Abstract
The impacts of climate change on streamflow in the upper Yangtze River basin were studied using four hydrological models driven by bias-corrected climate projections from five General Circulation Models under four Representative Concentration Pathways. The basin hydrological responses to climate forcing in future mid-century (2036–2065) and end-century (2070–2099) periods were assessed via comparison of simulation results in these periods to those in the reference period (1981–2010). An analysis of variance (ANOVA) approach was used to quantify the uncertainty sources associated with the climate inputs and hydrological model structures. Overall, the annual average discharge, seasonal high flow, and daily peak discharge were projected to increase in most cases in the twenty-first century but with considerable variability between models under the conditions of increasing temperature and a small to moderate increase in precipitation. Uncertainties in the projections increase over the time and are associated with hydrological model structures, but climate inputs represent the largest source of uncertainty in the upper Yangtze projections. This study assessed streamflow projections without considering water management practices within the basin.
Similar content being viewed by others
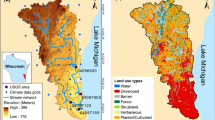
Avoid common mistakes on your manuscript.
1 Introduction
It is widely acknowledged that climate change will influence river flow regimes worldwide. Climate change can significantly alter the regional hydrologic cycle via changes in the various climatic elements (Chang et al. 2011; IPCC 2013). In the last two decades, the increased availability of modeling methods and input data has led to many hydrological impact studies in numerous river basins and countries (Jiang et al. 2007; Elshamy et al. 2009; Jha and Gassman 2014; Alfieri et al. 2015; Vetter et al. 2015). Furthermore, several studies have analysed changes in hydrological regimes across the global domain (Doll and Zhang 2010; Arnell and Gosling 2013). The main conclusion derived from these assessments is that substantial variability exists in the simulated impacts caused by climate scenarios and impact models.
Recently, some studies have explored the uncertainty in impact assessments (Bastola et al. 2011; Najafi et al. 2011; Hagemann et al. 2013; Dams et al. 2015). They concluded that many factors, including the choice of the General Circulation Model (GCM) and downscaling technique, the hydrological model (HM) structure and parametrisation are responsible for the uncertainty in river flow projections. However, the factors that induce the largest degree of uncertainty differ across climatic regions. Globally, in accordance with these studies, the largest source of uncertainty is usually associated with GCMs, but impact models could notably contribute to uncertainty in snow-dominated regions (Giuntoli et al. 2015). Therefore, the hydrological response to climate change should be assessed using multiple combinations of GCMs, emission scenarios, and hydrological models (Braud et al. 2010).
Originating from the Tibetan Plateau and reaching the Pacific Ocean after flowing through mainland China, the Yangtze River is the longest river on the Eurasian continent. A monsoon climate dominates in the region, and the large intra- and inner-annual variabilities in precipitation make the basin highly sensitive to flood and drought hazards. Several previous studies have attempted to assess the impacts of climate change on river flow in the Yangtze River basin under IPCC SRES emission scenarios, and they found that annual runoff might decrease in the twenty-first century in a warming world, with the most evident reductions occurring during the wet season (Cao et al. 2011; Zeng et al. 2012; Wang et al. 2015). However, it is difficult to sufficiently relate climate change signals to hydrological variations and project streamflow changes using one GCM/RCM and one HM due to the associated uncertainty. Therefore, to better understand the changes in streamflow in the upper Yangtze River basin, it is necessary not only to quantify the impacts of climate change on streamflow via multi-model simulations but also to provide assessments of the sources and ranges of uncertainty in the projections. This type of analysis has particular significance because the upper Yangtze River basin is the source area for a large water transfer project in China.
The main objective of this study was to explore the responses of annual average discharge, seasonal high flow and daily peak discharge to climate change in the twenty-first century in the upper Yangtze basin using climate inputs from five GCMs under four Representative Concentration Pathway (RCP) emission scenarios, as well as four HMs. Furthermore, the relative contributions of the uncertainty associated with models (GCMs and HMs) and RCPs in the streamflow projection were quantified, with the aim of supporting water management in the Yangtze River basin.
2 Study area, data and methodology
2.1 Study area
This study focused on the upper Yangtze River basin located upstream of the Cuntan hydrological gauging station (29°37′N, 106°36′E), which drains an area of approximately 8.6 × 105 km2 and provides more than 80% of the river flow to the Three Gorges Project. The upper Yangtze, with its various landscapes and abundant natural resources, plays an important role in the national socioeconomic development of China. A subtropical monsoon (a plateau monsoon in the headwater region) is prevalent in the study region, with average annual precipitation of 1018 mm during 1961–2010 (nearly 78% of it falling between May and September). The annual average temperature is 12.3°C during 1961–2010 (minimum 2.5°C in January and maximum 20.7°C in July). Mountains and plateaus constitute 90% of the basin area, but forest coverage is comparatively low, covering only 26% of the entire basin.
Of the 22% of land used for crops, slope cropland accounts for almost 45% of the area, leading to widespread water and soil losses. Because of the large topographic gradient and enormous water supply of approximately 10,000 m3/s (annual average), the upper Yangtze has rich hydropower resources, but it is also subject to destructive flash floods during the rainy season. During the second half of the twentieth century, many hydraulic structures (reservoirs, dams, and dikes) were built on the upper Yangtze to exploit the abundant water resources, generate hydropower, and protect against flood and drought hazards, and additional construction projects are planned in the upper reaches. As a main part of the river basin management practices, nearly 50 medium- to large-sized reservoirs are in operation currently, but only a few of them were constructed before the 1990s. The total capacity of the reservoirs on the upper Yangtze River was <2.5 billion m3 in the 1980s, but this has increased tremendously to >110 billion m3 currently.
2.2 Data and hydrological models
To run the HMs, a digital elevation model with a resolution of 90 m was constructed based on the Shuttle Radar Topography Mission database. The soil property data used herein were obtained from the Harmonized World Soil Database of the Food and Agriculture Organization of the United Nations (http://www.fao.org/), and the spatial distribution of soil types, i.e., the Chinese Soil Vector Map (1:1,000,000), was obtained from the Institute of Soil Science of the Chinese Academy of Sciences. A land use map (1:1,000,000) from the 1990s was acquired from the Data Center for Resource and Environmental Sciences of the Chinese Academy of Sciences.
The WATCH reanalysis data (http://www.eu-watch.org/data_availability) at a 0.5° resolution were used to provide climatic forcing for 1971–2001 according to the Inter-Sectoral Impact Model Inter-comparison Project (ISI-MIP)-2 simulation protocol (Essou et al. 2016). Daily discharge data for the Cuntan hydrological station on the upper mainstream section of the Yangtze River for the period 1961–2015 were derived from the China Hydrological Yearbook-Yangtze.
For future projections, four impact models were driven by the outputs from five GCMs (namely GFDL-ESM2M, HadGEM2-ES, IPSL-5 CM5ALR, MIROC-ESM-CHEM, and NorESM1-M, abbreviated as GFDL, Had, IPSL, MIROC and Nor respectively) of the CMIP5 project (Moss et al. 2010) under four RCP scenarios: RCP 2.6, 4.5, 6.0 and 8.5. The GCM input data were downscaled to a grid with a 0.5° resolution and bias corrected to ensure statistical agreement with the WATCH data within the framework of ISI-MIP (Hempel et al. 2013).
One conceptual model (HBV) and three process-based models (SWAT, SWIM and VIC) were used to assess the climate change impacts in the upper Yangtze. A brief introduction to the model characteristics is provided in the editorial of this special issue (Hattermann et al. 2016).
To represent the natural flow conditions, calibration and validation of the HMs were conducted based on the observed flow at the Cuntan station from 1971 to 1980 and 1981–1990, respectively. The simulation results of the HMs in this study did not account for human interference on the natural streamflow. Streamflow in the area has been essentially modified since the 1990s through flow regulation and water abstraction by dams, dikes, and reservoirs.
The four HMs were automatically calibrated using PEST software for the period 1971–1980, and then validated for the period 1981–1990. All calibration processes used the Nash-Sutcliffe efficiency (NSE) as an objective function (Nash and Sutcliffe 1970). The percent bias (PBIAS) between the observed and simulated flows was also used as a criterion for the evaluation of the parameterization results.
2.3 Uncertainty analysis
Analysis of variance (ANOVA), which is an approach that has been used extensively for uncertainty assessment in climate change studies (Yip et al. 2011; Bosshard et al. 2013), was applied to quantify the uncertainty sources in the future runoff projections in the upper Yangtze. Following the three–way ANOVA approach applied by Vetter et al. (2015), the contribution to the projected streamflow uncertainty is decomposed into three main components: GCMs, RCPs and HMs. Four residues related to the interaction terms are added to the main components by means of arithmetic averaging. The detailed procedure of using the three–way ANOVA approach for variance decomposition is explained by Vetter et al. (2015).
3 Results
3.1 Projected climate change
Climate change time series were derived for four RCP scenarios using five downscaled GCMs and analyzed for two 30-year periods, representing mid-century (2036–2065) and end-century (2070–2099) conditions. Changes in temperature and precipitation under the different RCPs projected by the GCMs in the two time horizons were compared to those in the reference period (1981–2010), and the results can be found in the introductory paper by Hattermann et al. (2016).
It is projected that temperature will rise substantially in the upper Yangtze, with the smallest increase in summer (JJA) during both time horizons, while the largest increase will likely occur in winter (DJF) or autumn (SON) depending on the emission scenario. Relative to 1981–2010, the area-averaged annual temperature changes estimated by different GCMs for 2036–2065 will range from 1.0–2.3°C under RCP 2.6, 1.5–2.5°C under RCP 4.5, 1.2–2.1°C under RCP 6.0, and 1.9–3.3°C under RCP 8.5. By the end of twenty-first century (2070–2099), these ranges will be 0.9–2.1°C under RCP 2.6, 1.9–3.2°C under RCP 4.5, 2.2–3.7°C under RCP 6.0, and 3.5–6.1°C under RCP 8.5 in the upper Yangtze. The lowest and highest increases were projected by the GFDL and MIROC models, respectively, in both periods.
The simulation results of the different GCMs suggest that annual precipitation will change by 1.4%–6.8%, 1.9%–10.2%, −4.2%–4.4% and 0.1%–7.2% in the mid-century period and by −0.5%–10.1%, 6.8%–12.7%, −1.6%–13.8% and 3.9%–21.8% in the end-century period under the RCP 2.6, 4.5, 6.0, and 8.5 scenarios, respectively. In total, over 90% of the simulations project an increasing average annual precipitation relative to that of the reference period, whereas a negative trend was projected under the RCP2.6 scenario by GFDL and under the RCP6.0 scenario by GFDL and Had. Although the projections of annual precipitation varied between GCMs, the ensemble means from the five models showed a small to moderate increase in precipitation in both the mid- and end-century periods under all four RCPs. Seasonally, winter precipitation, which accounts for <4% of the annual total, was projected to increase most significantly, by 2.1%–10.1% and 4.7%–20.3% based on the model ensembles under the different RCPs in the two time horizons, respectively. However, even with lower rates of change of 0.4%–4.7% and 4.5%–10.3% in the mid- and end-century periods, respectively, the increase in the amount of summer precipitation is still projected to be the largest. Therefore, the intra-annual variability in precipitation in the upper Yangtze River basin is expected to increase with the time.
3.2 Calibration and validation of hydrological models
The calibration and validation runs of the four HMs (HBV, SWAT, SWIM and VIC) were performed using WATCH forcing data, and the modeling results were compared with in situ observational records. Table 1 summarizes the statistics of the simulations in the calibration and validation periods from 1971 to 1990. All the HMs reproduce river flow well, with NSE values of 0.81–0.84 and PBIAS values of −7.4%–8.2% during the calibration period 1971–1980. The model performance in the validation period 1981–1990 is slightly poorer but still satisfactory, with NSE values of 0.78–0.81 and PBIAS values of −9.8%–6.1%.
The comparison of observed and simulated streamflows in the calibration and validation periods in the upper Yangtze shows also good agreement based on the long–term average monthly streamflow dynamics of all four HMs (Fig. 1a–b). However, it is apparent that in both periods, HBV overestimates discharge at the beginning of the year, while the other three models underestimate low flows. Furthermore, an overestimation of discharge in the flood season (July–September; JAS) is evident in the HBV and VIC simulations. HBV overestimates discharge at the end of the year, while SWIM underestimates discharge in November and December.
Comparison of simulated by four hydrological models and observed long-term average monthly streamflow in the calibration and validation periods (a and b, correspondingly) and comparison of simulated and observed annual Q10 (high flow with a 10% exceedance probability) in the calibration and validation periods (c and d, correspondingly) in the upper Yangtze
Considering the simulated values of annual Q10 (the 90th percentile flow, i.e. the flow which is equaled or exceeded for 10% of the flow record), all four HMs show good performance for the peak discharge conditions in the calibration period in the upper Yangtze, with the correlation coefficients between the observed and modeled Q10 values ranging between 0.87 and 0.96, and the associated bias of −0.1%–6.9%. Comparatively lower coefficients are obtained for the Q10 simulation in the validation period, with the correlation coefficients ranging between 0.52 and 0.66, and bias within the range of 3.1%–11.7% for the four HM simulations from 1981 to 1990 (Fig. 1c–d).
3.3 Projected climate change impacts
3.3.1 Annual average discharge
Relative changes in 30–year mean annual average discharge in the four HM simulations driven by the five GCMs under the four RCPs for the two future time horizons in comparison to the GCM-driven outputs in the reference period from 1981 to 2010 are presented in Fig. 2. For the upper Yangtze, high variability is found between the simulation results, but 69% of simulation results out of the 160 combinations of the five GCMs, four RCPs, and four HMs in the two time horizons, project an increasing trend in the annual average discharge in the twenty-first century. Notably, in the second time horizon, 83% of the simulations project an increasing trend.
The model ensemble averages of the results forced by the five GCMs for each HM (Fig. 2) show that positive trends in annual average discharge are simulated by HBV and SWAT under all four RCPs in both the mid- and end-century periods compared to the reference period. The highest increase is projected by HBV under the RCP8.5 scenario. Conversely, the annual average discharge is projected to decrease by SWIM and VIC under most RCPs in the mid-century period, but this trend becomes positive (increasing) in the end-century period. The ranges of simulation results obtained from the four HMs under the ensemble condition of the GCMs are −1.1%–3.9%, −0.7%–5.7%, −3.9%–1.0%, and-4.3%–2.3% in the mid-century period, and 0.5%–6.9%, 4.3%–12.1%, −2.7%–5.7%, and 0.6%–12.5% in the end-century period for RCPs 2.6, 4.5, 6.0 and 8.5, respectively.
The MIROC-driven projections show a uniform increase in annual average discharge under the four RCPs when considering all the HM simulation results in both the mid- and end-century periods relative to the reference period. A negative trend is obvious in the GFDL-driven outputs in the mid-century period, while a positive trend is evident in the Had, IPSL and Nor driven outputs under all four RCPs in the end-century period. The ranges of simulation results from the five GCMs under the ensemble condition of the HMs are −3.0%–4.3%, −1.6%–7.9%, −11.3%–2.0%, and −4.6%–2.2% in the mid-century period, and −4.0%–9.0%, 5.6%–12.5%, −7.0%–10.1%, and 0.3%–16.7% in the end-century for RCPs 2.6, 4.5, 6.0 and 8.5, respectively. In terms of the projected annual average discharge change in the twenty-first century relative to the reference period 1981–2010, the variability (interval length) in the GCM ensemble averages of the HMs is slightly lower than the variability between the different HMs for a particular GCM. Additionally, this variability is lower than the variability in the HM averages between GCMs.
3.3.2 Seasonal high flow
The changes in streamflow in the high-flow season of July to September (JAS), which usually accounts for >50% of annual runoff in the upper Yangtze, are shown in Fig. 3. This figure compares the 30-year mean values in the two future time horizons under the four RCPs with those of the reference period. Relative to 1981–2010, it is projected that the mean value of seasonal high flow will show a small to moderate increase in most scenarios during the twenty-first century. An increasing rate of change is evident for the RCP 4.5 in the mid-century period, and a higher increase might be expected by the end of the twenty-first century.
Considering all the GCM results, changes in seasonal high flow are most obvious in the simulations using the HBV model, with a variation of up to 15.1% under the RCP 8.5 scenario in the end-century. Changes are smaller in the SWIM model simulations, with a maximum variation of 6.1%. The ranges of the results of the four HMs under the ensemble condition of the GCMs are −0.9%–4.2%, 1.3%–7.2%, −3.4%–2.2%, and −2.8%–2.9% in the mid-century period, and 1.9%–7.8%, 5.4%–13.9%, 0.6%–8.0%, and 6.1%–15.1% in the end-century period for RCPs 2.6, 4.5, 6.0 and 8.5, respectively.
The largest change in seasonal high flow is found in the simulations driven by MIROC and Had under the RCP 8.5 scenario, followed by those driven by MIROC under RCP 6.0 and by GFDL and Had under RCP 4.5 in the end-century period. However, the Nor- and IPSL-driven projections show comparatively low variability in this season for all RCPs in both the mid- and end-century periods. The ranges of the five GCM-driven simulations are −3.7%–5.7%, −3.1%–8.9%, −10.5%–5.5%, and −5.6%–2.9% in the mid-century period, and −0.4%–10.2%, 2.1%–14.8%, −4.9%–14.9%, and −2.6%–19.7% in the end-century period for RCPs 2.6, 4.5, 6.0 and 8.5, respectively.
3.3.3 Daily peak discharge
The Generalized Logistic Distribution (GLD), which is the optimal distribution for fitting the discharge in the upper Yangtze based on the Kolmogorov–Smirnov goodness of fit test, was applied to describe the statistical distribution of the daily peak discharge (represented by annual Q10) for the simulations driven by five GCMs in the reference period and all climate projections in the two future time horizons. The distribution parameter is estimated based on the 30 data samples from each period using the L–moment estimator method. Only the simulation of the RCP 4.5 scenario is shown in Fig. 4 (the results of the other three scenarios showed similar distribution patterns) to compare the Probability Density Functions (PDF) of the peak discharge in the two future time horizons to that of the reference period.
The outputs of the four HMs have relatively lower medians but higher kurtosis values in the reference period than in the two future horizons for most cases. For the combinations of the simulations of the four HMs and five GCMs, peak discharge is projected to increase visibly in the first time horizon, with 90% of projections showing increasing trends based on a median increase ranging from 0.3%–13.1%. This ratio increased to 95% in the second time horizon, with a median increase ranging from 0.3%–17.3%. The outputs driven by GFDL, Had and MIROC for all HMs reflect a consistent increasing trend. The largest increase is projected by the Had-driven HBV simulation in the end-century period. The IPSL-driven outputs by SWAT and SWIM show that peak discharge might decrease in the period 2036–2065, although the largest decrease of 2.0% is projected by the Nor-driven VIC simulation in the period 2070–2099. In addition to the increase in the median, the PDFs further show that the distribution of the peak discharge in the scenario period exhibits large skewness in most cases. Approximately 70% of the projected peak discharge has a longer right hand tail in the mid- and end-century periods than under the current climate conditions.
With the increasing probability of extremes, the return period of peak discharge will likely decrease correspondingly. Nearly 73% of cases project that the 100–year return period event from 1981 to 2010 will become more frequent, i.e. shorter than 50–year event in the twenty-first century according to the GLD fitting results (Table A1 in Supplementary Material). The 100–year return level peak discharge from 1981 to 2010 is expected to increase by 27.5% and 49.7% in the 2036–2065 and 2070–2099 periods, respectively, based on the maximum values produced by the MIROC-driven HBV simulation (Table A2 in the Supplementary Material).
3.3.4 Uncertainty assessment
Simulated time series of the relative changes in annual average discharge, seasonal high flow, and daily peak discharge for the twenty-first century compared to the reference period show small to moderate increases in all 3 variables for the upper Yangtze, and the rate of change is smaller at the mid-century than at the end-century. The differences in individual simulation results based on the combinations of each GCM and HM from the multi-model means are noticeably smaller for the projected annual average discharge than for seasonal high flow and peak discharge in the entire twenty-first century; however, the magnitude of the range increases with time for all three variables (Fig. A1 in the Supplementary Material).
Based on the ANOVA method described in the study by Vetter et al. (2015), a set of 160 samples from 80 simulations obtained from the combinations of five GCMs, four RCPs and four HMs over the two time horizons was used in this study to decompose the total uncertainty into three main components based on variance. Figure 5 shows the main sources of uncertainty estimated for the percentage changes in annual average discharge, JAS seasonal high flow and daily peak discharge in the mid- and end-century periods relative to the reference period values.
For the changes in annual average discharge, the GCMs are the most important uncertainty contributor in the mid-century period, followed by the RCPs and HMs. The climate forcing inputs (GCMs and RCPs) jointly contribute to approximately 73% of the total uncertainty, which is much higher than the contribution of the HMs (27%). The contributions of both the GCMs and the RCPs to the projected seasonal high runoff uncertainty are higher than those of the HMs in the period 2036–2065. The GCMs and RCPs jointly contribute to approximately 75% of the total uncertainty. The contributions of the three main components to the uncertainty in the projected peak discharge in the first time horizon exhibit nearly equal weights as for the seasonal high runoff projection.
At the end-century period, the contributions of the GCMs to the uncertainties of annual, seasonal and peak discharge projections increase substantially. In all three cases, the GCMs are the dominant contributors of uncertainty, which alone explain approximately 50% of the total uncertainty in streamflow changes in the period 2070–2099.
4 Discussion and concluding remarks
Using bias-corrected GCM projections and four hydrological models, this paper presents an assessment of the future impacts of climate change on streamflow in the upper Yangtze. The focus of the study was to quantify the differences in the simulation of the hydrological responses to climate change attributable to the driving GCMs and impact models, HMs.
The results show that in the upper Yangtze, the climate change projections of five GCMs under four RCPs indicate an increase in temperature across the basin in all seasons. Regarding precipitation, the projections of five GCMs under four RCPs are different, but on average a small to moderate increase in annual precipitation is projected in both the mid- and end-century periods. Meanwhile, the intra-annual variability is projected to increase with time during the twenty-first century. Relative to the reference period, the direction of change in the projected annual average discharge is the same in most cases, with 69% of simulations projecting an increase, and some variability between models and scenarios. A similar increasing trend is also projected for the seasonal high flow. The probability distribution of daily peak flow is also projected to change substantially in the twenty-first century, and the shortened return period (the 100–year return period event from 1981 to 2010 will become more frequent, as projected in 73% of cases) would pose new challenges to the construction of hydraulic projects.
The findings from this study are not consistent with the results obtained in previous studies on the effects of climate change in the upper Yangtze. By applying various single-model approaches under IPCC SRES emission scenarios, Cao et al. (2011), Zeng et al. (2012) and Wang et al. (2015) concluded that annual runoff is likely to decrease in the twenty-first century, with the greatest reductions occurring in the wet season. The considerable differences between the previous and our research results are related to the selection of climate forcing models and scenarios (e.g., GCMs/RCMs and emission scenarios), impact models, and parameterization processes, and these diverging results reflect the uncertainty associated with impact studies. A previous study by Kay et al. (2009) suggested that the structure of GCMs is by far the largest source of uncertainty in the impact of climate change on flood frequency in England. However, the uncertainties associated with impact models could be higher than those attributable to climate inputs in regions dominated by snow and ice (Giuntoli et al. 2015), and are particularly dominant in many regions affected by declining water resources (Schewe et al. 2014). Therefore, uncertainty sources should be quantified and interpreted with caution while considering regional variations. A study on uncertainty conducted in a neighboring catchment, the 1.2 × 105 km2 upper Yellow River basin, showed that the contribution of HMs to the uncertainty in runoff projections was high and almost equal to that of GCMs (Vetter et al. 2015). The current study used a multi-climate model and multi-impact model projection approach in the 8.6 × 105 km2 upper Yangtze River basin, which has a very complex geographic environment, to investigate the uncertainty related to hydrological modeling in the context of climate change. The difference in the percentage change in the projected annual average discharge between the HMs (under the ensemble condition of GCMs) reached 11.9%, while that between the GCMs (under the ensemble condition of HMs) reached 16.4% by the end of the century under the RCP 8.5 scenario.
The seasonal high flow projections show an even higher discrepancy between the simulations driven by the different GCMs. Thus, our results indicate that the major source of uncertainty in the projections of future streamflow in the upper Yangtze is related to the choice of climate forcing (GCMs and RCPs), while the hydrological impact models play an important but secondary role. This finding is similar to that of Hagemann et al. (2013), who applied multiple GCMs and global hydrological models to project the future state of global water resources in 2071–2100. They indicated that the variability associated with impact models is larger than that of climate models in some regions, but in southwest China, where the upper Yangtze is located, the GCM uncertainty was more pronounced.
The present study applied the downscaled GCM forcing data prepared within the ISI–MIP framework to enable a quantitative assessment of the climate change impacts across different regions in a consistent setting. These data were bias corrected by referring to WATCH data. However, the complex terrain in southwest China makes it difficult for reanalysis data to reach satisfactory agreement with in situ records, and there is generally a colder and a drier bias in reanalysis datasets (Bao and Zhang 2012). Our study also indicates that the basin-averaged annual mean temperature and annual precipitation from the WATCH forcing data are 5.6°C and 24.5% lower, respectively, than those observed in the basin in 1971–2000. Despite the fact that the WATCH data capture the spatial patterns of climatic variables very well, with a spatial correlation with observations of >0.90 (Fig. A2 in the Supplementary Material), the underestimation of both winter temperature and summer precipitation might influence the hydrological regimes. This could induce a higher contribution to uncertainty in the streamflow projections in the upper Yangtze River from climate inputs than from the effects of HM structures.
Therefore, an additional calibration/validation of the four models was performed using observed climate data (see results in Fig. A3 in the Supplementary Material), and the impacts were compared. A comparison of the WATCH-driven calibration/validation (step 1) and observational data based calibration/validation (step 2) results and climate impact simulations based on these two steps in the mid- and end-century periods in terms of the relative differences can be found in Fig. A4 and Fig. A5 in the Supplementary Material. The GCM-driven outputs in the historical period effectively match the WATCH-driven results (Fig. A4, left), but the GCM-driven outputs corresponding to step 2 are obviously lower than the observed climate-driven results (Fig. A4, right) due to the underestimation of precipitation by WATCH. Nevertheless, the relative changes in the long–term average monthly discharge in the end-century relative to the reference period based on these two calibration steps are quite similar (Fig. A5 in the Supplementary Material).
It was noted in some previous studies that the hydrological response using bias-corrected forcing data might produce additional uncertainties (e.g. Ehret et al. 2012). Sometimes, uncertainty due to bias correction can be of the same order of magnitude as that related to the choice of GCM or HM (Hagemann et al. 2011). Therefore, to determine the causes of the differences, in future the analysis should be extended to include the use of non-bias-corrected climate inputs.
We focused on the projection of natural streamflow in the upper Yangtze River basin during the twenty-first century under modified climate conditions. The intention was to provide useful information regarding the likely changes in both the availability of water resources and the occurrence of hydrological extremes in the mid- and long terms. However, the Yangtze River is affected considerably by human activities, and the monthly dynamics of river runoff, flood volume, and peak timing can be altered correspondingly. The human interference has intensified since the 1990s in the upper Yangtze, and the fact that river basin management practices were not considered in the hydrological simulation in this study constitutes an additional source of uncertainty because of the structure of the HMs used for runoff projections in this basin.
Another limitation of the approach presented in this paper is linked to the calibration process. Our calibration was conducted based on maximizing the NSE criterion, and parameter sets were further evaluated by PBIAS. Climate change impacts were investigated under the assumption that the optimal parameter set found in the calibration process for the historical period, would remain valid in the future. Previous climate change impact studies have shown that uncertainty related to the HM structure is more significant than the effect of parameter uncertainty in some snow-dominated river basins (Poulin et al. 2011), while in snowless river basins, parameter uncertainty is highly significant in the summer season (Wilby 2005). With 15% of the catchment area in our study fed by snowmelt water, the choice of HM parameters might produce larger uncertainties in the projected discharge. Thus, multiple parameter sets generated by different methods under various conditions should be considered in impact studies in future to account for the full range of uncertainties.
Finally, we would like to note that any impact study based on a single impact model or a single climate forcing should be interpreted with caution. Given the uncertainties that exist in the HM parameterization and initialization processes, the research results presented here require further validation by incorporating management practices, and they should be compared to modeling studies in other river basins dominated by monsoon climates using multiple hydrological models with different levels of complexity.
References
Alfieri L et al (2015) Global warming increases the frequency of river floods in Europe. Hydrol Earth Syst Sci 19:2247–2260
Arnell NW, Gosling SN (2013) The impacts of climate change on river flow regimes at the global scale. J Hydrol 486:351–364
Bao XH, Zhang FQ (2012) Evaluation of NCEP-CFSR, NCEP-NCAR, ERA-interim, and ERA-40 reanalysis datasets against independent sounding observations over the Tibetan plateau. J Clim 26:206–214
Bastola S et al (2011) The role of hydrological modeling uncertainties in climate change impact assessments of Irish river catchments. Adv Water Resour 34:562–576
Bosshard T et al (2013) Quantifying uncertainty sources in an emsemble of hydrological climate-impact projection. Water Resour Res 49:1523–1536
Braud I et al (2010) The use of distributed hydrological models for the Gard 2002 flash flood event: analysis of associated hydrological processes. J Hydrol 394:162–181
Cao LJ et al (2011) Climate change effect on hydrological processes over the Yangtze River basin. Quat Int 244:202–210
Chang H et al (2011) Quantifying uncertainty in urban flooding analysis considering hydro-climatic projection and urban development effects. Hydrol Earth Syst Sci 15:617–633
Dams J et al (2015) Multi model approach to assess the impact of climate change on runoff. J Hydrol 529:1601–1616
Doll P, Zhang J (2010) Impacts of climate change on freshwater ecosystems: a global-scale analysis of ecologically relevant river flow alterations. Hydrol Earth Syst Sci 14:783–599
Ehret U et al (2012) HESS opinions “should we apply bias correction to global and regional climate model data?”. Hydrol Earth Syst Sci 16:3391–3404
Elshamy ME et al (2009) Impact of climate change on Blue Nile flows using bias-corrected GCM scenarios. Hydrol Earth Syst Sci 13:551–565
Essou GRC et al (2016) Can precipitation and temperature from meteorological reanalyses be used for hydrological modeling? J Hydrometeorol 17:1929–1950
Giuntoli I et al (2015) Future hydrological extremes: the uncertainty from multiple global climate and global hydrological models. Earth Syst Dynam 6:267–285
Hagemann S et al (2011) Impact of a statistical bias correction on the projected hydrological changes obtained from three GCMs and two hydrological models. J Hydrometeorol 12:556–578
Hagemann S et al (2013) Climate change impact on available water resources obtained using multiple global climate and hydrology models. Earth Syst Dynam 4:129–144
Hattermann et al (2016) Cross‐scale intercomparison of climate change impacts simulated by regional and global hydrological models in eleven large river basins. Clim Chang. doi:10.1007/s10584-016-1829-4
Hempel S et al (2013) A trend-preserving bias correction-the ISI-MIP approach. Earch Syst Dynam 4:219–236
IPCC (2013) Climate change 2013: the physical basis. In: Contribution of working group 1 to the fifth assessment report of the IPCC. Cambridge University Press, New York
Jha MK, Gassman PW (2014) Changes in hydrology and streamflow as predicted by a modelling experiment forced with climate models. Hydrol Process 28:2772–2781
Jiang T et al (2007) Comparison of hydrological impacts of climate change simulated by six hydrological models in the Dongjiang Basin, South China. J Hydrol 336:316–333
Kay AL et al (2009) Comparison of uncertainty sources for climate change impacts: flood frequency in England. Clim Chang 92:41–63
Moss RH et al (2010) The next generation of scenarios for climate change research and assessment. Nature 463:747–756
Najafi MR et al (2011) Assessing the uncertainties of hydrologic model selection in climate change impact studies. Hydrol Process 25:2814–2826
Nash JE, Sutcliffe JV (1970) River flow forecasting through conceptual models: part 1-a discussion of principle. J Hydrol 10:282–290
Poulin A et al (2011) Uncertainty of hydrological modelling in climate change impact studies in a Canadian, snow-dominated river basin. J Hydrol 409:626–636
Schewe J et al (2014) Multimodel assessment of water scarcity under climate change P. Natl Acad Sci 111:3245–3250
Vetter T et al (2015) Multi-model climate impact assessment and intercomparison for three large-scale river basins on three continents. Earth Syst Dynam 6:17–43
Wang YH et al (2015) Water resource spatiotemporal pattern evaluation of the upstream Yangtze River corresponding to climate changes. Quat Int 380-381:187–196
Wilby RL (2005) Uncertainty in water resource model parameters used for climate change impact assessment. Hydrol Process 19:3201–3219
Yip S et al (2011) A simple, coherent framework for partitioning uncertainty in climate projections. J Clim 24:4634–4643
Zeng XF et al (2012) Discharge projection in the Yangtze River basin under different emission scenarios based on the artificial neural networks. Quat Int 282:113–121
Acknowledgments
This study was jointly supported by the National Basic Research Program of China (973 Program) (2013CB430205, 2012CB955903), the National Natural Science Foundation of China (51309105, 91547208), and the Sino-German Cooperation Group Project (GZ912). The authors would like to thank the ISI-MIP modeling group for providing the climate data.
Author information
Authors and Affiliations
Corresponding authors
Additional information
This article is part of a Special Issue on “Hydrological Model Intercomparison for Climate Impact Assessment” edited by Valentina Krysanova and Fred Hattermann.
Electronic supplementary material
ESM 1
(DOCX 920 kb)
Rights and permissions
About this article
Cite this article
Su, B., Huang, J., Zeng, X. et al. Impacts of climate change on streamflow in the upper Yangtze River basin. Climatic Change 141, 533–546 (2017). https://doi.org/10.1007/s10584-016-1852-5
Received:
Accepted:
Published:
Issue Date:
DOI: https://doi.org/10.1007/s10584-016-1852-5