM), remaining phosphorus (P-rem), Zn, Fe, Mn, Cu, B, and S.
Weather variables
Weather data from outside and inside the canopy in both experimental plots were measured. Outside data were collected with a microclimate weather station (Campbell Scientific®) installed in the experimental plot to monitor maximum (Tmax), average (Tmean) and minimum temperatures (Tmin); maximum (Umax), average (Umean) and minimum relative humidities (Umin), wind velocity (WV), leaf wetness duration (LWD), and total precipitation (Prec.). Two temperature data loggers, an HT-500 Instrutherm® and a WatchDog® 1000 Series Micro Station, with temperature sensors and two leaf wetness sensors, were installed in both the center pivot and drip irrigation fields. The sensors were placed in the middle third interior of the canopy or distributed at random in the plants. The data loggers and microclimate stations were installed for obtaining repetitive measurements of variables inside the canopy. Thus, we obtained the monthly averages (from all devices) of temperatures (Tmean) and leaf wetness durations (LWD) from the WatchDog® micro station sensors inside the canopies.
The monthly cumulative rainfall and the monthly averages for all other climatic variables collected in the external stations were plotted, along with the incidence progress curves.
Correlations
Disease incidence was correlated with the external and internal canopy climate variables. The incidence of BES was averaged for every georeferenced point in both plots over the 30 day period before each disease assessment date, due to the latent period of C. coffeicola and the epidemiological curve of BES (Custódio et al. 2014).
Levels of leaf and soil nutrients were also correlated with the dates of higher disease incidence. Then, only those leaf and soil nutrients with a significant correlation with disease incidence were selected for geostatistical analysis.
The PROC CORR procedure of SAS v.9.3® statistical software (SAS Institute) was used to perform Pearson correlation analysis.
Geostatistical and spatial dependence analysis
Dates with high incidences of BES were selected for geostatistical analysis. The nutrients with the most significant correlations with disease incidence in both plots were also selected for geostatistical analysis. This analysis was not performed for soil fertility due to the absence of a significant correlation with disease incidence in both plots. Geostatistical analyses were carried out for both plots for 2013 and 2014 production, due to significant correlations with disease. The data were determined to be normally distributed, according to the frequency distribution histogram of the analysed variables.
Spatial dependence was analysed using semivariograms fitted based on the assumption of intrinsic stationarity, according to Burrough and McDonnell (1998). The spatial dependence level (SDL) of the best-fit model, or the nugget effect (Co) in relation to the level (Co + C), where C is the contribution or spatially dependent component, was calculated according to the following equation:
According to Cambardella et al. (1994), spatial dependence is strong when the SDL is lower than 25%, moderate with an SDL ranging from 26 to 75%, and weak with an SDL over 75%.
The semivariogram was adjusted to an exponential model for BES, in accordance with Alves et al. (2009). After fitting the semivariograms, the data interpolation by ordinary kriging was performed. Then, maps were constructed to visualize the spatial distribution patterns of the variables in crops at different evaluation times. ArcGIS 9.3 software (Environmental Systems Research Institute - ESRI) was used to adjust the semivariograms and plot the ordinary Kriging maps.
Results
Progress curves of brown eye spot
Average incidence of BES in the center pivot and drip irrigation plots ranged from 0 to 23% and 0–25%, respectively, from August 2012 to March 2015. Maximum incidences in the center pivot plot were 23% on August 24, 2013 and 21% on August 18, 2014 (Fig. 2a). The highest incidence in the drip irrigation plot (25%) occurred on June 4, 2014 (Fig. 2a). The lowest average temperatures and lowest cumulative rainfall also occurred in these periods; however, relative humidity was over 80% due to irrigation (Fig. 2b and c).
Incidence progress curves of brown eye spot of coffee in center pivot and drip irrigation plots (a), monthly averages of weather variables: maximum (Tmax), mean (Tmean), and minimum temperature (Tmin) (b), average relative humidity (UR) and accumulated precipitation (Prec) (c), collected outside the canopy from August 15, 2012 to March 14, 2015
Correlation between incidence of brown eye spot and weather data
The center pivot plot had a negative correlation between incidence and temperature and leaf wetness duration, both inside and outside the canopy, and between incidence and accumulated precipitation outside the canopy (Table 1). Incidence was negatively correlated with temperature and positively correlated with leaf wetness duration inside the canopy in the drip irrigation plot. The correlation between disease incidence and minimum temperature was also negative outside the canopy (Table 1).
Center pivot
Correlation between incidence and leaf and soil nutrients
Soil nutrients and disease incidence were not significantly correlated. There was a significant correlation between leaf nutrients (B, P and K) and the maximum disease incidences on April 17, 2013, August 24, 2013, June 4, 2014, and August 18, 2014. Due to this correlation, these nutrients were chosen for geostatistical analysis. B sampled in 2012 showed a negative correlation with disease incidence on April 17, 2013 (−0.43*), but a positive correlation for sample dates April, 17, 2013 (0.31*), and August 08, 2013 (0.50*); the correlation was again negative between B sampled in 2014 and disease incidence on sample dates April 17, 2013 (−0.29*), and August 24, 2013 (0.60*). The correlation between P leaf content and disease incidence was significant only for the leaves sampled in 2013, showing a negative correlation on August 24, 2013 (−0.51*). The correlation between K leaf content (for the 2012 leaf samples) and disease incidence was positive on August 24, 2013 (0.32*), and negative (for the 2013 leaf samples) on August 24, 2013 (−0.51).
Spatial distribution of brown eye spot, yield and mineral nutrition of plants
According to the geostatistical analysis, the intensity of disease varied throughout the sampled plot on the maximum incidence dates. An exponential model was fitted for maximum incidence dates, yield and leaf contents of B, P, and K (Table 2).
A defined focus of disease was found in the southern area of the plot in most of the incidence graphs (Fig. 3a - d). While the spatial distribution of incidence varied over time (Fig. 3a, b, c, and d), the highest incidences occurred in or near the same areas for each evaluation period.
Variations in yield occurred over space and time. The areas with the highest yields alternated between the 2 years: the southeast area produced up to 5744.68 kg/ha or close to 95.8 60 kg coffee green bags in 2013, while the northwest area produced up to 2363.91 kg/ha in 2014 (Fig. 4a and b). The spatial dependence of yield was strong in both years.
The areas with high yield before harvest had incidence rates as low as 6.1% in the evaluation performed on April 17, 2013, whereas post-harvest rates on August 24, 2013 increased dramatically, reaching up to 23%. The areas with low incidence of disease clearly produced higher yields in 2014, especially compared to the evaluation performed on August 18, 2014, when incidence was as high as 21%.
Furthermore, the kriging maps showed variation in the distributions of B, P, and K along the sampled plot. In 2012, B was concentrated in the north part of the plot (Fig. 5a), whereas in 2013, it was concentrated in the southeast (Fig. 5b), reaching levels within the critical range for the crop (40 to 100 mg/kg; Martinez et al. 2003). In 2014, B was also concentrated at the northwest part of the plot but was within the critical range for the crop throughout the plot (Fig. 5c). There was no variation in spatial dependence in sampling in 2012, 2013, and 2014 as all samples showed strong spatial dependence, with a range value of 391.18 m (Table 2).
For P in 2012 and 2014, levels were within the critical range for the crop (1.2 to 2.0 g/kg; Martinez et al. 2003), as shown in Fig. 5d and f, and in 2013, levels were within the critical range in the north part of the plot (Fig. 5e). Leaf content sampling in 2012, 2013, and 2014 showed moderate, strong, and weak spatial dependence, respectively, with values ranging from 188.26 to 391.18 m (Table 2).
In 2012, it was possible to observe K concentrated in some parts of the plot (Fig. 5g), but for this year, the nutrient was below the critical range for the crop (approximately 19 to 26 g/kg; Martinez et al. 2003). By the year 2013, the highest concentration was at the north (Fig. 5h) and reached critical levels for the crop. In the year 2014, the nutrient was distributed homogeneously and was within the critical range for the crop throughout the plot (Fig. 5i). Spatial dependence was moderate, strong, and weak in 2012, 2013, and 2014, respectively, with values ranging from 178.30 to 391.18 m (Table 2).
Drip irrigation
Correlation between incidence and nutrients of leaf and soil
Soil nutrients had no significant correlation with the incidence of disease. Leaf nutrients, B, P, and K, had a significant correlation with the dates of maximum incidences. B content showed a significant correlation in the 2013 leaf samples only. The correlation between nutrients and disease incidence was positive on April 17, 2013 (0.27*), and was negative for leaf P content between the 2012 samples and the incidence on June 4, 2014 (−0.27*). 2014 P samples and disease incidences were positively correlated on August 24, 2013 (0.30*), and June 4, 2014 (0.53*). The correlation between 2012 sampled K leaf content and disease incidence was positive on August 24, 2013 (0.35*), and positive between 2014 samples and disease incidences on April 17, 2013 (0.34*), and June 4, 2014 (0.28*).
Spatial distribution of brown eye spot, yield and mineral nutrition of plants
According to geostatistical analysis, the intensity of disease varied throughout the sampled plot on the dates of maximum incidence. The exponential model was fitted for maximum incidence, yield, and the leaf contents of B, P, and K (Table 3).
Incidence assessments on April 17, 2013, June 4, 2014, and August 18, 2014 showed weak spatial dependence, whereas on August 24, 2013, spatial dependence was strong, with range values from 135.09 to 438.43 m (Table 3). While incidence distribution varied across space and sample dates, the areas with higher disease intensities were the same, or close to the disease foci, for both periods (Fig. 6a to d).
Yield also varied throughout the plot in both harvest years, but the areas with higher yields alternated: the southeast grid area yielded 5065.53 kg/ha (84.4 60 kg coffee green bags) in 2013, whereas the northwest produced 5234.38 kg/ha (87.3 60-kg coffee green bags) in 2014 (Fig. 7a and b). Both years showed variation in spatial dependence, which was weak in 2013 and moderate for the 2014 yield, with range values from 160.78 to 457.80 m.
For B in 2012, concentration levels were within the critical range (40 to 100 mg/kg; Martinez et al. 2003) for the crop in the east part of the plot only (Fig. 8a). In 2013 and 2014 (Fig. 8b and c), B was distributed homogeneously and was within the critical range for the crop throughout the plot. B leaf content in 2012, 2013, and 2014 showed moderate, weak, and strong spatial dependence, respectively, with values ranging from 310.41 to 457.80 m (Table 3).
For P in 2012, (Fig. 8d) the nutrient levels were below the critical range in most of the plot, which for this nutrient, is 1.2 to 2.0 g/kg (Martinez et al. 2003). In 2013 and 2014, the concentration of P increased and was close to and/or within the critical range in most of the plot, with a homogenous distribution (Fig. 8e and f). The variation in the spatial dependence of P was strong, weak and moderate, in the years 2012, 2013 and 2014, respectively, with range values reaching 457.80 m (Table 3).
For K in 2012, the nutrient distribution in the plot varied (Fig. 8g), with all regions being within the critical range (19 to 26 g/kg; Martinez et al. 2003). In 2013, K was below the recommended levels for the crop in most of the plot (Fig. 8h), but in some parts, the concentration was close to the critical range. In 2014, the concentration of K was close to or within the critical range in the entire area (Fig. 8i). Samples of K leaf content in 2012 and 2014 showed moderate spatial dependence, but spatial dependence was weak in 2013, with range values from 350.28 to 457.80 m (Table 3).
Discussion
The temporal progression of BES varied during the period assessed in both irrigation systems. The highest incidences in the center pivot plot occurred at the same time in both years, August 2013 and August 2014. These results were similar to those of Custódio et al. (2014), who found higher incidence from May to July while studying the epidemiology of this disease with different water application levels in center pivot systems. In the drip irrigation plot, the highest incidences of disease occurred in April and August 2013 and June and August 2014. However, Talamini et al. (2003) and Santos et al. (2004) found the highest incidence of disease from May to June in drip irrigation systems in the south of Minas Gerais state, southern Brazil. Thus, there is a variation in the periods of higher incidence of this disease. This variation depends on the climatic variables, such as temperature, rain, number of sun hours, coffee yield and nutrient balance.
The highest disease incidences coincided with the periods of low temperature and rainfall, when the beginning of the harvest requires that there is no irrigation or fertilization (Sakyiama 2015). BES has a high correlation with nutritional imbalance, particularly for N, Ca and K (Pozza et al. 2000, 2001; Belan et al. 2015). According to Santos et al. (2008), high disease incidence can be observed during the fruiting season, when coffee plants are prone to nutritional imbalances caused by a drain on leaf nutrients for grain filling, which makes plants more susceptible to BES.
Spatial variability in plant yields observed in both irrigation systems over time is common in the production systems of Brazil’s coffee farms due to high plant densities, variations in soil fertility, texture and structure, total sun exposure and the physiological characteristics of coffee cultivars (DaMatta et al. 2007); even so, its correlation with BES has not yet been explored. Non-productive plants commonly grow alongside productive plants in coffee crops, which can also influence bearing tendencies, resulting in large fluctuations in yield year by year (Carvalho et al. 2004). This variability can directly affect the disease’s occurrence, as leaf nutrient uptake increases due to heavy loads. In addition, insufficient or unbalanced mineral nutrition affects disease intensity, which is higher in malnourished plants (Pozza et al. 2010). The yield maps clearly show this alternation in yields in both irrigation systems, with the highest production in 2013 in the east and the lowest production in the northwest grid area, with the opposite occurring in 2014.
Other studies also reported spatial variations in coffee yield. Sanchez et al. (2005) assessed the spatial variability of soil chemical characteristics and coffee productivity in different geomorphic surfaces. The authors found spatial dependence for all chemical attributes and yield. While mapping coffee productivity and its correlation with soil fertility in two areas in the São Paulo state, Molin et al. (2002) reported low spatial dependence between productivity and soil fertility. Thus, the spatial dependence of productivity depends on variables such as the biennial nature of coffee plants, variation in soil structure and texture, and soil fertility.
The exponential semivariogram model for all variables was fitted with the model used by Alves et al. (2009) for BES in coffee crops in Ijaci City, Minas Gerais state, Brazil, in non-irrigated crops. This same model was also fitted for two banana diseases associated with cercospora fungi, yellow and black Sigatoka. For Coffee Berry Disease (CBD), Mouen Bedimo et al. (2007) adjusted Gaussian, spherical or exponential models depending on the sampled site and the berries’ age in weeks. Freitas et al. (2015) fitted different models for assessing the relationship between yellow Sigatoka and soil fertility and mineral nutrition, in which the exponential model was the best fit for most variables. The exponential model was also the best fit for plant nutrition and soil fertility over space (Silva and Chaves 2001). This model has frequently been fitted with coffee diseases in Brazilian conditions.
Using kriging maps for BES, similar behaviour in both irrigation systems was observed. Higher intensities of disease in 2013, after the harvest, occurred at the east of the plot, which also showed higher yields and B content, whereas in 2014, low production and low levels of B were observed in the same plot. This can be explained by plant nutrition: the highest incidence and severity of BES was reported in plants with nutrient imbalances or deficiencies (Garcia Junior et al. 2003; Pozza et al. 2000). Furthermore, in areas with high yields, nutritional imbalances can be responsible for high disease incidence.
Nutrient imbalances can increase disease intensity and cause biochemical and structural changes in host defence mechanisms (Balardin et al. 2006; Develash and Sugha 1997; Rodrigues et al. 2003). This situation can occur after the harvest, due to the rarity of rain in coffee production areas between April and September. Water is necessary to transport nutrients to the leaves to produce resistance barriers. BES is one coffee disease with a major relation to nutrient balance. Pozza et al. (2000) analysed the mineral nutrition of coffee seedlings in a nutrient solution and its effect on the progress of BES. The authors found increased disease severity and defoliation when N doses were reduced and K doses were increased, which interfered with other nutrients such as B and P. According to these authors, reduced leaf Ca favoured the pathogen. Similarly, Garcia Junior et al. (2003) found a significant interaction between K and Ca in a nutrient solution in the area under the progress curve of BES of coffee, demonstrating the influence of nutrition on the intensity of disease. Belan et al. (2015) also observed the movement of K and Ca outside and inside BES lesions. Pozza et al. (2001) reported that BES was more likely to occur in crops with unbalanced nutritional statuses and in higher yield years, as described above. Therefore, nutritional balance can be as important as the level of a specific element, thus emphasizing the importance of adequate conditions of soil fertility and nutrient supply to plants for disease control (Taiz and Zeiger 2013).
Alves et al. (2009) reported a range of 46 to 57 m for BES in dryland areas, while this study found a range of up to 457.80 m. This variable is the distance containing spatially correlated samples, and its measurement is important in experimental planning and evaluation, as it can help define the sampling procedure (McBratney and Webster 1983). The higher range found in the current study can be explained by the greater nutritional imbalance produced by high yields in coffee areas with irrigated systems. Thus, farmers should use this tool to correct both soil fertility and plant nutrition, seeking to reduce BES intensity, even while producing large yields.
The research on precision agriculture in coffee has been implemented in Brazil, mainly due to the highly technical nature of the cultivation of many crops and the high economic value added (Silva et al. 2008). However, the research on precision farming as it relates to the space-time variability of crops is still limited, which hinders its practical use in farming. The levels of nutrients in both the soil and the leaves should be monitored throughout the crop cycle, as leaf content variation across the area could directly interfere with yield and disease intensity, requiring the replacement of plants at specific points. Due to spatial variation over time, cultural practices should fit the irrigation system. Thus, inputs such as fertilizers and fungicides can be distributed evenly and in smaller amounts for the real needs of each crop, thereby increasing the environmental, financial, and social sustainability of the entire coffee production chain.
References
Alves, M. C., Silva, F. M., Pozza, E. A., & Oliveira, M. S. (2009). Modeling spatial variability and pattern of rust and brown eye spot in coffee agroecosystem. Journal of Pest Science, 82, 137–148. https://doi.org/10.1007/s10340-008-0232-y.
Balardin, R. S., Dallagnol, L. J., Didoné, H. T., & Navarini, L. (2006). Influência do fósforo e do potássio na severidade da ferrugem-da-soja Phakopsora pachyrhizi. Fitopatologia Brasileira, 31(5), 462–467.
Belan, L. L., Pozza, E. A., Freitas, M. L. O., Pozza, A. A. A., Abreu, M. S., & Alves, E. (2015). Nutrients distribution in diseased coffee leaf tissue. Australasian Plant Pathology, 44, 105–111.
Burrough, P. A., & McDonnell, R. A. (1998). Principles of geographical information systems. Oxford: University Press.
Cambardella, C. A., Moorman, T. B., Novak, J. M., Parkin, T. B., Karlen, D. L., Turco, R. F., & Konopka, A. E. (1994). Field scale variability of soil properties in Central Iowa soils. Soil Science Society of America Journal, 58(5), 1501–1511.
Carvalho, L. G., Sediyama, G. C., Cecon, P. R., & Alves, H. M. R. (2004). A regression model to predict coffee productivity in southern Minas Gerais, Brazil. Revista Brasileira de Engenharia Agrícola e Ambiental, 8(2), 204–211.
Custódio, A. A. P., Pozza, E. A., Custódio, A. A. P., Souza, P. E., Lima, L. A., & Silva, A. M. (2014). Effect of center-pivot irrigation in the rust and brown eye spot of coffee. Plant Disease, 98(7), 943–947.
DaMatta, F. M., Ronchi, C. P., Maestri, M., & Barros, R. S. (2007). Ecophysiology of coffee growth and production. Brazilian Journal of Plant Physiology, 19(4), 485–510.
Datnoff, L. E., Elmer, W. H., & Huber, D. M. (2007). Mineral nutrition and plant disease. St. Paul: APS Press.
Develash, R. K., & Sugha, S. K. (1997). Factors affecting development of downy mildew (Peronospora destructor) of onion (Allium cepa). Indian Journal of Agricultural Sciences, 67(2), 71–74.
FAOSTAT http://www.fao.org/faostat/en/#data/QC Brazilian Coffee green production, 2018.
Freitas, A. S., Pozza, E. A., Alves, M. C., Coelho, G., Rocha, H. S., & Pozza, A. A. A. (2015). Spatial distribution of yellow Sigatoka leaf spot correlated with soil fertility and plant nutrition. Precision Agriculture, 17(1), 93–107.
Garcia Junior, D., Pozza, E. A., Pozza, A. A. A., Souza, P. E., Carvalho, J. G., & Balieiro, A. C. (2003). Incidência e severidade da cercosporiose do cafeeiro em função do suprimento de potássio e cálcio em solução nutritiva. Fitopatologia Brasileira, 28(3), 286–291.
Guimarães, P. T. G., Garcia, A. W. R., Alvarez, V. H. A., Prezotti, L. C., Viana, A. S., Miguel, A. E., Malavolta, E., Corrêa, J. B., Lopes, A. S., Nogueira, F. D., Monteiro, A. V. C., Oliveira, J. A. Cafeeiro. (1999). Cafeeiro. In: Ribeiro, A. C., Guimarães, P. T. G., Alvarez, V. H. A. (Eds.), Recomendações para o uso de corretivos e fertilizantes em Minas Gerais (pp. 289-302). 5 ª Aproximação. Viçosa: Comissão de Fertilidade do Solo do Estado de Minas Gerais (CFSEMG).
Huber, L., & Gillespie, T. J. (1992). Modeling leaf wetness in relation to plant disease epidemiology (Vol. 30, pp. 553–577).
Huerta, S. A. (1963). Par de folhas representativo del estado nutricional del cafeto. Cenicafé, 14(2), 11–127.
Lima, L. M., Pozza, E. A., & Santos, F. S. (2012). Relationship between incidence of Brown eye spot of coffee cherries and the chemical composition of coffee beans. Journal of Phytopathology, 160, 209–211.
Malavolta, E., Vitti, G. C., Oliveira, S. A. (1997). Avaliação do estado nutricional das plantas: princípios e aplicações. 2.ed. Piracicaba: Potafos.
Martinez, H. E. P., Menezes, J. F. S., Souza, R. B., Venegas, V. H. A., & Guimarães, P. T. G. (2003). Faixas críticas de concentrações de nutrientes e avaliação do estado nutricional de cafeeiros em quatro regiões de Minas Gerais. Pesquisa Agropecuária Brasileira, 38(6), 703–713.
Mcbratney, A. B., & Webster, R. (1983). How many observations are needed for regional estimation of soil properties? Soil Science, 135(3), 177–183.
Molin, J. P., Ribeiro Filho, A. C., Torres, F. P., Shiraisi, L. E., Sartori, S., & Sarriés, G. A. (2002). Mapeamento da produtividade de café e sua correlação com componentes de fertilidade do solo em duas áreas pilotos. In L. A. Balastreire (Ed.), Avanços na agricultura de precisão no Brasil no período de 1999–2001 (pp. 58–65). Piracicaba: Potafos.
Mouen Bedimo, J. A., Bieysse, D., & Nottéghem, J. L. (2007). Spatio-temporal dynamics of arabica coffee berry disease caused by Colletotrichum Kahawae on a plot scale. Plant Disease, 91, 1229–1236.
Musoli, C. P., Pinard, F., Charrier, A., Kangire, A., Hoopen, G. M., Kabole, C., Owgang, J., Bieysse, D., & Cilas, C. (2008). Spatial and temporal analysis of coffee wilt disease caused by Fusarium xylarioides in Coffea canephora. European Journal of Plant Pathology, 122(4), 451–460.
Pereira, S. P., Bartholo, G. F., Baliza, D. P., Sobreira, F. M., & Guimarães, R. J. (2011). Crescimento, produtividade e bienalidade do cafeeiro em função do espaçamento de cultivo. Pesquisa Agropecuária Brasileira, 46(2), 152–160.
Pinard, F., Makune, S. E., Campagne, P., & Mwangi, J. (2016). Spatial distribution of coffee wilt disease under roguing and replanting conditions: A case study from Kawery estate in Uganda. Plant Disease, 106(11), 2016.
Pozza, E. A., & Alves, M. C. (2008). Impacto potencial de mudanças climáticas sobre as doenças fúngicas do cafeeiro no Brasil. In R. Ghini & E. Ramada (Eds.), Mudanças climáticas: impactos sobre doenças de plantas no Brasil (pp. 220–238). Brasília: Embrapa.
Pozza, A. A. A., Martinez, H. E. P., Pozza, E. A., Caixeta, S. L., & Zambolim, L. (2000). Intensidade da mancha de olho pardo em mudas de cafeeiro em função de doses de N e de K em solução nutritiva. Summa Phytopathologica, 26(1), 29–33.
Pozza, A. A. A., Martinez, H. E. P., Caixeta, S. L., Cardoso, A. A., Zambolim, L., & Pozza, E. A. (2001). Influência da nutrição mineral na intensidade da mancha-de-olho-pardo em mudas de cafeeiro. Pesquisa Agropecuária Brasileira, 36(1), 53–60.
Pozza, E. A., Carvalho, V. L., & Chalfoun, S. M. (2010). Sintomas de injúrias causadas por doenças do cafeeiro (pp. 67-106). In R. J. Guimarães, A. N. G. Mendes, & D. P. Baliza (Eds.), Semiologia do cafeeiro. Lavras: Editora UFLA.
Rodrigues, F. A., Benhamou, N., Datnoff, L. E., Jones, J. B., & Bélanger, R. R. (2003). Ultrastuctural and cytochemical aspects of silicon-mediated rice blast resistance. Phytopathology, 93(5), 535–546.
Rotem, J., & Palti, J. (1969). Irrigation and plant diseases. Annual Review of Phytopathology, 7(9), 267–288.
Sakyiama, N. (2015). Café arábica do plantio à colheita. Viçosa: Editora UFV.
Sanchez, R. B., Marques Júnior, J., Pereira, G. T., & Souza, Z. M. (2005). Variabilidade espacial de propriedades de latossolo e da produção de café em diferentes superfícies geomórficas. Revista Brasileira de Engenharia Agrícola e Ambiental, 9(4), 489–495.
Santos, F. D. S., Souza, P. E., & Pozza, E. A. (2004). Epidemiologia da cercosporiose em cafeeiro (Coffea arabica L.) fertirrigado. Summa Phytopathologica, 30(1), 31–37.
Santos, F. D. S., Souza, P. E. D., Pozza, E. A., Miranda, J. C., Carvalho, E. A., Fernandes, L. H. M., & Pozza, A. A. A. (2008). Adubação orgânica, nutrição e progresso de cercosporiose e ferrugem-do-cafeeiro. Pesquisa Agropecuária Brasileira, 43(7), 783–791.
Silva, P. C. M., & Chaves, L. H. G. (2001). Avaliação e variabilidade espacial de fósforo, potássio e matéria orgânica em Alissolos. Revista Brasileira de Engenharia Agrícola e Ambiental, 5(3), 431–436.
Silva, F. M., Souza, Z. M., Figueiredo, C. A. P., Vieira, L. H. S., & Oliveira, E. (2008). Variabilidade espacial de atributos químicos e produtividade da cultura do café em duas safras agrícolas. Ciência e Agrotecnologia, 32(1), 231–241.
Subbarao, K. V., Hubbard, J. C., & Schulbach, K. F. (1997). Comparison of lettuce diseases and yield under subsurface drip and furrow irrigation. Phytopathology, 87(8), 877–883.
Taiz, L., & Zeiger, E. (2013). Fisiologia Vegetal.3 ed. Porto Alegre: Artmed.
Talamini, V., Pozza, E. A., Souza, P. E., & Silva, A. M. (2003). Progresso da ferrugem e da cercosporiose em cafeeiro (Coffea arabica L.) com diferentes épocas de início e parcelamentos da fertirrigação. Ciência e Agrotecnologia, 27(1), 141–149.
Xiao, C. L., & Subbarao, K. V. (2000). Effects of irrigation and Verticillium dahliae on cauliflower root and shoot growth dynamics. Phytopathology, 90(9), 995–1004.
Xiao, C. L., Subbarao, K. V., Schulbach, K. F., & Koice, S. T. (1998). Effects of crop rotation and irrigation on Verticillium dahliae microsclerotia in soil and wilt in cauliflower. Phytopathology, 88(10), 1046–1055.
Acknowledgements
The authors would like to thank the Conselho Nacional de Desenvolvimento Científico e Tecnológico (CNPq), Fundação de Amparo a Pesquisa do Estado de Minas Gerais (FAPEMIG) and Coordenação de Aperfeiçoamento de Pessoal de Nível Superior (CAPES) for financial support.
Author information
Authors and Affiliations
Corresponding author
Ethics declarations
Conflict of interest
Disclosure of potential conflicts of interest.
Ethical approval
This article does not contain any studies with human participants performed by any of the authors.
Rights and permissions
About this article
Cite this article
da Silva, M.G., Pozza, E.A., Chaves, E. et al. Spatio-temporal aspects of brown eye spot and nutrients in irrigated coffee. Eur J Plant Pathol 153, 931–946 (2019). https://doi.org/10.1007/s10658-018-01611-z
Accepted:
Published:
Issue Date:
DOI: https://doi.org/10.1007/s10658-018-01611-z