Abstract
If the pattern of appliance use varies between households, then we expect that appliance replacement cycle also varies between households. Although many studies identified early adopters of new energy-efficient products, few studies have examined households that have been using old energy-inefficient products and how their characteristics are associated with product replacement. In this study, we initially modify a survival function and develop an economic model to evaluate the impact of household characteristics on appliance replacement. We subsequently apply the model in microlevel data analysis from the Survey on Carbon Dioxide Emission from Households obtained from the Ministry of the Environment of Japan. We use information about age distribution of refrigerators (REFs) and examine how family size, household income, and age of household’s head affect the replacement cycle of REFs. Our empirical results reveal that (1) large-sized, (2) high-income, and (3) young households replace REFs more rapidly. These findings suggest that policies that encourage small-sized, elderly, and low-income households to replace old appliances are needed.
Similar content being viewed by others
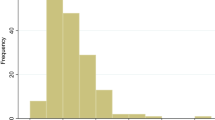
Avoid common mistakes on your manuscript.
1 Introduction
Energy efficiency of home electric appliances has been improving annually. Particularly, households can lower their energy consumption by replacing old appliances with new energy-efficient models. In line with this, governments have been promoting appliance replacement for many years to reduce residential energy consumption. For example, they have introduced labeling programs that enable households to make product choices more easily based on the energy-efficiency information of appliances. For instance, the US Environmental Protection Agency (EPA) first introduced the Energy Star program in 1992 to help households easily recognize energy-efficient products through Energy Star labels (USEPA 2020). Following the implementation of Energy Star labels in the US, the Japanese government introduced the Energy Saving Labeling program in 1995, which enables consumers to examine the energy efficiency of products through green/orange marks. To further enhance the program’s function, the Japanese government introduced the Unified Energy Saving Labeling Program in 2006. Under this new labeling program, retailers need to indicate products’ energy efficiency by the number of stars and report the estimated annual electricity bill. Therefore, consumers require less cognitive skills to identify the energy efficiency of products than before (Wang and Matsumoto 2020).
In recent years, some governments provided households with subsidies and a stronger appliance replacement incentive. However, the effects of such subsidy policies raised concerns. For example, the US Department of Energy developed the State Energy-Efficient Appliance Rebate Program to support residential consumers in purchasing energy-efficient appliances (Office of Energy Efficiency and Renewable Energy 2015). Although this program spent approximately $300 million between 2009 and 2015, Houde and Aldy (2017) found that consumers upgraded their old appliances with higher quality but less energy-efficient models. Similarly, the Japanese government implemented a rebate program, the so-called eco-point program (EPP), between 2009 and 2011. The EPP provided subsidies to households who purchased refrigerators (REFs), air conditioners (ACs), and digital televisions (TVs) that are labeled with more than four stars under the Unified Energy Saving Labeling Program (Ministry of the Environment 2009). As the cessation of analog broadcasting was scheduled in 2011, the government promoted the replacement of old analog TVs with new digital TVs through the EPP. However, Yamaguchi et al. (2016) reported that the EPP expanded the market share of energy-efficient TV models but incentivized consumers to purchase TVs with large screen size. Their finding suggests that households will not replace an old appliance with a new model only for energy-efficiency purpose.
In addition, previous studies about eco-subsidy programs reported that the effect of such programs is limited, that is, only certain types of households replaced old appliances with new energy-efficient models (Wang and Matsumoto 2019; Nakano and Washizu 2017). Wang and Matsumoto (2019) examined the impact of subsidy programs on the selection of hybrid electric vehicle (HEV). They applied the multinomial model to identify the types of households that switched from conventional gasoline vehicles to HEVs. The estimated results demonstrate that certain types of households, for example, high-income households, small-sized households, switched to HEVs during the subsidy programs. In other words, their findings imply that other households continue to use their old appliances, regardless of the substantial amount of subsidy available.
One of the reasons why households continue to use old appliances can be considered as the existence of status quo bias (Blasch and Daminato 2018; Schleich et al 2016). Blasch and Daminato (2018) examined how status quo bias affects the replacement of old appliances; they found that the degree of status quo bias is intimately associated with household socioeconomic characteristics, such as age, income, and household type.
If the pattern of appliance use varies between households, then we expect that the appliance replacement cycle also varies between households. Large-sized households replace washing machines more rapidly because they frequently wash large bulk of clothes at once. In contrast, young people replace their smartphones more frequently as they intend to do more tasks with it. Although certain households may replace appliances for energy conservation, other households may pay less attention to appliances’ energy efficiency and continue to use their old appliances until totally depreciated.
Many studies identified early adopters of new energy-efficient products (Campbell et al. 2012; van Rijnsoever et al. 2009), as well as the role of psychological biases on replacement of appliances (Schleich et al. 2019). However, to the best of our knowledge, only Fernandez (2000, 2001) has identified the relationship between households’ socioeconomic characteristics and product replacement from the aspect of appliance’s survival probabilities. Given that certain households do not replace appliances despite the evident benefits, the relationship between households’ socioeconomic characteristics and appliance replacement cycle needs to be determined for residential energy conservation. In this study, we first develop an economic model to describe the survival probabilities of appliance and evaluate the impact of households’ socioeconomic characteristics on home appliance replacement.
Fernandez (2000, 2001) studied Americans’ replacement habits of electric space heaters and central air conditioners for the empirical analyses. In contrast, we targeted REFs in this study. We believe REFs are much more suitable for the study of appliance replacement than heaters and ACs. Although the usage of heaters and ACs vary considerably between households, it is often difficult to know it.Footnote 1 In the empirical section, we use microlevel data from the 2016 Survey on Carbon Dioxide Emission from Households (Ministry of the Environment 2016). Survey respondents in SCDEH 2016 were asked about the age of their REFs and their socioeconomic characteristics. Based on the information about the age distribution of REFs, we examine how family size, age of household’s head, and household income affect the replacement cycle of REFs. Our empirical results reveal that (1) large-sized, (2) high-income, and (3) young households replace REFs more rapidly.
The remaining of this paper is structured as follows. In the next section, we propose an economic model of home appliance replacement. We assume a maximum product useful life and derive the age distribution of appliance in the steady state. We show how households’ socioeconomic characteristics are associated with appliances’ survival probability and age distribution. Then we apply the model to microlevel data analysis based on SCDEH 2016. In Sects. 3 and 4, we summarize the data and specify the empirical model, respectively. In Sect. 5, we report the empirical findings. Lastly, in Sect. 6, we provide policy recommendations and the conclusion.
2 Economic model of product replacement cycle
For illustrative purposes, we initially present a simple model without utilizing households’ socioeconomic characteristics. We assume that each household owns only one appliance with a maximum useful life of 2 years. Half of the products sold during a market break in the first year whereas the other half operates until the second year. We assume that households continue to use a product until totally depreciated. Therefore, half of the households need to replace products at the end of the first year, whereas the remaining half continues to use the products until the end of the second year. The above-mentioned condition can be characterized by the survival function as follows:
where \(t\) is the product age. This survival function is illustrated in Fig. 1a.
Estimating the survival function directly is often difficult because participants in the survey may not recall their products’ year of final use. Nevertheless, their products’ age and manufacturing year were commonly asked. Therefore, researchers can generally use information about the number of households that use products with a certain useful life.
In the above-mentioned example, researchers observe the share of households using a brand-new product \(p\left(0\le t<1\right)\) and those that use products in their second-year of useful life \(p\left(1\le t<2\right)\). In the steady state, we require the condition of \(p\left(0\le t<1\right)+p\left(1\le t<2\right)=1\). Furthermore, if the total number of households that own a product does not change over time, then \(\frac{1}{2}p\left(0\le t<1\right)=p\left(1\le t<2\right)\) is required. By solving the system of equations, we obtain \(p\left(0\le t<1\right)=\frac{2}{3}\) and \(p\left(1\le t<2\right)=\frac{1}{3}\) as the steady-state distribution of product age as shown in Fig. 1b.
If a product can be used for up to T years, then the survival function can be described similar to Fig. 2a. Product usage varies between households; thus, we expect that the shapes of survival functions also vary between households. The solid curve indicates the survival function of type \(k\) households, who maintain a product in good shape that gradually depreciates. On the contrary, the dotted curve indicates the survival function of type \(l\) households, who do not use a product properly and depreciate it rapidly. Among type \(k\) households, the share of households that use brand-new products is
whereas the share of households that use a \(t\)-year-old product is
Figure 2b shows that the share of households that use a \(t\)-year-old product among type \(k\) households is presented by the solid curve whereas those among type \(l\) households are presented by the dotted line. We define the share of households that use the product whose age between \(a\) and \(b\) as follows:
The strength of the above-mentioned approach is the inclusion of product use heterogeneity between households. For example, although large-sized households use products frequently and thus replace them early, small households use products less frequently and thus will not replace products for long periods. Parameter \(\lambda \) in our model reflects this difference in product use between households.
3 Data
3.1 Appliance selection
In this study, we use microlevel data from the SCDEH 2016. As a general government statistical survey of households nationwide, the SCDEH 2016 is conducted by the Ministry of the Environment of Japan and uses in-person and Internet surveys (Ministry of the Environment 2019). The survey includes samples of 16,402 households (8802 households from in-person survey and 7600 households from the Internet survey) in Japan. The survey contains information about household energy usage, socioeconomic characteristics of subjects, dwelling conditions, and ownership, age, and use of appliances; information about household energy usage was collected every month during the sampling period (between October 2014 and September 2015), whereas the other information was obtained in August 2015.
Although households were asked about the age of ACs, REFs, and TVs in the survey, we will focus on REFs in this analysis due to the following four reasons. First, many households own multiple TVs and ACs; SCDEH 2016 shows that a Japanese household owns 1.24 REFs, 1.96 TVs, and 2.32 ACs on average. Although households were asked to specify their main TV and AC, the pattern of appliance use becomes complex when multiple appliances are used. Second, the ownership and use of ACs vary greatly between regions and among households with different socioeconomic characteristics. Some households living in northern Japan do not have AC. Households in Hokkaido, the northernmost region of Japan, only own 0.36 AC on average, which is much lower than the national average of AC ownership. In addition, the survey reports that wealthy households use ACs more frequently. Approximately 72.37% of households whose annual income exceeded JPY 10 million use ACs for more than four hours a day in August whereas only 63.14% of households whose annual income is below JPY 2.5 million use ACs at that intensity. Third, majority of ACs sold in Japan have space-heating function. Thus, the same machine can be used for space cooling and heating. Nevertheless, households may choose other options, such as kerosene stoves or city gas for space heating. Although households living in relatively warm areas use ACs for space heating, those living in cold areas use primarily kerosene for space heating. According to SCDEH 2016, approximately 26.0% of households use ACs as a main space-heating tool at the national level whereas only 0.79% of households in Hokkaido use it as the main tool. In contrast, approximately 55.59% of households in Hokkaido use kerosene stoves as the main space-heating tool. Similarly, wealthy households use ACs and city gas for space heating, but less wealthy households use kerosene. Therefore, we need to consider households’ energy choice when discussing ACs’ replacement cycle, thus making the analysis more complex.
Finally, with regard to TVs, analog broadcasting was replaced with digital broadcasting on July 24, 2011. During the transition period, most households replaced their CRT TVs with liquid–crystal-display TVs. We consider TVs as not an appropriate product for the present analysis due to their major technological advancement and the impact of a huge policy intervention on TVs for the last two decades.
Figure 3 shows the distribution of production year (age) of the main REFs reported in SCDEH 2016.Footnote 2 Approximately 62.1% of households use REFs manufactured in the last 10 years. However, some households use extremely old REFs. For instance, 2.7% of households stated that they continued to use REFs manufactured more than 25 years ago.
As mentioned in Sect. 2, we assume that households purchase an appliance with a maximum product useful life of \(T\) years and continue to use until it totally depreciated. Therefore, households that purchased a used REF have to be removed from the dataset. Since it is not known whether households purchased a used or new REF in the SCDEH 2016, we remove the households that are likely to own a used REF in the following steps.
We take account of the age of households’ head when setting the maximum product useful life of REFs.Footnote 3 We assume that the maximum product useful life is censored according to the age of household’s head. Specifically, we set the censored value to 10 years for households whose head’s age is between 20 and 29, 20 years for those between 30 and 39 years, and 30 years for those between 40 and 49, respectively. If the reported age of REF is beyond the corresponding censored value, we assume that a household uses a used REF and removed it from the dataset. As a result, the sample size was reduced to 10,013.
3.2 Descriptive statistics
In this study, we consider three types of household socioeconomic characteristics: (1) family size, (2) household annual income, and (3) age of household’s head, which are closely associated with household appliance replacement as well as household investment in energy-efficient appliances (Blasch and Daminato 2018; Wang et al 2019; Belaida and Garcia 2016; Ward et al 2011; Murray and Mills 2011). Table 1 presents the descriptive statistics of these variables.
Although the data show 10,043 households, some of these households did not answer the questions appropriately. As a result, the available data collected for analyses for family size, household annual income, and age of household’s head are 10,013, 9113, and 10,013, respectively.
For the family size analysis, we choose single-person household as the base. We examine whether replacement cycle rate increases or decreases along with the increase in household members. Considering that large-sized households use larger REFs and stock more food compared with small-sized households, they are expected to replace their REFs more rapidly.
In the household income analysis, lowest income households are selected as the base. We examine whether the replacement cycle becomes faster or slower as household income increases. Previous studies find that wealth constraint is an issue among low-income households’ adoption of new technologies (Li and Just 2018). Thus, we expect that the appliance replacement cycle becomes faster as household income increases.
Finally, in the household head’s age analysis, household heads between 20 and 29 years old were selected as the base. We examine whether appliance replacement cycle becomes faster or slower as the household head’s age increases. Given that older households are more likely to use products for a long time, we expect that the replacement cycle of REFs will be slower as the age of household’s head increases.
3.3 Kolmogorov–Smirnov test
Before conducting the model analysis, we conducted a two-sample Kolmogorov–Smirnov test (K–S test) to investigate whether REFs’ replacement cycle varies among different types of households. The K–S test is a non-parametric test that examines the equality of distributions between two samples. After classifying households based on family size, household income, and age of household’s head, we conducted the K–S test to examine whether the age distributions of REFs differ between groups.
We also conducted the K–S test among three family-size groups: single-person households, medium-sized households composed of 2–3 persons, and large-sized households composed of more than 4 persons.
With regard to household income, we conducted the K–S test between three income groups: low-, middle-, and high-income households, with annual income below JPY 2.5 million, between JPY 2.5 million and JPY 10 million, and over JPY 10 million, respectively.
Finally, with regard to age of household’s head, we conducted the K–S test between four generation groups: young, middle-aged, mature-aged, and senior households, with ages below 30, 30–49, 50–64, and over 65 years, respectively.
Table 2 shows the results of the K–S test on whether REFs’ age distributions differ between households with different socioeconomic characteristics. First, based on family size, single-person and medium-sized households statistically significantly differ at 10% level. On the contrary, single-person and large-sized households statistically significantly differ at 1% level. This finding implies that REFs’ age distribution of single-person families differs from those of the other two types of households. Similarly, medium- and large-sized households statistically significantly differ at 1% level. Therefore, age distributions of REFs differ between medium- and large-sized households.
Low- and middle-income households and low- and high-income households both statistically significantly differ at 1% level. However, we did not obtain a statistically significant result between middle- and high-income households. These results suggest that low-income households’ age distribution of REFs differs from those of the remaining income groups.
Finally, with regard to age of household’s head, K–S test results suggest that the age distributions of REFs differ in all comparison groups, excluding the comparison between mature-aged and senior households. These results suggest that REFs’ replacement cycle differs among age groups.
Non-parametric K–S test result clearly revealed that household socioeconomic characteristics and REFs’ replacement cycle are significantly related. In the next section, we propose a parametric model that shows how socioeconomic characteristics influence the replacement cycle of REFs.
4 Empirical model
This study uses available information on the number of households that use products that have been previously used for certain years. Let \({p}_{v}\left({\lambda }_{ki}\right)\) denote the probability that household \(i=1,\cdots ,n\) characterized by socioeconomic characterizes \(k=1,\cdots , K\) uses the product in the vintage group \(v=1,\cdots ,m\). Here, \({\lambda }_{ki}\) is the variable that characterizes the shape of the survival function, and we assume that \({\lambda }_{ki}=\alpha +{\beta x}_{ki}\), where \({x}_{ki}\) is the \(k\) th socioeconomic characteristics of household \(i\), \(\alpha \) and \(\beta \) are the associated parameters. The likelihood estimation is given by
where \(I\left({v}_{i}\right)\) is the index function taking a value of 1 when household \(i\) is in the product-age group \(v\), and zero otherwise. Using the definition in Sect. 2 and taking log of it, we obtain the log-likelihood function as follows:
where \({T}_{m}\) is the maximum product useful life that reflects the age of household’s head, \(a\) and \(b\) are lower and upper bounds of the product age of the group \(v\), respectively.
For empirical estimation, we consider a simple survival function as follows:
where \({T}_{p}\) is the maximum product useful life solely determined by product physical characteristics.
For this survival function, the share of households using a brand-new product becomes
The share that household \(i\) uses the product in the vintage group \(v\) is
We insert Eq. (4) into the likelihood function of Eq. (1) to find optimal parameters \(\widehat{\alpha }\) and \(\widehat{\beta }\). The lager \(\beta \) means that the survival rate of the product is low; thus, the replacement cycle is faster.
We conduct the maximum likelihood estimation for family size, household annual income, and age of household’s head. Previous studies argue that energy-efficiency investment has a turning point; that is, an individual accelerates energy-efficiency investment until s/he reaches to a threshold age and starts to slow it down thereafter. Based on the findings of previous research, we consider the quadratic function \({\lambda }_{ki}=\alpha +{{\beta }_{1}x}_{ki}+{\beta }_{2}{x}_{ki}^{2}\) for the estimation analysis of household’s head age. Finally, we set \({T}_{p}\) = 36.
5 Empirical result
5.1 Impact of household characteristics on appliance replacement
Table 3 presents the estimation results of Eq. (1). Models 1, 2 and 3 represent the estimation results for family size, household annual income, and age of household’s head, respectively.Footnote 4 The parameters \(\alpha \) and \(\beta \) are statistically significant at the 1% level in Model 1 and Model 2. The results suggest that family size and household income affect the replacement cycle of REFs. The estimated parameter \(\beta \) is positive in Models 1 and 2. This result implies that an increase in family size and household income decreases the survival probability of REFs; large families and high-income households replace REFs more rapidly.
The replacement cycle of REFs may be associated with life stage, for example, it is common that households would replace a REF at the time of marriage or having children. Furthermore, previous studies reported that there is an inverse u-shaped relationship between age and energy efficiency investment (Wang et al. 2019; Belaida and Garcia 2016). To evaluate life stage impacts on the replacement cycle, we include the quadratic terms in the estimation of age of households’ head.
We compare the effect of the age of households’ head across different family structures: all households (Model 3–1), multi-person households (Model 3–2), single households (Model 3–3), and couple-only households (Model 3–4).
The estimated \(\beta \mathrm{s}\) are statistically significant in Model 3–2, while they are not statistically significant in Model 3–1 and Model 3–3. This result suggests that age of households’ head does affect the replacement cycle of REFs for multi-person households but not for single-person households. In Model 3–2, \({\beta }_{1}\) is negative. This result implies that an increase in the age of households’ head of multi-person households increases the survival probabilities of REFs.
We focused on couple-only households and examined the effect of age of households’ head on the replacement cycle of REFs in Model 3–4. The estimated parameters signs are the same as in Model 3–2. However, the size of \({\beta }_{1}\) in Model 3–4 is smaller than that in Model 3–2. These results suggest that the survival rate of REFs for couple-only households is higher than multi-person households, namely couple-only households replace REFs more slowly than multi-person households.
On the other hand, the estimated \({\beta }_{2}\mathrm{s}\) are positive in both Model 3–2 and Model 3–4, whereas the size of \({\beta }_{2}\) in Model 3–4 is larger than that in Model 3–2. These results suggest that although the increase in the age of households’ head decreases the replacement cycle of REFs, such tendency would inverse when the households’ head reaches to a threshold age. Specifically, the calculated threshold age is 79 years for multi-person households, while that for couple-only households is 69 years; couple-only households accelerate the replacement cycle of REFs at a relatively earlier stage than multi-person households. It cannot be clearly said from the present data analysis why such a U-shaped relationship is observed. One possible explanation is the change in risk aversion. People become more concerned about risk factors as they get older. Senior people will concern about the possibility of the breakage of an old refrigerator more seriously and thus may replace it earlier.
5.2 Estimated survival probabilities for appliances
By substituting the estimated \(\widehat{\alpha }\) and \(\widehat{\beta }\) from the three models into Eq. (3), we obtain REFs’ survival function for family size, household annual income, and age of household’s head.
We calculate the survival probabilities of REFs. Figure 4 shows a comparison of the survival functions of single-person households with those of medium- and large-sized households. The figure shows that the survival function for single-person households is flatter than that for medium- and large-sized households. The survival probabilities after 1 year are similar between the three household sizes: 96.34% (single-person), 95.69% (medium-sized), and 93.98% (large-sized). However, the difference in the survival probability among the three types of households widens as the year of use increases. This tendency is particularly clear between large-sized and the other two types of households. The survival probabilities for single-person households are 82.03%, 64.99%, and 48.98% in the 5th, 10th, and 15th year, respectively. On the contrary, the survival probabilities for medium-sized households are 79.15%, 60.11%, and 43.04%, respectively. Lastly, the survival probabilities for large-sized households are 71.93%, 48.81%, and 30.49%, respectively. Therefore, large-sized households replace REFs more rapidly than small-sized households.
With regard to household income, we compare the survival probabilities of REFs among three income groups: low-, middle-, and high-income groups. Figure 5 illustrates the survival functions of these three groups. The figure shows that low-income group’s survival function is the flattest whereas that for high-income group is the steepest. According to our estimation, the survival probabilities in the third year are 81.09%, 86.55%, and 89.10% among the high-, middle-, and low-income groups, respectively. The survival probabilities in the 10th year are 45.67%, 58.27% and 64.93%, respectively. Those in the 15th year are 27.30%, 40.88%, and 48.91%, respectively. These results suggest that high-income households replace REFs rapidly, unlike low-income households who continue to use old REFs.
Finally, as shown in Fig. 6a and b, we compared the survival probabilities of REFs for multi-person households and couple households between three generation groups: young, middle-aged, and senior households.Footnote 5 The figures show that the survival function of the senior household is the flattest, whereas that of the young household is the steepest both among multi-person households and couple households. The results suggest that young households tend to replace REFs more rapidly than senior households. The three generation groups do not significantly differ in the initial year. That is, the survival probabilities for multi-person households among young, middle-aged, and senior households are 93.10%, 94.42%, and 95.83%, respectively, whereas those for couple households are 91.01%, 93.85%, and 96.08%, respectively. However, the difference between the three generation groups increases as the year of use increases. The survival probabilities for multi-person households among young households are 68.43%, 43.80%, and 25.48% in the 5th, 10th, and 15th year, respectively, whereas those for couple households are 60.67%, 33.70%, and 16.51%, respectively. However, those for multi-person households among senior households are 79.77%, 61.14%, and 44.27% in the 5th, 10th, and 15th year, respectively, whereas those for couple households are 80.85%, 62.97%, 46.49%, respectively. Therefore, young households are likely to replace REFs earlier compared with senior households who are likely to continue to use old REFs.
5.3 Model fit
To evaluate the model’s accuracy, we compare the age distribution of the main REF in SCDEH 2016 (observed distribution) with the one predicted from our estimation result (predicted distribution). As shown in the results in Table 4, the smaller the difference between the observed and predicted probabilities, the more accurate the model becomes.
The model predicts well the age distributions of REFs purchased before 2006. For example, the predicted shares of REFs purchased between 1996 and 2000 for single-person, medium-, and large-sized households are 12.44%, 11.56%, and 9.16%, respectively. These values are almost equal to those of the observed shares at 12.15%, 12.37%, and 9.72% (see Table 4). On the contrary, the model underpredicts the share of new REFs (REFs purchased after 2011) but overpredicts the share of relatively new REFs (REFs purchased between 2006 and 2010).
6 Conclusion
Despite the promotion of new energy-efficient appliances by governments, certain households continue to use old energy-inefficient home appliances. Households continue to use old home appliances need to be identified to promote energy-efficient home appliances. In this study, we developed an economic model that shows how household socioeconomic characteristics determine appliance replacement cycle. We applied the replacement model for the analysis of the microlevel data from SCDEH 2016.
Empirical findings from this study can be summarized as follows. First, we conducted a non-parametric K–S analysis to examine whether the replacement cycle of REFs depends on family size, household income, and age of household’s head. Second, we conducted the parametric analysis based on the proposed model. In both cases, we obtained fairly consistent results, that is, REFs’ replacement cycle differs between households with varied socioeconomic characteristics. Third, parametric analysis results revealed that the speed of REF replacement becomes faster as the size and income level of households increase but becomes slower as the age of household head increases.
Empirical evidence strongly indicates the need for governments to consider the effects of household socioeconomic characteristics on households’ appliance replacement behavior when promoting the energy-efficient appliances.
Many governments have implemented policies that improve the energy efficiency of home appliances for the past several decades. Thus, the energy efficiency of home appliances has improved drastically. For instance, the implantation of Japan’s Top Runner Programme in 1998 has improved the increase in energy efficiency of REFs by 43% on average from 2005 to 2010 (Wang and Matsumoto 2020). However, not all households benefit from the energy-efficiency improvement. Given the fact that small-sized, senior, and low-income households are likely to use old energy-inefficient home appliances, promotion programs that target these households are expected to be effective for residential energy saving.
The Japanese government introduced the Unified Energy Saving Labeling Program to enable households recognize products’ energy efficiency through the product energy efficiency indicated by the products’ number of stars and estimated annual electricity bill. However, the program can influence household behavior only when households replace appliances. The program will not influence the behavior of households who continue to use old appliances because they will not recognize the energy losses that they incur from the use of old inefficient appliances. Households can reduce their electricity bill from using REFs by approximately 63% if they replace REFs manufactured in 2007 with REFs manufactured in 2016 (Agency for Natural Resources and Energy 2017). However, households cannot recognize such information under the present program. Therefore, additional programs need to be implemented that would enable households to recognize energy saving benefit.
Although we presented a general algorithm to evaluate household socioeconomic characteristics on product replacement, we applied a simple model for empirical analysis. This approach would result in slight underestimation of new products’ survival probabilities. Thus, development of more sophisticated empirical models is recommended for future research.
Notes
Detailed reasons regarding the appliance selection are discussed in Sect. 3.1.
In SCDEH 2016, REF with the largest internal volume is defined as the main REF owned by the household.
Only 10,043 out of 11,632 households answered the age of households’ head. We focus on these 10,043 households henceforth in the remaining analyses.
We further estimated these models for households with only one REF. The estimation results are similar to the results presented in Table 3. This fact implies that the ownership of REFs does not affect the impact of family size/household income/age of household’s head on the replacement cycle of the main REF.
Estimated parameters in Model 3–2 and Model 3–4 are used for the calculation, respectively.
References
Agency for Natural Resources and Energy (2017) Energy efficiency catalog. https://www.enecho.meti.go.jp/category/saving_and_new/saving/general/more/pdf/winter2017.pdf. Accessed 2 Apr 2020
Belaida F, Garcia T (2016) Understanding the spectrum of residential energy-saving behaviours: French evidence using disaggregated data. Energy Econ 57:204–214
Blasch J, Daminato C (2018) Behavioral anomalies and energy-related individual choices: the role of status-quo bias. https://doi.org/10.2139/ssrn.3289624. https://ssrn.com/abstract=3289624
Campbell AR, Ryley T, Thring R (2012) Identifying the early adopters of alternative fuel vehicles: a case study of Birmingham, United Kingdom. Transp Res Part A 46(8):1318–1327
Fernandez VP (2000) Decisions to replace consumer durables goods: an econometric application of winter and renewal processes. Rev Econ Stat 82(3):452–461
Fernandez VP (2001) Observable and unobservable determinants of replacement of home appliances. Energy Econ 23:305–323
Houde S, Aldy JE (2017) Consumers’ response to state energy efficient appliance rebate programs. Am Econ J Econ Policy 9(4):227–255. https://doi.org/10.1257/pol.20140383
Li J, Just RE (2018) Modeling household energy consumption and adoption of energy efficient technology. Energy Econ 72:404–415
Ministry of the Environment (2009) Green home appliances eco-point program. <https://www.env.go.jp/policy/ep_kaden/about/index.html. Accessed 27 Feb 2020
Ministry of the Environment (2016) Survey on carbon dioxide emission from households
Ministry of the Environment (2019) The survey on carbon dioxide emission from households. http://www.env.go.jp/earth/ondanka/ghg/kateiCO2tokei.html. Accessed 10 Oct 2020 (in Japanese)
Murray AG, Mills BF (2011) Read the label! Energy star appliance label awareness and uptake among U.S. consumers. Energy Econ 33:1103–1110
Nakano S, Washizu A (2017) Changes in consumer behavior as a result of the home appliance eco-point system: an analysis based on micro data from the Family Income and Expenditure Survey. Environ Econ Policy Stud 19(3):459–482
Office of Energy Efficiency and Renewable Energy (2015) State energy-efficient appliance rebate program: volume 2 – program results. https://www.energy.gov/eere/buildings/downloads/state-energy-efficient-appliance-rebate-program-volume-2-program-results. Accessed 21 Feb 2020
Schleich J, Gassmann X, Faure C, Meissner T (2016) Making the implicit explicit: a look inside the implicit discount rate. Energy Policy 97:321–331
Schleich J, Gassmann X, Meissner T, Faure C (2019) A large-scale test of the effects of time discounting, risk aversion, loss aversion, and present bias on household adoption of energy-efficient technologies. Energy Econ 80:377–393
United States Environmental Protection Agency (2020) About ENERGY STAR. https://www.energystar.gov/about. Accessed 23 Apr 2020
van Rijnsoever FJ, Rogier A, Donders T (2009) The effect of innovativeness on different levels of technology adoption. J Am Soc Inf Sci Technol 60(5):984–996. https://doi.org/10.1002/asi.21029
Wang J, Matsumoto S (2019) Can subsidy programs change the customer base of next generation vehicles?. RIEEM Discussion Paper Series, No. 1904
Wang J, Matsumoto S (2020) Climate policy in household sector. In: Arimura TH, Matsumoto S (eds) Carbon pricing in Japan, 3rd edn. Springer Nature, Berlin, pp 45–60
Wang J, Sugino M, Matsumoto S (2019) Determinants of household energy efficiency investment: analysis of refrigerator purchasing behavior. Int J Econ Policy Stud 13(2):389–402
Ward DO, Clark CD, Jensen KL, Yen ST, Russell CS (2011) Factors influencing willingness-to-pay for the ENERGY STAR label. Energy Policy 39(3):1450–1458
Yamaguchi K, Matsumoto S, Tasaki T (2016) Effect of an eco-point program on consumer digital TV selection. In: Matsumoto S (eds) Environmental subsidies to consumers: How did they work in the Japanese market?. Routledge, London and New York, pp 76–90
Acknowledgements
This research was supported by the Environment Research and Technology Development Fund (2-1707) of the Environmental Restoration and Conservation Agency and KAKENHI (18K01578) of the Japan Society for the Promotion of Science. This research was also supported by Aoyama Gakuin University Research Institute “Early Eagle” grant program for promotion of research by early career researchers. An earlier version of this paper was presented at the annual conference of Society of Environmental Economics and Policy Studies; we received valuable comments from Professor Isamu Matsukawa. We would also like to thank Fumihiro Goto for his accurate and helpful advices.
Author information
Authors and Affiliations
Corresponding author
Additional information
Publisher's Note
Springer Nature remains neutral with regard to jurisdictional claims in published maps and institutional affiliations.
About this article
Cite this article
Wang, J., Matsumoto, S. An economic model of home appliance replacement: application to refrigerator replacement among Japanese households. Environ Econ Policy Stud 24, 29–48 (2022). https://doi.org/10.1007/s10018-020-00295-2
Received:
Accepted:
Published:
Issue Date:
DOI: https://doi.org/10.1007/s10018-020-00295-2