Abstract
This study primarily investigated the forecasting of the growth trend in renewable energy consumption in China. Only 22 samples were acquired for this study because renewable energy is an emerging technology. Because historical data regarding renewable energy were limited in sample size and the data were not normally distributed, forecasting methods used for analyzing large amounts of data were unsuitable for this study. Grey system theory is applied to system models involving incomplete information, unclear behavioral patterns, and unclear operating mechanisms. In addition, it can be used to perform comprehensive analyses, observe developments and changes in systems, and conduct long-term forecasts. The most prominent feature of this theory is that a minimum of only four data sets are required for establishing a model and that making stringent assumptions regarding the distribution of the sample population is not required. However, to address the limitations of previous studies on grey forecasting and to enhance the forecasting accuracy, this study adopted the grey model (1, 1) [GM(1, 1)] and the nonlinear grey Bernoulli model (1, 1) [(NGBM)] for theoretical derivation and verification. Subsequently, the two models were compared with a regression analysis model to determine the models’ predictive accuracy and goodness of fit. According to the indexes of mean absolute error, mean square error, and mean absolute percentage error, NGBM(1, 1) exhibited the most accurate forecasts, followed by GM(1, 1) and regression analysis model. The results indicated that the modified NGBM(1, 1) grey forecasting models demonstrated superior predictive abilities among the compared models.
Similar content being viewed by others
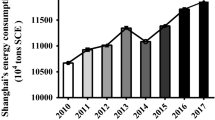
Avoid common mistakes on your manuscript.
Introduction
Generally, green energy includes solar, hydraulic, wind, marine, and geothermal energy (Bardi et al. 2013). Renewable energy is advantageous in that it induces a low level of pollution (Bartłomiej et al. 2015); however, it is constrained by natural conditions (e.g., requiring resources such as hydraulic, wind, and solar energy) (Deborah et al. 2013). Furthermore, generating electricity through renewable energy is expensive because investment and maintenance expenses involved are substantial, yet production efficiency is relatively low (Farah et al. 2013).
Numerous scientists are seeking new technologies and methods for improving renewable energy (Akay et al. 2013). Several studies have indicated that renewable energy is certain to play an increasingly critical role as earth’s resources are depleted (Zhang et al. 2014).
McLellan et al. (2012) and Prakash et al. (2013) have reported that renewable energy offers the following advantages over conventional energy:
-
(a)
Renewability Conventional forms of primary energy, such as coal, petroleum, and natural gas, are formed through long geological processes. Earth has limited reserves of these forms of energy (Federica and Idiano 2015). Rahim and Victoria (2015) have reported that based on the current mining capacity, these forms of energy will ultimately become exhausted. People must seek and use sustainable energy sources that cannot be exhausted to replace these conventional forms of energy before their depletion. New forms of renewable energy, such as wind energy, solar energy, and biomass energy, are ideal alternatives.
-
(b)
Cleanliness The use of conventional energy leads to deadly pollution and environmental destruction (Farhad et al. 2015).
China surpassed the United States as the number one consumer of primary energy in 2010 and retained this position in both 2011 and 2012, truly becoming a major energy consumer. In 2012, the annual growth in Chinese petroleum consumption was 5.3 %, which was once again the highest increase in petroleum consumption globally. Chinese coal consumption also constituted 50.2 % of global coal consumption in 2012; this marked the first time China exceeded 50 % of global coal consumption. China’s primary energy consumption structure remains focused on coal. In 2012, coal constituted 67.8 % of the Chinese primary energy consumption, followed by petroleum at 18.2 % and natural gas at 4.8 %. Renewable energy constituted 9.2 % of the Chinese energy consumption (China Energy Statistical Year book 2013).
Chinese economy is currently the second largest economy in the world. It also consumes the most energy and emits the most greenhouse gases. China’s energy efficiency remains poor. With global climate change, environmental degradation, and shortages of conventional energy, a general consensus worldwide has been developed regarding the necessity of renewable energy. Following trends in environmental protection and the depletion of oil reserves, renewable energy has also become the core focus of China’s energy policy. In 2012, the 12 Five-Year Plan for National Economic and Social Development of the People’s Republic of China was presented. This plan included concrete indicators for renewable energy, projecting renewable energy consumption to reach approximately 10 % of the total energy consumption by 2015 and approximately 15 % of the total energy consumption by 2020 (Zhao et al. 2014).
Forecasting trends in renewable energy in China is extremely crucial. If trends in Chinese renewable energy consumption can be predicted accurately, then the development of renewable energy in China and even the entire world can be grasped. In addition, forecasting trends in renewable energy is a major part of green production.
This study forecasted growth trends in renewable energy consumption in China. Because historical data on renewable energy were limited in sample size and because the data were not normally distributed, forecasting methods used for analyzing large amounts of data (such as conventional regression analysis, neural networks, and genetic algorithms) were unsuitable for this study.
Grey system theory is applicable to system models with incomplete information, unclear behavior, and unclear operating mechanisms (Deng 2003). It can be used to perform a comprehensive analysis, observe developments and changes in systems, and conduct long-term forecasts (Deng 2004). The most outstanding feature of grey system theory is that it can be used to establish models with a minimum of only four data samples. Furthermore, the distribution of sample populations does not require making numerous stringent assumptions. Numerous studies have indicated that the original grey model (1, 1) [GM(1, 1)] has an extremely high predictive accuracy when data from small samples were used (Chen and Chen 2011).
Numerous studies have argued that GM(1, 1) is highly accurate only when the experimental sample data exhibit steady growth trends. Li et al. (2009) and Lee et al. (2014) have reported that when sample data demonstrated large fluctuations, GM(1, 1) required revision to increase its predictive accuracy. Such revised models include the nonlinear Bernoulli modification model and Markov modification model.
To improve the weak points of previous studies and to increase the predictive accuracy, this study used the GM(1, 1) model along with the revised nonlinear grey Bernoulli model (NGBM) to perform theoretical derivation and scientific verification. This study compared the results of the grey models with those of a regression analysis model to confirm the predictive accuracy and fitness of these three methods. This study determined the optimal prediction model among the three models. Finally, according to the derived results, we present a conclusion and suggestions for future research.
Literature review
Grey system theory and grey prediction
Herbig et al. (1993) stated that prediction is used to estimate future events or situations that organizations cannot control and to provide managers with a basis for planning. Therefore, prediction is vital in decision-making processes.
Deng (1982) proposed grey system theory, which can be used to perform relational analysis and model construction in ambiguous system models with incomplete information. Using grey system theory entails employing prediction and decision-making methods to investigate and understand system conditions. In grey system theory, black is used to represent a lack of information, white is used to represent complete information, and grey is used to represent insufficient or incomplete information. Kwonpongsagoon et al. (2007) indicated that Deng originally applied grey system theory to control areas, but subsequent scholars have developed and applied it to other fields, such as management decisions, socioeconomic research, and meteorological and water conservation forecast.
Grey system theory can be used to effectively process uncertainty, multivariate inputs, discrete data, and incomplete data (Chang et al. 2013). Grey theory is applicable to small, uncertain samples. The applications of grey theory differ from those of probability (statistics), which is used to analyze large, uncertain samples, and fuzzy theory, which is used to analyze cognitive uncertainty (Sadeghi et al. 2013).
According to the classifications by Xiong et al. (2010), Chang (2012), and Lee et al. (2014), studies on grey system theory can be divided into the following classes: (1) grey generating techniques, (2) grey relational analysis, (3) grey model construction, (4) grey prediction (5), grey decision making, and (6) grey control.
In recent years, grey forecasting has been applied to various fields, including management decisions, socioeconomic research, meteorological and water conservation forecasting, and disaster prevention. For example, Hu (2004) used GM(1, 1) to establish a forecast model to help consumers make optimal choices when purchasing new cars. Consumers must enter only their preferred brand, price, safety, functionality, and fuel consumption data to make optimal choices. Wang (2010) used an improved GM(1, 1) to forecast the number of Hong Kong, U.S., and German tourists who visited Taiwan between 1989 and 2000. The conclusion of this study was that the predictive accuracy of the improved GM(1, 1) was extremely high. Furthermore, because large samples were not necessary, the improved model greatly reduced the cost and time required for data collection. Kayacan et al. (2010) used the NGBM and grey Markov model to forecast the U.S. dollar and euro exchange rate between 2005 and 2007. The results of this study indicated that the models had high accuracy when the experimental sample data showed steady growth trends. Akay et al. (2013) used various models to forecast electricity demand in Turkey and observed that grey theory demonstrated the most satisfactory predictions. Lee et al. (2014) used grey system models and fuzzy time series to forecast trends in electrochromic electronic materials. The results indicated that the revised GM(1, 1) was the optimal forecasting model for small samples.
The aforementioned studies have reflected the following advantages of grey prediction: (1) Grey prediction does not require substantial amounts of historical data. The amount of data can be selected according to actual conditions and requirements. In general, forecasting models can be established with no less than four data pieces. (2) Grey prediction does not require the use of numerous associated factors. Data are easy to obtain, considerably reducing the time and cost of collecting data. (3) Grey prediction is extremely accurate.
Researchers in previous related studies have focused primarily on using one or two grey prediction methods to improve the accuracy of their predictions. However, the same methods may not be appropriate for sample data obtained from different industries. Therefore, to improve the weak points of previous studies and to increase the predictive accuracy, this study used the GM(1, 1) model and the revised NGBM(1, 1) model for theoretical derivation and verification. In addition, this study performed a comparison using regression analysis to assess the advantages and disadvantages of grey prediction with small samples and to confirm the predictive accuracy and goodness of fit of the three models. Finally, this study determined the optimal prediction model among the three models.
Methodology
GM(1, 1)
GM(1, 1) is a model used in grey theory for performing forecasts. It expresses a first-order differential and has a single input variable. (Chang 2012). Grey prediction is a method of performing forecasts with existing data based on GM(1, 1). It is used to investigate the future dynamic conditions of numerous elements within a series (Avinash et al. 2012).
The primary advantage of grey prediction is that it does not entail using much data and its mathematical foundation is simple. Because the GM(1, 1) requires as little as four data pieces, rolling checks in series with at least four data pieces can be used to test the reliability of the prediction model. In addition, the residuals of the predicted and actual values reflect the reliability of the prediction model. Lower residuals reflect greater reliability.
In this study, series prediction, which is the direct establishment of grey prediction models according to given information, was addressed. The steps for establishing GM(1, 1) for grey prediction are outlined as follows:
(a) First, define the given data as the original series:
(b) Use a single AGO to sum the established original series. The following generating sequence is obtained:
where
(c) Establish the GM(1, 1) differential equation as a differential equation with one order and one variable as follows. In the following equation, b is a constant term:
The derivative definition can be stated as follows:
If ∆t = 1, then
In addition, X (1) ≅ Z (1) and
The following expression is obtained using Eqs. (4), (5), (6) and (7):
(d) Use the least square method and differential and difference equations to obtain parameters a and b.
Thus,
(e) Use the grey differential equation to obtain the grey AGO equation:
Next, use the IAGO for reduction to obtain the required forecasting model:
NGBM(1, 1)
The NGBM is an original prediction model derived from GM(1, 1) combined with the Bernoulli equation, a basic differential equation (Li et al. 2010). This model retains the two advantages of GM(1, 1): it has a simple derivation process and requires only four data samples for modeling. In addition, in the NGBM, the prediction error of GM(1, 1) is reduced and the predictive accuracy of GM(1, 1) on nonlinear data types is improved (Feng et al. 2012).
GM(1, 1) is a special case of the NGBM. The procedures involved in deriving the NGBM equation are outlined as follows:
-
(a)
The obtained data are defined as an original series. New series can be obtained using the AGO for calculation. The first three equations for the NGBM are identical to Eqs. (1)–(3) for GM(1, 1).
-
(b)
At this point, the NGBM equations differ from those of GM(1, 1). Use the Bernoulli equation to establish the NGBM differential and difference models.
The NGBM differential equation is as follows:
$$\frac{{{\text{d}}X^{(1)} }}{{{\text{d}}t}} + aX^{(1)} = b\left[ {X^{(1)} } \right]^{r}.$$(14)The following NGBM differential equation can be obtained by substituting Eqs. (18) into Eqs. (4), (5), (6), and (7) of GM(1, 1):
$$X^{\left( 0 \right)} \left( k \right) + aX^{\left( 1 \right)} \left( k \right) = b\left[ {Z^{\left( 1 \right)} (k)} \right]^{r} , \quad k \, = \, 2,3,4, \ldots n.$$(15) -
(c)
Use the least squares method and NGBM differential and difference equations to obtain a and b.
Thus,
$$\theta = \left[ {a,b} \right]^{\text{T}} = (B^{\text{T}} B)^{ - 1} B^{\text{T}} Y,\,Y = B\theta$$(16)$$Y = \left[ {X^{(0)} (2),X^{(0)} (3), \ldots ,\,X^{(0)} (n)} \right]^{\text{T}}$$(17)$$B = \left[ {\begin{array}{*{20}l} { - \frac{1}{2 }\left[ {X^{\left( 1 \right)} \left( 1 \right) + X^{\left( 1 \right)} \left( 2 \right)} \right]} & {\left\{ { - \frac{1}{2 }\left[ {X^{\left( 1 \right)} \left( 1 \right) + X^{\left( 1 \right)} \left( 2 \right)} \right]} \right\}^{r} } \\ { - \frac{1}{2 }\left[ {X^{\left( 1 \right)} \left( 2 \right) + X^{\left( 1 \right)} \left( 3 \right)} \right]} & {\left\{ { - \frac{1}{2 }\left[ {X^{\left( 1 \right)} \left( 1 \right) + X^{\left( 1 \right)} \left( 2 \right)} \right]} \right\}^{r} } \\ \qquad \qquad \; \; \; \vdots & \qquad \qquad \quad \; \vdots \\ { - \frac{1}{2 }\left[ {X^{\left( 1 \right)} \left( {n - 1} \right) + X^{\left( 1 \right)} \left( n \right)} \right]} & {\left\{ { - \frac{1}{2 }\left[ {X^{\left( 1 \right)} \left( {n - 1} \right) + X^{\left( 1 \right)} n} \right]} \right\}^{r} } \\ \end{array} } \right].$$(18) -
(d)
Use the grey differential equation to derive the grey AGO equation:
$$\hat{X}^{\left( 1 \right)} \left( {k + 1} \right) = \left[ {\left[ {X^{(0)} (1)^{(1 - r)} - \frac{u}{a}} \right]^{{e^{{ - a\left( {1 - r} \right)k}} }} + \frac{b}{a}} \right]^{{\left( {\frac{1}{1 - r}} \right)}} ,\,k = 1,2,3, \ldots n.$$(19) -
(e)
Reduce Eq. (19) using the IAGO to obtain the required forecasting model:
$$\hat{X}^{\left( 0 \right)} \left( k \right) = \hat{X}^{\left( 1 \right)} \left( k \right) - \hat{X}^{\left( 1 \right)} \left( {k - 1} \right), \quad k = 1,2,3 \ldots ,n.$$(20)
Predictive accuracy measurement
The estimated difference between the actual values and the predicted values obtained using a prediction model is considered the prediction error (Li et al. 2011). As a judgment method, determining the prediction error indicates the success of a forecasting model. In this study, we adopted the three most indicative measurement models—the mean absolute error (MAE), mean squared error (MSE), and mean absolute percentage error (MAPE)—to measure the accuracy of the forecasting models (Chang et al. 2013).
The various error types are defined as follows:
-
(1)
Lower MAE indicates a more satisfactory predictive ability.
$${\text{MAE}} = \frac{\mathop \sum \nolimits \left| e \right|}{n}$$(21) -
(2)
Lower MSE indicates a more satisfactory predictive ability.
$${\text{MSE}} = \frac{{\mathop \sum \nolimits e^{2} }}{n - 1}$$(22) -
(3)
Lower MAPE indicates a more satisfactory predictive ability.
$${\text{MAPE}} = \frac{{\mathop \sum \nolimits \left| \frac{e}{a} \right|}}{n} \times 100\;\% ,\quad a = {\text{ actual value}}$$(23)
Lower errors indicate higher accuracy. According to Lewis (1982), MAPE values lower than 10 % indicate predictions with high predictive accuracy (Table 1).
Results and discussion
After numerous years of development, the ratio of renewable energy consumption to overall primary energy consumption in China has increased annually. In this study, we collected 22 data samples. In 1991, the percentage of renewable energy consumption within overall energy consumption was 4.8 %; furthermore, it was 7.5 % in 2001, 6.7 % in 2006, 8.6 % in 2010, and 9.2 % in 2012 (Table 2).
GM(1, 1) results
This study established GM(1, 1) using four data samples and employed MATLAB for the calculation. This study then used Eqs. (1)–(13) to derive the predicted values. The predicted value for the percentage of renewable energy consumption from the overall energy consumption in 1995 was 5.3 %. In 2001, 2006, 2010, and 2012, these values were predicted to be 7.1, 7.4, 8.4, and 9.1 %, respectively; Table 3 shows other details. The predicted values for 2013, 2014, and 2015 were 9.4, 9.6, and 10.1 %, respectively.
This study subsequently used Eqs. (21)–(23) in Excel to calculate the predictive accuracy indicators for GM(1, 1). The MAE was 0.394, MSE was 0.244, and MAPE was 5.855 %; Table 4 shows the detailed data.
NGBM(1, 1) results
This study applied NGBM(1, 1) for prediction and used MATLAB for calculation. This study then used Eqs. (14)–(20) to derive the predicted values. The predicted value for the percentage of renewable energy consumption from the overall energy consumption in 1995 was 5.6 %. In 2001, 2006, 2010, and 2012, the predicted values were 7.3, 7.4, 8.5, and 9.1 %, respectively; Table 3 shows other details. The predicted values for 2013, 2014, and 2015 were 9.5, 9.8, and 10.3 %, respectively.
This study subsequently used Eqs. (21)–(23) in Excel to calculate the predictive accuracy indicators for NGBM(1, 1). The MAE was 0.333, MSE was 0.186, and MAPE was 4.893 %; Table 4 shows the detailed data.
Regression analysis results
To compare the predictive accuracy of these methods, this study performed forecasts using a regression analysis model and compared the results with those of the aforementioned models.
This study employed Minitab to establish a regression analysis model and scatter plot using the 1991–2012 data shown in Table 2. Table 3 and Fig. 1 show the detailed data. The R2 and adjusted R2 of this model were both 1.0. The predicted values for 2013, 2014, and 2015 were 8.5, 8.7, and 8.8 %, respectively.
The regression equation can be expressed as follows:
This study then used Eqs. (21)–(23) in Excel to calculate the predictive accuracy indicators for the regression analysis model. The MAE was 0.430, MSE was 0.265, and MAPE was 6.026 %; Table 4 shows the detailed data.
Discussion
This study used the MAE, MSE, and MAPE to compare the predictive accuracy of the three grey prediction models. NGBM(1, 1) exhibited the highest predictive accuracy, followed by GM(1, 1). The results indicated that the revised grey prediction models, NGBM(1, 1), registered more satisfactory forecasts than the GM(1, 1) did.
According to Lewis (1982), a MAPE lower than 10 % indicates a high predictive accuracy. The MAPEs of NGBM(1, 1), GM(1, 1), and regression analysis model were 4.893, 5.855, and 6.026 %, respectively. Therefore, NGNM(1, 1) returned the most accurate forecasts, followed by GM(1, 1) and regression analysis model. The results indicated that the modified NGBM(1, 1) exhibited superior predictive abilities among the compared models.
This study compared the predictive accuracy of the grey prediction models with that of the regression analysis model. According to the MAE, MSE, and MAPE values, the forecasts of the grey prediction models were more satisfactory than those yielded by the regression analysis model (Table 4).
Figure 2 illustrates the comparison of the predicted values from the three models with the actual values. NGBM(1, 1) demonstrated the highest predictive accuracy, followed by GM(1, 1) and finally the regression analysis model.
The obtained results confirmed that grey prediction demonstrates the following advantages: (1) Grey prediction does not require substantial amounts of historical data. Data amounts are selected according to only actual circumstances and requirements. In general, a forecasting model can be established using a minimum of four data samples. (2) Grey prediction methods involve simple calculations. (3) Grey prediction does not require an excessive number of related factors. Data required for grey prediction are obtained easily, and data collection time and costs are considerably lower compared with those required in other methods. (4) Grey prediction is highly accurate.
The results obtained in this study indicated that in the revised grey prediction models, NGBM(1, 1) yielded a higher predictive accuracy than that of the GM(1, 1) when small data samples were used. The predictive capabilities of the grey prediction models were also superior to those of the conventional regression analysis model.
Conclusion
Forecasting involves estimating events or circumstances that organizations cannot control; it is performed to provide a basis for managers to formulate plans. Therefore, forecasting is critical during decision-making processes. In this study, we forecasted growth trends in renewable energy consumption in China. Renewable energy technologies are emergent. We used only 22 data samples in this study. Because historical data about renewable energy were limited in sample size and because the data were not normally distributed, forecasting methods used for analyzing large amounts of data were unsuitable for this study.
According to the empirical results, the study compared the predictive accuracy of the grey prediction models with that of the regression analysis model. The MAE, MSE, and MAPE results indicated that NGBM(1, 1) demonstrated the highest predictive accuracy, followed by GM(1, 1). The regression analysis model demonstrated the lowest predictive accuracy among the models. In addition, the study verified that when small data samples were used, in the modified grey prediction models, NGBM(1, 1) yielded more accurate predictions than the GM(1, 1) did.
Numerous previous industrial studies may have been unable to make accurate predictions because they could not obtain substantial amounts of historical data. This paper suggested that subsequent researchers build on the foundation of this study and select different industries to perform cumulative studies.
References
Akay D, Boran FE, Yilmaz M, Atak M (2013) The evaluation of power plants investment alternatives with grey relational analysis approach for Turkey. Energy Sour Part B: Econ Plan Policy 8(1):35–43
Avinash S, Vipul J, Felix TS (2012) An integrated approach for machine tool selection using fuzzy analytical hierarchy process and grey relational analysis. Inter J Prod Res 50(12):3211–3221
Bardi U, Asmar TE, Lavacchi A (2013) Turning electricity into food: the role of renewable energy in the future of agriculture. J Clean Prod 53(8):224–231
Chang AY (2012) Prioritising the types of manufacturing flexibility in an uncertain environment. Inter J Prod Res 50(8):2133–2149
Chang CJ, Li DC, Dai WL, Chen CC (2013) Utilizing an adaptive grey model for short-term time series forecasting—a case study of wafer-level packaging. Math Prob Eng 2013:1–6
Chen SM, Chen CD (2011) Handling forecasting problems based on high-order fuzzy logical relationships. Exp Syst Appl 38(4):3857–3864
Cucchiella Federica, D’Adamo Idiano (2015) Residential photovoltaic plant: environmental and economical implications from renewable support policies. Clean Technol Env Policy. doi:10.1007/s10098-015-0913-1
Deborah P, Giuseppe G, Enrico B, Daniele R (2013) Production of green energy from co-digestion: perspectives for the Province of Cuneo, energetic balance and environmental sustainability. Clean Technol Env Policy 15(6):1055–1062
Deng JL (1982) Control problems of grey system. Syst Cont Lett 1(5):288–294
Deng JL (2003) Literalizing GRA axioms. J Grey Syst 4:399–400
Deng JL (2004) Grey management: grey situation decision making in management science. J Grey Sys 2:93–96
Farah H, Armin D, Ali K (2013) Production of biodiesel as a renewable energy source from castor oil. Clean Technol Env Policy 15(6):1063–1068
Farhad M, Hossain M Hasanuzzaman, Rahim NA, Ping HW (2015) Impact of renewable energy on rural electrification in Malaysia: a review. Clean Technol Env Policy 17(4):859–871
Feng SJ, Ma YD, Song ZL, Ying J (2012) Forecasting the energy consumption of China by the grey prediction model. Energy Sou Part B: Econ Plan Policy 7(4):376–389
Herbig P, Milewicz J, Golden JE (1993) Forecast: who, what, when, and how. J Bus Forecast 15(5):395–412
Hu PY (2004) Using a grey multipurpose decision system for car purchasing. J Grey Sys 7(1):11–14
Igliński B, Piechota G, Iglińska A, Cichosz M, Buczkowski R (2015) The study on the SWOT analysis of renewable energy sector on the example of the Pomorskie Voivodeship (Poland). Clean Technol Env Policy. doi:10.1007/s10098-015-0989-7
Kayacan E, Ulutas B, Kaynak O (2010) Grey system theory-based models in time series prediction. Exp Syst Appl 37(2):1784–1789
Khoie Rahim, Yee Victoria E (2015) A forecast model for deep penetration of renewables in the Southwest, South Central, and Southeast regions of the United States. Clean Technol Env Policy 17(4):957–971
Kwonpongsagoon S, Bader HP, Scheidegger R (2007) Modelling cadmium flows in Australia on the basis of a substance flow analysis. Clean Technol Env Policy 9(4):313–323
Lee YC, Wu CH, Tsai SB (2014) Grey system theory and fuzzy time series forecasting for the growth of green electronic materials. Inter J Prod Res 52(10):2931–2945
Lewis CD (1982) Industrial and business forecasting methods. Butterworths, London
Li DC, Yeh CW, Chang CJ (2009) An improved grey-based approach for early manufacturing data forecasting. Comp Indus Eng 57(4):1161–1167
Li GD, Masuda S, Wang CH, Nagai M (2010) The hybrid grey-based model for cumulative curve prediction in manufacturing system. Int J Adv Manuf Technol 47(4):337–349
Li DC, Chang CJ, Chen WC, Chen CC (2011) An extended grey forecasting model for omnidirectional forecasting considering data gap difference. Appl Math Mod 35(10):5051–5058
McLellan BC, Corger GD, Giurco DP, Ishihara KN (2012) Renewable energy in the minerals industry: a review of global potential. J Clean Prod 32(9):32–44
National Bureau of Statistics of the People’s Republic of China (2013) China energy statistical year book. China Statistics Press, Beijing 2013
Prakash K, Urmila D, Heriberto C (2013) Model-based approach to study the impact of biofuels on the sustainability of an ecological system. Clean Technol Env Policy 15(1):21–33
Sadeghi M, Hajiagfa SH, Hashemi SS (2013) A fuzzy grey goal programming approach for aggregate production planning. Int J Adv Manuf Technol 64(9–12):1715–1727
Wang CH (2010) Predicting tourism demand using fuzzy time series and hybrid grey theory. Tour Manag 25(3):367–374
Xiong W, Liu L, Xiong M (2010) Application of gray correlation analysis for cleaner production. Clean Technol Env Policy 12(4):401–405
Zhang Q, Zhou D, Fang X (2014) Analysis on the policies of biomass power generation in China. Renew Sust Energy Rev 32:926–935
Zhao X, Burnett JW, Fletcher JJ (2014) Spatial analysis of China province-level CO2 emission intensity. Renew Sust Energy Rev 33(May):1–10
Author information
Authors and Affiliations
Corresponding author
Rights and permissions
About this article
Cite this article
Tsai, SB. Using grey models for forecasting China’s growth trends in renewable energy consumption. Clean Techn Environ Policy 18, 563–571 (2016). https://doi.org/10.1007/s10098-015-1017-7
Received:
Accepted:
Published:
Issue Date:
DOI: https://doi.org/10.1007/s10098-015-1017-7