Abstract
Accurate estimation of reference evapotranspiration (ETo) is a challenging task in windy regions with sparse data recording. This study aimed to assess the accuracy of daily and monthly ETo estimated by Penman–Monteith FAO-56 (PM) fed with ERA5-Land reanalysis (PMERA5-Land), temperature-based PM using the default 2 m wind speed (u2) of 2 m s−1 (PMT2), local u2 (PMTua), seasonal u2 (PMTus) and monthly u2 average (PMTum), Hargreaves-Samani (HS), and recalibrated PMT (RPMT) and HS (RHS) against PM in 31 water-limited windy sites. The most accurate ETo estimates were produced by RPMT and RHS for the majority of cases. The HS, PMT2, and PMTua failed to provide reliable ETo estimates (i.e., normalized root mean square error (nRMSE) of < 30%) in most locations on daily step. The HS, PMT2, and PMTua performed weak in the regions with a large u2 variation. The PMERA5-Land-, PMTua-, and PMTum-estimated ETo had a nRMSE < 30% for 87% of cases on monthly scale, and for more than half of the areas on daily step, respectively. Overall, PMERA5-Land seems the best suited when complete required data set for calibration are missing. Except for PMERA5-Land, the alternative models gave ETo estimates with significantly (p < 0.05) larger nRMSE in the locations with a large u2 variance. This implies that u2 variation should also be considered for ETo simulation in windy environments. These results can expand our understanding on crop water demand estimation and drought monitoring in data-limited windy areas.
Similar content being viewed by others
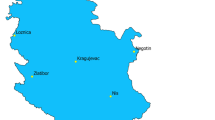
Explore related subjects
Discover the latest articles, news and stories from top researchers in related subjects.Avoid common mistakes on your manuscript.
1 Introduction
The lion’s share of renewable water consumption is dedicated to the agriculture sector on the globe (Huang et al. 2019; Morison et al. 2007). Thus, determining crop water requirement is of high importance to curb consumptive water use in water-scarce regions. The major component of crop water requirement includes replenishing soil moisture depleted by evapotranspiration (Jensen and Allen, 2016). Evapotranspiration (ET) is one of the most important components of the hydrological cycle, returning approximately 60% of global precipitated water back to the atmosphere (Hirschi et al. 2017). The ET is typically estimated rather than directly measured chiefly due to prohibitive costs and measurement-related technical difficulties (Qiu et al. 2022). Hitherto, some approaches such as the Ritchie’s method (Ritchie 1972; Sau et al. 2004), the complementary relationship (Brutsaert 2015; Brutsaert et al., 2020), the Budyko’s framework (Guo et al. 2019; Sposito 2017), remote sensing energy balance methods (Bastiaanssen et al. 1998), and the FAO-56 method (Allen et al. 1998, 2006) have been introduced to estimate evapotranspiration on different spatiotemporal scales. Among them, the FAO-56 approach has been broadly applied in agrohydrological studies in which evapotranspiration is estimated based on the reference evapotranspiration (ETo) and crop coefficient (kc). Evapotranspiration from a reference crop has been first proposed by Jensen (1968) and Wright and Jensen (1972) to avoid the ambiguities surrounding the concept of potential evapotranspiration. Doorenbos and Pruitt (1977) have defined the reference crop evapotranspiration as the rate of evapotranspiration from an extensive green grass with 8- to 15-cm height, actively growing and completely shading the ground without suffering disease, water, and nutrition stress.
The Penman–Monteith equation derived based on thermodynamic and aerodynamic principles is the most solid approach to estimate ETo in different circumstances (Allen, 1986; Monteith, 1965). Allen et al. (1998) parametrized the original Penman–Monteith equation for a reference surface with height of 12 cm, a fixed surface resistance of 70 s m−1, and an albedo of 0.23 (herein referred to as PM). ASCE (2005) also proposed a modified version of PM which performs very similarly to the PM developed by Allen et al. (1998) in daily or coarser steps for the short reference crop (Itenfisu et al. 2003). Although the PM’s superiority has been confirmed in different conditions (Allen et al. 2006; Pereira et al. 2015), it demands five datasets, i.e., minimum and maximum temperature (Tmin and Tmax), dew point temperature (Tdew) or relative humidity (RH), solar radiation (SR) or sunshine hour (SH), and near-surface wind speed. These datasets may be missing or of poor quality particularly in developing countries (Jensen et al. 1997; Nouri and Homaee 2018). For instance, SR (or SH) data are missing during 1979–1982 in Iran (Nouri and Homaee 2018). Wind speed at 2-m height (u2) has been reported as the most contributing factor affecting the ETo trend and dynamics in water-limited areas (Dinpashoh et al. 2011; McVicar et al. 2012; Nouri and Homaee 2018). Thus, ETo estimation seems a challenging task in water-limited environments under u2 data limitation.
Different data limitation scenarios for ETo modeling can be considered. Temperature data are the most available variables to estimate ETo. Therefore, a list of temperature-based methods has been proposed to estimate ETo under data scarcity (Allen et al. 1998; Blaney and Criddle 1950; Hargreaves and Samani 1982; Priestley and Taylor 1972; Thornthwaite 1948; Turc, 1961). The temperature-based PM (PMT) (Allen et al. 1998; Paredes and Pereira 2019) and Hargreaves-Samani (HS) (Hargreaves and Samani 1985; Samani 2000) are among the well-known temperature-based ETo models extensively employed to simulate ETo only based on Tmin and Tmax, in data-poor conditions. Unlike for non-windy environments, the original forms of temperature-based ETo models are mostly unable to simulate ETo with sufficient accuracy in the regions experiencing extreme wind speeds (Chen et al. 2005; Moratiel et al. 2020; Nouri and Homaee 2018). Nouri and Homaee (2018) and Moratiel et al. (2020) stated that u2 quantities beyond 2.5 m s−1 are likely to result in erroneous ETo estimation under data limitation.
In the cases when weather data are available for a while, some techniques have been introduced to reduce the error in ETo estimates which can be used in extreme conditions. The empirical constants of temperature-based equations can be updated to improve the accuracy of ETo estimates (Nouri and Homaee 2022; Raziei and Pereira 2013; Tabari and Talaee 2011). Moreover, local/regional u2 average (at different temporal scales) in lieu of the default value of 2 m s−1 suggested by Allen et al. (1998) has been also utilized to feed PMT (Nouri and Homaee 2018; Raziei and Pereira 2013; Trajkovic and Gocic 2021; Trajkovic and Kolakovic 2009). Such modifications have been often developed and evaluated for non-windy areas for the full-length datasets in the literature. However, it is more realistic to update temperature-based models for a limited time slice (i.e., calibration duration), and then evaluate the performance of updated equations for another time period (i.e., validation duration). This is more practical for the data-limited conditions in which PM-estimated ETo series are absent for a desired period. Moreover, previous studies have commonly focused on the u2 average, as a metric to assess the performance of temperature-based models. However, considering u2 variation, as one of the most important u2 characteristics, seems to be useful for the locations with high wind runs. It is noteworthy that u2 has relatively higher spatiotemporal variability, as it is a vector quantity but other variables are scalar quantities (Campbell and Norman 1998).
As noticed earlier, estimating ETo using modified temperature-based equations needs annual/seasonal/monthly u2 datasets in addition to complete Tmin and Tmax data. Besides complete Tmin and Tmax datasets, recalibration practice also requires complete ETo series at least for a limited period of time. For the scenario in which in situ measurements are absolutely unavailable (for example between the sites), reanalysis datasets can be applied to compute ETo by PM (Nouri and Homaee 2022; Paredes et al. 2018; Pelosi and Chirico 2021; Pelosi et al. 2020; Raziei and Parehkar 2021). Reanalysis data combine the forecasts and archived observations (e.g., in situ measurements and satellite retrievals) by using assimilation approaches to produce gridded weather datasets over long-term runs (Dee et al. 2014; Parker 2016). Recently, reanalysis datasets have received much attention in different disciplines to fill data gap, as they provide a broad range of climatic data over the globe across a wide range of spatiotemporal resolutions. The ERA5-Land is the state-of-the-art of reanalysis recently created by the European Centre for Medium-Range Weather Forecasts, which is the enhanced version of ERA5 (Muñoz-Sabater et al. 2021). Although ERA5-Land products have been widely employed in different fields (Cao et al. 2020; Ramirez Camargo and Schmidt 2020; Stefanidis et al. 2021; Wu et al. 2021), there are only two investigations using ERA5-Land to model ETo (Pelosi and Chirico 2021; Pelosi et al. 2020). Compared with the studies employing modified ETo models under data scarcity, there are a few studies applying reanalysis data to force PM (Nouri and Homaee 2022; Paredes et al. 2018; Pelosi and Chirico 2021; Pelosi et al. 2020; Raziei and Parehkar 2021). It is noteworthy that these studies applied the gridded forcings under non-extreme conditions.
Thus far, no study has been specifically conducted to examine the performance of different ETo modeling approaches for windy water-limited areas under different data limitation scenarios. Consequently, this study aimed to assess the performance of PMERA-Land, PMT fed with the default u2 value of 2 m s−1; local, seasonal, and monthly u2 averages; recalibrated PMT, and original and recalibrated HS against PM in some water-limited windy sites on both daily and monthly scales.
2 Methodology
2.1 The study area and observed data
Iran is a country lying in the Middle East with a broad range of climate regimes from hyper-arid to humid (Bannayan et al. 2020; Nouri and Homaee 2021a). This climate diversity is accounted for by the existence of the Alborz Mountains in northern Iran and the Zagros Mountains in western Iran (Fig. 1). There are some windy regions in the country which are suitable for building wind power plants (Mohammadzadeh Bina et al. 2018; Mostafaeipour et al. 2011). Climatic data are recorded by the Ministry of Energy (MOE) and the Meteorological Organization (IRIMO) in Iran (Nouri and Homaee 2021b, 2022). MOE records precipitation, Tmin and Tmax, on a monthly basis. IRIMO also records and archives a wide range of climatic data in hourly to monthly scales. But IRIMO data have a sparse spatial resolution, and are also missing for some time periods. In situ monthly temperature observations provided by both IRIMO and MOE are, however, available in a finer spatial resolution in Iran. In the present study, Tmin (at 2-m height), Tmax (at 2-m height), sunshine hour (SH), Tdew (at 2-m height), precipitation, and wind speed (at 10-m height, u10) were retrieved from IRIMO for 31 sites with a u2 average of 2.60 < m s−1 (Fig. 1, Table 1) (https://data.irimo.ir/login/login.aspx). It is noteworthy that the threshold value of 2.6 m s−1 was determined by plotting the percentile rank against u2 average for the studied areas (Table 1) and auxiliary datasets (146 sites) used by Nouri and Homaee (2018) (Table 1 and Fig. 1 in supplementary material). Accordingly, the value corresponding to the 80th percentile (~ 2.6 m s−1) was considered the threshold value for windy areas. Nouri and Homaee (2018) and Moratiel et al. (2020) proposed the threshold value of 2.5 m s−1 for windy areas, where the alternative models provide less reliable ETo estimates.
As solar radiation is not directly recorded in our study area, it was determined based on the Angstrom formula (Allen et al. 1998). The datasets were split into the calibration (9–10 years) and validation (8–10 years) sets based on the data availability (Table 1). As argued earlier, this is more realistic for data-sparse areas where required data are missing for a given time.
The climate of the sites was classified based on the aridity index (AI) presented by UNEP (1997). The AI values of < 0.05, 0.05–0.20, 0.20–0.50, 0.50–0.65, 0.65–1.00, and > 1.00 represent the hyper-arid, arid, semi-arid, dry sub-humid, moist sub-humid, and humid climates, respectively. The studied sites have an AI value of less than 0.65 (Table 1). These regions are called water-limited or drylands, where hydrological processes such as evapotranspiration crucially depend on water availability (Huang et al. 2017). The most arid and wettest surveyed areas were Zabol (with AI of 0.01) and Baneh (with AI of 0.46), respectively (Table 1).
Prior to application, the quality and integrity of data were evaluated. The time series were firstly plotted and checked visually. The trends in cumulative Tmin, u2, Tmax, Tmean, Tdew, and SH for each site were also compared with those obtained in adjacent sites as recommended by Pelosi and Chirico (2021). No anomaly in trends and time series was detected for Tmin, u2, Tmax, Tmean, Tdew, and SH. The outliers were examined based on first (Q1) and third (Q3) quartiles, and interquartile range (IQR = Q3 – Q1). The upper and lower limits of outliers were defined to be Q1 − (1.5 × IQR) and Q3 + (1.5 × IQR), respectively (Rousseeuw and Hubert 2011). Accordingly, less than 0.1% of daily Tmin, Tmax, Tmean, SH, and RH data were detected as the outliers. The 2.3%, on average, of daily u2 data were also categorized as the outliers. After consulting with the IRIMO experts, we did not remove the u2 data identified as the outliers, and considered them the extreme events. Note that the windy areas often experience extreme wind runs.
Less than 5% of daily Tmin, Tmax, Tmean, Tdew, and u2 data were missing during the calibration and validation sets (Tables 2 and 3 in supplementary material). The missing daily Tmin and Tmax were estimated by using daily Tmean according to Allen et al. (1998). However, more than 20% of daily SH data were missing in 17 sites for the validation period (Table 3 in supplementary material). For these cases, SR was approximated based on Tmin and Tmax as recommended by Allen (1996) and Samani (2000) (Eq. 4). According to Fig. 2 in the supplementary material, this method estimated SR with an acceptable accuracy in the calibration duration when the observed SH series are available.
2.2 Reanalysis data
The hourly and monthly ERA5-Land products were retrieved from https://cds.climate.copernicus.eu/. It is worth noting that ERA5-Land produces the climatic data for the horizontal resolution of 9 km, which is much finer as compared to ERA5 (31 km) and ERA-Interim (80 km) (Muñoz-Sabater et al. 2021). The temporal resolution of ERA5-Land is the same as that of ERA5. The ERA5-Land temperature (K), 10 m u- and v-components of wind speed (m s−1), surface solar radiation downwards (J m−2), and dew point temperature (K) were obtained. The lowest and highest diurnal temperature data were considered as Tmin and Tmax, respectively. Moreover, ERA5-Land u2 was computed by (https://confluence.ecmwf.int/pages/viewpage.action?pageId=133262398):
where ucom and vcom are, respectively, the 10 m u- and v-components of wind speed (the eastward and northward components of wind at 10 m height, m s−1), and α equals to 0.75 which converts u10 to u2 according to Allen et al. (1998). The Tmean was calculated as the arithmetic mean of daily Tmin and Tmax.
The reanalysis data of four pixels nearby each station were interpolated using the bilinear interpolation approach. Prior to interpolation, the Tmin and Tmax outputs were corrected using the environmental lapse rate (ELR), because the values of Tmin and Tmax are associated with the elevation at each grid. The ELR corrects the impact of elevation difference between the closest grid cells and a given site. The ELR algorithm was applied to the neighboring grids according to:
where TERA denotes ERA5-Land Tmin or Tmax products, α stands for the ELR coefficient, and Z and ZERA are, respectively, the elevation of the site and the pixel.
The ELR coefficient can be determined by regressing the temperature against the elevation. The slope of this linear regression approximately represents the ELR coefficient (Pelosi and Chirico 2021). Since the reliability of the correlations greatly depends on the data density, we used the monthly temperature data for 164 sites encompassing those used by Nouri and Homaee (2018) as well as those listed in Table 1. The correlation of Tmin and Tmax with elevation for each 12 months is shown in Figs. 3 and 4 in the supplementary material. The elevation variations explain at most 70% of Tmin and Tmax changes in our study area, illustrating that other factors, e.g., overlaying air masses and the terrain shape, contribute to the temperature deviation. The average linear ELR coefficient (α) for Tmin and Tmax was calculated to be − 0.0064 and − 0.0052 °C/m, respectively (Figs. 3 and 4 in supplementary material). The rule-of-thumb value of − 0.0065 °C/m is considered for the linear ELR in the literature (Dutra et al. 2020). This ELR value (− 0.0065 °C/m) is very close to that obtained for the Tmin correction; however, it somewhat differs from that we determined for Tmax particularly in summertime.
2.3 The ETo models
The benchmark ETo (mm day−1) was estimated by using PM at both daily and monthly scales:
where ETo is the reference crop evapotranspiration (mm day–1), Δ is the slope of saturation vapor pressure curve (kPa °C−1), Rn is the net radiation at the reference crop surface (MJ m−2 day−1), G is the soil heat flux density (MJ m−2 day−1) which is considered zero for daily scale, and approximated by Tmean,i+1 − Tmean,i−1 on monthly scale, Tmean is the daily mean air temperature at 1.5- to 2.5-m height (°C), U is the average wind speed at 2 m height (m s−1), es is the saturation vapor pressure at 1.5- to 2.5-m height (kPa), ea is the actual vapor pressure at 1.5- to 2.5-m height (kPa), es-ea is the vapor pressure deficit (VPD) at 1.5 to 2.5 m height (kPa), and γ represents the psychrometric constant (kPa °C−1).
The PMERA5-Land is the PM fed with the aforementioned ERA5-Land simulations. In order to assess the sensitivity of ETo estimates to error in a given reanalysis product, PM was forced by the ground measurements and a specific ERA5-Land product. The PMERA5-LandX indicates PM model run by “X” product of ERA5-Land package as well as ground observations. For instance, PMERA5-LandTmin is the PM forced by observed Tmax, Tdew, SR, and u2 series and ERA5-Land Tmin product.
To compute ETo by PMT, Allen et al. (1998) represented some relationships to approximate VPD and Rn from Tmin and Tmax. When no SR (or SH) data are available, it can be estimated by use of the Hargreaves’ radiation formula:
where Ra is the extraterrestrial radiation, and Krs is an empirical constant suggested to be 0.16 for interior cases or 0.19 (°C−0.5) for coastal locations (Samani 2000).
In case Tdew (or RH) is missing, ea can be approximated by:
In Eq. 5, Tdew is assumed to be equal to Tmin which is valid only in moist sub-humid climate. Thus, the following relationships have been proposed for other climatic regimes: Tdew = Tmin − 4 in hyper-arid areas, Tdew = Tmin − 2 for arid regimes, Tdew = Tmin − 1 for semi-arid/dry sub-humid environments, and Tdew = Tmean − 2 for humid regions (Paredes and Pereira 2019; Todorovic et al. 2013).
For the cases in which u2 is unavailable, Allen et al. (1998) has suggested using 2 m s−1 (the u2 averaged for 2000 stations on the globe). In addition to the value of 2 m s−1, we considered the local, monthly, and seasonal u2 average for the period of calibration given in Table 1. The PMT fed with the default value of 2 m s−1, local, monthly, and seasonal u2 average are hereafter referred to as PMT2, PMTua, PMTus, and PMTum, respectively.
The HS was computed as follows (Hargreaves and Samani 1985; Samani 2000):
As mentioned earlier, the original forms of PMT and HS use the Krs values of 0.16 or 0.19 °C−0.5. In this study, we also readjusted Krs by the generalized reduced gradient nonlinear optimization algorithm established by Lasdon et al. (1978) using monthly ETo datasets for the calibration period (Table 1). The recalibrated HS and PMT are referred to as RHS and RPMT, respectively. The objective function (OF) for optimization was:
where nRMSE denotes the normalized root mean square error (refer to Sect. 2.4 and Eq. 8).
The readjusted Krs values are listed in Tables 2 and 3. The monthly data were employed for the recalibration as they are more likely to be available in data-poor areas.
Analysis of variance (ANOVA) was utilized to identify the influence of u2 average and variance on error in ETo estimated by the investigated ETo models. The significance of effects was tested using the F-test (Fisher’s test).
2.4 Accuracy indicator
The performance of alternative models against PM and the error in the reanalysis forcings were analyzed by using the nRMSE and relative mean bias error (rMBE) during the validation step (Table 1):
where Xo and Xs are, respectively, the observations and simulations,\(\overline{{X }_{o}}\) represents the average of observed values, and n is the number of pair comparisons.
The rMBE is a statistic widely utilized to quantify the model bias error. The 0.0% of rMBE denotes no bias. The negative and positive values of rMBE indicate the model underestimation and overestimation, respectively. The performance of alternative models is perfect for nRMSE of 10% > , good when the metric varies from 10 to 20%, fair when nRMSE is between 20 and 30%, and poor (unreliable) for nRMSE of 30% < (Dettori et al. 2011; Ku et al. 2018; Nouri and Homaee 2022).
3 Results and discussion
3.1 Wind speed characteristics
The u2 average varies from 2.6 m s−1 (in Qeshm) to 4.8 m s−1 (Zabol) in the calibration period. The highest u2 average was calculated for Zabol (4.8 m s−1) in the calibration duration followed by Firozkuh (GAW) (4.3 m s−1) and Manjil (4.2 m s−1) (Fig. 2a). Figure 2b and c show that the locations lying on the flanks of the Zagros Mountains and the stations situated along the northern strips of the Persian Gulf and the Gulf of Oman had a lower monthly (0.8 > m2 s−2) and daily (4.0 > m2 s−2) u2 variance. A higher u2 variation was, however, found for the mountainous sites located in the Alborz (i.e., Firozkuh (GAW) and Manjil) and the sites surrounding the Dasht-e-Kavir (i.e., Damghan, Naien, Ardestan, Sabzevar, and Kahak). Zabol and Zahak sites exhibited the highest and third-highest u2 variance, respectively. These two hyper-arid windy locations are affected by summertime wind extremes, which cause dust-related problems in southeastern Iran (Alizadeh-Choobari et al. 2014). Three classes of “a,” “b,” and “c” were also defined for u2 average and variance values. The range of u2 average was 2.7–3.0, 3.0–3.5, and 3.5–4.8 m s−1 for “a,” “b,” and “c” classes, respectively. On monthly scale, u2 variance varied in the range of 1.3–2.5, 2.5–3.5, and 3.5–11.0 m2 s−2, respectively. Daily u2 variance also ranged from 0.1 to 0.5 m2 s−2 in class “a,” 0.1 to 0.5 m2 s−2 in class “b,” and 0.8 to 5.0 m2 s−2 in class “c.”
Figure 3 shows the correlation strength between u2 and the PM-estimated ETo in our studied areas. Since the ETo series are characterized by the seasonality, the associations were assessed in monthly intervals. The average correlation coefficients were determined to be 0.29, 0.39, 0.62, and 0.53 during the December-January–February (DJF, winter), March–April-May (MAM, spring), June-July–August (JJA, summer), and September–October-November (SON, autumn) periods, respectively. Consequently, it can be concluded that more than half of the ETo variations can be accounted for by the u2 variations in summer and autumn, demonstrating that the u2 variability substantially contributes towards the summer and autumn ETo variability. It also implies that reducing u2 can result in a considerable decline in ETo during summer when the agricultural water demand is at its maximum value. Nouri et al. (2017) and Dinpashoh et al. (2011) also found u2 as the most contributing factor affecting the ETo dynamics in water-limited areas of Iran.
3.2 Error analysis for monthly ETo
The nRMSE of monthly PMT2-estimated ETo ranged from 14.3 to 56.0% (with an average of 29.8%) for the regions studied. The PMT2 modeled monthly ETo with a nRMSE exceeding 30% in 44.1% of areas (Fig. 4(a)). Application of average local u2 instead of the default u2 value to run PMT decreased the average nRMSE from 29.8 to 21.9%. The monthly PMTua-estimated ETo also showed a nRMSE above 30% for 29.4% of studied areas (Fig. 4(b)). The PMTua estimated monthly ETo reliably (i.e., nRMSE < 30%) for three sites (Salafchegan, Hendijan, and Delijan) with monthly u2 variance below 0.80 m2 s−2, where PMT2 performed poorly. However, PMTua provided monthly ETo estimates with an acceptable accuracy in only 2 (i.e., Damghan and Kahak) out of 11 windy areas with a large monthly u2 variance (0.80 < m2 s−2). This implies that application of constant local u2 average instead of 2 m s−1 is unlikely to improve the accuracy of ETo estimates under data limitation for the locations with a large u2 variance. On monthly scale, the superiority of PMTua against PMT2 has been confirmed in some literature (Nouri and Homaee 2018; Raziei and Pereira 2013; Trajkovic and Gocic 2021). However, for the windy cases with high summertime u2 which leads to an increased u2 variance, PMTua also provided poor ETo estimates (Nouri and Homaee 2018). Figure 4(e) shows that RPMT gave reliable monthly ETo estimates in all investigated sites.
The PM forced with ERA5-Land outputs produced monthly ETo with an average nRMSE of 22.4%. A nRMSE exceeding 30% was computed for monthly PMERA5-Land-estimated ETo in four sites including Manjil, Ardestan, Damghan, and Aligodarz (Fig. 4(h)). Similar to PMTua, PMTus and PMTum provided accurate monthly ETo estimates for the regions with a low monthly u2 variance (0.80 > m2 s−2) (Fig. 4(c, d)). The ETo was also modeled accurately by PMTus and PMTum in 7 out of 11 windy sites where monthly u2 varied in a larger range (0.80 < m2 s−2). The nRMSE fell to less than 30% by using PMTus and PMTum in five windy sites with a monthly u2 variance exceeding 0.91 m2 s−2 (i.e., Manjil, Torbat-e-jam, Zabol, Sahand, and Sabzevar), wherein PMT2 and PMTua gave erroneous monthly ETo estimates. However, no version of PMT estimated monthly ETo reliably (i.e., nRMSE < 30%) for four windy areas of Zahak, Firozkuh (GAW), Ardestan, and Naien with a high u2 variance (Fig. 4(a–d)). The average difference between the nRMSE of PMT2-simulated ETo and the nRMSE of PMTua-, PMTus-, and PMTum-estimated ETo was around 7.0% for the areas with a low monthly u2 variance (0.80 > m2 s−2), and more than 10% for the locations with a large monthly u2 variance (0.80 < m2 s−2). Thus, using seasonal/monthly u2 average appears to enhance the accuracy of monthly ETo estimates for the windy environments with large u2 variations. Because PMTus and PMTum performed similarly for almost all cases, one can sufficiently improve the PMT performance on monthly scale using only seasonal u2 series.
The HS provided the monthly ETo estimates with a nRMSE of 30% < for 58.8% of the studied regions (Fig. 4(f)). The HS performed poorly (i.e., nRMSE > 30%) in 39% of the windy areas with a low u2 variance (0.8 > m2 s−2) and all windy sites with a high u2 variance (0.8 < m2 s−2). Therefore, original HS is not suited to estimate monthly ETo in data-limited windy areas with large monthly u2 variations. The recalibration decreased the average nRMSE of monthly ETo estimates from 29.8% (for HS) to 16.1% (for RHS). The monthly ETo was modeled reasonably well by RPMT and RHS for all sites except Manjil which is characterized with complex terrains (Fig. 4(e, g)). This highlights the significance of recalibration for accurately estimation of monthly ETo in windy environments. It should be noted that recalibration needs the PM-estimated ETo series at least for a limited time period which are often missing in data-limited areas. The PMTum, PMTus, and PMERA5-Land simulated monthly ETo with an acceptable accuracy for 87% of the cases studied. Thus, when the PM-estimated ETo series are unavailable, PMTum, PMTus, and PMERA5-Land can be used to reliably estimate monthly ETo in windy sites. However, since PMERA5-Land needs no ground measurements, it seems preferable for data-poor regions.
For most cases, there was an insignificant difference (p > 0.05) between the means of nRMSE values calculated in different u2 average classes (Fig. 5(a)). Except for PMERA5-Land, the average nRMSE was significantly higher in the sites with a larger u2 variance (i.e., class “c”) with respect to that obtained for the sites grouped in “a” and “b” classes (Fig. 5(b)). As already noticed, the alternative models gave erroneous ETo estimates for the regions with monthly u2 variance larger than 0.8 m2 s−2 (class “c” of u2 variance). The u2 variance seems thus to be more contributing to the absolute error of monthly ETo estimates than the u2 average. In other words, the u2 variance seems to be a more important factor as compared to the u2 average for modeling ETo with reduced datasets in windy environments. The ETo may be simulated more accurately in a region with a higher u2 average but a lower u2 variance with respect to an area with a lower u2 average but a larger u2 variance. For instance, PMT2, PMTua, PMTus, PMTum, HS, and RHS provided more accurate ETo results for TIA with the u2 average of 3.7 m s−1 and the u2 variance of 0.5 m2 s−2 relative to Damghan having the u2 average of 2.8 m s−1 and the u2 variance of 1.1 m2 s−2.
The box plots of the nRMSE (%) of monthly ETo estimated by the studied models in different monthly u2 average and variance classes (“a,” “b,” and “c”). (The boxes’ boundaries indicate the 25th and 75th percentiles, the lines within the boxes mark the median, and the inner and outer fences represent the lowest and highest values, respectively. Furthermore, different capital letters indicate the significant difference at 95% probability level)
The average rMBE values obtained for PMT2, PMTua, PMTus, PMTum, HS, and PMERA5-Land ranged from − 8.4 to − 23.8%, demonstrating the models’ tendency to underestimate monthly ETo (Fig. 6). The average rMBE was calculated to be + 3.3% and + 0.3% for RHS and RPMT, respectively. Hence, RHS and RPMT did not show a clear tendency to overestimate or underestimate (Fig. 6(e, g)). It seems that recalibration corrected the bias error of the temperature-based models. The PMT2, PMTua, and HS estimate ETo with a higher accuracy within the u2 range of 1.5–2.5 m s−1 (Moratiel et al. 2020; Nouri and Homaee 2018). Therefore, these temperature-based equations are anticipated to underestimate for the regions in which u2 is beyond 2.5 m s−1 (like our studied areas).
3.3 Error analysis for daily ETo
The average nRMSE of daily PMT2- and PMTua-estimated ETo exceeded 30%. The daily ETo was modeled inaccurately (i.e., nRMSE > 30%) for the majority of sites (Fig. 7(a, b)). The average daily ETo simulated by PMTus, PMTum, RPMT, and PMERA5-Land varied in the range of 25.6–29.2%. At daily scale, RPMT and PMERA5-Land performed satisfactorily (i.e., nRMSE < 30%) for more than two-third of surveyed stations (Fig. 7(e, h)). Similar to monthly scale, PMT2, PMTua, PMTus, PMTum, and PMERA5-Land underestimated daily ETo (Fig. 8(a–h)). It seems that although averaged values of monthly/seasonal u2 may explain monthly u2 variations and enhance the accuracy of monthly ETo estimates, they failed to consider daily u2 variability and improve the ETo estimation on daily basis. The PM-based alternatives simulated monthly ETo reliably (i.e., nRMSE < 30%), but daily ETo inaccurately (i.e., nRMSE > 30%) for 19.3 to 32.2% of studied locations (Figs. 4 and 7). Despite that monthly and daily average values of the climatic factors are the same, the larger variation in daily scale, in particular the u2 variation in the windy sites, explains the higher error in daily ETo as compared with monthly ETo. For instance, daily and monthly u2 variance was found to be 0.99 and 3.58 m2 s−2, on average, respectively (Fig. 2). Similar to monthly ETo, the average error in daily ETo differs insignificantly (p > 0.05) across three u2 average classes (Fig. 9(a)). However, a statistically significant difference was detected between the mean of nRMSE obtained in class “c” of daily u2 variance relative to that determined for class “a” and “b” (Fig. 9(b)).
The box plots of the nRMSE (%) of daily ETo estimated by the studied models in different daily u2 average and variance classes (“a,” “b,” and “c”). (The boxes’ boundaries indicate the 25th and 75th percentiles, the lines within the boxes mark the median, and the inner and outer fences represent the lowest and highest values, respectively. Furthermore, different capital letters indicate the significant difference at 95% probability level)
The HS modeled daily ETo with a nRMSE above 30% for 82.6% of cases, demonstrating poor performance of original HS on daily scale in the windy environments (Fig. 7(f)). Daily ETo was, however, simulated unreliably by RHS at only six windy sites, i.e., Zabol, Zahak, Bilesawar, Ardebil, Damghan, and Manjil (19.4% of cases), the sites with a daily u2 variance larger than 4.0 m2 s−2 (Fig. 7(g)). There was a tendency towards underestimation of daily ETo by HS for all areas (Fig. 8(f)). The RHS and RPMT did not, however, show a clear pattern of underestimation/overestimation over the study area (Fig. 8(e, g)). Considering the reliable performance of RHS and RPMT for the majority of stations (Fig. 7(e, g)), updating Krs based on monthly datasets for a limited period (e.g., 10 years) is likely to increase the accuracy of daily ETo modeling by using reduced datasets for the windy environments. This has been also proven in the related literature (Nouri and Homaee 2018, 2022; Raziei and Pereira 2013; Tabari and Talaee 2011).
Raziei and Pereira (2013) also applied RHS and RPMT to estimate daily ETo in 40 sites in Iran, 3 of which (Sabzevar, Bam and Zabol) are windy. They reported a RMSE of 0.62, 0.34, and 2.86 mm day−1 for daily ETo in Sabzevar, Bam, and Zabol, respectively. Given to the average daily ETo of 3.9, 5.3, and 7.4 mm day−1 in Sabzevar, Bam, and Zabol during 1971–2005 (the study period in Raziei and Pereira (2013)), the nRMSE was calculated to be 15.9%, 6.4%, and 38.6% in Sabzevar, Bam, and Zabol, respectively. Our results also indicate acceptable performance of RHS and RPMT (nRMSE < 30%) for Sabzevar and Bam, and poor performance of these equations (i.e., nRMSE > 30%) in Zabol, which are in line with the results of Raziei and Pereira (2013). The readjusted Krs values listed in Tables 2 and 3 differ to some extent from those presented in the related literature. For instance, the updated Krs for HS was 0.249 for Bam in the present study (Table 2), whereas it has been reported to be 0.259 (in 1994–2005) and 0.193 (during 1971–2005) by Tabari and Talaee (2011) and Raziei and Pereira (2013), respectively. This can be attributed to the difference in the study length, climate variability, and the recalibration method used in these works. For the case of Bam, the average u2 is, respectively, 1.82, 2.28, and 2.95 m s−1 during 1971–2005, 1994–2005, and 2001–2010 (the calibration period in the current study). This high u2 variation is likely to result in different readjusted Krs quantities for this windy area. Nouri and Homaee (2018), Nouri and Homaee (2022), and Ravazzani et al. (2012) warned against utilizing updated empirical constants obtained for a specific time period to simulate ETo for the other durations. They also concluded that recalibrating temperature-based models by the readjusted constants reported in the literature may worsen the accuracy of ETo estimation in data-limited conditions under climate change.
The most reliable daily and monthly ETo estimates were provided by RHS and RPMT for the majority of windy cases. In case complete monthly datasets are available for a while, RHS and RPMT can thus be the most suited alternatives to model ETo in data-scarce windy areas. At daily scale, PMERA5-Land outperformed PMT2, PMTua, PMTus, PMTum, and HS for about 58% of windy cases. This might be ascribed to the fact that ERA5-Land provides more realistic u2 dynamics as compared to considering fixed u2 values. When complete monthly ETo series do not exist, the PM forced by ERA5-Land outputs seems to give more accurate daily ETo estimates for the windy sites. In addition, PMERA5-Land estimated daily ETo with an adequate accuracy in Ardebil, Zahak, and Zabol, three sites where the u2 variation is appreciable and all other models performed unsatisfactorily (Fig. 7).
Figure 10 shows the nRMSE of ERA5-Land u2, SR, Tmin, Tmax, Tdew, and Tmean simulations for our studied areas. The nRMSE obtained for daily and monthly Tdew and u2 exceeded 30% for more than 87% of the surveyed sites. The unsatisfactorily daily and monthly Tmin estimates (i.e., nRMSE > 30%) were also found for 48.4 and 45.2% of the sites investigated, respectively. However, there was an acceptable absolute error (i.e., nRMSE < 30%) for Tmax, Tmean, and SR in more than 90% of sites on both daily and monthly scales. Hence, Tmean, which is directly considered in PM (refer to Eq. 3), was satisfactorily reconstructed by ERA5-Land for the majority of cases. Consequently, u2 and Tdew were most prone to error. This has been also indicated in the literature (Aboelkhair et al. 2019; Nouri and Homaee 2022; Raziei and Parehkar 2021; Ricard and Anctil 2019). ERA5-Land underestimated u2, Tmin, Tmax, Tmean, and Tdew, and overestimated SR for the most cases (Fig. 11). The sensitivity of PM-estimated ETo to error in Tdew, Tmin, Tmax, u2, and SR products is shown in Fig. 12. The average nRMSE of ETo simulated by PMERA5-LandTmin, PMERA5-LandTmax, PMERA5-LandTdew, and PMERA5-LandSR did not exceed 7.2%. However, there were nRMSE values of 27.9% and 22.8%, on average, for daily and monthly ETo modeled by PMERA5-Landu2, respectively. This illustrates that error in u2 estimates contributes substantially to error in ETo estimates. In other words, error in u2 influences more significantly the accuracy of ETo results, since the aerodynamic component of the evapotranspiration process is dominant in windy conditions. The greater sensitivity of ETo estimates to error in u2 reanalysis data has been also shown by Pelosi and Chirico (2021). Pelosi et al. (2020) also associated the weaker performance of the PM forced by UERRA MESCAN-SURFEX data during April, May, and September, when the aerodynamic term is prevalent, with the high uncertainty in u2 forcing. As u2 is oftentimes produced with an insufficient accuracy by reanalyses (Nouri and Homaee 2022; Raziei and Parehkar 2021; Ricard and Anctil 2019), improvement in accuracy of u2 products can highly enhance the accuracy of ETo estimated by using reanalyses.
The box plots of the nRMSE (%) of monthly and daily ETo estimated by PMERA5-LandTmin, PMERA5-LandTmax, PMERA5-LandSR, PMERA5-Landu2, and PMERA5-LandTdew. (The PMERA5-Landx indicates the PM fed by the in-situ measurements and x from ERA5-Land products. The values on the upper whisker boundary indicate the average of nRMSE (%) quantities. The boxes’ boundaries indicate the 25th and 75th percentiles, the lines within the boxes mark the median, and the inner and outer fences represent the lowest and highest values, respectively)
The PMTua, PMTum, PMTus, RPMT, and RHS performed weaker against the original forms (PMT2 and HS) in Bilesawar, a northwestern windy location. This can be elucidated by the large difference between the u2 average in the calibration and validation periods. The u2 average is 3.8 and 2.5 m s−1 in the calibration and validation sets in Bilesawar, respectively (Fig. 13). This can be ascribed to the impacts of climate change and variability and/or construction activities around the weather station on the u2 trend. Similar to recalibration, using local, seasonal, and monthly u2 average values available for a limited duration may deteriorate the accuracy of ETo estimates.
Importing data from nearby sites and geostatistical interpolation are the other alternatives to model ETo under data limitation (Allen et al. 1998; Nouri and Homaee 2022; Pelosi et al. 2020; Tomas-Burguera et al. 2018, 2017). However, the accuracy of these approaches strongly relies on the density and distribution of nearby sites (Tomas-Burguera et al. 2018). When the distance between sites is quite large and data density is low, ETo may not be modeled reliably by using the abovementioned methods. Nouri and Homaee (2022) concluded that the PM forced with reanalysis data performed more accurately with respect to the PM fed by interpolated variables in Iran. The u2 spatial variability is also another uncertainty source for these approaches in windy environments. The investigated windy sites are mostly surrounded with non-windy areas. As an instance, Zabol and Zahak, two southeastern windy sites, are neighbored with three non-windy sites, i.e., Nehbandan, Zahdan, and Birjand with the average long-term u2 ranging from 1.75 to 2.48 m s−1. Consequently, using u2 data from neighboring sites and interpolating ETo may not be promising alternatives to estimate ETo in such windy regions. Nouri and Homaee (2022) reported a relatively low accuracy for interpolation-based ETo estimates in Zabol and Zahak due to data sparsity and high u2 variability.
4 Conclusions
The Penman–Monteith FAO-56 (PM) forced with ERA5-Land products (PMERA5-Land), temperature-based PM computed by the default 2-m wind speed (u2) of 2 m s−1 (PMT2), local u2 (PMTua), seasonal u2 (PMTus), and monthly u2 average (PMTum), Hargreaves-Samani (HS), recalibrated PMT (RPMT), and recalibrated HS (RHS) were employed to model reference evapotranspiration (ETo) under different data limitation scenarios in some water-limited windy areas. The uncalibrated models gave inaccurate daily ETo estimates in the majority of cases. The recalibrated models, however, estimated monthly and daily ETo with an acceptable accuracy for more than 80% of cases. The PMERA5-Land also produced daily and monthly ETo estimates with an adequate accuracy in the most windy cases. Although readjusting the empirical coefficients of temperature-based models highly improves the accuracy of ETo results, it is burdened with complete monthly weather datasets which are often missing in data-scarce regions. As a result, when complete monthly datasets do not exist, the PM fed with ERA5-Land data is likely to be the best option in different temporal resolutions under windy conditions. The fact that PMERA5-Land requires no in situ recordings highlights further the importance of using ERA5-Land forcings in windy ungagged areas. Given that the long-term ERA5-Land outputs are available in raster format and different time steps at a relatively fine spatial resolution, these datasets can be applied to feed decision support systems under data limitation.
Data availability
The observed metrological data were retrieved from https://data.irimo.ir/login/login.aspx. The ERA5-Land reanalyses can be downloaded from https://cds.climate.copernicus.eu/.
Code availability
Not applicable.
References
Aboelkhair H, Morsy M, El Afandi G (2019) Assessment of agroclimatology NASA POWER reanalysis datasets for temperature types and relative humidity at 2 m against ground observations over Egypt. Adv Space Res 64:129–142. https://doi.org/10.1016/j.asr.2019.03.032
Alizadeh-Choobari O, Zawar-Reza P, Sturman A (2014) The “wind of 120days” and dust storm activity over the Sistan Basin. Atmos Res 143:328–341. https://doi.org/10.1016/j.atmosres.2014.02.001
Allen RG (1986) A Penman for all seasons. J Irrig Drain Eng 112:348–368. https://doi.org/10.1061/(ASCE)0733-9437(1986)112:4(348)
Allen RG (1996) Assessing integrity of weather data for reference evapotranspiration estimation. J Irrig Drain Eng 122:97–106. https://doi.org/10.1061/(ASCE)0733-9437(1996)122:2(97)
Allen RG, Pereira LS, Raes D, Smith M (1998) Crop evapotranspiration-guidelines for computing crop water requirements-FAO Irrigation and drainage paper 56. Food and Agriculture Organization of the United Nations, Rome, Italy, p. 300
Allen RG, Pruitt WO, Wright JL, Howell TA, Ventura F, Snyder R, Itenfisu D, Steduto P, Berengena J, Yrisarry JB, Smith M, Pereira LS, Raes D, Perrier A, Alves I, Walter I, Elliott R (2006) A recommendation on standardized surface resistance for hourly calculation of reference ETo by the FAO56 Penman-Monteith method. Agric Water Manage 81:1–22. https://doi.org/10.1016/j.agwat.2005.03.007
ASCE (2005) The ASCE Standardized Reference Evapotranspiration Equation. In: Richard GA, Walter IA, Elliott R, Howell T, Itenfisu D, Jensen M (Eds) Task Committee on Standardization of Reference Evapotranspiration, Environmental and Water Resources Institute of the American Society of Civil Engineers, p. 59
Bannayan M, Asadi S, Nouri M, Yaghoubi F (2020) Time trend analysis of some agroclimatic variables during the last half century over Iran. Theor Appl Climatol 140:839–857. https://doi.org/10.1007/s00704-020-03105-7
Bastiaanssen WGM, Menenti M, Feddes RA, Holtslag AAM (1998) A remote sensing surface energy balance algorithm for land (SEBAL). 1. Formulation J Hydrol 212–213:198–212. https://doi.org/10.1016/S0022-1694(98)00253-4
Blaney HF, Criddle WD (1950) Determining water requirements in irrigated areas from climatological and irrigation data. Soil Conservation Service Technical Paper 96, Soil Conservation Service. US Department of Agriculture, Washington, USA, p. 44
Brutsaert W (2015) A generalized complementary principle with physical constraints for land-surface evaporation. Water Resour Res 51:8087–8093. https://doi.org/10.1002/2015WR017720
Brutsaert W, Cheng L, Zhang L (2020) Spatial distribution of global landscape evaporation in the early twenty-first century by means of a generalized complementary approach. J Hydrometeorol 21:287–298. https://doi.org/10.1175/JHM-D-19-0208.1
Campbell GS, Norman JM (1998) Wind. In: Campbell GS, Norman JM (eds) An introduction to environmental biophysics. Springer, New York, New York, NY, pp 63–75
Cao B, Gruber S, Zheng D, Li X (2020) The ERA5-Land soil temperature bias in permafrost regions. Cryosphere 14:2581–2595. https://doi.org/10.5194/tc-14-2581-2020
Chen D, Gao G, Xu C-Y, Guo J, Ren G (2005) Comparison of the Thornthwaite method and pan data with the standard Penman-Monteith estimates of reference evapotranspiration in China. Clim Res 28:123–132. https://doi.org/10.3354/cr028123
Dee DP, Balmaseda M, Balsamo G, Engelen R, Simmons AJ, Thépaut JN (2014) Toward a consistent reanalysis of the climate system. Bull Am Meteorol Soc 95:1235–1248. https://doi.org/10.1175/bams-d-13-00043.1
Dettori M, Cesaraccio C, Motroni A, Spano D, Duce P (2011) Using CERES-Wheat to simulate durum wheat production and phenology in Southern Sardinia, Italy. Field Crop Res 120:179–188. https://doi.org/10.1016/j.fcr.2010.09.008
Dinpashoh Y, Jhajharia D, Fakheri-Fard A, Singh VP, Kahya E (2011) Trends in reference crop evapotranspiration over Iran. J Hydrol 399:422–433. https://doi.org/10.1016/j.jhydrol.2011.01.021
Doorenbos J, Pruitt WO (1977) Guidelines for predicting crop water requirements. FAO irrigation and drainge papers, No. 24, Food and Agricultural Organization of the United Nations, Rome, Italy, p. 154
Dutra E, Muñoz-Sabater J, Boussetta S, Komori T, Hirahara S, Balsamo G (2020) Environmental lapse rate for high-resolution land surface downscaling: an application to ERA5. Earth and Space Science 7:e2019EA000984. https://doi.org/10.1029/2019EA000984
Guo A, Chang J, Wang Y, Huang Q, Guo Z, Li Y (2019) Uncertainty analysis of water availability assessment through the Budyko framework. J Hydrol 576:396–407. https://doi.org/10.1016/j.jhydrol.2019.06.033
Hargreaves G, Samani Z (1982) Estimating potential evapotranspiration. J Irrig Drain Div 108:225–230
Hargreaves G, Samani Z (1985) Reference crop evapotranspiration from temperature. Appl Eng Agric 1:96. https://doi.org/10.13031/2013.26773
Hirschi M, Michel D, Lehner I, Seneviratne SI (2017) A site-level comparison of lysimeter and eddy covariance flux measurements of evapotranspiration. Hydrol Earth Syst Sci 21:1809–1825. https://doi.org/10.5194/hess-21-1809-2017
Huang J, Li Y, Fu C, Chen F, Fu Q, Dai A, Shinoda M, Ma Z, Guo W, Li Z, Zhang L, Liu Y, Yu H, He Y, Xie Y, Guan X, Ji M, Lin L, Wang S, Yan H, Wang G (2017) Dryland climate change: recent progress and challenges. Rev Geophys 55:719–778. https://doi.org/10.1002/2016rg000550
Huang Z, Hejazi M, Tang Q, Vernon CR, Liu Y, Chen M, Calvin K (2019) Global agricultural green and blue water consumption under future climate and land use changes. J Hydrol 574:242–256. https://doi.org/10.1016/j.jhydrol.2019.04.046
Itenfisu D, Elliott Ronald L, Allen Richard G, Walter Ivan A (2003) Comparison of reference evapotranspiration calculations as part of the ASCE standardization effort. J Irrig Drain Eng 129:440–448. https://doi.org/10.1061/(ASCE)0733-9437(2003)129:6(440)
Jensen DT, Hargreaves GH, Temesgen B, Allen RG (1997) Computation of ETo under nonideal conditions. J Irrig Drain Eng 123:394–400. https://doi.org/10.1061/(ASCE)0733-9437(1997)123:5(394)
Jensen ME (1968) Water consumption by agricultural plants. In: Kozlowski TT (ed) Water deficits and plant growth, vol 2. Academic Press. New York, USA, pp 1–22
Jensen ME, Allen R, G. (2016) Estimates of irrigation water requirements and streamflow depletion. in Jensen ME, Allen Richard G (eds.) Evaporation, Evapotranspiration, and Irrigation Water Requirements, 2nd edn. ASCE Manuals and Reports on Engineering Practice No. 70, pp. 435–455
Ku H-H, Jeong C, Colyer P (2018) Modeling long-term effects of hairy vetch cultivation on cotton production in Northwest Louisiana. Sci Total Environ 624:744–752. https://doi.org/10.1016/j.scitotenv.2017.12.165
Lasdon LS, Waren AD, Jain A, Ratner M (1978) Design and testing of a generalized reduced gradient code for nonlinear programming. ACM Trans Math Software (TOMS) 4:34–50
McVicar TR, Roderick ML, Donohue RJ, Li LT, Van Niel TG, Thomas A, Grieser J, Jhajharia D, Himri Y, Mahowald NM, Mescherskaya AV, Kruger AC, Rehman S, Dinpashoh Y (2012) Global review and synthesis of trends in observed terrestrial near-surface wind speeds: Implications for evaporation. J Hydrol 416–417:182–205. https://doi.org/10.1016/j.jhydrol.2011.10.024
Mohammadzadeh Bina S, Jalilinasrabady S, Fujii H, Farabi-Asl H (2018) A comprehensive approach for wind power plant potential assessment, application to northwestern Iran. Energy 164:344–358. https://doi.org/10.1016/j.energy.2018.08.211
Monteith JL (1965) Evaporation and environment. Symposia of the Society for Experimental Biology:205–234
Moratiel R, Bravo R, Saa A, Tarquis AM, Almorox J (2020) Estimation of evapotranspiration by the Food and Agricultural Organization of the United Nations (FAO) Penman-Monteith temperature (PMT) and Hargreaves-Samani (HS) models under temporal and spatial criteria – a case study in Duero basin (Spain). Nat Hazard 20:859–875. https://doi.org/10.5194/nhess-20-859-2020
Morison JIL, Baker NR, Mullineaux PM, Davies WJ (2007) Improving water use in crop production. Philos Trans R Soc Lond B Biol Sci 363:639–658. https://doi.org/10.1098/rstb.2007.2175
Mostafaeipour A, Sedaghat A, Dehghan-Niri AA, Kalantar V (2011) Wind energy feasibility study for city of Shahrbabak in Iran. Renew Sustain Energy Rev 15:2545–2556. https://doi.org/10.1016/j.rser.2011.02.030
Muñoz-Sabater J, Dutra E, Agustí-Panareda A, Albergel C, Arduini G, Balsamo G, Boussetta S, Choulga M, Harrigan S, Hersbach H, Martens B, Miralles DG, Piles M, Rodríguez-Fernández NJ, Zsoter E, Buontempo C, Thépaut JN (2021) ERA5-Land: a state-of-the-art global reanalysis dataset for land applications. Earth Syst Sci Data Discuss 2021:1–50. https://doi.org/10.5194/essd-2021-82
Nouri M, Homaee M (2018) On modeling reference crop evapotranspiration under lack of reliable data over Iran. J Hydrol 566:705–718. https://doi.org/10.1016/j.jhydrol.2018.09.037
Nouri M, Homaee M (2021a) Contribution of soil moisture variations to high temperatures over different climatic regimes. Soil Tillage Res 213:105115. https://doi.org/10.1016/j.still.2021.105115
Nouri M, Homaee M (2021b) Spatiotemporal changes of snow metrics in mountainous data-scarce areas using reanalyses. J Hydrol. https://doi.org/10.1016/j.jhydrol.2021.126858
Nouri M, Homaee M (2022) Reference crop evapotranspiration for data-sparse regions using reanalysis products. Agric Water Manage 262. https://doi.org/10.1016/j.agwat.2021.107319
Nouri M, Homaee M, Bannayan M (2017) Quantitative trend, sensitivity and contribution analyses of reference evapotranspiration in some arid environments under climate change. Water Resour Manage 31:2207–2224. https://doi.org/10.1007/s11269-017-1638-1
Paredes P, Martins DS, Pereira LS, Cadima J, Pires C (2018) Accuracy of daily estimation of grass reference evapotranspiration using ERA-Interim reanalysis products with assessment of alternative bias correction schemes. Agric Water Manage 210:340–353. https://doi.org/10.1016/j.agwat.2018.08.003
Paredes P, Pereira LS (2019) Computing FAO56 reference grass evapotranspiration PM-ETo from temperature with focus on solar radiation. Agric Water Manage 215:86–102. https://doi.org/10.1016/j.agwat.2018.12.014
Parker WS (2016) Reanalyses and observations: what’s the difference? Bull Am Meteorol Soc 97:1565–1572. https://doi.org/10.1175/bams-d-14-00226.1
Pelosi A, Chirico GB (2021) Regional assessment of daily reference evapotranspiration: Can ground observations be replaced by blending ERA5-Land meteorological reanalysis and CM-SAF satellite-based radiation data? Agric Water Manage 258. https://doi.org/10.1016/j.agwat.2021.107169
Pelosi A, Terribile F, D’Urso G, Chirico G (2020) Comparison of ERA5-Land and UERRA MESCAN-SURFEX reanalysis data with spatially interpolated weather observations for the regional assessment of reference evapotranspiration. Water 12. https://doi.org/10.3390/w12061669
Pereira LS, Allen RG, Smith M, Raes D (2015) Crop evapotranspiration estimation with FAO56: Past and future. Agric Water Manage 147:4–20. https://doi.org/10.1016/j.agwat.2014.07.031
Priestley CHB, Taylor RJ (1972) On the assessment of surface heat flux and evaporation using large-scale parameters. Mon Wea Rev 100:81–92. https://doi.org/10.1175/1520-0493(1972)100%3c0081:Otaosh%3e2.3.Co;2
Qiu R, Li L, Liu C, Wang Z, Zhang B, Liu Z (2022) Evapotranspiration estimation using a modified crop coefficient model in a rotated rice-winter wheat system. Agric Water Manage 264. https://doi.org/10.1016/j.agwat.2022.107501
Ramirez Camargo L, Schmidt J (2020) Simulation of multi-annual time series of solar photovoltaic power: Is the ERA5-land reanalysis the next big step? Sustainable Energy Technologies and Assessments 42. https://doi.org/10.1016/j.seta.2020.100829
Ravazzani G, Corbari C, Morella S, Gianoli P, Mancini M (2012) Modified Hargreaves-Samani equation for the assessment of reference evapotranspiration in Alpine river basins. J Irrig Drain Eng 138:592–599. https://doi.org/10.1061/(ASCE)IR.1943-4774.0000453
Raziei T, Parehkar A (2021) Performance evaluation of NCEP/NCAR reanalysis blended with observation-based datasets for estimating reference evapotranspiration across Iran. Theor Appl Climatol 144:885–903. https://doi.org/10.1007/s00704-021-03578-0
Raziei T, Pereira LS (2013) Estimation of ETo with Hargreaves-Samani and FAO-PM temperature methods for a wide range of climates in Iran. Agric Water Manage 121:1–18. https://doi.org/10.1016/j.agwat.2012.12.019
Ricard S, Anctil F (2019) Forcing the Penman-Montheith formulation with humidity, radiation, and wind speed taken from reanalyses, for hydrologic modeling. Water 11. https://doi.org/10.3390/w11061214
Ritchie JT (1972) Model for predicting evaporation from a row crop with incomplete cover. Water Resour Res 8:1204–1213. https://doi.org/10.1029/WR008i005p01204
Rousseeuw PJ, Hubert M (2011) Robust statistics for outlier detection. Wires Data Min Knowl Discovery 1:73–79. https://doi.org/10.1002/widm.2
Samani Z (2000) Estimating solar radiation and evapotranspiration using minimum climatological data. J Irrig Drain Eng 126:265–267. https://doi.org/10.1061/(ASCE)0733-9437(2000)126:4(265)
Sau F, Boote KJ, Bostick WM, Jones JW, Mínguez MI (2004) Testing and improving evapotranspiration and soil water balance of the DSSAT crop models. Agron J 96:1243–1257. https://doi.org/10.2134/agronj2004.1243
Sposito G (2017) Understanding the Budyko Equation. Water 9. https://doi.org/10.3390/w9040236
Stefanidis K, Varlas G, Vourka A, Papadopoulos A, Dimitriou E (2021) Delineating the relative contribution of climate related variables to chlorophyll-a and phytoplankton biomass in lakes using the ERA5-Land climate reanalysis data. Water Res 196:117053. https://doi.org/10.1016/j.watres.2021.117053
Tabari H, Talaee PH (2011) Local calibration of the Hargreaves and Priestley-Taylor equations for estimating reference evapotranspiration in arid and cold climates of iran based on the Penman-Monteith model. J Hydrol Eng 16:837–845. https://doi.org/10.1061/(asce)he.1943-5584.0000366
Thornthwaite CW (1948) An approach toward a rational classification of climate. Geogr Rev 38:55–94. https://doi.org/10.2307/210739
Todorovic M, Karic B, Pereira LS (2013) Reference evapotranspiration estimate with limited weather data across a range of Mediterranean climates. J Hydrol 481:166–176. https://doi.org/10.1016/j.jhydrol.2012.12.034
Tomas-Burguera M, Beguería S, Vicente-Serrano S, Maneta M (2018) Optimal interpolation scheme to generate reference crop evapotranspiration. J Hydrol 560:202–219. https://doi.org/10.1016/j.jhydrol.2018.03.025
Tomas-Burguera M, Vicente-Serrano SM, Grimalt M, Beguería S (2017) Accuracy of reference evapotranspiration (ET o ) estimates under data scarcity scenarios in the Iberian Peninsula. Agric Water Manage 182:103–116. https://doi.org/10.1016/j.agwat.2016.12.013
Trajkovic S, Gocic M (2021) Evaluation of three wind speed approaches in temperature-based ET0 equations: a case study in Serbia. Arabian Journal of Geosciences 14. https://doi.org/10.1007/s12517-020-06331-5
Trajkovic S, Kolakovic S (2009) Estimating reference evapotranspiration using limited weather data. J Irrig Drain Eng 135:443–449. https://doi.org/10.1061/(ASCE)IR.1943-4774.0000094
Turc L (1961) Estimation of irrigation water requirements, potential evapotranspiration: a simple climatic formula evolved up to date. Ann Agron 12:13–49
UNEP (1997) World atlas of desertification. In: Middleton N and Thomas D (eds) Arnold, Hodder Headline group, 2nd edn. UK, London, p. 182
Wright JL, Jensen ME (1972) Peak water requirements of crops in southern Idaho. J Irrig Drain Div 98:193–201. https://doi.org/10.1061/JRCEA4.0013020
Wu Z, Feng H, He H, Zhou J, Zhang Y (2021) Evaluation of soil moisture climatology and anomaly components derived From ERA5-Land and GLDAS-2.1 in China. Water Resour Manage 35:629–643. https://doi.org/10.1007/s11269-020-02743-w
Acknowledgements
We would like to thank three anonymous reviewers for their constructive and helpful comments. The first author is also deeply indebted to Iran Meteorological Organization (IRIMO) and its experts for providing required data and invaluable advice.
Funding
This work was financed by grants from the Iran Soil and Water Research Institute as the project number 1400/11530/243.
Author information
Authors and Affiliations
Contributions
Milad Nouri conceptualized the methodology framework, validated the results, and was a major contributor in writing the manuscript. Niaz Ali Ebrahimipak provided the required resources, and edited and proofread the main text. Seyedeh Narges Hosseini analyzed and visualized the data, and contributed in writing the manuscript.
Corresponding author
Ethics declarations
Ethics approval/declarations
All authors provided ethical approval to submit the manuscript.
Consent to participate
All authors consent to participate of the present study.
Consent for publication
All authors consent to the publication of the article in Theoretical And Applied Climatology.
Competing interests
The authors declare no competing interests.
Additional information
Publisher's note
Springer Nature remains neutral with regard to jurisdictional claims in published maps and institutional affiliations.
Supplementary Information
Below is the link to the electronic supplementary material.
Rights and permissions
Springer Nature or its licensor holds exclusive rights to this article under a publishing agreement with the author(s) or other rightsholder(s); author self-archiving of the accepted manuscript version of this article is solely governed by the terms of such publishing agreement and applicable law.
About this article
Cite this article
Nouri, M., Ebrahimipak, N.A. & Hosseini, S.N. Estimating reference evapotranspiration for water-limited windy areas under data scarcity. Theor Appl Climatol 150, 593–611 (2022). https://doi.org/10.1007/s00704-022-04182-6
Received:
Accepted:
Published:
Issue Date:
DOI: https://doi.org/10.1007/s00704-022-04182-6