Abstract
This paper examines the impact on the life cycle greenhouse gas (GHG) emissions reduction when fossil-fueled ICE gasoline, diesel and natural gas vehicles, hybrid electric vehicles (HEVs) and plug-in hybrid electric vehicles (PHEVs) are banned in a step-by-step manner from 2035. We examine the impact of vehicle bans on life cycle GHG emissions and on the marginal cost (MC) of emissions reduction using four different scenarios defined by hydrogen production method, renewable energy share, and infrastructure development for refueling stations. The vehicle penetration and the fuel demand are determined by a consumer choice model characterized by a multinomial logit algorithm. Our analysis found that vehicle bans significantly promote battery electric vehicles (BEVs) for mini-sized vehicles and hydrogen fuel cell vehicles (FCVs) for light and heavy-duty vehicles. A vehicle ban that excludes BEVs and FCVs from 2035 under an enhanced infrastructure plan can reduce the life cycle GHG emissions as much as 438 million tonnes by 2060 compared to the 2017 level. The MC of the life cycle GHG mitigation decreases continuously and reaches as low as $482 per tonne CO2eq in 2060. However, if PHEVs are excluded from the ban, the life cycle GHG emissions are reduced more by 88 Mt-CO2eq in 2060 at a lower MC of $122 per tonne CO2eq. This is due to decreases in GHG emissions from VP where the replacement of PHEVs for BEVs and FCVs reduces the production of batteries and fuel cells.
Graphic abstract
The main structure of the model.

Similar content being viewed by others
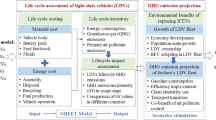
Avoid common mistakes on your manuscript.
Introduction
The transport sector in Japan contributed 19.2% of the nationwide greenhouse gas (GHG) emissions in 2017 reaching 248 million tonnes-CO2 equivalent (Mt-CO2eq) with the road transport alone generating 224 Mt-CO2eq (MOE 2019). On the basis of the Paris Agreement, GHG emissions from the transport sector are aimed to be cut to 163 Mt-CO2eq by 2030 which is a 34.3% reduction over 13 years (MOE 2016).
The principal policies for reducing GHG emissions from road transport by the Government are to promote low emission vehicles (LEVs) such as battery electric vehicles (BEVs), hybrid electric vehicles (HEVs), plug-in hybrid electric vehicles (PHEVs), natural gas vehicles (NGVs) and hydrogen fuel cell vehicles (FCVs) through low-emission vehicle rebates, the tax reduction under eco-car tax program and increasing the number of battery charging, natural gas and hydrogen refueling stations (ANRE 2016; METI 2016b; JGA 2017; NGVPC 2019b). Furthermore, hydrogen production alternatives and the target price of hydrogen for FCVs after 2030 have been proposed. After 2030, carbon dioxide and capture storage (CCS) is planned to be introduced for natural gas reforming as well as technology using renewables, and the hydrogen price for FCVs reduced from $0.95 per Nm3 ($10.58 per kg) in 2018 to $0.284 per Nm3 ($3.16 per kg) in 2030. The price is planned to be further reduced to $0.19 per Nm3 ($2.12 per kg) toward 2050 (ANRE 2019). Infrastructure development of natural gas and hydrogen refueling stations is also proposed, and a total of 900 hydrogen refueling stations and 1000 natural gas refueling stations are planned to be developed by 2030 (JGA 2019; METI 2019; NGVCR 2017).
Despite these promotion policies, the market penetration of LEVs has well below the roadmap targets of the Government. In 2018, there were 103,569 BEVs, 103,211 PHEVs and 2440 FCVs (AIRIA 2019; NGVPC 2019a). The Japanese Government targets for BEVs, PHEVs and FCVs are 0.7-1 million BEVs and PHEVs combined by 2020, and 0.2 million FCVs by 2025 with 0.8 million FCVs by 2030 (ANRE 2016; METI 2016a).
The previous study by Watabe et al. (2019) examined the impact of carbon tax and infrastructure development of battery charging and refueling stations on the market penetration of LEVs and the life cycle (LC) GHG emissions from 2016 to 2060 using the system dynamics simulation model of the Japanese energy systems. Vehicle shares are determined endogenously by using a multinomial logit algorithm, and the demand for vehicle fuels such as gasoline, diesel, natural gas, electricity and hydrogen is determined by the total number of vehicle fleets, fuel economy and annual distance travelled. It was found that infrastructure development with a carbon tax of $2.74 per tonne CO2 equivalent will reduce 29% tank-to-wheel (TtW) GHG emissions by 2030. Implementing a higher carbon tax of either $50 or $100 per tonne-CO2eq with an enhanced infrastructure development plan reduced TtW GHG emissions by 31% and the market share of BEVs, PHEVs and FCVs combined as 13% in 2030, being below the government roadmap targets. In 2060, the market shares of LEVs were estimated as 30% in all vehicles markets, leading to a TtW GHG emissions reduction of 68%, but GHG emissions from vehicle production (VP) growth of 50% up to 180% in 2060. As a result, LC GHG emissions can drop by 35%, compared with 2016 levels.
The major difference between the present paper and Watabe et al. (2019) is that different policy instruments are used to reduce GHG emissions. Watabe et al. (2019) focused on a carbon tax, while the present paper focuses on fossil-fueled vehicle bans combined with renewable fuel supports. Both papers are the first studies to endogenously model vehicle fleet propagation and associated GHG emissions within the context of the Japan’s electricity and hydrogen generation systems.
From a methodological perspective, the current study contributes to the previous study by Watabe et al. (2019) as follows. Firstly, the scope of the model has been extended by developing a new sector for natural gas reforming for hydrogen production to examine the difference from the green hydrogen produced by distributed solar electrolysis. Secondly, new recent data for the costs of electric vehicles are used for a careful analysis of transition cost. This is very important as the cost of batteries has declined significantly in recent years. Thirdly, the presented extensive scenario analysis, which has been performed for the first time for Japan, evaluates the transition process from a different perspective both by comparing supportive policies which enhance the supply of renewable fuels, and by using banning strategies which represent restraining policies banning the registration of new fossil fuel vehicles. Implementing a ban on registration of new fossil-fueled vehicles is consistent with some EU countries proposing banning internal combustion engine vehicles (ICEVs) of gasoline and diesel in the next ten to twenty years, as the European Union Parliament voted for a 40% CO2 emissions reduction from vehicle fleets by 2030 (EU 2018). The defined scenarios in the present analysis assume rapid transitions toward electric and hydrogen vehicles, caused by the fact that the elements of the vehicle choice set are no longer fixed over time. Few studies in the literature have defined these types of scenarios as target-driven or normative scenarios to explore an accelerated transition process (Riesz et al. 2016; Shafiei et al. 2017a, b). These scenarios are intended to assess the implications of transition to LEVs through stringent policies supporting a rapid shift to electricity and hydrogen fuels. The comparative analysis of simulation results has important information for policymakers on the cost and emissions implications of banning policies.
The rest of the paper is organized as follows. The next section investigates the related literature to the present paper. Section 3 discusses the model structure and assumptions, and Sect. 4 explains scenarios used in the analysis. Section 5 demonstrates and discusses the results of the analysis. The last section concludes the paper with policy recommendations.
Related literature
There are previous studies on the deployment of LEVs and GHG emissions in Japan. The previous studies have analyzed different policy measures other than a vehicle ban policy to see the impact on the share of LEVs and the resulting GHG emissions. Gonzalez Palencia et al. (2016) examined the diffusion of BEVs and fuel cell HEVs to reduce CO2 emissions using a vehicle stock turnover model. They focus on downsizing vehicles from normal-size to mini-sized vehicles (MVs) to improve fuel economy, materials usage and energy consumption during the vehicle production process. The market penetration of MVs is determined by exogenously defined growth rate of new vehicle sales based on the data from International Energy Agency (IEA) and the Japanese Ministry of Environment. They showed that BEVs share over 80% of light-duty passenger vehicles in 2050 and MVs share in light-duty passenger vehicles ranges from 47 to 91%, leading to a well-to-wheels (WtW) emissions reduction of 58–88% by 2050.
Yabe et al. (2012) project the market penetration rates of BEVs and PHEVs and the resulting CO2 emissions reduction from 2010 to 2050. They calculated the optimal share of BEVs and PHEVs and power generation sources using a learning curve for lithium-ion batteries to minimize the total cost of operating vehicles and power supply. BEVs and PHVs will share about 25% of the market in 2050, and CO2 emissions reduction is as much as 63% by 2050.
Using the AIM/Enduse, a bottom-up model which simulates a country’s CO2 emissions from energy and material flow, Oshiro and Matsui (2015) show that HEVs share over 50% of vehicles in 2050. If implementing a carbon tax scheme to achieve the 2 °C target, BEVs and FCVs share about 90% and 60% in passenger and freight transport, respectively, in 2050, reducing 81% of CO2 emissions compared to the 1990 level.
Vehicle bans have been studied for different countries other than Japan. Shafiei et al. (2017a) analyzed the rapid transition toward BEVs and FCVs by implementing a petroleum fuel vehicle ban from 2035 in Iceland. They found that the BEV transition would be the most cost-effective measure at reducing GHG emissions from energy supply and consumers. Shafiei et al. (2017b) also studied the case of a petroleum vehicle ban from 2030 in New Zealand and found that a fully decarbonized transport sector can be achieved by 2048. Since Iceland and New Zealand do not produce automobiles, VP GHG emissions are not considered.
System dynamics models have been applied in the vehicle analyses of transitioning to LEVs. Leaver and Gillingham (2010) evaluated the economic impact of hydrogen fuel cell and battery electric technologies on the economy of New Zealand using UniSyD_NZ. The deployment of FCVs in Korean was examined by a system dynamics simulation model (Park et al. 2011). Pasaoglu et al. (2016) examined powertrain technology transitions in light-duty passenger vehicles in the EU. A series of studies on transitioning to LEVs and the impact on GHG emissions reduction in Iceland and New Zealand were performed by Shafiei et al. (2014, 2018) using the system dynamics approach. These studies have proved that system dynamics is a useful tool to assess the transition process toward LEVs and the pathway of associated energy sectors as well as GHG emissions.
Methodology
We analyze the impact of banning fossil fuel vehicles on the market penetration of LEVs and the LC GHG emissions using the system dynamics simulation model, UniSyD_JP, which is primarily developed from the adaption and updating of the New Zealand model, UniSyD_NZ (Leaver et al. 2009, 2012) for the Japan’s energy economy. Japan’s energy and industrial sectors are quite different from New Zealand, and furthermore, unlike New Zealand, Japan is a major automobile manufacturing country and also Japan’s population is predicted to decline at an annual rate of 0.078% (IPSS 2019). These require significant modification and expansion of the model. Leaver et al. (2012) describe in detail the programming logic and assumptions for the 53 sectors and 13 regions of UniSyD_NZ, while UniSyD_JP consists of 108 sectors and 10 regions.
Model structure
The main structure of the UniSyD_JP model is shown in Fig. 1. The box inside the consumer’ vehicle choice includes the attributes which affect the vehicle choice. Fuel prices are determined by electricity, hydrogen and fossil fuels markets. Consumer’s vehicle choice determines vehicle stocks and GHG emissions from WtT, TtW and VP. Gross domestic product (GDP) and population growth affect the vehicle fleet growth and the electricity demand. The readers are referred to Watabe et al. (2019) for more information. The main difference of the present model from the previous study by Watabe et al. (2019) is threefold. Firstly, the elements of the vehicle choice set are no longer fixed and vary over time in order to analyze a vehicle ban policy. Secondly, the natural gas reforming for hydrogen production sector is newly constructed to examine the difference from green hydrogen produced by solar electrolysis. Thirdly, a learning curve of battery technology is modified based on Shafiei et al. (2019). UniSyD_JP simulates the transition process and equilibrium paths on all vehicle fleets, energy prices and GHG emissions from 2018 to 2060.
The model consists of energy sectors and vehicle sectors. Energy sectors include electricity, hydrogen and fossil fuels markets, which determine electricity, hydrogen and natural gas prices. Vehicle sectors include consumer’s vehicle choice and vehicle markets, which determine the demand for fuel for each vehicle fleet type and the market penetration of vehicles using the multinomial logit model. Vehicle fleets are classified by powertrain and weight into MVs, light-duty vehicles (LDVs) and heavy-duty vehicles (HDVs). Fuels used for ICEVs are gasoline, diesel and natural gas, while HEVs and PHEVs use either gasoline or diesel. HDVs are 3.5 tonnes and over in weight, and LDVs are less than 3.5 tonnes in weight. MVs are defined as an engine size of 0.66 L or less. MVs are not available for diesel and hydrogen. PHEVs using gasoline are not considered for HDVs. The battery sizes of PHEVs are 2 kWh, 8.8 kWh and 51 kWh for MVs, LDVs and HDVs, respectively. The battery sizes of BEVs are 16 kWh, 62 kWh and 324 kWh for MVs, LDVs and HDVs, respectively. The assumed battery sizes are the average of Japanese major automobile manufacturers.
Electricity market
The demand for electricity is driven by the demand of BEVs and PHEVs, hydrogen compression at refueling stations, as well as industrial, commercial and residential demand. The electricity demand of BEVs and PHEVs is determined by the number of these vehicles in the market, fuel economy and annual travel distance. The electricity demand of industrial, commercial and residential sectors is driven by the GDP.
Electricity is supplied by power generation using coal, natural gas, oil, nuclear and renewable energy. The ratio of energy sources is based on the marginal cost (MC) and the energy mix plan of the Government (ANRE 2018c). Technological development for coal and natural gas power generation is incorporated into the model with CCS, which is planned from 2030 with as much as 90% of CO2 capture (METI 2018). Renewable energy contributed 15% of the electricity supply in 2016, and it is planned to increase to 22–24% in 2030. The reduction in renewable electricity costs from 2030 to 2060 follows the projection in the Long-Term Energy Demand and Supply Outlooks (ANRE 2015). New renewable energy plants are constructed based on the long-term outlook of the potential capacity (ANRE 2017). The MC of power generation from each technology is estimated from the Long-Term Energy Demand Supply Outlook Committee of the Government (METI 2015a).
Nine nuclear reactors with 9130 MW are operating, and the remaining 36 reactors are waiting for the approval to resume operation (ANRE 2018b). Resuming operation requires meeting safety measures set by the Nuclear Regulatory Commission and approval from the prefecture where reactors are sited. Because of the uncertainties regarding nuclear power generation, we assume that the operating nine nuclear reactors will be decommissioned after a lifetime of 40 years.
Hydrogen market
It is assumed that hydrogen for FCVs is supplied by either natural gas reforming or solar electrolysis. Under the hydrogen fuel cell roadmap by the government, hydrogen for FCVs is produced by off-site natural gas reforming by 2030. After 2030, CCS will be introduced with natural gas reforming. The roadmap suggests that carbon-free technology for hydrogen production can be introduced after 2030, although no specific plans are addressed (ANRE 2016). The total supply cost of hydrogen in 2018 is $0.95 per Nm3 ($10.58 per kg), and it will be reduced to $0.284 per Nm3 ($3.16 per kg) in 2030. The cost is planned to further reduce to $0.19 per Nm3 ($2.12 per kg) toward 2050 (ANRE 2019).
As an alternative green hydrogen production scheme, we consider the case where hydrogen is produced by alkaline electrolysis with off-site solar power. The solar power facility is off-grid so that the electricity cost for hydrogen production is only associated with solar power generation technology. Meanwhile, hydrogen transported to a refueling station is compressed at the station where the electricity for hydrogen compression is from the grid network. The cost of solar power is $0.16 per kWh in 2017 and is expected to reduce to $0.05 per kWh in 2035 (ANRE 2018a).
Electricity consumption for electrolysis in 2018 is set as 4.1 kWh per Nm3 (45.66 kWh per kg) (NEL 2019). It is assumed that the electricity consumption for electrolysis in 2060 to reach the theoretical minimum of 3.54 kWh per Nm3 (39.42 kWh per kg) (EU 2014). We assume that hydrogen is compressed and dispensed at refueling stations at 700 bars with associated electricity consumption as low as 2.7 kWh per kg (NREL 2014).
Fossil fuel market
The demand for fossil fuels for vehicles such as gasoline, diesel and natural gas is determined by vehicle stock, the annual travel distance and fuel economy. The prices of gasoline, diesel and natural gas are estimated by an econometric analysis with an international oil price projected by World Energy Outlook 2017 (IEA 2017). Domestic reserves are used for natural gas, and reticulation cost is added to an estimated imported price.
Consumer vehicle choice
A consumer vehicle choice is characterized by a multinomial logit model which forecasts the probability that consumers purchase a specific vehicle, \( \pi_{it} \), as defined in Eq. (1). Consumer’s preference for a vehicle is defined by a utility function as in (2) that includes attributes of vehicle price, fuel cost, maintenance cost, battery replacement cost, driving range and fuel availability.
where subscripts denote a vehicle type i at time t; Pit is the vehicle price, Fit is the fuel cost, Mit is the maintenance cost, Bit is the battery replacement cost, Rit is driving range, Iit is the fuel availability, \( \varepsilon_{it} \) is the error term, and \( \alpha_{j} \) (j = 1,…,7) represent the consumer preferences on the attributes.
The vehicle fleet growth follows a modified Sigmoid curve (Leaver and Watabe 2016) where the upper limit of the Sigmoid curve is the maximum vehicle per capita as defined in Eq. (3):
where Vk t is the vehicles per capita for vehicle weight category k (k = MV, LDV and HDV) at time t, Vmax is the maximum achievable vehicles per capita, a is the fraction of room to increase vehicles per capita, and b is the growth rate of vehicles.
The Japanese population is predicted to decline continuously at an annual rate of 0.078%, with the number of registered vehicles predicted to continue to decline at a higher rate than the population (IPSS 2019; Nomura Research Institute 2015). Since 2011, LDVs and HDVs have decreased at annual rates of 0.65% and 0.86%, respectively, and MVs have increased at the annual rate of 0.19%. The overall number of vehicles per capita is expected to decrease continuously (JAMA 2017; MOE 2009).
The number of vehicles per capita in 2016 was 0.173, 0.36 and 0.0117 for MVs, LDVs and HDVs, respectively, with the shares of MVs, LDVs and HDVs being 27.2%, 55.5% and 17.3%, respectively (EDMC IEEJ 2017; AMA 2017). These data are used for the starting year of the simulation in 2018. The initial annual travel distances are 7475 km, 9120 km and 18,000 km for MVs, LDVs and HDVs, respectively (MLIT 2009).
Vehicle data
This subsection explains the data used for the attributes in the utility function given in (2). The purchase prices of gasoline ICEVs of MVs, LDVs and HDVs as the average prices of the three biggest automobile manufacturers in Japan: Toyota, Nissan and Honda, in 2018 are $13,265, $25,582 and $204,660, respectively. The prices of LDVs and HDVs other than gasoline ICEVs are set by the relative prices to a gasoline ICEV. For HDVs where the relative prices to the gasoline ICEVs are unavailable, we set the prices based on vehicle weights and the average engine size. For MVs other than gasoline ICEVs, prices are determined by additional costs to a gasoline ICEV (Yabe et al. 2012). The price of NGVs is based on Knittle (2012). The relative prices in 2060 are estimated by the reduction in the battery cost and other components.
The battery cost will decrease with a reduction rate of 6-9% from doubling of cumulative production to as low as $150 per kWh in 2025 (Nykvist and Nilsson 2015). The battery cost further reduces by 9% to $93.3 kWh in 2030 (NEDO 2015). It is assumed that the battery cost reduces to the lowest level of $75 per kWh in 2040, and there is no further reduction until 2060 (Shafiei et al., 2019).
The data on annual maintenance costs, driving range and fuel economy are shown in Tables 1 and 2.
Fuel availability is defined based on the number of refueling and recharging stations. Table 3 shows the initial cost and the annual running cost of different refueling stations. The initial cost and the running cost of each type of refueling stations are assumed to decrease annually to 2030; i.e., for hydrogen, 4.7% and 7.62%, respectively (ANRE 2016), for natural gas, 1.3% and 1.2%, respectively (JGA 2017), and for battery charging stations, 3% and 3%, respectively (METI 2016b, c).
Table 4 shows the number of refueling stations by 2030 proposed and planned by the Government (JGA 2019; METI 2019; NGVPC 2017). The proposed plan is based on the target market penetration of BEVs, PHEVs, FCVs and NGVs. 18,400 charging stations for BEVs and PHEVs in 2030 will achieve an average distance of 10 km between charging stations. There are 6920 fast charging stations for BEVs and PHEVs in 2016 where it takes 60 min to charge a 62 kWh battery capacity (Nissan 2019).
Table 5 shows the infrastructure development plan after 2030. Since there is no proposed government plan after 2030, we assume the number of refueling stations in 2060 based on the distance between the stations. The base plan is the same as the proposed government plan and the enhanced plan is for the case in which more refueling and recharging stations are necessary due to the banning of fossil-fueled vehicles. 23,000 charging stations in 2060 for BEVs serve a charging station for every 8 km distance, and 6100 refueling stations in 2060 for FCVs serve a refueling station every 30 km. It should be noted that under the scenario analysis below in Sect. 4, NGVs are banned after 2045 and refueling stations for NGVs are used until the end of NGVs’ life in 12–18 years. For the enhanced plan, the average distance between stations for BEVs, FCVs and NGVs is 5 km, 10 km and 10 km, respectively.
GHG emissions
GHG emissions from WtT, TtW and VP are estimated by their emission factors (Tables 6, 7, 8). For BEVs and FCVs, TtW value is zero and WtT depends on renewable energy share of electricity generation for BEVs and hydrogen produced by natural gas reforming either with or without CCS for FCVs. When producing hydrogen by solar electrolysis, GHG emissions are also generated because the LC GHG emission factor of solar technology production is considered. GHG emissions from electricity consumed by hydrogen compression at a refueling station are also counted. The GHG emission factor of electricity depends on the shares of renewables and fossil fuels, and it is endogenously calculated at each time step.
For an emission factor from VP, we use the data available in the literature. Ishizaki and Nakano (2018) and Kawamoto et al. (2019) estimated GHG emissions from production of batteries, engines, bodies and chassis parts. Sano et al. (2018) examined the LC GHG emissions of VP by focusing on the impact of recycling materials of retired vehicles on the reduction in GHG emissions. Yamada et al. (2005) estimated LC GHG emissions of FCVs.
We use the data available in these studies for LDVs to estimate the corresponding emission factors of VP for MVs and HDVs based on vehicle weight, battery sizes, motor weight, and fuel cell size. It is also assumed that the efficiency for VP with respect to GHG emissions is improved by 1% annually until 2030 which is the emission reduction target of VP from 2014 to 2030 by the automobile manufacturing industry (JAMA 2019). Expecting the emissions reduction to exhibit an exponential decrease, we assume that after 2030, the reduction will improve by a half of the present rate.
GHG emissions from battery production are also available in the literature. Kawamoto et al. (2019) show that GHG emissions from battery production are as low as 121 kg-CO2eq per kWh. On the other hand, Ishizaki and Nakano (2018) assumed that GHG emissions from battery production to be 100 kg-CO2eq per kWh to estimate the LC GHG emissions from LDVs. It is assumed that GHG emissions from battery production of 121 kg-CO2eq per kWh will be reduced to 100 kg-CO2eq per kWh by 2030. After 2030, the assumed improvement in battery technology (Shafiei et al. 2019) will improve the emissions reduction by 0.5% annually.
Main assumptions and limitation
Data and assumptions influence the results of simulation. WtT, TtW and VP GHG emission factors, the purchase price of gasoline ICEVs and infrastructure costs are based on data from the roadmap of the Government, the associated Agencies and the previous literature and are assumed to remain constant to 2060. All exogenous financial data is adjusted to the modelling datum of 2018 dollars. Predicted efficiency improvements and associated changes in emission factors in gasoline ICEVs after 2018 are included in the UniSyD_JP model. Gasoline, diesel and natural gas prices used in the analysis are estimated based on the projected international oil price by IEA (2017). Like these fossil fuel prices, the equilibrium path of the electricity supply–demand is affected by the projected coal and natural gas prices. Uncertainly regarding the international oil price affects the vehicle choice through fuel price. Learning curves of battery and solar electrolysis are modeled based on the previous studies from literature and official documents of the Government. Besides the data, powertrain, vehicle types and battery sizes for BEVs and PHEVs and fuel cells for FCVs remain unchanged so that these assumptions affect the rationale of consumer vehicle choice.
One way to remedy the limitation regarding the exogenous data is to construct additional sectors as the subset of UniSyD_JP to estimate time series values for the exogenous data such as the costs of batteries and electrolyzers. The construction of such modeling features needs a detail analysis of industrial production processes.
Scenario definition
We define five scenarios as shown in Table 9 based on the type of a vehicle to be banned, the start year of banning, hydrogen production method, the renewable share for electricity supply and refueling infrastructure development plan. We define the Government base plan as Business-as-Usual (BaU) in which no vehicle ban is planned. Hydrogen for FCVs is produced by natural gas reforming, and after 2030, CCS will be introduced. Renewable energy share for electricity generation in 2030 is targeted at 25%. It is assumed that renewable energy share can reach 50% in 2060.
Scenario 1 considers the case in which the both gasoline and diesel ICEVs ban is implemented from 2035 under BaU. A vehicle ban means that no new sale is admitted for a type of vehicles listed in a banning policy. Scenario 2 considers an aggressive case where MVs and LDVs of NGVs and gasoline and diesel HEVs are also banned from 2040 in addition to the banning scheme under Scenario 1. Furthermore, hydrogen is produced by alkaline electrolysis using off-grid solar electricity and the renewable energy share will be 100% by 2060. Infrastructure development is considered as the enhanced plan. Scenario 3 is more aggressive plan than Scenario 2 by imposing the ban on HDVs of NGVs as well as PHEVs from 2045.
Scenario 4 considers the extreme case where all vehicles except BEVs and FCVs are banned from 2035. Hydrogen production, electricity generation and infrastructure development are the same as defined in Scenarios 2 and 3.
Results and discussion
This section examines the impact of a fossil-fueled vehicle ban on the market penetration of vehicle fleets, the resulting GHG emissions from WtT, TtW, VP, the life cycle GHG emissions, the MC of the LC GHG emissions reduction and the cumulative LC GHG emissions reduction.
A fossil-fueled vehicles ban will reduce the demand for gasoline, diesel and natural gas. On the other hand, it will increase the consumption of electricity for BEVs and hydrogen for FCVs. Furthermore, the production of BEVs and FCVs generates more GHG emissions than other types of vehicles. The scenario analysis provides a detailed picture of how a fossil-fueled vehicle ban affects the market penetration of other vehicles. Furthermore, it will provide how the life cycle GHG emissions and the marginal cost of reducing life cycle GHG emissions will be changed from 2018 to 2060.
Vehicle market penetration
Figure 2 depicts the penetration of all types of vehicles classified in weight under BaU and Scenarios 1–4.
Under BaU, no vehicle ban is implemented while recharging and refueling stations for BEVs, FCVs and NGVs are developed under the Government base plan. Hydrogen for FCVs is produced by natural gas reforming combined with CCS after 2030. The share of renewables’ electricity generation is based on the energy mix of the Government Plan. The total number of MVs increases from 31.4 million in 2018 to 32.6 million in 2060. Gasoline ICEVs decrease continuously, but they still dominate the MV market with about 14 million vehicles and a share of 42.6% in 2060. BEVs and NGVs increase continuously to 1.5 million and 2.34 million in 2060, respectively.
Unlike MVs, the total number of LDVs decreases from 46.7 million in 2018 to 35.6 million in 2060. Gasoline ICEVs, diesel ICEVs and gasoline HEVs continue to decrease. On the other hand, the share of diesel HEVs among LDVs is less than 10%, exhibiting a slight increase until 2040 and then declining toward 2060. Despite the continuous decrease in gasoline ICEVs, there will be 923 million with the share of 26% in 2060. NGVs, PHEVs, BEVs, and FCVs increase continuously and reach 3.16 million, 9.2 million, 1.54 million and 3.52 million with the shares of 0.9%, 27.7%, 4.3% and 10% in 2060, respectively. The number of FCVs will be about 148,000 and 265,000 in 2025 and 2030, respectively, which fail to meet the corresponding government targets of 200,000 and 800,000, respectively.
The total number of HDVs decreases from 14.6 million in 2018 to 0.93 million in 2060. Diesel ICEVs have a significant decrease until 2040, but maintain the highest share of 28.5% in 2060. Gasoline ICEVs show a slight increase until 2043 and thereafter keep almost constant. NGVs, diesel PHEVs, BEVs and FCVs continue to increase. However, NGVs, BEVs and FCVs share only 9%, 0.3% and 2.3% in 2060, respectively. Diesel PHEVs share 12.7% in 2060, which is higher than the total share of NGVs, BEVs and FCVs.
Under Scenario 1, both gasoline and diesel ICEVs are banned from 2035. The ban reduces both gasoline and diesel ICEVs shares for MVs, LDVs and HDVs in a different manner. For MVs, after 2035, the ban expands the shares of gasoline HEVs and gasoline PHEVs at the annual growth rates of 2.7% and 4%, respectively. In 2060, gasoline HEVs and gasoline PHEVs share 31.4% and 38.4% in the MVs market, while BEVs share only 7.2% in 2060.
For LDVs, the ban decreases not only gasoline and diesel ICEVs but also gasoline HEVs. These three types of vehicles share 7.2%, 2.3% and 18.9% in 2060, respectively. After the ban, FCVs increase at the annual growth rate of 10.4% and share 14% in the LDVs market in 2060, while gasoline PHEVs share 24.8%. On the other hand, BEVs increase at the annual growth rate of 5% and a share stays only 6.1% in 2060.
For HDVs, the ban on both gasoline and diesel ICEVs makes all other types of vehicles increase. After the ban, FCVs exhibit the highest annual growth rate of 14.1%, being higher than NGVs of 4.3% and diesel PHEVs of 4.5%. However, FCVs’ share remains 3.8% in 2060, being far less than 14.6% of NGVs and 20.8% of diesel PHEVs.
Under Scenario 2, MVs and LDVs of NGVs, gasoline and diesel HEVs are banned from 2040 in addition to gasoline and diesel ICEVs banned in 2035. Hydrogen is produced by solar electrolysis, and an enhanced plan is introduced for infrastructure development. For MVs, gasoline PHEVs dominate and share 64% of the MVs market in 2060. BEVs increase over time, but the share remains 12.6% in 2060.
For LDVs, the market shares for gasoline and diesel PHEVs, BEVs and FCVs increase over time. FCVs expand at the highest annual growth rate of 12% after 2035 and share 26.2% of the LDV market in 2060. Meanwhile, both gasoline and diesel PHEVs grow at 4% annually, reaching combined share of 49.7% in 2060.
For HDVs, NGVs increase at the annual growth rate of 6.2% and share 23% of HDVs in 2060. FCVs grow at a faster rate than NGVs but share only 9.2% in 2060. Diesel PHEVs increase at a 6.2% growth rate, taking up the share of 32.5% in 2060.
Scenario 3 considers the case where an additional ban on PHEVs and HDVs of NGVs is implemented from 2045, and Scenario 4 considers the case where all vehicles except BEVs and FCVs are banned from 2035. Under both scenarios, the penetration of BEVs and FCVs is higher than other types of vehicles. However, if PHEVs are excluded from the ban, a sufficient penetration of BEVs and FCVs is not expected. Furthermore, even under Scenario 4, the penetration of BEVs for LDVs and HDVs is as much as about 20% and 5%, respectively (Fig. 3).
Life cycle GHG emissions
GHG emissions from WtT, TtW and VP perspectives depend on vehicle profiles depicted in Fig. 2, fuel consumptions and GHG emission factors. Figure 4 shows the life cycle GHG emissions which are the sum of the emissions from WtT, TtW and VP under BaU and Scenarios 1–4. The life cycle GHG emissions will be highest in Scenario 1 and lowest in Scenario 2.
Figure 5 depicts how the vehicle ban affects WtT GHG emissions compared to BaU for all Scenarios. Under Scenario 1, WtT GHG emissions are similar to BaU until 2042; thereafter, the WtT GHG emissions will be greater than BaU and continue to increase until 2060. This phenomenon is due to the uptake of FCVs with a higher penetration rate than BaU after 2036, while hydrogen is produced by natural gas reforming.
Under Scenarios 2 and 3, TtW GHG emissions are similar until 2042 but with lower emissions relative to BaU. After 2042, the emissions reduction in Scenario 2 is lower than Scenario 3. Because hydrogen is produced by solar electrolysis under both scenarios 2 and 3 so that the electricity supply for BEVs is a major factor for a change in WtT GHG emissions. The GHG emission factor of electricity depends on renewable energy share and decreases continuously from 0.48 to 0.14 kg-CO2eq per kWh based on WtT GHG emission factors of gasoline, diesel, and natural gas given in Table 6 and the BEV’s fuel economy determined endogenously in the model. BEVs result in generating more WtT GHG emissions per km than ICEVs running on gasoline, diesel, and natural gas. Therefore, the uptake of FCVs can effectively contribute to the reduction in WtT GHG emissions under Scenarios 2 and 3 compared to BaU.
Under Scenario 4, GHG emissions from WtT exceed BaU from 2036 to 2048. During this period, there is a rapid growth of BEVs as well as electricity consumption. After 2048, BEVs capture 60% of the MV market and FCVs capture 40% of both the LDV and HDV markets. Therefore, an increase in GHG emissions from electricity generation is offset by a reduction in GHG emissions from hydrogen production using solar electrolysis.
Figure 6 depicts total TtW GHG emissions in all scenarios compared to BaU. All scenarios generate less TtW GHG emissions than BaU. As banning policy is expanded from gasoline and diesel ICEVs to HEVs, PHEVs and NGVs, more TtW GHG emissions are mitigated.
Figure 7 shows the impact of a vehicle ban on GHG emissions from the VP aspect. BEVs, HEVs, PHEVs and FCVs require concomitant production of batteries, motors, fuel cell stacks, and other associated components so that additional energy will be consumed compared to ICEVs. Therefore, an earlier implementation of banning strategies leads to a higher GHG emissions from the VP perspective. There is less than one-year delay from vehicle sale to vehicle production.
Figure 8 depicts the impact of vehicle ban on the life cycle GHG emissions. Since TtW GHG emissions account for 59–77% of the life cycle GHG emissions from 2018 to 2060, the simulated trends are similar to those of TtW GHG emissions as presented in Fig. 6. Under Scenario 1, the life cycle GHG emissions will be reduced to as much as 11 Mt-CO2eq. Scenario 2 has the highest impact on the reduction in the life cycle GHG emissions and reduces the emissions by 37 Mt-CO2eq. The emissions reduction under Scenario 3 is almost equivalent to Scenario 2 until 2045. After 2045, the ban of PHEVs is implemented and Scenario 3 results in more emissions than Scenario 2 due to the production process batteries and fuel cells. Scenario 4 bans all vehicle types except BEVs and FCVs from 2035 and results in a significant penetration of BEVs and FCVs, as shown in Figs. 1 and 2, which needs more production of batteries and fuel cells and, thus, more GHG emissions from VP. Hence, from 2035 to 2039, the emissions are greater than BaU. After 2039, Scenario 4 is almost the same as Scenario 2.
Figure 9 depicts the impact of the four scenarios on the reduction in the cumulative life cycle GHG emissions. Scenario 1 reduces emissions by 0.19 billion tonnes-CO2eq (Bt-CO2eq) by 2060. Scenario 2 is the most effective policy providing cumulative mitigation of 0.51 Bt-CO2eq by 2060. The amounts of the cumulative emissions reduction in Scenario 3 and 4 are almost equivalent after 2052 at 0.43 Bt-CO2eq by 2060. Thus, the two-stage banning policy, that is, the ban of ICEVs from 2035 and the ban of HEVs and NGVs of MVs and LDVs from 2040 is the most effective strategy to reduce GHG emissions toward 2060.
Marginal cost of reducing life cycle GHG emissions
We examine the marginal cost of reducing life cycle GHG emissions by implementing vehicle ban under Scenarios 1–4. The marginal cost is defined as the changes in the cost of mitigating the life cycle GHG emissions with respect to BaU. The changes in the total cost include the capital costs of vehicles, maintenance cost, fuel expenditures and investment in infrastructure development.
Figure 10 shows the marginal cost of mitigation after 2040, and Fig. 11 scales up the graphs of Fig. 10 after 2046. The marginal costs in Scenarios 1 and 4 decrease continuously. There are two peaks of the marginal costs under Scenarios 2 and 3 in 2041 and 2046 caused by incremental capital expenditure on new vehicles in the next year after the start year of the banning policy. Consumers are not allowed to purchase vehicles banned which are less expensive than LEVs. Except for the peak points, the marginal costs continuously decrease until 2060.
Under Scenarios 1–4, the marginal cost reaches $390, $517, $2044 and $1238 per tonne-CO2eq, respectively, in 2046 and, thereafter, it decreases to − $56, $122, $541 and $482 per tonne-CO2eq, respectively, in 2060. The marginal cost is lowest in Scenario 1 and the highest in Scenario 3. The marginal cost in Scenario 4 is much higher than that in Scenario 2. The impact of both Scenario 2 and Scenario 4 on the life cycle GHG emissions reduction after 2040 is not much different but Scenario 4 incurs substantial costs in purchasing BEVs and FCVs. Under Scenario 3, a huge capital cost is born after banning PHEVs being replaced by BEVs and FCVs, and it can increase GHG emissions from VP. Under Scenario 1, the MC will be negative after 2056. This is caused by fuel savings outweighing an incremental capital cost over BaU and infrastructure development remains the same as the basic plan.
Conclusion and policy recommendations
We have examined the impacts of a fossil-fueled vehicle ban on the life cycle GHG emissions and assessed mitigation costs. BaU and four banning scenarios were defined to examine how the type of vehicle banned and the timing of ban affect the vehicle fleet profiles, the life cycle of GHG emissions, and the associated costs. The results of the analysis provide important policy implications for vehicle bans, infrastructure development for refueling and recharging stations, hydrogen production methods, and renewable energy shares.
Vehicle bans can significantly promote LEVs. However, the diffusion speed depends on when the bans start and what types of vehicles are candidates for a ban. In our analysis, a ban starts from 2035 and thus, BaU continues to until 2035. Under BaU to 2035, the Government fails to meet its emissions reduction target by 10% achieving only 24.3% for the period of 2017–2030 with a $50 per tonne carbon tax likely needed to achieve this target without selective vehicle banning (Watabe et al. 2019). Our analysis shows that the Government target of 0.8 million FCVs by 2030 will not be reached before 2036 under any ban policy and only banning PHEVs will provide the rapid growth of FCVs.
When ICEVs, HEVs and NGVs of HDVs are banned and PHEVs, BEVs and FCVs are permitted (Scenarios 1 and 2), the market penetration of BEVs and FCVs remains small to 2060, but PHEVs increase constitutes 70% of MVs, 50% of LDVs and 33% of HDVs by 2060. When PHEVs are added to the ban (Scenarios 3 and 4), BEVs and FCVs expand their shares in all vehicle weight categories. BEVs dominate the share of MVs, but shares of LDVs and HDVs are less than 20% and 5% in 2060, respectively. PHEVs are replaced by BEVs for MVs and FCVs for LDVs and HDVs. FCVs increase their share to as much as 60% in 2060 for both LDVs and HDVs in 2060.
A vehicle ban has different impacts on GHG emissions for WtT, TtW and VP. WtT GHG emissions comprise about 11–22% of total emissions and are influenced principally by the hydrogen production methods, and the proportions of electricity from the national grid. Emissions increase over BaU when hydrogen is produced by natural gas reforming. TtW GHG emissions comprise about 29–77% of total emissions. They are always less than BaU and reduced further as the vehicle ban policy becomes more stringent. VP GHG emissions comprise about 12–53% of total emissions, and they increase as the vehicle ban policy becomes more stringent. When all vehicles expect BEVs and FCVs are banned, TtW GHG emissions comprise 29% of total emissions in 2060 and VP GHG emissions comprise 53% of total emissions in 2060.
The vehicle ban policy is more effective in reducing the life cycle GHG emissions than a carbon tax of $50 to $100 per tonne-CO2eq when combined with accelerated infrastructure development plan. The life cycle GHG emissions reduction is about ten times higher than using a carbon tax of $50 per tonne to $100 per tonne introduced by Watabe et al. (2019). Banning gasoline and diesel ICEVs only reduces the life cycle GHG emissions about 4 times more than the case of a carbon tax of $50 to $100 per tonne-CO2eq. The marginal costs of the life cycle GHG emissions reduction are continuously decreasing and will be $122 per tonne-CO2eq and $541 per tonne-CO2eq in 2060 under Scenarios 2 and 3, respectively. On the other hand, the marginal costs of implementing carbon taxes of $50 per tonne-CO2eq and $100 per tonne-CO2eq will be about $220 per tonne-CO2eq to $250 per tonne-CO2eq from 2028 to 2034. Thereafter, they will increase rapidly exceeding over $1000 per tonne-CO2eq after 2044 and 2060, respectively (Watabe et al. 2019).
The impact on GHG emissions of policy instruments such as a carbon tax or vehicle bans is governed by the complex interactions of variables in the commercial, industrial and residential sectors that include consumer preferences and the economics of infrastructure and electricity and hydrogen production. In theory, a carbon tax as a market-based incentive changes consumers’ vehicle choice behavior through pricing. It is indirect regulation. The impact on GHG emissions reduction is less immediate than direct regulation. A vehicle ban policy is direct regulation that restricts consumers’ vehicle choice. The impact on GHG emissions reduction is more immediate than indirect regulation, but it is a blunt policy instrument that is more restrictive on consumer choice.
The analysis shows that the vehicle ban reduces the life cycle GHG emissions further and faster than the carbon tax of $50–$100 per tonne-CO2eq at less mitigation cost. In market-based instruments and direct regulations noted above, policies are evaluated in static theory that exclude the dynamic impact of associated changes in electricity and hydrogen production and pricing of technologies such as batteries and fuel cells. A dynamic analysis shows that the vehicle ban can contribute to a better environmental outcome and be more cost-effective than the carbon tax.
Concerning policy implementations, it should be noted firstly that a vehicle ban increases the life cycle GHG emissions for 3–5 years after vehicle bans start due to increased GHG emissions from both battery and fuel cell production. Secondly, banning all fossil fuel vehicles may not necessarily result in the lowest life cycle GHG emissions. Among the vehicle ban policies examined, banning all vehicles except NGVs of HDVs, PHEVs, BEVs and FCVs mitigates the most emissions and can reduce more than 4 Mt-CO2eq annually to the additional ban of NGVs of HDVs and PHEVs.
The marginal cost of the life cycle GHG emission is an important policy consideration. For the first ten years of the vehicle ban, 2035–2045, the more stringent the vehicle ban policy the higher is the mitigation cost. In particular, banning all vehicles except BEVs and FCVs from 2035 (Scenario 4) results in the marginal cost being 2-7.6 times higher than other ban cases. If the same policy is implemented from 2045 (Scenario 3), the marginal cost is $58 to $806 per tonne-CO2eq higher than cases defined in Scenarios 3 and 4.
The most durable policy to 2060 based on the marginal cost GHG emissions and the mitigation cost is to ban ICEVs, NGVs of MVs and LDVs, and HEVs from 2035 (Scenario 2) and to use distributed solar electrolysis for hydrogen production with incentives for enhancing infrastructure development. The vehicle ban policy can reduce the life cycle GHG emissions as much as 37 Mt-CO2eq annually, while using a carbon tax, $50 per tonne-CO2eq, only reduces emissions by as much as 3.5 Mt-CO2eq annually (Watabe et al. 2019).
In summary, a higher carbon tax can be effective until a vehicle ban is implemented, but does not need to remain high once the ban is implemented. The vehicle ban is strong regulation, and it needs the time for the industry to adjust strategies. We find that the best strategy is to ban all vehicle types except PHEVs, BEVs and FCVs in a stepwise fashion. Some fossil fuel use remains with PHEVs. PHEVs capture majority of all vehicle weight categories at 69% of MVs, 50% of LDVs and 33% of HDVs in 2060. For a carbon-free vehicle market, it is essential to reduce GHG emissions from the production process of batteries and fuel cells as well as reducing the costs associated with renewable energy supply and battery and fuel cell production.
Abbreviations
- BaU:
-
Business as usual
- BEV:
-
Battery electric vehicle
- Bt-CO2eq:
-
Billion tonnes-CO2 equivalent
- CCS:
-
Carbon capture storage
- FCV:
-
Hydrogen fuel cell vehicle
- GDP:
-
Gross domestic product
- GHG:
-
Greenhouse gas
- HDV:
-
Heavy-duty vehicle
- HEV:
-
Hybrid electric vehicle
- ICEV:
-
Internal combustion engine vehicle
- LC:
-
Life cycle
- LDV:
-
Light-duty vehicle
- LEV:
-
Low-emission vehicle
- MC:
-
Marginal cost
- Mt-CO2eq:
-
Million tonnes-CO2 equivalent
- MV:
-
Mini-sized vehicle
- NGV:
-
Natural gas vehicle
- PHEV:
-
Plug-in hybrid electric vehicle
- TtW:
-
Tank-to-wheel
- VP:
-
Vehicle production
- WtT:
-
Well-to-tank
- WtW:
-
Well-to-wheel
References
Agency for Natural Resources and Energy (ANRE) (2015) Long term energy demand and supply outlooks (in Japanese). ANRE Web. http://www.enecho.meti.go.jp/committee/council/basic_policy_subcommittee/mitoshi/cost_wg/006/pdf/006_05.pdf. Accessed 5 Sept 2018
Agency for Natural Resources and Energy (ANRE) (2016) Strategic roadmap to hydrogen and fuel cell (in Japanese)
Agency for Natural Resources and Energy (ANRE) (2017) Renewable energy: disclosure of information a (in Japanese). ANRE Web. https://www.fit-portal.go.jp/PublicInfoSummary. Accessed 15 Apr 2018
Agency for Natural Resources and Energy (ANRE) (2018a) On the acceleration for the reduction in cost of power generation (in Japanese). METI Web. https://www.meti.go.jp/shingikai/enecho/denryoku_gas/saisei_kano/pdf/008_02_00.pdf. Accessed 15 July 2018
Agency for Natural Resources and Energy (ANRE) (2018b) Status of nuclear power plants in japan (in Japanese). ANRE Web. www.enecho.meti.go.jp/category/electricity_and_gas/nuclear/001/pdf/001_02_001.pdf. Accessed 20 July 2018
Agency for Natural Resources and Energy (ANRE) (2018c) Provision for energy mix in 2030: whole disposition (in Japanese). ANRE Web. https://www.enecho.meti.go.jp/committee/council/basic_policy_subcommittee/025/pdf/025_008.pdf. Accessed 20 June 2019
Agency for Natural Resources and Energy (ANRE) (2019) Action plan of the Ministry of Economy, Trade and Industry about hydrogen and fuel cells (in Japanese). METI Web. https://www.meti.go.jp/shingikai/energy_environment/suiso_nenryo_dennchi_fukyu/pdf/006_01_00.pdf. Accessed 20 June 2019
Automobile Inspection and Registration Information Association (AIRIA) (2019) Statistics for registered vehicles (in Japanese). https://www.airia.or.jp/publish/statistics/number.html. AIRIA Web. Accessed 15 Sept 2019
Energy Data and Modelling Center The Institute of Energy Economics, Japan (EDMC IEEJ) (2017) World greenhouse gas emissions. In: handbook of Japan’s and world energy and economic statistics. The Energy Conservation Center, Japan, Tokyo
European Union (EU) (2014) Study on the development of water electrolysis in the European Union. The Fuel Cells and Hydrogen Joint Undertaking Web. https://www.fch.europa.eu/sites/default/files/study%20electrolyser_0-Logos_0_0.pdf. Accessed 10 Mar 2019
European Union (EU) (2018) Parliament pushed for cleaner cars on EU roads by 2030. European Parliament Web. https://www.europarl.europa.eu/news/en/press-room/20180925IPR14306/parliament-pushes-for-cleaner-cars-on-eu-roads-by-2030. Accessed 5 May 2019
Gl Pasaoglu, Harrison G, Jones L, Hill A, Beaudet A, Thiel C (2016) A system dynamics based market agent model simulating future powertrain technology transition: scenarios in the EU light duty vehicle road transport sector. Techno Forecast Soc Change 104:133–146. https://doi.org/10.1016/j.techfore.2015.11.028
Gonzalez Palencia JC, Araki M, Shiga S (2016) Energy, environmental and economic impact of mini-sized and zero-emission vehicle diffusion on a light-duty vehicle fleet. Appl Energy 181:96–109. https://doi.org/10.1016/j.apenergy.2016.08.045
International Energy Agency (IEA) (2017) Global energy & CO2 status report 2017. https://www.connaissancedesenergies.org/sites/default/files/pdf-actualites/geco2017.pdf. Accessed 25 May 2019
Ishizaki K, Nakano M (2018) The environmental performance of current mass-produced passenger vehicles in Japan (in Japanese). Trans JSME. https://doi.org/10.1299/transjsme.18-00050
Japan Automobile Manufacturers Association (JAMA) (2019) CO2 emissions from vehicle production (in Japanese). JAMA Web. www.jama.or.jp/eco/earth/earth=05=g01.html. Accessed 1 Mar 2019
Japan Gas Association (JGA) (2017) Toward promotion of natural gas vehicles: 2017-2018 (in Japanese). JGA, Tokyo
Japan Gas Association (JGA) (2019): Penetration of natural gas vehicles. (in Japanese). https://www.gas.or.jp/ngvj/spread/index.html. Accessed 10 Apr 2019
Japan LP Gas Association (JLPGA) (2017) List of caloric values and CO2 emissions of fuels (in Japanese). http://www.j-lpgas.gr.jp/nenten/data/co2_list.pdf. JLPGA Web. Accessed 5 Mar 2019
Japanese Ministry of Economy, Trade and Industry (METI, Automobile Division Manufacturing Industries Bureau) (2016a) Policy measures for EV and PHV (in Japanese)
Japanese Ministry of Economy, Trade and Industry (METI) (2015a) Long-term energy demand supply outlook: evaluation on electricity generation cost (in Japanese), Report for Advisory Committee for Energy and Resources
Japanese Ministry of Economy, Trade and Industry (METI) (2015b) Top-runner system (in Japanese). https://www.enecho.meti.go.jp/category/saving_and_new/saving/data/toprunner2015j.pdf. Accessed 11 May 2019
Japanese Ministry of Economy, Trade and Industry (METI) (2016b) Expenses for infrastructure development for promoting the streamlining of energy utilization: report on infrastructure of EV PHV charging stations (in Japanese)
Japanese Ministry of Economy, Trade and Industry (METI) (2016c) Report of EV and PHV roadmap (in Japanese)
Japanese Ministry of Economy, Trade and Industry (METI) (2016d): Roadmap on technology for the next generation thermal power generation (in Japanese). NDL Web. https://warp.da.ndl.go.jp/info:ndljp/pid/10159415/www.meti.go.jp/press/2016/06/20160630003/20160630003-1.pdf. Accessed 5 Apr 2018
Japanese Ministry of Economy, Trade and Industry (METI) (2018) Expert committee for the ideal way of demonstration and research project on CCS (in Japanese). METI Web. http://www.meti.go.jp/committee/kenkyukai/sangi/ccs_jissho/pdf/001_05_00.pdf. Accessed 20 Sept 2018
Japanese Ministry of Economy, Trade and Industry (METI) (2019) Operating cost for promoting clean energy vehicles: subsidies (in Japanese). METI Web. https://www.meti.go.jp/main/yosangaisan/fy2019/pr/en/seizou_taka_03.pdf. Accessed 20 July 2019
Japanese Ministry of Environment (MOE) (2009) Trend of automobiles development and forecast of market penetration (in Japanese). MOE Web. https://www.env.go.jp/air/report/h21-01/2.pdf. Accessed 6 Apr 2019
Japanese Ministry of Environment (MOE) (2016) Pledged draft on greenhouse gas emissions (in Japanese). MOE Web. https://www.env.go.jp/earth/ondanka/ghg/mat01_indc.pdf. Accessed 10 May 2019
Japanese Ministry of Environment (MOE) (2019) Greenhouse gas emissions in fiscal year 2017 (in Japanese). MOE Web. https://www.env.go.jp/earth/ondanka/ghg-mrv/emissions/results/honbun2017-2.pdf. Accessed 25 June 2019
Japanese Ministry of Land, Infrastructure, Transport and Tourism (MLIT) (2009) Automobile transportation statistical yearbook 2009 (in Japanese). MLIT Web. https://www.e-stat.go.jp/stat-search/files?page=1&layout=datalist&toukei=00600330&kikan=00600&tstat=000001078083&cycle=8&year=20091&month=0&stat_infid=000031848854&result_back=1&tclass1val=0. Accessed 10 June 2019
Japanese Ministry of Land, Infrastructure, Transport and Tourism (MLIT) (2018) Automobile fuel consumption yearbook 2018 (in Japanese). MLIT Web. https://www.e-stat.go.jp/stat-search/files?page=1&layout=datalist&toukei=00600370&kikan=00600&tstat=000001051698&cycle=8&result_page=1&tclass1val=0. Accessed 10 June 2019
Kawamoto R, Mochizuki H, Moriguchi Y, Nakano T, Motohashi M, Sakai Y, Inaba A (2019) Estimation of CO2 emissions of internal combustion engine vehicle and battery electric vehicle using LCA. Sustainability. https://doi.org/10.3390/su11092690
Knittle C (2012) Leveling the playing field for natural gas in transportation. Discussion Paper 2012-03, The Hamilton Project, Brookings, Washington, DC
Leaver J, Gillingham K (2010) Economic impact of the integration of alternative vehicle technologies into the New Zealand vehicle fleet. J Clean Prod 18:908–916. https://doi.org/10.1016/j.clepro.2009.10.018
Leaver J, Watabe A (2016) Transition to hydrogen fuel cell and battery electric vehicle fleets in Japan and New Zealand. In: Proceedings of the 21st world hydrogen energy conference. Zaragoza, Spain
Leaver J, Gillingham K, Leaver L (2009) Assessment of primary impacts of a hydrogen economy in New Zealand using UniSyD. Int J Hydrogen Energy 34(7):2855–2865. https://doi.org/10.1016/j.ijhydene.2009.01.063
Leaver J, Leaver L, Gillingham K, Bagllino A (2012) A systems dynamics modelling of New Zealand’s energy economy: a guide to UniSyD 5.1. https://books.google.co.nz/books/about/System_Dynamics_Modelling_of_New_Zealand.html?id=GzZQMwEACAAJ&redir_esc=y
Mizuho Information and Research Institute, Inc (2016) Evaluation report on life cycle greenhouse gas emissions from hydrogen (in Japanese). Mizuho IR Web. https://www.mizuho-ir.co.jp/publication/report/2016/pdf/wttghg1612.pdf. Accessed 1 June 2019
National Institute of Population and Social Security Research (IPSS) (2019) 2019 Statistical information on population (in Japanese) IPSSWeb. http://www.ipss.go.jp. Accessed 1 May 2019
National Renewable Energy Laboratory (NREL) (2014) Hydrogen station compression, storage, and dispensing technical status and costs. Technical Report. NREL/BK-6A10-58564, Golden, Colorado
NEL (2019) The World’s most efficient and reliable electrolysers. Energy Storage Europe Web. https://www.eseexpo.de/vis-content/event-energy2019/exh-energy2019.2621590/Energy-Storage-Europe-2019-Nel-Hydrogen-Paper-energy2019.2621590-kPvvuuRHSyuOm7zdR0AkdA.pdf. Accessed 20 Sept 2019
New Energy and Industrial Technology Development Organization (NEDO) (2015) Toward the formulation of technical strategies for the field of vehicle storage batteries (in Japanese). TSC Foresight 5:1–21
Next Generation Vehicle Promotion Center (NGVPC) (2017) Trends in infrastructure. NGVPC Web. http://www.cev-pc.or.jp/english/infrastructure.html. Accessed 7 Aug 2019
Next Generation Vehicle Promotion Center (NGVPC) (2019a) EV and PHEV Statistics. NGVPC Web. http://www.cev-pc.or.jp/tokei/hanbai.html. Accessed 7 Aug 2019
Next Generation Vehicle Promotion Center (NGVPC) (2019b) Clean vehicle rebates (in Japanese). NGVPC Web. http://www.cev-pc.or.jp/hojo/pdf/h31/H31_meigaragotojougen.pdf. Accessed 7 Aug 2019
Nissan Motor Corporation (2019) Battery charging and driving range of Nissan Leaf (in Japanese). Nissan Web. https://www3.nissan.co.jp/vehicles/new/leaf/charge.html. Accessed 1 Oct 2019
Nomura Research Institute (NRI) (2015) The registered passenger vehicles decline outweighs the number of households decreasing by 9% after 15 years (in Japanese). NRI Web. https://www.nri.com/-/media/Corporate/jp/Files/PDF/news/newsrelease/cc/2015/150605.pdf. Accessed 1 June 2019
Nykvist B, Nilsson M (2015) Rapidly falling costs of battery packs for electric vehicles. Nat Clim Change 5:329–332. https://doi.org/10.1038/nclimate2564
Oshiro K, Matsui T (2015) Diffusion of low emission vehicles and their impact of CO2 emission reduction in Japan. Energy Policy 81:215–225. https://doi.org/10.1016/j.enpol.2014.09.010
Park S, Kim J, Lee D (2011) Development of a market penetration forecasting model for hydrogen fuel cell vehicles considering infrastructure and cost reduction effects. Energy Policy 39:3307–3315. https://doi.org/10.1016/j.enpol.2011.03.021
Riesz J, Sotiriadis C, Amback D, Donovan S (2016) Qualifying the costs of a rapid transition to electric vehicles. Appl Energy 180:287–300. https://doi.org/10.1016/j.apenergy.2016.07.131
Sano K, Tomioka K, Ooi Y (2018) LCA study of automobile recycling: CO2 reduction by recycling of each material (in Japanese). Trans Soc Automot Eng Jpn 49:845–848. https://doi.org/10.11351/jsaeronbun.49.845
Shafiei E, Davidsdottir B, Leaver J, Stefansson H (2014) Potential impact of transition to a low-carbon transport system in Iceland. Energy Policy 69:127–142. https://doi.org/10.1016/j.enpol.2014.03.013
Shafiei E, Davidsdottir B, Leaver J, Stefansson H, Asgeirsson EI (2017a) Energy, economic, and mitigation cost implications of transition toward a carbon-neutral transport sector: a simulation-based comparison between hydrogen and electricity. J Clean Prod 141:237–247. https://doi.org/10.1016/j.clepro.2016.09.064
Shafiei E, Davidsdottir B, Leaver J, Stefansson H, Asgeirsson EI (2017b) Cost-effectiveness analysis of inducing green vehicles to achieve deep reductions in greenhouse gas emissions in New Zealand. J Clean Prod 150:339–351. https://doi.org/10.1016/j.jclepro.2017.03.032
Shafiei E, Davidsdottir B, Fazeli R, Leaver J, Stefansson H, Asgeirsson EI (2018) Macroeconomic effects of fiscal incentives to promote electric vehicles in Iceland: implications for government and consumer costs. Energy Policy 114:431–443. https://doi.org/10.1016/j.enpol.2017.12.034
Shafiei E, Davidsdottir B, Stefansson H, Asgeirsson EI, Fazeli R, Gestsson MH, Leaver J (2019) Simulation-based appraisal of tax-induced electro-mobility promotion in Iceland and prospects for energy-economic development. Energy Policy 133:110894. https://doi.org/10.1016/j.enpol.2019.110894
Toyota Motor Corporation (2004) Well-to-wheel analysis of greenhouse gas emissions of automotive fuels in the japanese context- Well-to-Tank Report
Watabe A, Leaver J, Ishida H, Shafiei E (2019) Impact of low emissions vehicles on reducing greenhouse gas emissions in Japan. Energy Policy 130:227–242. https://doi.org/10.1016/j.enpol.2019.03.057
Yabe K, Shinoda Y, Seki T, Tanaka H, Akisawa A (2012) Market penetration speed and effects on CO2 reduction of electric vehicles and plug-in electric vehicles in Japan. Energy Policy 45:529–540. https://doi.org/10.1016/j.enpol.2012.02.068
Yamada Y, Ariyama Y, Ino H, Harada K (2005) Life cycle assessment (Energy consumption and CO2 emission) of fuel cell vehicle in comparison with gasoline and natural gas vehicles (in Japanese). J Jpn Inst Metals 69:237–240
Acknowledgements
The UniSyD_JP model was developed while the authors were visiting Unitec Institute of Technology (Unitec), New Zealand. We would like to thank Unitec for financial support and generous hospitality while visiting Unitec.
Author information
Authors and Affiliations
Corresponding author
Ethics declarations
Conflicts of interest
The authors declare that they have no known competing financial interests or personal relationships that could have appeared to influence the work reported in this paper.
Additional information
Publisher's Note
Springer Nature remains neutral with regard to jurisdictional claims in published maps and institutional affiliations.
Rights and permissions
About this article
Cite this article
Watabe, A., Leaver, J., Shafiei, E. et al. Life cycle emissions assessment of transition to low-carbon vehicles in Japan: combined effects of banning fossil-fueled vehicles and enhancing green hydrogen and electricity. Clean Techn Environ Policy 22, 1775–1793 (2020). https://doi.org/10.1007/s10098-020-01917-9
Received:
Accepted:
Published:
Issue Date:
DOI: https://doi.org/10.1007/s10098-020-01917-9