Abstract
Purpose
Hormone receptor-positive (HR+) and human epidermal growth factor receptor 2 negative (HER2−) early breast cancer (BC) is the most prevalent BC subtype with substantial biological heterogeneity. Although clinicopathological (CP) characteristics have a clear prognostic value, additional biomarkers could refine survival prediction and guide treatment decision.
Methods
Copy number aberrations and somatic driver mutations were obtained with OncoScan CGH array and sequencing of 36 genes on HR+/HER2− node-positive early BC patients treated with chemotherapy from the PACS04 trial. We built a two-gene genomic score (GS) associated with distant disease-free survival (DDFS), whose prognostic value was assessed on the external METABRIC data (n = 1413) using overall survival (OS) and breast cancer-specific survival (BCSS).
Results
In the PACS04 trial (n = 327), the median follow-up for DDFS (65 events) was 9.6 years. FGFR1 amplifications (\({\text{HR}}_{\text{Amplification}}\) = 2.44, 95% CI [1.25; 4.76], p = 0.009) and MAP3K1 mutations (\({\text{HR}}_{\text{Mutation}}\) = 0.10, [0.01; 0.78], p = 0.03) were associated with DDFS beyond CP characteristics. A prognostic GS combining FGFR1 amplifications and MAP3K1 mutations added more information to CP model (\(\chi_{\text{DDFS}}^{2}\) = 12.97, \(p_{\text{DDFS}}\) < 0.001 and \(\chi_{\text{OS}}^{2}\) = 11.52, \(p_{\text{OS}}\) < 0.001). In the METABRIC study (n = 1413), FGFR1 amplifications (\({\text{HR}}_{\text{Amplification}}\) = 2.00 [1.40; 2.87], p < 0.001) and MAP3K1 mutations (\({\text{HR}}_{\text{Mutation}}\) = 0.58 [0.41; 0.83], p = 0.003) were significantly associated with BCSS beyond CP characteristics. The prognostic GS added significant prognostic information to CP model (\(\chi_{\text{BCSSS}}^{2}\) = 15.39, \(p_{\text{BCSS}}\) < 0.001 and \(\chi_{\text{OS}}^{2}\) = 5.62, \(p_{\text{OS}}\) = 0.02).
Conclusion
In axillary node-positive, HR+, and HER2− early BC, amplifications of FGFR1 gene were strongly associated with increased risk for distant disease, while mutations of MAP3K1 gene were significantly associated with decreased risk.
Similar content being viewed by others
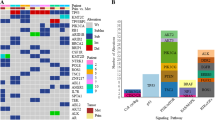
Avoid common mistakes on your manuscript.
Introduction
Hormone receptor-positive (HR+) and human epidermal growth factor receptor 2 negative (HER2−) early breast cancer (BC) is the most common BC subtype, accounting for 73% of all BC in the Unites States in 2010 [1]. In this BC subtype, patients with node-positive disease have a worse prognosis than node-negative patients. Patients with early HR+/HER2− node-positive BC are usually treated with adjuvant therapy to reduce the risk of distance disease and recurrence: chemotherapy to treat micro-metastatic disease, radiotherapy to destroy any cancer cells that remain in the breast area after surgery, and hormone therapy that is given during at least 5 years to stop estrogen receptor signaling.
Currently, to estimate an individual prognosis for clinical decision-making, clinicopathological (CP) characteristics such as age, tumor size, lymph node status, tumor grade, HER2 status, proliferation, and percentage of cells expressing estrogen and progesterone receptors are usually considered [2]. Although CP characteristics are prognostic, they do not explain all the variations in survival, as early BC patients may develop distant metastasis [3, 4] during or after chemotherapy or hormone therapy. Hence, in addition to relevant classical CP characteristics, it is of interest to study the prognostic value of additional biomarkers.
Previous studies have shown that high amplifications of CCND1 (11q13) [5, 6], FGFR1 (8p12) [7, 8], ZNF2017 (20q13) [9,10,11], and ERBB2 (17q12) [12,13,14] are associated with a poor outcome and may contribute to hormone therapy resistance. Amplifications of these genes are observed, respectively, in approximately 19.6%, 22.8%, 8.5%, and 9.9% [15] of cases. Other studies have also shown that PIK3CA [16], TP53 [17], and MAP3K1 [18] mutations are significantly associated with survival and are the most frequently mutated genes in BC with a prevalence of 36%, 37%, and 8%, respectively [19]. The aim of this study was to evaluate which genomic alterations could refine survival prediction. Using data from the PACS04 trial, we evaluated the prognostic value of the copy number of predefined well-known genes (CCND1, FGFR1, ZNF217, and ERRB2) and mutations of a panel of driver genes in addition to conventional CP characteristics. We built a genomic score to discriminate patients with different levels of risk and investigated the prognostic value in the external METABRIC cohort.
Materials and methods
Patients
Training data: PACS04 [20] was a phase III trial that included 1194 patients with node-positive, HR+, and HER2− early BC. After conservative surgery, patients were randomized to receive either six cycles of 5-fluorouracil, cyclophosphamide, and epirubicin (FEC) or six cycles of epirubicin and docetaxel 75 mg/m2 (ED). Radiotherapy was given after adjuvant chemotherapy followed by hormone therapy. Three hundred and twenty-seven patients treated with ED had clinical, DNA copy number, and somatic mutation information available. Copy numbers were obtained by the OncoScan Comparative Genomic Hybridization (CGH) array and somatic mutations by targeted sequencing (TS).
Validation data: METABRIC (Molecular Taxonomy of Breast Cancer International Consortium) [21] was a Canada–UK project that included 2509 patients with early BC. One thousand four hundred and thirteen patients had HR+ and HER2− primary tumors and had survival outcome, clinical, DNA copy number, and somatic mutation information available. Copy number and somatic driver mutations were generated using Affymetrix SNP 6.0 CGH array and TS of 173 genes. Patient selection for PACS04 and METABRIC data is summarized in Fig. 1.
Flow chart for the training and the external data. PACS04 Programme Adjuvant Cancer du Sein, METABRIC Molecular Taxonomy of Breast Cancer International Consortium, HR+ hormone receptor positive, HER2− human epidermal growth factor receptor 2 negative, BC breast cancer, TS targeted sequencing, CGH comparative genomic hybridization, FEC 5-fluorouracil (5-FU) + epirubicin + cyclophosphamide, ED epirubicin + docetaxel
DNA isolation, hybridization, and sequencing
Tumor DNA was successfully extracted from formalin-fixed and paraffin-embedded (FFPE) samples from PACS04 trial patients. Five hundred samples were collected and hybridization was performed on 433. DNA copy numbers were assessed using Oncoscan FFPE assay kit. The data were normalized and segmented via rCGH [22] algorithm, and the copy number threshold to determine amplification and deletion was, respectively, greater than 4 copies (log-2-ratio > 1) [23] and less than 1 copy (log-2-ratio < − 1) [23]. Targeted next-generation sequencing of a panel of 36 genes was performed on 394 primary breast tumor tissues from PACS04 trial. Only mutations with variant allele frequency (VAF) greater than 10% were considered. A filter method was applied to selected genes with a prevalence of mutation greater than 3% to make sure that the statistical analyses converged.
Statistical analysis
In the training data (PACS04 trial), to compare the quantity of lost information, the differences in patient characteristics between the patients included and excluded from the analysis were tested using, respectively, Kruskal–Wallis and Fisher’s exact test for continuous and categorical variables. The primary endpoint for the statistical analysis was distant disease-free survival (DDFS), defined as the time from randomization to the first recurrence (distant site) or death from any cause. The secondary endpoint was overall survival (OS), defined as the time from randomization to death from any cause. Patients who did not experience events were censored at the date of last follow-up. The correlation between CP and genomic features was analyzed using Spearman correlation and correlation coefficients value and 95% confidence intervals (CI) were obtained using 5000 bootstrap repetitions. We checked the hypothesis of log-linearity of continuous covariates in the Cox model using splines with 2 degrees of freedom. A power calculation of this study was performed. Assuming a genomic biomarker with a prevalence of 25% of alteration in the analysis population at a two-sided significance level of 0.05, if the hazard ratio (HR) for DDFS is equal to 2, then the power to reject the null hypothesis that the HR is equal to 1 with a 0.05 significance level will be 77%. In univariable analysis, the prognostic value of each genomic characteristic on DDFS was performed using Cox regression and Kaplan–Meier curves. The threshold of two-sided significance was fixed at 5%. In multivariable analysis, we estimated the CP Cox model containing age at randomization (years), tumor size (mm), tumor grade (SBR), lymph node status, estrogen receptor (ER, %), and progesterone receptor (PR, %). The CP model was compared with the model containing CP and relevant genomic (RG) characteristics using the likelihood ratio test to make sure that RG added complementary information to the traditional CP model. We also calculated the relative contribution of each variable in the Cox regression model. For internal validation, the area under the receiver operating characteristic (ROC) curve (AUC) [24] value at 5 and 10 years was used for evaluating the discrimination of prognostic CP and CP + RG models.
The genomic score (GS) was defined as the linear combination of the copy number and mutation genes in the CP + RG model. We also used the likelihood ratio test to evaluate the ability of the GS to predict OS by comparing CP model with CP + GS model.
In the validation data (METABRIC study), the DDFS endpoint was not available. Only the OS endpoint—defined as the time from diagnosis to death from any cause—and breast cancer-specific survival (BCSS) endpoint—defined as the time from diagnosis to death from BC—were available. Patients who did not experience the event were censored at the date of last follow-up. First, we built the CP + RG model found in the training data (PACS04) to ensure that the results were consistent in both data sets. Second, the GS built in the training data (PACS04) was validated in the validation data (METABRIC) to evaluate its ability to predict BCSS and OS. The CP model was compared to the CP + GS model, using the likelihood ratio test and AUC values at 5 and 10 years. We used R software version 3.5.1 for the statistical analysis and threshold of two-sided significance was fixed at 5%.
Data availability
The data sets generated during and/or analyzed during the current study are not publicly available in respect with the patient consent forms. Data access can be requested from the corresponding author.
Results
Description of PACS04 study population (training population)
Three hundred and twenty-seven patients (27%) with node-positive, HR+/HER2− BC treated with ED were selected. The other 867 patients (73%) did not have CGH data (66%) or TS data (6%) available or were not treated with ED (~ 1%). No significant differences were noted in the patient characteristics between selected (n = 327) and unselected (n = 867) patients (Table 1). The proliferation marker Ki-67 was excluded from the rest of the analyses because of too many missing values.
The copy number status of some well-known genes (CCND1, FGFR1, ZNF217, and ERBB2) was obtained from CGH data. 21.4%, 15%, 12.5%, and 5.2% of patients, respectively, had amplifications for CCND1, FGFR1, ZNF217, and ERBB2 (Fig. 2). In the TS data based on a panel of 36 sequenced genes (PIK3CA, TP53, MAP3K1, BRCA2, ATM, GATA3, NF1, MTOR, AKT1, BRCA1, TSC2, MAP2K4, PTEN, TSC1, ESR1, PALB2, EGFR, ERBB2, FBXW7, KEAP1, RAD51B, RAD51C, STK11, FGFR4, PIK3R1, SF3B1, ALK, CTNNB1, DDR2, ERBB3, ERBB4, FGFR2, KDR, MET, RET, and RHOA), 12 genes (33%) were selected and 24 genes (67%) were excluded because the prevalence of mutation was less than 3% (Supplementary Table S1). PIK3CA, TP53, and MAP3K1 were the most mutated genes and the prevalence was, respectively, 29.1%, 14.7%, and 14% (Fig. 2). All genomic characteristics are described in supplementary data (Supplementary Tables S1 and S2).
Landscape of somatic driver alterations in node-positive HR+/HER2− breast cancer (PACS04 trial). CCND1 Cyclin D1, FGFR1 fibroblast growth factor receptor 1, ZNF217 zinc finger protein 217, ERBB2 receptor tyrosine kinase 2. Normal, copy number equal to 2. Amplification, copy number greater than 4. Deletion, copy number less than 1. Only mutations with a prevalence of 3% or greater are displayed. PIK3CA, Phosphatidylinositol 4,5-bisphosphate 3-kinase catalytic. TP53, Tumor Protein p53. MAP3K1, Mitogen-Activated Protein Kinase Kinase Kinase 1. BRCA2, Breast Cancer 2. ATM, Ataxia Telangiectasia Mutated. GATA3, GATA binding protein 3. NF1, Neurofibromin 1. MTOR, Mechanistic Target of Rapamycin Kinase. AKT1, Serine-threonine protein Kinase 1. BRCA1, Breast cancer 1. TSC2, Tuberous Sclerosis 2 protein. MAP2K4, Mitogen-Activated Protein Kinase Kinase 4
Correlation between CP and genomic features in the training data (PACS04 trial)
The correlation between CP and genomic features is given in Table 2.
Copy numbers and mutations associated with DDFS in the training data (PACS04 trial)
After a median follow-up of 9.6 years, we observed 65 events for 327 patients in the analysis population. In the univariable analysis, copy number alterations of FGFR1 (\({\text{HR}}_{\text{Amplification}}\) = 2.18, 95% CI [1.21; 3.91], p < 0.01 and \({\text{HR}}_{\text{Deletion}}\) = 3.22, 95% CI [1.28; 8.12], p = 0.01) and ZNF217 (\({\text{HR}}_{\text{Amplification}}\) = 1.99, 95% CI [1.08; 3.65], p = 0.03) were associated with an increased risk of distant disease, whereas MAP3K1 mutations (\({\text{HR}}_{\text{Mutation}}\) = 0.09, 95% CI [0.01; 0.63], p = 0.02) were associated with a decreased risk of distant disease (Table 3). The Kaplan–Meier analysis showed the similar results for FGFR1 (\(p_{{\log {\text{ - rank}}}} = 0.0024\)), ZNF217 (\(p_{{\log {\text{ - rank}}}} = 0.024\)) and MAP3K1 (\(p_{\log {\text{ - rank}}} = 0.0023\)) (Figs. 3 and 4). The verification of the log-linearity hypothesis is provided in Supplementary Figs. S1, S2, and S3.
Survival curves according to copy number status of well-known genes in the training data (PACS04 trial). N number of patients, N-event number of events. Normal: copy number equal to 2; Amplification: copy number greater than 4; Deletion: copy number less than 1; p: p value associated with the log-rank test
In multivariable analysis, the model containing CP and relevant genomic characteristics selected during the univariable analysis step (CP + FGFR1 + ZNF217 + MAP3K1) showed that ZNF217 copy numbers (\({\text{HR}}_{\text{Amplification}}\) = 1.67, 95% CI [0.79; 3.53], p = 0.2) were not associated with DDFS (Table 4) and did not add further prognostic information (χ2= 1.62, p = 0.2) compared to the CP + FGFR1 + MAP3K1 model (Table 5). The final Cox model selected was CP + FGFR1 + MAP3K1 and showed that FGFR1 amplifications (\({\text{HR}}_{\text{Amplification}}\) = 2.44, 95% CI [1.25; 4.76], p = 0.009) were associated with a poor prognosis and MAP3K1 mutations (\({\text{HR}}_{\text{Mutation}}\) = 0.10 [0.01; 0.78], p = 0.03) were associated with a good prognosis (Table 4). MAP3K1 mutations alone added information to the CP model (χ2= 7.49, p = 0.006) whereas FGFR1 copy numbers alone did not (χ2= 4.96, p = 0.081). It was the combination of FGFR1 copy numbers and MAP3K1 mutations that added relevant complementary information to CP model (χ2= 15.08, p < 0.01) in determining DDFS (Table 5). These results were also confirmed by the AUC criteria. The AUC at 5 and 10 years were higher for CP + FGFR1 + MAP3K1 model in comparison with the CP model. The AUC values were, respectively, 81.39 and 67.47 at 5 and 10 years for CP model and were, respectively, 84.99 and 72.86 at 5 and 10 years for CP + FGFR1 + MAP3K1 model. The ROC curves are given in Supplementary Fig. S4 and Table S3. The relative contribution of FGFR1 (rank = 3, χ2= 8.50, p = 0.014) and MAP3K1 (rank = 4, χ2= 4.71, p = 0.03) was significant in determining DDFS, after the usual important prognostic variables tumor size and lymph node status ranked 1st and 2nd (Table S4).
Development of a genomic score based on the training data (PACS04 trial)
From the CP + FGFR1 + MAP3K1 model, we established a Genomic Score (GS) using only the coefficients associated with FGFR1 copy numbers and MAP3K1 mutations. The GS was split into three groups for biological relevance. The GS distribution is given in Fig. 5. Low risk corresponds to MAP3K1 mutated (GS < 0); moderate risk corresponds to MAP3K1 not mutated and FGFR1 not amplified (GS = 0); high risk corresponds to MAP3K1 not mutated and FGFR1 amplified (GS > 0).
The Kaplan–Meier figure of DDFS according to this grouping shows a clear separation (Fig. 6). As per the definition of the score, it added additional information (\(n_{\text{DDFS}}\) = 307, \(n{\text{ - event}}_{\text{DDFS}}\) = 58, \(\chi_{\text{DDFS}}^{2}\) = 12.97, \(p_{\text{DDFS}}\) < 0.001 and \(n_{\text{OS}}\) = 307, \(n{\text{ - event}}_{\text{OS}}\) = 45, \(\chi_{\text{OS}}^{2}\) = 11.52, \(p_{\text{OS}}\) < 0.001) to the CP model for DDFS and OS. The model description is given in Supplementary Table S5. The comparison between a CP + KI67 + GS model versus a CP + KI67 model on the small subset of 158 patients with KI67 data showed that the GS added additional information to this model especially for OS (\(n_{\text{DDFS}}\) = 158, \(n{\text{ - event}}_{\text{DDFS}}\) = 29, \(\chi_{\text{DDFS}}^{2}\) = 2.92, \(p_{\text{DDFS}}\) = 0.083 and \(n_{\text{OS}}\) = 158, \(n{\text{ - event}}_{\text{OS}}\) = 24, \(\chi_{\text{OS}}^{2}\) = 4.06, \(p_{\text{OS}}\) = 0.041).
Survival curves according to the Genomic Score in the training data (PACS04 trial). DDFS distant disease-free survival, OS overall survival N number of patients, N-event number of events, p log-rank p value. Low risk, MAP3K1 mutated. Moderate, MAP3K1 not mutated and FGFR1 not amplified. High risk, MAP3K1 not mutated and FGFR1 amplified
Validation of the genomic score in the external data (METABRIC trial)
In the external data set (METABRIC), we first checked the log-linearity hypothesis of continuous covariates (Supplementary Figure S5) and evaluated the prognostic value of FGFR1 copy numbers and MAP3K1 mutations on BCSS and OS. In univariable analysis (Fig. 7), FGFR1 copy numbers were significantly associated with the BCSS (\(p_{\text{BCSS}}\) < 0.001) and OS (\(p_{\text{OS}}\) < 0.001) whereas MAP3K1 mutations were only significantly associated with BCSS (\(p_{\text{BCSS}}\) < 0.01) but not with OS (\(p_{\text{OS}}\) = 0.28). In addition to CP parameters, FGFR1 copy numbers were significantly associated with BCSS (\({\text{HR}}_{\text{Amplification}}\) = 2.00, 95% CI [1.40; 2.87], p < 0.001) and OS (\({\text{HR}}_{\text{Amplification}}\) = 1.54, 95% CI [1.15; 2.07], p = 0.004); MAP3K1 mutations were strongly associated with BCSS (\({\text{HR}}_{\text{Mutation}}\) = 0.58, 95% CI [0.41; 0.83], p = 0.003) but were not significantly associated with OS (\({\text{HR}}_{\text{Mutation}}\) = 0.82, 95% CI [0.65; 1.02], p = 0.08) (Supplementary Table S7). FGFR1 and MAP3K1 added information (\(\chi_{\text{BCSS}}^{2}\) = 21.03, \(p_{\text{BCSS}}\) < 0.001 and \(\chi_{\text{OS}}^{2}\) = 10.36, \(p_{\text{OS}}\) = 0.005) to the CP model for BCSS and OS (Supplementary Table S8). The detail of the relative contribution of FGFR1 and MAP3K1 is given in Supplementary Table S9. The AUC values at 5 and 10 years for BCSS and OS were higher for CP + FGFR1 + MAP3K1 model (\({\text{AUC}}\left( {5\,{\text{years}}} \right)_{\text{BCSS}}\) = 74.47, \({\text{AUC}}\left( {10 \,{\text{years}}} \right)_{\text{BCSS}}\) = 73.35, \({\text{AUC}}\left( {5 {\text{years}}} \right)_{\text{OS}}\) = 71.77 and \({\text{AUC}}\left( {10 \,{\text{years}}} \right)_{\text{OS}}\) = 73.34) than CP model (\({\text{AUC}}\left( {5 {\text{years}}} \right)_{\text{BCSS}}\) = 74.12, \({\text{AUC}}\left( {10 \,{\text{years}}} \right)_{\text{BCSS}}\) = 72.24, \({\text{AUC}}\left( {5 \,{\text{years}}} \right)_{\text{OS}}\) = 71.29 and \({\text{AUC}}\left( {10\,{\text{years}}} \right)_{\text{OS}}\) = 72.66). The ROC curves are given in Supplementary Figures S6 and S7, and Table S10. Copy number alterations of FGFR1 gene increased the risk of death from BC whereas mutations of MAP3K1 decreased this risk. Applying our genomic score in the external data set, the Kaplan–Meier analysis indicated that it was significantly associated (\(p_{\text{BCSS}}\) < 0.001 and \(p_{\text{OS}}\) = 0.0015) with survival (Fig. 8). It provides additional information (\(n_{\text{BCSS}}\) = 1333, \(n{\text{ - event}}_{\text{BCSS}}\) = 392, \(\chi_{\text{BCSS}}^{2}\) = 15.39, \(p_{BCSS}\) < 0.001 and \(n_{OS}\) = 1333, \(n{\text{ - event}}_{\text{OS}}\) = 763, \(\chi_{\text{OS}}^{2}\) = 5.62, \(p_{\text{OS}}\) = 0.02) to CP model. The detail of CP + GS model is given in Supplementary Table S11. The results were consistent with the results found in the training data. The patients’ characteristics from the METABRIC study are described in supplementary Table S6. In the validation data set, the genomic score was validated on all patients with HR+ and HER2- BC without distinguishing patients with lymph node invasion to maximize statistical power. A sensitivity analysis was conducted looking only at the population of patients with lymph node-positive disease. In univariable analysis, the genomic score was significantly associated (\(p_{\text{BCSS}}\) < 0.01) with BCSS but less strongly with OS (\(p_{OS}\) = 0.07) (Supplementary Figure S8). In the multivariable analysis, in addition to CP parameters, it was strongly associated (HR = 1.25, 95% CI [1.04; 1.49], \(\chi_{\text{BCSS}}^{2}\) = 5.88, \(p_{\text{BCSS}}\) = 0.01) with the BCSS but to a lesser extent with OS (HR = 1.09, 95% CI [0.97; 1.2], \(\chi_{\text{OS}}^{2}\) = 1.91, \(p_{\text{OS}}\) = 0.2) (Supplementary Table S14 and Table S15). The details of CP + FGFR1 + MAP3K1 model are given in supplementary Table S12 and Table S13.
Survival curves according to the Genomic Score in the validation data (METABRIC trial). BCSS breast cancer-specific survival, OS overall survival, N number of patients. N-event number of events. p p-value associated with the log-rank test. Low risk, MAP3K1 mutated. Moderate, MAP3K1 not mutated and FGFR1 not amplified. High risk, MAP3K1 not mutated and FGFR1 amplified
Discussion
In HR+/HER2− BC, a key aim of precision medicine is to tailor adjuvant clinical management based on individual risk of relapse. This BC subtype is a heterogeneous disease based on genomic characteristics. We therefore performed a comprehensive analysis of a large population of patients with HR+/HER2− early BC, describing relevant genomic characteristics of these tumors and their associations with usual CP characteristics as well as their prognosis. The analysis indicated that FGFR1 copy number and MAP3K1 mutation added strong complementary information to CP features in determining the risk of distant relapse. FGFR1 amplification was identified as an independent risk factor while MAP3K1 mutation was associated with a protective effect. These results are consistent with previous studies [25, 26]. FGFR1 gene encodes a tyrosine kinase receptor that plays an important role in the development of BC and amplification of FGFR1 has oncogenic properties. Recently, it has been shown that activation of FGFR1 drives the invasive behavior of BC cells [25]. MAP3K1, a serine-threonine kinase, mediates apoptosis through activation of JNK pro-apoptosis protein; however, MAP3K1 mutations are associated with a low proliferation index and a low tumor grade [18].
In our analysis, ERBB2 amplification was not associated with DDFS in univariable analysis. This result was expected because our BC subgroup is HR-positive and HER2-negative. Although ZNF217 amplification was strongly associated with an increased risk of distant disease in the univariable analysis, it did not remain significant in the multivariable analysis. ZNF217 did not provide any information to CP + FGFR1 + MAP3K1 model, probably because it was slightly correlated with the tumor grade and mutational status of the MAP3K1 gene.
We retrieved the genomic part of the CP + FGFR1 + MAP3K1 model to create a genomic score. This score adjusted with CP features provided additional information to the initial CP model in determining the risk of death. The division of the score into three relevant categories made it possible to distinguish different levels of risk. Patients with a mutation of the MAP3K1 gene have a very good prognosis. Those with no alteration of FGFR1 and MAP3K1 genes have a moderate risk. And finally, those with an amplification of FGFR1 gene and no mutation of MAP3K1 gene have a higher risk. In the validation sample (METABRIC), the results were in line with the results found in the training sample (PACS04). FGFR1 amplification and MAP3K1 mutation were both associated with BCSS. Using the score that was established in the training data, the results showed that it added relevant information to the CP model in determining the risk of relapse (local and distant) and death. In the subset of patients with lymph node-positive disease in the METABRIC study, the genomic score continued to add additional prognostic information for BCSS.
However, our study has several limitations. In PACS04 trial, the sample size was relatively small with few events and the proliferation biomarker (Ki-67) was only available on a small subset of patients. In the METABRIC study, the DDFS endpoint was not available and the type of chemotherapy was not specified. We can also note that in the three prognostic groups generated, frequencies are relatively high in the training set (PACS04) and lower in the external validation data (METABRIC).
From a clinical standpoint, our study proposes a new way of classifying patients with HR+ and HER2- lymph node-positive BC based on known genes. Taking into account the copy number of FGFR1 and the mutational status of MAP3K1 improves the understanding of the prognosis and can help decision-making for the adjuvant treatment.
Conclusion
Currently, only CP parameters are taken into account to predict distant disease and guide HR+ and HER2− lymph node-positive BC adjuvant treatment. Our study showed that in addition to CP parameters, copy number alterations of FGFR1 gene and mutational status of MAP3K1 gene could improve distant disease prediction. FGFR1 amplification was associated with a high risk of distant disease whereas MAP3K1 mutation was associated with a low risk of distant disease.
The genomic score combining both genomic features classified patients into three subgroups with different prognosis: low risk (MAP3K1 mutated), moderate risk (MAP3K1 not mutated and FGFR1 not amplified), and high risk (MAP3K1 not mutated and FGFR1 amplified). Consideration of FGFR1 and MAP3K1 could be a new way to refine the treatment decision-making.
References
Howlader N, Altekruse SF, Li CI, Chen VW, Clarke CA, Ries LAG et al (2014) US incidence of breast cancer subtypes defined by joint hormone receptor and HER2 status. J Natl Cancer Inst. https://doi.org/10.1093/jnci/dju055
Cianfrocca M, Goldstein LJ (2004) Prognostic and predictive factors in early-stage breast cancer. Oncologist 9:606–616
Rugo HS (2008) The importance of distant metastases in hormone-sensitive breast cancer. Breast 17(Suppl 1):S3–S8
Rosano GMC (2007) The importance of distant metastasis and its impact on survival rates on early-stage hormone receptor-positive breast cancer. US Oncol Dis 1:22–25
Mohammadizadeh F, Hani M, Ranaee M, Bagheri M (2013) Role of cyclin D1 in breast carcinoma. J Res Med Sci 18:1021–1025
Roy PG, Pratt N, Purdie CA, Baker L, Ashfield A, Quinlan P et al (2010) High CCND1 amplification identifies a group of poor prognosis women with estrogen receptor positive breast cancer. Int J Cancer 127:355–360
Turner N, Pearson A, Sharpe R, Lambros M, Geyer F, Lopez-Garcia MA et al (2010) FGFR1 amplification drives endocrine therapy resistance and is a therapeutic target in breast cancer. Cancer Res 70:2085–2094
Drago JZ, Niemierko A, Spring L, Moy B, Juric D, Isakoff SJ et al (2017) FGFR gene amplification and response to endocrine therapy in metastatic hormone receptor positive (HR+) breast cancer. JCO 35:1013
Vendrell JA, Thollet A, Nguyen NT, Ghayad SE, Vinot S, Bièche I et al (2012) ZNF217 is a marker of poor prognosis in breast cancer that drives epithelial-mesenchymal transition and invasion. Cancer Res 72:3593–3606
Littlepage LE, Adler AS, Kouros-Mehr H, Huang G, Chou J, Krig SR et al (2012) The transcription factor ZNF217 is a prognostic biomarker and therapeutic target during breast cancer progression. Cancer Discov 2:638–651
Krig SR, Miller JK, Frietze S, Beckett LA, Neve RM, Farnham PJ et al (2010) ZNF217, a candidate breast cancer oncogene amplified at 20q13, regulates expression of the ErbB3 receptor tyrosine kinase in breast cancer cells. Oncogene 29:5500–5510
Kim Y-S, Won YS, Park KS, Song BJ, Kim JS, Oh SJ et al (2008) Prognostic significance of HER2 gene amplification according to stage of breast cancer. J Korean Med Sci 23:414–420
Borg A, Baldetorp B, Fernö M, Killander D, Olsson H, Sigurdsson H (1991) ERBB2 amplification in breast cancer with a high rate of proliferation. Oncogene 6:137–143
Carr JA, Havstad S, Zarbo RJ, Divine G, Mackowiak P, Velanovich V (2000) The association of HER-2/neu amplification with breast cancer recurrence. Arch Surg 135:1469–1474
Letessier A, Sircoulomb F, Ginestier C, Cervera N, Monville F, Gelsi-Boyer V et al (2006) Frequency, prognostic impact, and subtype association of 8p12, 8q24, 11q13, 12p13, 17q12, and 20q13 amplifications in breast cancers. BMC Cancer 6:245
Cizkova M, Susini A, Vacher S, Cizeron-Clairac G, Andrieu C, Driouch K et al (2012) PIK3CA mutation impact on survival in breast cancer patients and in ERα, PR and ERBB2-based subgroups. Breast Cancer Res 14:R28
Fernández-Cuesta L, Oakman C, Falagan-Lotsch P, Smoth K-S, Quinaux E, Buyse M et al (2012) Prognostic and predictive value of TP53 mutations in node-positive breast cancer patients treated with anthracycline- or anthracycline/taxane-based adjuvant therapy: results from the BIG 02-98 phase III trial. Breast Cancer Res 14:R70
Pham TT, Angus SP, Johnson GL (2013) MAP3K1: genomic alterations in cancer and function in promoting cell survival or apoptosis. Genes Cancer 4:419–426
Network Cancer Genome Atlas (2012) Comprehensive molecular portraits of human breast tumours. Nature 490:61–70
Roché H, Allouache D, Romieu G, Bourgeois H, Canon J, Serin D et al (2009) Five-year analysis of the FNCLCC-PACS04 trial: FEC100 vs ED75 for the adjuvant treatment of node positive breast cancer. Cancer Res 69:602
Curtis C, Shah SP, Chin S-F, Turashvili G, Rueda OM, Dunning MJ et al (2012) The genomic and transcriptomic architecture of 2,000 breast tumours reveals novel subgroups. Nature 486:346–352
Commo F, Guinney J, Ferté C, Bot B, Lefebvre C, Soria J-C et al (2016) rCGH: a comprehensive array-based genomic profile platform for precision medicine. Bioinformatics 32:1402–1404
Adélaïde J, Finetti P, Bekhouche I, Repellini L, Geneix J, Sircoulomb F et al (2007) Integrated profiling of basal and luminal breast cancers. Cancer Res 67:11565–11575
Blanche P. TimeROC: time-dependent ROC curve and AUC for censored survival data. https://cran.r-project.org/web/packages/timeROC/timeROC.pdf
Gelsi-Boyer V, Orsetti B, Cervera N, Finetti P, Sircoulomb F, Rougé C et al (2005) Comprehensive profiling of 8p11-12 amplification in breast cancer. Mol Cancer Res 3:655–667
Griffith OL, Spies NC, Anurag M, Griffith M, Luo J, Tu D et al (2018) The prognostic effects of somatic mutations in ER-positive breast cancer. Nat Commun 9:3476
Acknowledgements
We thank Yuki Takahashi for some editorial assistance.
Funding
This study was funded by grants from ANR and CGI (RHU MyPROBE and ANR-17-RHUS-0008) and Inca (PAIR SEIN14-049 IDF-ANDRE).
Author information
Authors and Affiliations
Corresponding author
Ethics declarations
Conflict of interest
The authors declare no relevant conflict of interest.
Ethical approval
All procedures performed in studies involving human participants were in accordance with the ethical standards of the institutional and/or national research committee and with the 1964 Helsinki declaration and its later amendments or comparable ethical standards.
Informed consent
Informed consent was obtained from all individual participants included in the PACS04 study.
Additional information
Publisher's Note
Springer Nature remains neutral with regard to jurisdictional claims in published maps and institutional affiliations.
Electronic supplementary material
Below is the link to the electronic supplementary material.
Rights and permissions
About this article
Cite this article
Carene, D., Tran-Dien, A., Lemonnier, J. et al. Association between FGFR1 copy numbers, MAP3K1 mutations, and survival in axillary node-positive, hormone receptor-positive, and HER2-negative early breast cancer in the PACS04 and METABRIC studies. Breast Cancer Res Treat 179, 387–401 (2020). https://doi.org/10.1007/s10549-019-05462-y
Received:
Accepted:
Published:
Issue Date:
DOI: https://doi.org/10.1007/s10549-019-05462-y