Abstract
The purpose of this study was to develop the multiple regression models to evaluate the formation of trihalomethanes (THMs) and haloacetonitriles (HANs) during chlorination of source water with low specific ultraviolet absorbance (SUVA) in Yangtze River Delta, China. The results showed that the regression models of THMs exhibited good accuracy and precision, and 86–97 % of the calculated values fell within ±25 % of the measured values. While the HANs models showed relatively weak evaluation ability, as only 75–83 % of the calculated values were within ±25 % of the measured values. The organic matter [dissolved organic carbon (DOC) or UV absorbance at 254 nm] and bromide exerted the most important influence on the formation of HANs. While for THMs, besides the organic matter and bromide, reaction time was also a key factor. Comparing the models for total THMs (T-THMs) in this study with others revealed that the regression models from the low SUVA waters may have low DOC coefficients, but high bromide coefficients as compared with those from the high SUVA waters.
Similar content being viewed by others
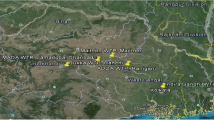
Explore related subjects
Discover the latest articles, news and stories from top researchers in related subjects.Avoid common mistakes on your manuscript.
Introduction
Chlorine is widely used in disinfection of drinking water because of its high efficiency in eliminating water borne pathogens, low cost and simple operation. However, chlorine reacts with the dissolved organic matter (DOM) in source water, leading to the formation of disinfection by-products (DBPs) (Richardson et al. 2007; Guilherme and Rodriguez 2015; Kim et al. 2015). To date, hundreds of DBPs have been identified in drinking water, among which the carbonaceous DBPs [e.g., trihalomethanes (THMs), haloacetic acids (HAAs)] are strictly regulated by many agencies due to their high levels occurred in chlorinated water and the potential health risks to human (Richardson et al. 2007; Salas et al. 2013). The concentrations of unregulated nitrogenous DBPs [e.g., haloacetonitriles (HANs)] have been reported to be much lower than THMs and HAAs in drinking water (Krasner et al. 2006; Chen and Westerhoff 2010; Xue et al. 2014), yet the cytotoxicity and genotoxicity posed by HANs may be similar or even higher as compared to that posed by THMs and HAAs (Muellner et al. 2007; Richardson et al. 2007). Accordingly, the nitrogenous DBPs have been receiving increasing concerns and attentions in recent years.
The DOM is regarded as the precursor for DBPs formation during chlorination. The dissolved organic carbon (DOC), dissolved organic nitrogen (DON) and UV absorbance at 254 (UV254) are commonly used to quantify the DOM level in source water (Nikolaoua and Lekkasa 2001; Pifer and Fairey 2014). While the specific UV absorbance at 254 nm (SUVA254), which is calculated by UV254 times 100 divided by DOC, is widely used to characterize the aromaticity of the DOM (Liang and Singer 2003). Generally speaking, the higher the DOM level, the higher the DBPs yields (Xie 2004). Moreover, properties of DOM also exert great influence on the formation of DBPs. The DOM with high SUVA254 may produce high yields of THMs and HAAs per unit carbon as compared to those with low SUVA254 (Uyak and Toroz 2007; Hong et al. 2009). Conversely, the DOM with high nitrogen content may contribute more to the formation of nitrogen DBPs (Nikolaoua and Lekkasa 2001; Fang et al. 2010; Hong et al. 2015). Besides the DOM level and its properties, other water quality parameters (e.g., bromide, pH) as well as the disinfection conditions (e.g., chlorine dose, reaction time, temperature) may also pose complex effects on DBPs formation (Hong et al. 2013b; Kim et al. 2015).
To better understand the relationships between the DBPs formation and the factors (including water quality and disinfection conditions), a series of regression models have been developed (Chowdhury et al. 2009; Chen and Westerhoff 2010; Abdullah and Hussona 2013). These models could be an effective way to identify the key factors influencing the DBPs formation and be a good alternative for evaluating DBPs formation in practice, which could alleviate the laborious work and reduce the expensive cost during DBP analysis (Hong et al. 2007; Chowdhury et al. 2009). Thus, the models have a wide range of applications in the design and management of drinking water supply system, in toxicological and epidemiological risk assessment studies and in risk-cost trade-off analyses (Chowdhury et al. 2009).
However, the models in available references mostly focused on THMs, HAAs, with very little attention to nitrogen DBPs, e.g., HANs (Chowdhury et al. 2009; Chu et al. 2012; Mukundan and Van Dreason 2014). According to the toxicological studies, the common HANs species such as dichloroacetonitrile (DCAN), bromochloroacetonitrile (BCAN) and dibromoacetonitrile (DBAN) are genotoxic and potentially carcinogenic to human (Bull and Robinson 1985; Daniel et al. 1986). Developing models on HANs is fundamentally important. On the other hand, due to the different source water quality and diverse disinfection conditions, DBPs formation may vary extensively. The site-specific regression models may over-predict or under-predict the DBPs formation (Sohn et al. 2004; Chowdhury et al. 2009). For example, Yoon et al. found that the predicted THMs levels using USA model were much higher than the measured levels for the source water in Korean (Yoon et al. 2003). Therefore, for a specific area, it is important to develop its own models to evaluate the DBPs formation and identify the key factors.
Tai Lake, Qiantang River and Jinlan Reservoir are three important source drinking waters in Yangtze River Delta, China (Hong et al. 2013b; Hong et al. 2015). Jinlan Reservoir is located in a remote area with limited human activity. The water in the reservoir had low DOC level (1.3 mg L−1) and low SUVA254 value (1.3 L mg−1m−1) (Hong et al. 2013b). Qiantang River, especially the downstream, received a great deal of human activity. Theoretically, originating from the degradation of higher plant tissues from the watershed and having high SUVA254, the allochthonous DOM should be the important composition of DOM for a typical river (Wetzel 2001; Hong et al. 2008). Yet due to the impacts from the sanitary, industrial and agricultural effluent, the water in Qiantang River contained high level of DOC (5.96 mg L−1) but with low SUVA value (0.794 L mg−1m−1) (Dong et al. 2012; Hong et al. 2015). Tai Lake is super-eutrophic and algal bloom occurred every year in recent decade. The DOM in Tai Lake was very high (DOC = 10.3 mg L−1) and also had low SUVA value (0.52 L mg−1m−1) (Hong et al. 2015). With the fast development of economy and improvement of living standards, DBPs formation in this area is increasingly becoming a public concern. However, except for a few studies on DBPs formation (Hong et al. 2013a, b; Hong et al. 2015), there is no available report related with THMs and HANs models for these waters. Moreover, to our knowledge, there are no available models exclusively for the water with low SUVA values. Therefore, generation of DBPs models using these waters may provide new knowledge to DBPs formation.
To address our objectives, a series of chlorination experiments were carried out using the source water collected from Tai Lake, Qiantang River and Jinlan Reservoir. Multiple regression models were generated for evaluating the formation of THMs and HANs, and the key factors were identified as well. In addition, the regression models developed in this study (DOM with low SUVA) were also compared with those from the reference (DOM with high SUVA).
Materials and methods
Field sampling and the water quality
The detailed information about the sampling site and the water quality have been described in our previously studies (Hong et al. 2013b; Hong et al. 2015) and were presented in Table 1. Tai Lake had highest organic level (DOC, DON and UV254), followed by Qiantang River and Jinlan Reservoir.
What worth concerning was that the bromide level in Tai Lake (248 µg L−1) was much higher than that in Qiantang River (73 µg L−1). This may be because (1) to alleviate the low water level in winter and the algal bloom occurred in summer, Tai Lake drew water from Yangtze River every year, which contained high bromide level due to the in burst of salt tide from estuaries (You et al. 2012). While for Qiantang River, the saltwater intrusions reduced to some extent due to the effective measures taken by the local government; (2) lake is a relative steady and independent system, the pollutants are difficult to be removed once they are introduced; yet river is a mobile system, and the contaminants can be diffused or diluted quickly.
Database description
The data of THMs (including the trichloromethane (TCM), dichlorobromomethane (DCBM), chlorodibromomethane (CDBM) and tribromomethane (TBM)) and HANs (including trichloroacetonitrile (TCAN), DCAN, BCAN and DBAN) for Jinlan Reservoir water originated from our previous studies (Hong et al. 2013a, b), while for Tai Lake and Qiantang River, the THMs and HANs data were obtained by chlorination tests according to the orthogonal design, which was the same as Jinlan reservoir (Hong et al. 2013b, S-Table 1). The design included the factors of chlorine dose (Cl2/DOC = 0.5, 1.5, 3.0 mg mg−1), pH (6, 7, 8), Br− level (ambient (am), am+100, am+200 and am+400 µg L−1), reaction time (2, 24, 72 h) and temperature (10, 20, 30 °C), and only one parameter varied at a time, while other parameters were kept at the “baseline” condition (i.e. Cl2/DOC = 1.5 mg/mg, pH 7.0, time = 24 h, Br−=am, and temperature = 20 °C).
Chlorination tests were carried out in a series of glass tubes. Each treatment had two replicates. After spiked with chlorine, the water samples were incubated in the dark for 2–-72 h. Determination of THMs and HANs was performed according to U.S. EPA551.1. Specifically, the residual chlorine in water sample was quenched with ammonium chloride. Then the water sample was adjusted to pH 5.0. After adding methyl tert-butyl ether (containing 1,2-dibromopropane as internal standard) and Na2SO4, the water sample was then shaken vigorously for two minutes. Finally, the supernatant was injected into GC-ECD system. The detection limits and the recovery rates for THMs (TCM, DCBM, CDBM and TBM) and HANs (TCAN, DCAN, BCAN and DBAN) are shown in S-Table 2.
Development of regression models
Step-wise multiple regression procedure in SPSS software (Version 16.0) was used to develop the models of DBPs, which included THMs (TCM, DCBM, total THMs (T-THMs)) and HANs (DCAN, BCAN, total-HANs (T-HANs)). Other DBPs were not included because their levels in some treatments were below the detection limit (S-Table 3–5). Before model generation, the DBPs concentrations, the water quality parameters (DOC, DON, UV254 and Br−) and the chlorination factors (Cl2/DOC, time, temperature and pH) were transformed to logarithmic values. Then the logarithmic forms of THMs and HANs were defined as dependent variable (log10 Y); logarithmic forms of water quality and chlorination factors were defined as independent variables (log10 X i ). The independent variables (log10 X i ) enter into the equation in order of their partial correlations with the dependent variable (log10 Y). Thus, the most important factors are identified, and the regression models of log10 Y = a0 + a1log10 X 1 + a2log10 X 2….. + ailog10 X i were developed. Finally, the developed models were transformed to the equivalent equation of Y = 10a0 X a11 X a22 ….X aii .
Results and discussion
Regression models for THMs and HANs
THMs and HANs levels in chlorinated waters are summarized in Table 2. In terms of T-THMs and T-HANs, the highest levels were observed in Tai Lake, followed by Qiantang River, and the last was Jinlan Reservoir. But the mean T-THM levels were all within the range of DBPs standard regulated by China (Ministry of Health 2006).
The multiple regression models of DBPs are presented in the following Eqs. (1)–(6). It shows that both T-THMs and T-HANs were positively correlated with DOC, Br−, chlorine dose (Cl2/DOC), temperature (Temp) and reaction time (t) (Eq. 1, 4). These results were generally expected. The high DOC concentration means the high level of DBPs precursor, which may significantly enhance the formation of THMs and HANs (Nikolaoua and Lekkasa 2001). In this study, the contribution of DOC to T-THMs and T-HANs yields mainly derived from the DOC-dependent increase in TCM and DCAN (Eq. 2, 5), respectively. The bromide, which acts as the inorganic precursors during DBPs formation, was quickly transformed to hypobromous acid (HOBr) during chlorination. Similar to hypochlorous acid (HOCl), the HOBr can react with DOM leading to the formation of brominated DBPs (Symons et al. 1993; Hong et al. 2013b). Due to the more powerful substitution ability of HOBr and the higher atomic weight of bromine than chlorine (Symons et al. 1993; Xie 2004), the brominated DBPs (DCBM, BCAN) as well as the total DBPs (T-THMs and T-HANs) increased (Eq. 1, 3, 4, 6) but the chlorinated DBPs (TCM, DCAN) decreased (Eq. 2, 5) with the increase in bromide level. Moreover, formation of DBPs is a multi-stage process, and some intermediate by-products may form during chlorination (Bond et al. 2012; Shah and Mitch 2012). A suitable increase in chlorine dose and reaction time may result in a more complete reaction and produce more of the downstream products such as the THMs and HANs. Therefore, the observation that chlorine dose and reaction time had positive influence on THMs and HANs formation (Eqs. 1–6) was reasonable. In addition, HANs belong to the intermediate DBPs, too much chlorine or long reaction time may accelerate its decomposition and lead to the reduction of HANs yields (Xie 2004; Yang et al. 2007), but this situation did not occur in this study. The elevation of temperature can accelerate the reaction rate, which may result in the quick accumulation of the stable DBPs such as the THMs. On the other hand, the elevated temperature may accelerate the hydrolysis and decomposition of the unstable DBPs such as the HANs (Yang et al. 2007). Thus, the temperature-dependent increase in THMs (Eq. 1, 2, 3) was within the expectation. While for the yields of T-HANs and DCAN (Eq. 4, 5), temperature also showed positive effects, implying that the generation outweighed the decomposition.
The regression models in this study also revealed that pH had positive effect on THMs formation (Eq. 1, 2, 3). This was because the alkaline conditions can facilitate the hydrolysis of intermediate DBPs to form THMs (Xie 2004). As an intermediate product, HANs may also undergo base-catalyzed decomposition (Yang et al. 2007), yet in this study, no significant effect of pH was observed on HANs formation (Eqs. 4–6,). In addition, DOC/UVA254 other than DON showed positive effects on HANs formation (Eqs. 4–6), which seems not to be consistent with previous studies that higher level of organic nitrogen led to the more yields of HANs (Reckhow et al. 1990; Nikolaoua and Lekkasa 2001). This may be because not all of the organic nitrogen is potent precursors for HANs. For example, under the same chlorination conditions, the HANs yields from asparagic acid, alanine, tyrosine and tryptophane were great, yet the HANs yields from glycine, isoleucine and other amino acids were negligible (Yang et al. 2012). In other words, the characteristics of the DON rather than the amount of DON may influence the yields of nitrogen DBPs (Chu et al. 2010).
According to the partial correlation coefficients (Table 3), DOC and Br− exerted the most important influence on the formation of T-HANs and DCAN, while for T-THMs and TCM, DOC, Br− as well as the reaction time showed the dominant effect; as far as the brominated DBPs (BDCM and BCAN) were concerned, the bromide level showed the most important influence. In addition, the partial correlation coefficients of reaction time, temperature and pH in THMs models were dominantly higher than those of HANs. The related mechanism may be associated with the stability of THMs (stable end product) and HANs (relatively unstable, intermediate product).
The regression models of (1)–(6) were generally valid to describe the experimental data with R 2 values ranging from 0.93 to 0.97, compared with other DBPs formation models with R 2 values ranging from 0.28 to 0.98 (Chowdhury et al. 2009). Independent sample t test in which the significance level was performed also showed that there were no significant difference between the measured date and the predicted data for all of the models (S-Table 6), indicating that the observed data were equivalent to the predicted one. Figure 1 shows the internal evaluation of DBPs models. An ideal simulation corresponds to an intercept of 0, a slope of 1, and R 2 of 1.0 (Sohn et al. 2004). In present study, the slopes of the predicted versus measured THMs ranged from 0.99 to 1.05, and the corresponding R 2 values ranged between 0.94 and 0.96 (Fig. 1a–c), demonstrating that the THMs models had good accuracy and precision. Conversely, the HANs models showed similar accuracy (slope: 0.96-1.02) but less precision (R 2: 0.77–0.92) (Fig. 1d–f). As for the models of T-THM, TCM and BDCM, 86.1–97.2 % of the predicted values fell within ±25 % of the measured values (defined as “normal values”), with only small fractions (0–2.8 %) of extreme values (out of ±40 % of the measured values). While for the HANs models, T-HANs, DCAN and BCAN appear weaker evaluation ability (normal values: 75–83 %; extreme values: 5.6–13.9 %).
Comparison of T-THMs models developed from DOM with different SUVA values
In order to evaluate the contribution of DOM with different SUVA to DBPs formation, T-THMs model (DOC-based) developed in this study was compared with those from other studies, which also took the similar power function form (i.e. DBPs = k(DOC)aBr−bTempc…..) (Table 4). Considering that several papers developed the models but did not provide SUVA values, the papers used to compare in Table 4 only included those provided SUVA values, and shared the roughly similar water quality and chlorination conditions (Amy et al. 1998; Sohn et al. 2004; Hong et al. 2007, 2008). The coefficients represent the effect of factor on DBPs formation (Sohn et al. 2004). Results show that the highest coefficient of DOC (CDOC) in T-THMs models was observed for the raw water in USA (CDOC = 1.098), followed by the raw water in Pearl River Delta (CDOC = 0.852), the coagulated water in USA (CDOC = 0.801), and the least was the raw water in Yangtze River Delta in this study (CDOC = 0.369).The rank of the DOC coefficients generally agreed with the corresponding SUVA values (Table 4, Eqs. 1–4), i.e. the higher the SUVA, the higher the DOC coefficient. This may be because the DOM with high SUVA was more reactive precursors for THMs formation (Nikolaoua and Lekkasa 2001; Uyak and Toroz 2007). That is to say if other parameters keep the same, less THMs will form for low SUVA waters.
Moreover, the coefficients of bromide (CBr) in T-THMs models also appeared to be SUVA dependent (Table 4). CBr for water with high SUVA values was much lower (CBr = 0.068 in Eq. 1) than those with low SUVA values (CBr = 0.218–0.223 in Eq. 2, 4 and 5). The underlying reason may be that the bromide was more reactive with hydrophilic DOM (had low SUVA) as compared with the hydrophobic DOM (had high SUVA) (Liang and Singer 2003). This means that under the same conditions, a greater percentage of bromide could be substituted into THMs molecules for the water with lower SUVA values, and thus make a greater contribution to the formation of THMs. Based on this pattern, it can be inferred that during DBPs formation, bromide tend to be a key factor for the water with low SUVA, and likely to be a minor factor for the water with high SUVA.
Comparison of the models in Table 4 further revealed that the coefficients of reaction time (C t ) were closely related with the length of the reaction time. C t values in short-time models (t = 1–2 h, C t = 0.34; t = 2–72 h, C t = 0.305) were generally higher than those in long-time models (t = 2–96 h, C t = 0.246; t = 6–168 h, C t = 0.258; t = 2–168 h, C t = 0.263–0.264). These results indicated that THMs formation was more rapid in short term than in long term.
The above comparisons indicated that the regression models originated from various waters were site specific. The applications of the models for evaluating THMs formation should consider the water quality, especially the SUVA. It not only influenced the total yields of THMs, but also controlled the contribution of bromide to THMs formation. Besides SUVA, the boundary of reaction time as well as other factors also should be taken into account when using one model to evaluate the THMs formation in another place.
Conclusions
The regression models could well evaluate the formation of THMs and HANs during chlorination of source water from Tai Lake, Qiantang River and Jinlan Reservoir. As for the models for TCM, BDCM and T-THMs, 86–97 % of the calculated values fell within ±25 % of the measured values. While the models for DCAN, BCAN and T-THANs showed relatively weak evaluation ability, as only 75–83 % of the calculated values were within ±25 % of the measured values. The DOM level (DOC/UV254) and the bromide concentration were the key factors controlling the HANs formation. In terms of THMs formation, DOM, bromide as well as the reaction time exerted the most important influence. Comparison of T-THMs models generated from various waters shows that the coefficients of DOC and bromide were generally dependent on SUVA values. The T-THMs models from the low SUVA water may have low DOC coefficients but high bromide coefficients. In addition, the coefficients of time seemed to be close related with the length of the chlorination time.
References
Abdullah, A. M., & Hussona, S. E. D. (2013). Predictive model for disinfection by-product in Alexandria drinking water, northern west of Egypt. Environmental Science and Pollution Research, 20, 7152–7166.
Amy, G. L., Siddiqui, M., Ozekin, K., Zhu, H. W., & Wang, C. (1998). Empirical based models for predicting chlorination and ozonation byproducts: haloacetic acids, chloral hydrate, and bromate. USEPA, EPA report CX 819579.
Bond, T., Goslan, E. H., Parsons, S. A., & Jefferson, B. (2012). A critical review of trihalomethane and haloacetic acid formation from natural organic matter surrogates. Environmental Technology Reviews, 1, 93–113.
Bull, R. J., & Robinson, M. (1985). Carcinogenic activity of haloacetonitrile and haloacetone derivatives in the mouse skin and lung. In R. L. Jolley (Ed.), Water Chlorination: Chemistry, Environmental Impact and Health Effects, (Vol. 5, pp. 221–227). Chelsea: Lewis Publishers, Inc.
Chen, B. Y., & Westerhoff, P. (2010). Predicting disinfection by-product formation potential in water. Water Research, 44, 3755–3762.
Chowdhury, S., Champagne, P., & McLellan, P. J. (2009). Models for predicting disinfection byproduct (DBP) formation in drinking waters: A chronological review. Science of the Total Environment, 407, 4189–4206.
Chu, W. H., Gao, N. Y., Deng, Y., & Krasner, S. W. (2010). Precursors of dichloroacetamide, an emerging nitrogenous DBP formed during chlorination or chloramination. Environmental Science and Technology, 44, 3908–3912.
Chu, W. H., Gao, N. Y., Yin, D. Q., Deng, Y., & Templeton, M. R. (2012). A predictive model for the formation potential of dichloroacetamide, a nitrogenous disinfection by-product formed during chlorination. International Journal of Environmental Science and Technology, 9, 701–704.
Daniel, F. B., Schenck, K. M., & Mattox, J. K. (1986). Genotoxic properties of haloacetonitriles: Drinking water by-products of chlorine disinfection. Fundamental and Applied Toxicology, 6, 447–453.
Dong, M. Q., Dai, R., Li, X. M., & Zhang, B. (2012). Risk and control of water quality from Qiangtang River. Water Supply and Drainage: Supplement, 38, 62–65. (in Chinese).
Fang, J. Y., Ma, J., Yang, X., & Shang, C. (2010). Formation of carbonaceous and nitrogenous disinfection by-products from the chlorination of Microcystis aeruginosa. Water Research, 44(6), 1934–1940.
Guilherme, S., & Rodriguez, M. J. (2015). Short-term spatial and temporal variability of disinfection by-product occurrence in small drinking water systems. Science of the Total Environment, 518–519, 280–289.
Hong, H. C., Liang, Y., Han, B. P., Mazumder, A., & Wong, M. H. (2007). Modeling of trihalomethane (THM) formation via chlorination of the water from Dongjiang River (source water for Hong Kong’s drinking water). Science of the Total Environment, 385, 48–54.
Hong, H. C., Wong, M. H., Mazumder, A., & Liang, Y. (2008). Trophic state, natural organic matter content, and disinfection by-product formation potential of six drinking water reservoirs in the Pearl River Delta, China. Journal of Hydrology, 359, 164–173.
Hong, H. C., Wong, M. H., & Liang, Y. (2009). Amino acids as precursors of trihalomethane and haloacetic acid formation during chlorination. Archives of Environmental Contamination and Toxicology, 56, 638–645.
Hong, H., Huang, F., Lin, H., Yu, H., Wang, F., & Ding, L. (2013a). Factors affecting formation of haloacetonitriles and haloketones during chlorination/monochloramination of Jinlan Reservoir water. Water Science and Technology: Water Supply, 13, 1123–1129.
Hong, H. C., Xiong, Y. J., Ruan, M. Y., Liao, F. L., Lin, H. J., & Liang, Y. (2013b). Factors affecting THMs, HAAs and HNMs formation of Jin Lan Reservoir water exposed to chlorine and monochloramine. Science of the Total Environment, 444, 196–204.
Hong, H., Qian, L., Xiong, Y., Xiao, Z., Lin, H., & Yu, H. (2015). Use multiple regression models to evaluate the formation of halonitromethane via chlorination/chloramination of water collected from Tai Lake and Qiantang River, China. Chemosphere, 119, 540–546.
Kim, D., Amy, G. L., & Karanfil, T. (2015). Disinfection by-product formation during seawater desalination: A review. Water Research, 81, 343–355.
Krasner, S. W., Weinberg, H. S., Richardson, S. D., Pastor, S. J., Chinn, R., Sclimenti, M. J., et al. (2006). Occurrence of a new generation of disinfection byproducts. Environmental Science and Technology, 40, 7175–7185.
Liang, L., & Singer, P. C. (2003). Factors influencing the formation and relative distribution of haloacetic acids and trihalomethanes in drinking water. Environmental Science and Technology, 37, 2920–2928.
Ministry of Health, PRC (2006). Water quality standards for drinking water GB5749-2006. Beijing, P.R. China.
Muellner, M. G., Wagner, E. D., McCalla, K., Richardson, S. D., Woo, Y. T., & Plewa, M. J. (2007). Haloacetonitriles versus regulated haloacetic acids: Are nitrogen-containing DBPs more toxic? Environmental Science and Technology, 41, 645–651.
Mukundan, R., & Van Dreason, R. (2014). Predicting trihalomethanes in the New York city water supply. Journal of Environmental Quality, 43, 611–616.
Nikolaoua, A. D., & Lekkasa, T. D. (2001). The role of natural organic matter during formation of chlorination by-products: A review. Acta Hydrochimica et Hydrobiologica, 29, 63–77.
Pifer, A. D., & Fairey, J. L. (2014). Suitability of organic matter surrogates to predict trihalomethane formation in drinking water sources. Environmental Engineering Science, 31, 117–126.
Reckhow, D. A., Singer, P. C., & Malcolm, R. L. (1990). Chlorination of humic materials: Byproduct formation and chemical interpretations. Environmental Science and Technology, 24, 1655–1664.
Richardson, S. D., Plewa, M. J., Wagner, E. D., Schoeny, R., & Demarini, D. M. (2007). Occurrence, genotoxicity, and carcinogenicity of regulated and emerging disinfection by-products in drinking water: a review and roadmap for research. Mutation Research, 636, 178–242.
Salas, L. A., Cantor, K. P., Tardon, A., Serra, C., Carrato, A., Garcia-Closas, R., et al. (2013). Biological and statistical approaches for modeling exposure to specific trihalomethanes and bladder cancer risk. American Journal of Epidemiology, 178, 652–660.
Shah, A. D., & Mitch, W. A. (2012). Halonitroalkanes, halonitriles, haloamides, and N-nitrosamines: A critical review of nitrogenous disinfection byproduct formation pathways. Environmental Science and Technology, 46, 119–131.
Sohn, J., Amy, G., Cho, J., Lee, Y., & Yoon, Y. (2004). Disinfectant decay and disinfection by-products formation model development: Chlorination and ozonation by-products. Water Research, 38, 2461–2478.
Symons, J. M., Krasner, S. W., Simms, L. A., & Sclimenti, M. (1993). Measurement of THM and precursor concentrations revisited: The effect of bromide ion. Journal—American WaterWorks Association, 85, 51–62.
Uyak, V., & Toroz, I. (2007). Disinfection by-product precursors reduction by various coagulation techniques in Istanbul water supplies. Journal of Hazardous Materials, 141, 320–328.
Wetzel, R. G. (2001). Limnology: Lake and river ecosystems (3rd ed.). San Diego, USA: Academic Press.
Xie, Y. F. (2004). Disinfection byproducts in drinking water: Formation, analysis and control. Washington, DC: Lewis Publishers.
Xue, C., Wang, Q., Chu, W., & Templeton, M. R. (2014). The impact of changes in source water quality on trihalomethane and haloacetonitrile formation in chlorinated drinking water. Chemosphere, 117C, 251–255.
Yang, X., Shang, C., & Westerhoff, P. (2007). Factors affecting formation of haloacetonitriles, haloketones, chloropicrin and cyanogen halides during chloramination. Water Research, 41, 1193–1200.
Yang, X., Shen, Q. Q., Guo, W. H., Peng, J. F., & Liang, Y. M. (2012). Precursors and nitrogen origins of trichloronitromethane and dichloroacetonitrile during chlorination/chloramination. Chemosphere, 88, 25–32.
Yoon, J., Choi, Y., Cho, S., & Lee, D. (2003). Low trihalomethane formation in Korean drinking water. Science of Total Environment, 302, 157–166.
You, Z. L., Zhu, J. H., Zhang, X., & Hua, W. (2012). The mechanism and control of bromate formation from raw Tai Lake water, which contained bromide. Water Waste Eng (supplement), 38, 20–23. (in Chinese).
Acknowledgments
This study was financially supported by National Natural Science Foundation of China (No. 21107099, 41373141 and 21377002), Zhejiang Provincial Natural Science Foundation of China (Grant No. Y5110157), Special Foundation for provincial scientific research institutions provided by Science and Technology Department of Zhejiang Province (No. 2015F50014), Chinese Academy of Sciences President’s International Fellowship Initiative (No. 2013T1G0038), and the Foundation of Science and Technology Bureau of Jinhua (No. 2014A33208), which the authors highly appreciate.
Author information
Authors and Affiliations
Corresponding authors
Electronic supplementary material
Below is the link to the electronic supplementary material.
Rights and permissions
About this article
Cite this article
Hong, H., Song, Q., Mazumder, A. et al. Using regression models to evaluate the formation of trihalomethanes and haloacetonitriles via chlorination of source water with low SUVA values in the Yangtze River Delta region, China. Environ Geochem Health 38, 1303–1312 (2016). https://doi.org/10.1007/s10653-016-9797-1
Received:
Accepted:
Published:
Issue Date:
DOI: https://doi.org/10.1007/s10653-016-9797-1