Abstract
Rapid urbanization increases urban air temperature, considerably affecting health, comfort, and the quality of life in urban spaces. The accurate assessment of outdoor thermal comfort is crucial for urban health. In the present study, a high-resolution mesoscale model coupled with a layer Urban Canopy Model (WRF-UCM) is implemented over the city of Hyderabad (17.3850° N, 78.4867° E) to simulate urban meteorological conditions during the summer and winter period of 2009 and 2019. The universal thermal climate index (UTCI) has been estimated using the model-derived atmospheric variables and a human biometeorology parameter to assess the linkages between the outdoor environment and thermal comfort. Results revealed that during summer, the city experiences nearly 50 h of very strong thermal stress, whereas about 120 h of slight cold stress are experienced during winter. The urban area in Hyderabad expanded from 5 to 15% during the study period, leading to a 2.5℃ (2.8 ℃) increase in land surface temperature, and a 1.2 (1.9 ℃) rise in air temperature at 2 m height and 1.5 (2.5 ℃) UTCI during summer (winter) time. The analysis reveals that the maximum UTCI values were noticed over built-up areas compared to other land classes during daytime and nighttime. The results derived from the present study have shown that the performance of WRF-UCM-derived UTCI reasonably portrayed the significant impact of urbanization on thermal comfort over the city and provided useful insights with regard to urban comfort and welfare.
Similar content being viewed by others
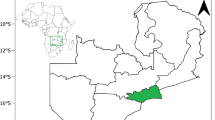
Avoid common mistakes on your manuscript.
Introduction
According to World Bank data, India has witnessed enormous urban growth in the past decade (https://data.worldbank.org/indicator/SP.URB.TOTL.IN.ZS?%20locations=IN). This data shows almost 35% urban expansion till 2017 and more than 45% urban growth by 2030. This drastic rise in urban expansion and associated land use and land cover (LULC) changes may lead to a rise in land surface temperature (LST) and urban heat island (UHI) over urban regions. Some early studies, such as (Kadaverugu 2023; Sultana and Satyanarayana 2023) have reported urban impacts of (UHI) and surface energy exchanges and analyzed basic meteorological variables over urban regions. These UHI zones will significantly impact outdoor thermal comfort (Ren et al. 2023) and the elevated temperatures in urban set-up, resulting in significant health issues (Chen and Ng 2012; Jiang et al. 2019). Hence, it is essential to understand the variation in outdoor thermal comfort over cities. Several indices are developed over the globe, such as Heat Index (HI) (Rothfusz 1990), wind chill index (WCT) (Siple et al. 1945), wet bulb globe temperature (WBGT) (Yaglou and Health DM-AI 1957), physiological equivalent temperature (PET) (Höppe 1999) Universal Thermal Climate Index (UTCI) (Jendritzky 2008), standard effective temperature (SET) (Nishi and Gagge 1977) and Physiological subjective temperature and physiological strain (PST, Psh) based on man-environment heat exchange (MENEX) (Błażejczyk 1994) model first published in 1994 is used to assess thermal comfort conditions. According to (Blazejczyk et al. 2012; Zare et al. 2018), several human heat budget models (PET, SET, PST, PhS) showed a strong correlation with UTCI. Indices derived from simpler formulas (HI, WBGT, WCT) had a weaker correlation with UTCI, where as HI, and WCT do not include radiation options in their formulas. However, non-radiation equation such as WBGT is more commonly used in bioclimatic research than their radiation counterparts. The analysis of synoptic and microclimatic data suggests that specific indices accurately represent bioclimatic conditions only under certain circumstances. In contrast, UTCI is a versatile index that effectively represents a variety of climates, weather conditions, and locations.
Thus, most of the studies include physiological responses by utilizing different biophysical parameters to address outdoor thermal comfort. In general, energy budget models (Farajzadeh et al. 2015) show a strong upper hand by taking precise human physiological responses in the estimation of thermal comfort.
Recently few studies (Park et al. 2014; Elraouf et al. 2022; Kotharkar and Dongarsane 2024), reported changes in UTCI due to urban morphology by considering ENVI-met software. In these studies, the MRT will be estimated in urban set-up by longwave and shortwave refections due to urban canopy by simulations. Study by (Kotharkar and Dongarsane 2024) showed differences in thermal comfort over different LCZs (Local Climate zones) and stated that sparsely built areas experience extreme thermal stress during the daytime and moderate stress during the nighttime.
The aforementioned studies utilized the local scale models (ENVI-met) but in order to study city-scale outdoor thermal comfort, some studies (Hamed et al. 2023; Naskar et al. 2024; Zeng et al. 2020) utilized the ERA5-Heat and ERA5-interim data is used. Those studies UTCI variations over cities and also in the study (Hamed et al. 2023) decadal change is reported and its rise about 0.1 to 0.6 ℃.
Along with reanalysis and modelled UTCI, some of the prominent and recent research over India on outdoor thermal comfort includes (Das and Das 2020; Kumar and Sharma 2022a, b; Sen and Nag 2019), but all these models lack in synoptic scale modelling over entire cities.
Though the mentioned research not exclusively reported LULC (Urban expansion) impacts on outdoor thermal comfort, few studies (Kusaka et al. 2012; Dutta et al. 2021; Zhao et al. 2021), mainly focused on changes in LULC reported there is an increment in the air temperature about 0.8 to 1.2 ℃ in a decade and due to the increase of impervious land surface and LULC change to the summer UHI intensity is increased about 0.76 ℃.
Therefore, the present study mainly focuses on employing energy budget models based on the Universal Thermal Climate Index (UTCI) to evaluate outdoor thermal comfort, incorporating the physiological response of individuals with weather research and forecast model (WRF). Recent advancements in scientific methods allow us to estimate energy budget models by mesoscale simulations like WRF-UCM (Di Napoli et al. 2021) Thus, an attempt is made to estimate the outdoor thermal comfort using an energy budget model such as UTCI by WRF-UCM with high spatial resolution. For the estimation of UTCI, the information of major meteorological parameters T2m (°C) (air temperature at 2 m), RH (%) (relative humidity), and WS (ms− 1) (wind speed) along with critical physical quantity MRT (mean radiant temperature) is required. The MRT (°C) is a vital measurement that reflects how people perceive radiation. The inclusion of UTCI in the study of outdoor thermal comfort has a great advantage because UTCI is independent of both spatial and temporal scales. To the best of the current literature, the proper spatial estimation of UTCI over different LULC classes, especially with mesoscale models in India, has not been reported extensively. By considering all the above aspects, this study attempts to estimate UTCI using WRF-UCM simulated with a high-resolution spatial resolution over the metropolitan city of Hyderabad, India.
Materials and methods
Study area
Hyderabad (17.38° N, 78.48° E) serves as the capital of the state of Telangana, India. It is the fourth most populous city in India, with approximately 6.8 million inhabitants, an area of 1136.2 km2 (Sultana and Satyanarayana 2018) and is characterized by the presence of multiple artificial lakes, such as Hussain Sagar (17.4239° N, 78.4738° E) and Osman Sagar (17.3763° N, 78.2989° E), along with various water tanks and ponds. Hyderabad is currently experiencing rapid urban development. The city’s climate is predominantly tropical with wet-dry conditions, occasionally leaning towards slightly semi-arid conditions (Norman 1995). In the summer months (March to June), the maximum temperature often surpasses 40 °C, while the winter months (November to February) the observed minimum temperatures ranging from 15 to 20 °C (source: https://www.worldweatheronline.com/hyderabad-weather-averages/andhra-pradesh/in.aspx). From Fig. 1b and c, a substantial urban region increment (5 to 15%) is observed. Most of the cultivated/vegetation turned to built-up, and the from the rest of the LULC which contains cultivated (∼ 70%)/deep vegetation (14%) has been converted to are land (5 to 13%). Most of the urbanization took place in the southern side of Hyderabad. Though most vegetative land is presented in Hyderabad, our regions are mostly cultivated (about 70%), which means having lesser NDVI, no substantial forest, or deep vegetation is found.
Data
In this study, the performance of the WRF and WRF-UCM models in simulating urban meteorological parameters for the Hyderabad metropolitan city. The model initial conditions are taken from the National Centres for Environmental Prediction (NCEP) FNL (Final) Operational Global Analysis, with a resolution of 0.25° × 0.25° over Hyderabad. In the present study the model derived air temperature, relative humidity, and wind speed, are used for estimating the Universal Thermal Climate Index (UTCI). The representative dates of summer 2009 and 2019 are 29-03-2009 to 05-04-2009 and 10-04-2019 to 18-04-2019, similarly for winter 27-01-2010 to 03-02-2010 and 29-01-2020 to 05-02-2020 are chosen for the study which contain a total of 32 days. The above dates are chosen very meticulously so that there are no thunderstorms/heavy rains, or highly hazy conditions. In summer (winter) 2009, 133 (137) hours are fair, and the remaining hours are partly cloudy. Whereas in 2019 summer (winter), 82 (73) hours are fair 79 (69) hours are partly cloudy, about 30 h are foggy, only 1 h is rainy, and the remaining hours are a bit hazy and cloudy mixed conditions. So, these fair and partly cloudy hours won’t much impact the analysis to compare between 2009 and 2019. The detailed data is given in supplementary for further verification.
Model description
In the present study, the Weather Research Forecasting model (WRF) (version 4.0 UG) with and without a single-layer urban canopy model developed by the National Center for Atmospheric Research (NCAR) was utilized to assess the performance evaluation of simulating the urban boundary layer parameters. Four nested domains are considered for the Hyderabad city region in the present study with a spatial resolution of 12 km for the parent domain (D01), 4 km for the first nested domain (D02), and 1.34 km for the second nested domain (D03) and 0.45 km the innermost nested domain (D04) containing the city region, as shown in Fig. 1a. The inner domain is centered at 17.3850° N, 78.4867° E, and consists of 240 × 240 grids (which means 240 × 240 pixels and each pixel contains area 0.45 km2). Figure 1b and c depict the LULC classification for 2009 and 2019 in the innermost domain D04 taken from ISRO-derived IRS-P6 AWiFS gridded data. Figure 1d represents the administrative boundary of the city and the mandals (the zones of the city) for analysis purposes. The model schemes and parametrizations are considered based on earlier studies such as (Bhati and Mohan 2018; Sultana and Satyanarayana 2023). The model details are given in Table 1.
UTCI model
UTCI is defined as the air temperature (Ta) obtained by balancing the energy of actual meteorological variables with reference values. The offset deviation of UTCI from air temperature depends on several actual meteorological variables such as of air temperature, MRT, WS, and RH, expressed as water vapor pressure (Vp) orRH. (Bröde et al. 2012a)
In the current study, the UTCI-based thermal comfort category is utilized. Accordingly, if the magnitude of UTCI is > 46 is considered as extreme heat stress; 38 to 46 very strong heat stress; 32 to 38 strong heat stress; 26 to 32 moderate heat stress; 9 to 26 no thermal stress; and 0 to 9 slight cold stress.
UTCI is an energy budget model that states the heat exchanges between the surrounding environment and the human body, including physiology and clothing insulation (Havenith et al. 2012a). UCTI estimation is based on the UTCI-fiala model (Fiala et al. 2012; Havenith et al. 2012b) that relies on a dynamic multi-node thermoregulation model of the human body applies for any spatial and temporal scales.
So, UCTI is extensively used over the globe for current and future scales (Bröde et al. 2012b; Coccolo et al. 2016; Błażejczyk et al. 2018). Despite the potential uses of UTCI assessment for a better understanding of thermal comfort in bio-meteorological aspects, it involves complex calculations. The parameters involved in the estimation of UTCI are T2m, RH, WS, and MRT. In the aforementioned meteorological parameters, the calculation of MRT is not straightforward. The estimation of MRT is always problematic (Kántor and Unger 2011). Recent studies, such as (Di Napoli et al. 2020), successfully demonstrated the calculation of MRT from NWP models.
The MRT is a critical physical quantity representing how the human body experiences thermal load when attempting to maintain thermal equilibrium with the surroundings.
MRT from numerical weather prediction models can be computed in the step-by-step algorithm. At first, the calculation of the surface projection factor \( {f}_{p}\).
Here γ is the complementary angle to the solar zenith angle (γ in degrees). In the second step, the thermal and solar radiation will be calculated. These two radiations have two components again. There are five components, namely, the downwelling thermal component from the atmosphere (\( {L}_{surf}^{dn}\)) and the upwelling thermal component from the ground (\( {L}_{surf}^{up}\)) and direct components from the sun (\( {I}^{*}\)) and a diffuse component of the isotropic diffuse solar radiation flux \( {S}_{surf}^{dn,diff}\) and the surface-reflected solar radiation flux \( {S}_{surf}^{up}\). This computation’s details are described by (Di Napoli et al. 2020).
The MRTequation proposed by (Staiger and Matzarakis 2010) has been rewritten by (Di Napoli et al. 2020) as follows.
Where \( {I}^{*}\) is calculated from the diffuse component of the isotropic diffuse solar radiation flux and cosine of solar zenith angle
The MRT derived from NWP models was validated throughout the globe with proper sun’s position across the time periods. The sun’s position and its effect were included by the solar zenith angle \( ({{\rm{\theta }}}_{0}) \)in Eqs. 2 and 4. To calibrate the performance of the above equation, they validated it with radiation monitoring stations and showed a coefficient of determination greater than 0.88; the average bias was equal to 0.42 °C.
In (Di Napoli et al. 2020; Napoli et al. 2021) study, ERA5-HEAT was compared to observations from 177 meteorological stations in the surface synoptic observations (SYNOP) network. These SYNOP stations were carefully selected outside forested areas and with elevations similar to those represented in the model (with a difference of less than 5 m). The observations included 2-meter air temperature, 2-meter dew point temperature, wind speed at 10 m, and total cloud cover for the year 2018. These data were then input into the RayMan Pro software (version 3.0 Beta) to calculate the observed MRT and the UTCI at each SYNOP station. To assess the agreement between observed data (oi) and reanalysis data (mi) extracted from the grid cells where the stations are located, the RMSE is used: The majority of stations exhibited an R-squared value above 0.6, with the deviation RMSE of MRT and UTCI reanalysis data from observed values are observed. On average, MRT deviated by 8.6 ± 2.5 °C, while UTCI deviated by 5.2 ± 2.5 °C.
Results and discussion
Evaluation of WRF and WRF-UCM simulated surface meteorological parameters
The diurnal as well as day-to-day variation of the air temperature simulated by WRF and WRF-UCM, and ERA-5 data at the city location, RGI airport (17.2370° N, 78.4300° E), are depicted along with the available observations during the summertime of 2009 and 2019 in Fig. 2a and b, respectively. The model simulations reasonably captured the diurnal as well as diurnal patterns as seen in the observations. It is noticed that the WRF- UCM and ERA-5 values reasonably match the observations, whereas WRF values are overestimated, especially during local noon and night hours. (Sultana and Satyanarayana 2023), have reported that WRF overestimates the air temperature compared with WRF-UCM, as seen in our results. It is seen that the peak temperatures during the study period have been in the range of 36 to 38 ℃ in 2009 and have been raised to 38 to 40 ℃ in 2019. In terms of statistical analysis. Whereas in winters (Fig. 2c and d), the peak temperatures over the 8 days slightly increased from 27 to 29 ℃ in 2009, whereas in 2019, the temperature is observed to be 30 to 32 ℃.
The RMSE of WRF and WRF-UCM is 4 and 3.67 ℃ in the summer-2009 and 3.76 and 3.29 ℃ in the summer-2019, respectively, showing the improved simulations by WRF-UCM. Similarly, the RMSE of WRF and WRF-UCM is 4 and 3.67 ℃ in winter-2009, and 3.76 and 3.29 ℃ in winter-2019 is reported.
The simulations of relative humidity in the summertime of 2009 and 2019 are validated with the observations at RGI airport and presented in Fig. 2e and f. The pattern of modeled diurnal variations reasonably matches well with the observations. The WRF model and ERA-5 clearly underestimate peak time humidity levels where, whereas WRF-UCM has shown improvement compared to observations. The peak humidity over the 8 days is noticed to be 50 to 60% in 2009, whereas in 2019, the humidity is observed to be 80%.
The statistical analysis reveals the RMSE of WRF and WRF-UCM is 16.70% and 15.74% in 2009 and 15.21% and 13.87% in 2019. Similar patterns are noticed in winter times in the relative humidity, as shown in Fig. 2g and h. It is noticed that the RMSE of WRF and WRF-UCM is 16.70% and 11.80% in 2009 and 20.08% and 18.67% in 2019, respectively.
The WRF and WRF-UCM simulated temperatures in 2009 and 2019 are validated with observations along with ERA-5 data at a built-up and city centre location, Begumpet airport (17.4496° N, 78.4712° E), and ERA-5 data are depicted in Fig. 3a and b. The diurnal patterns match the observations well. The WRF showed a similar overestimation in peak time temperatures compared to WRF-UCM. The range of temperatures is slightly higher at 1 to 2 ℃ at the Begumpet to RGI airport location. In terms of statistical analysis, the RMSE of WRF and WRF-UCM is 1.67 and 1.60 ℃ in 2009 and 1.83 and 1.78 ℃ in 2019. Similarly, in winter (Fig. 3a and b), the RMSE of WRF and WRF-UCM is 1.49 and 1.40 ℃ in 2009 and 1.54 and 1.21 ℃ in 2019, respectively. The simulated relative humidity in the summer seasons of 2009 and 2019 are validated with the observations at Begumpet airport (Fig. 3e and f). The model simulations capture the diurnal variations of relative humidity well, as seen in the observations. The WRF model clearly underestimates peak time humidity levels, similar to those of rural stations. The peak humidity levels range from 80 to 100% in both winters. In terms of statistical analysis, the RMSE of WRF and WRF-UCM is 10.67% and 10.51% in 2009 and 13.01% and 9.07% in 2019. From Fig. 3g and h, a similar behavior of WRF (underestimation at noon and late night times) is observed. In terms of statistical analysis, the RMSE of WRF and WRF-UCM is 9.26% and 9.01% in 2009 and 11.38% and 9.97% in 2019.
From Fig. 4, the mean bias errors between MODIS and WRF (WRF-UCM) are 3.29 (2.52), 3.11 (2.32), 3.00 (2.14), 4.72 (3.18) ℃ for summer 2009, winter 2009, summer 2019 and winter 2019 respectively. From which WRF-UCM shows almost 1–2℃ upper handover WRF, and for further verification, bias plots are given in supplementary.
Spatial variation of UTCI during 2009 and 2019 during summer and winter
Daytime
In this section, four block regions, B1, B2, B3, and B4, over Hyderabad city are considered to represent four different land classifications to analyze the variation in the outdoor thermal capacity. Block B1 is Hussain Sagar (17.42 N, 78.47E) region, a large water body, B2 is Kasu Bramhanada Reddy Park (B2, 17.42 N, 78.41E), B3 (urban region) and B4 (dominated by barren land region) over the city.
Figure 5a and b represent the spatial variation of UTCI during the daytime (mean of 00UTC to 12 UTC) in the summer of 2009 and 2019, respectively.
The cooling effect is noticed near the water bodies over blocks B1 during the summer of 2009 and 20,019, as shown in Fig. 5a and b. The ability of water bodies to cool the nearby environment is reasonably good in terms of urban health. A similar effect of such urban cool patches is reported in the literature (Sultana and Satyanarayana 2019, 2022; Prasad and Satyanarayana 2023). The B2 region UTCI values showed an increase from 2009 to 2019. The B3 region is the most urban region, and the B4 region is a combined urban and barren (Prasad and Satyanarayana 2023). These two regions exhibited moderate/strong comfort in 2009 to strong/very strong heat stress in 2019 summer.
Figure 5c and d represent the spatial variation of UTCI during the daytime (00 UTC to 12 UTC) in winter 2009 and 2019, respectively. Interestingly B1 region is exhibiting lesser cooling in contrast to summertime. The B2 region is the northwestern part of the city, exhibiting lesser UTCI in both 2009 and 2019. The B3 and B4 regions show an increase in the magnitude of UTCI from 2009 to 2019 but still fall under moderate thermal stress to moderate stress conditions. In general, it is noticed that the summertime UTCI in Hyderabad falls under the very strong thermal stress category, whereas in winter, the city falls under no thermal stress to moderate stress conditions. The range of UTCI is about 35 to > 39 ℃, in summer, which is a highly intolerable limit. Similar high thermal stress is reported by (Kumar and Sharma 2022c; Shukla et al. 2022). The analysis reveals that the different land surfaces over the city exhibited varying thermal comfort.
Night time
Figure 5e and f represent the spatial variation of UTCI during the nighttime (13 UTC to 23 UTC) during the summertime of 2009 and 2019. The summer nighttime UTCI in Hyderabad falls under the moderate to no thermal stress category. The range of UTCI is about 24 to 29 ℃ (in 2009) and > 30 ℃ (in 2019), which is moderately comfortable. In contrast to the daytime cooling effect of waterbodies B1 region, there is a slightly elevated UTCI near water bodies during the nighttime of winter 2009 and 2019. Since the heat capacity of water (Hamoodi et al. 2019) is high compared to other land covers, slight warming conditions have prevailed at night. This unique phenomenon portrays the importance of water bodies within the city by making it cool during the day and slightly warmer at night, which provides highly comfortable conditions. From Fig. 5g and h, the range of UTCI on winter nights is 16 to 22℃, which makes highly comfortable conditions. At night times, regions B2 and B3 became moderate stress from no thermal stress from 2009 to 2019 in the summer. The B4 regionally exceeded > 26 ℃ (moderate heat stress) from 2009 to 2019. In winter, all study regions come under no thermal stress.
Mandal-wise variation of UTCI during summer and winter during 2009 and 2019
Hyderabad city has 16 Mandal regions, as shown in Fig. 1, and all these zones fall under varying land use classes. In this section, the change in the outdoor thermal comfort over these mandals from 2009 to 2019 during summer (Fig. 6a) as well as winter (Fig. 6b). Figure 6a depicts the category of UTCI in a 8 days for each hour of the 2009 and 2019 summer time. Significant change is noticed in UTCI in each of the mandals between 2009 and 2019. Most of the mandals exhibited about 50 h of very strong stress during summer days. Interestingly, some mandals have exhibited extreme heat stress change during the study period. Amberpet (17.39° N, 78.516° E), Charminar (17.36° N, 78.47° E), and Nampally (17.38° N, 78.46° E) witnessed the 10 h of extreme heat stress in 2019, but less than 5 h extreme heat stress was noticed in 2009. The remaining hours are distributed between moderate thermal stress and slight cold stress. Summertime results reveal a substantial decrease in the number of hours of the slight cold stress category from 2009 to 2019. In winters, from Fig. 6b, it is observed that Most of the mandals exhibited about 30 h of moderate stress from 2009 to 2019. Very few mandals showed the change from moderate to strong heat stress from 2009 to 2019. In winter, particularly at nighttime, there is slight cold stress in Hyderabad for about > 120 h.
The mean UTCI and T2m variation on different LULC classes
The dotted lines in Fig. 7a and b represent the UTCI value, and the solid line corresponds to air temperature at 2 m. In both summer and winter, the variations of UTCI and T2m are similar; most water bodies are cooler at noon times and slightly higher in the early mornings.
Vegetation shows the lowest temperatures, followed by bare land and built-up. The built-up temperatures are very high at noon, reaching about 45℃ in summers and 35℃ in winters. Similar patterns are observed in (Yeo et al. 2021), where the forest region is much colder than all other LULC. Only water bodies show little contrast signature, which is lowest in summer and second lowest in winter.
The observation from Fig. 7 states that there is a high elevated UTCI at noon and a dip in the early morning compared to air temperature. The probable cause for this UTCI variation compared to T2m is the consideration of mean radiant temperature. To investigate this pattern, UTCI and corresponding MRT are plotted in Fig. 7c and d.
From Fig. 7c and d, the MRT was very high at noon (red color line plot), while UTCI (contour plot) was at its maximum and almost reached near zero in the early morning. MRT involves two major components: long-wave and short-wave radiation flux. The short-wave flux is zero when the sun is absent, and the long-wave starts decreasing from sunset and reaches a minimal point by early morning. Thus, nighttime MRT is minimal, whereas at noon, both contribute to very high numbers and a high UTCI. From this investigation, the major driver of UTCI at noon is MRT, whereas at night, T2m is significant.
Impact of urbanization on thermal comfort and other meteorological variables
Figure 8a and b represent urban fractions in 2009 and 2019, and Fig. 8C represents urban growth (10%) from 2009 to 2019. So, as urban areas in Fig. 8a and b are taken as reference and calculated mean urban temperatures and Tsk and UTCI over time.
In order to distinguish the change in heat stress due to urbanization, but not limited to local climate change, we have conducted two numerical experiments with WRF-UCM. In the first simulation, we have not considered any change of LULC during the study period and hence considered the same LULC of 2009 (as shown in Fig. 1b) for the 2019 simulation. The second simulation by considering the LULC change as depicted in Fig. 1c.
The first simulation, where no change in LULC revealed an increase of 1.8 ℃ in skin temperature, 0.3 ℃ in UTCI and 0.01 ℃ in T2m in summer (Fig. 8d). Similarly, in the winter season, it is seen that an increase of 2.0 ℃ in skin temperature, 1.7 ℃ in UTCI and 1.5 ℃ in T2m (Fig. 8e). These changes are mainly attributable to local climate change over the study region without any increase in urban fraction.
The second simulation, where the change in LULC and increase in urban fraction are considered, revealed an increase of 2.5 ℃ in skin temperature, 1.4 ℃ in UTCI and 1.2 ℃ in T2m in summer seasons (Fig. 8d). Similarly, in winter season, it is noticed that an increase of 2.8 ℃ in skin temperature; 2.5 ℃ in UTCI and 1.9 ℃ in T2m (Fig. 8e). These changes are mainly attributable to an increase in urbanization.
From this analysis, higher temperatures are noted in urban expansion than in conditions without urban expansion. Thus, urban expansion contributed 28% (29%) to skin temperature, 78% (32%) to UTCI, and 99% (21%) to air temperature, which is an increase due to the urban expansion factor in summer (winter), respectively.
The results clearly indicate that the increase in heat stress is mainly due to urbanization. The spatial variation plots related to these simulations are included in the supplementary of the revised manuscript.
Comparison of WRF-UCM-derived UTCI and ERA5-UTCI
Since the present study is considering the methodology from the ERA5 documentation (Di Napoli et al. 2021), we want to cross-compare the mean UTCI derived and ERA5-UTCI over the innermost domain. Figure 9, a, b, c, d represent the WRF-UCM and ERA5-UTCI over the summer and winter seasons of the study period.
From Fig. 9a and b, WRF-UCM-derived UTCI and ERA5-UTCI have a small bias observed in summer at noon times. Meanwhile, WRF-UCM-derived UTCI results in winter (Fig. 9c and d) match well with ERA5 data in noon times and small bias in evening times. Since the UTCI is sensitive to meteorological variables, each day bias is calculated at 32 days of the simulation period, estimated mean bias with confidence interval (CI95%), and standard deviation. From this statistical analysis, the confidence interval is calculated for the model for bias. The mean bias with [CI− 95%, CI+ 95%] about 4.5 ℃[4.5–0.5 ℃, 4.5 + 0.5 ℃] and standard deviation is about 1 ℃ and CI95%=±0.5 ℃.
The limitation of this model is that it cannot calculate precise MRT since we are going to a higher spatial resolution where the basic assumption of radiation fluxes may not be accurate. In this study (Di Napoli et al. 2020), MRT was calculated assuming that the angle factor is set to 0.5, the urban canopy plays a vital role, and reflection fluxes may change the angle factor. Though we have limitations, the bias from the above comparison is minimal since the bias range is below one class of thermal stress.
Conclusions
In the present study, outdoor thermal comfort has been derived by employing two mesoscale models, WRF and WRF-UCM with a high spatial resolution over the metropolitan city of Hyderabad. Results reveals that WRF-UCM, which considered the urban fraction performed better than WRF. The model estimated UTCI and atmosphereic variables are validatedwith ERA-5 data as well as with available meteorological observations. In order to estimate urban spatial statistics, the urban LST of the model was validated with MODIS satellite-derived LST. A significant increase in the urban fraction was noticed from 2009 to 2019.The urban regions were exposed to very high thermal stress and exhibited more than 50 h of extreme/very strong heat stress in summers and about 30 h of moderate stress in winters that could lead to most discomfort to pedestrians and workers.
Numerical experiments with and without considering of urban expansion during the study period has shown an increase of 78% (32%) of UTCI in summer (winter) is due to urbanization and 22% (68%) due to local climate change in summer (winter). Summers in Hyderabad are characterized by intense heat stress, while winters experience mild to no thermal stress. The present study revealed considerable changes in outdoor thermal comfort changing from strong heat stress and very strong heat stress conditions as seen in the mandal-wise analysis over Hyderabad majorly due to increase in urbanization along with local climate change. Therefore, local authorities should implement mitigation strategies. The analysis shows that vegetated zones provide the highest comfort, particularly at night, highlighting the significance of natural vegetation in cities and its cooling capacity.
Decadal variation of urban fraction, air temperature, skin temperature and UTCI during 2009 and 2019, (a) urban frac in 2009 (b) urban frac in 2019 (c) increment in urban frac (d) change in variables in 2009 and 2019 (without and with urban expansion) in summer (e) change in variables in 2009 and 2019 (without and with urban expansion) in winter
References
Bhati S, Mohan M (2018) WRF-urban canopy model evaluation for the assessment of heat island and thermal comfort over an urban airshed in India under varying land use/land cover conditions. Geosci Lett 5:1–19. https://doi.org/10.1186/S40562-018-0126-7/FIGURES/12
Blazejczyk K, Epstein Y, Jendritzky G, Staiger H, Tinz B (2012) Comparison of UTCI to selected thermal indices. Int J Biometeorol 56:515–535. https://doi.org/10.1007/S00484-011-0453-2/FIGURES/12
Błażejczyk A, Błażejczyk K, Baranowski J, Kuchcik M (2018) Heat stress mortality and desired adaptation responses of healthcare system in Poland. Int J Biometeorol 62:307–318. https://doi.org/10.1007/S00484-017-1423-0/TABLES/5
Błażejczyk K (1994) New climatological and physiological model of the human heat balance outdoor (MENEX) and its applications in bioclimatological studies in different scales. Zeszyty Instytutu Geogr i Przestrzennego Zagospodarowania PAN 27–58
Bröde P, Fiala D, Błażejczyk K, Holmér I, Jendritzky G, Kampmann B, Tinz B, Havenith G (2012a) Deriving the operational procedure for the Universal Thermal Climate Index (UTCI). Int J Biometeorol 56:481–494. https://doi.org/10.1007/S00484-011-0454-1/FIGURES/12
Bröde P, Krüger EL, Rossi FA, Fiala D (2012b) Predicting urban outdoor thermal comfort by the Universal Thermal Climate Index UTCI-a case study in Southern Brazil. Int J Biometeorol 56:471–480. https://doi.org/10.1007/S00484-011-0452-3/TABLES/3
Chen L, Ng E (2012) Outdoor thermal comfort and outdoor activities: a review of research in the past decade. Cities 29:118–125. https://doi.org/10.1016/J.CITIES.2011.08.006
Coccolo S, Kämpf J, Scartezzini JL, Pearlmutter D (2016) Outdoor human comfort and thermal stress: a comprehensive review on models and standards. Urban Clim 18:33–57. https://doi.org/10.1016/J.UCLIM.2016.08.004
Das M, Das A (2020) Exploring the pattern of outdoor thermal comfort (OTC) in a tropical planning region of eastern India during summer. Urban Clim 34:100708. https://doi.org/10.1016/J.UCLIM.2020.100708
Di Napoli C, Hogan RJ, Pappenberger F (2020) Mean radiant temperature from global-scale numerical weather prediction models. Int J Biometeorol 64:1233–1245. https://doi.org/10.1007/S00484-020-01900-5/FIGURES/6
Di Napoli C, Barnard C, Prudhomme C, Cloke HL, Pappenberger F (2021) ERA5-HEAT: a global gridded historical dataset of human thermal comfort indices from climate reanalysis. Geosci Data J 8:2–10. https://doi.org/10.1002/GDJ3.102
Dutta D, Gupta S, Dutta D, Gupta · S (2021) Rising trend of air pollution and its decadal consequences on meteorology and thermal comfort over gangetic West Bengal, India. 689–720. https://doi.org/10.1007/978-3-030-63422-3_32
Elraouf RA, ELMokadem A, Megahed N, Eleinen OA, Eltarabily S (2022) Evaluating urban outdoor thermal comfort: a validation of ENVI-met simulation through field measurement. J Build Perform Simul 15:268–286. https://doi.org/10.1080/19401493.2022.2046165
Farajzadeh H, Saligheh M, Alijani B, Matzarakis A (2015) Comparison of selected thermal indices in the northwest of Iran. Nat Environ Change 1:1–20
Fiala D, Havenith G, Bröde P, Kampmann B, Jendritzky G (2012) UTCI-Fiala multi-node model of human heat transfer and temperature regulation. Int J Biometeorol 56:429–441. https://doi.org/10.1007/S00484-011-0424-7/TABLES/4
Hamed MM, Kyaw AK, Nashwan MS, Shahid S (2023) Spatiotemporal changes in universal thermal climate index in the Middle East and North Africa. Atmos Res 295:107008. https://doi.org/10.1016/J.ATMOSRES.2023.107008
Hamoodi MN, Corner R, Dewan A (2019) Thermophysical behaviour of LULC surfaces and their effect on the urban thermal environment. J Spat Sci 64:111–130. https://doi.org/10.1080/14498596.2017.1386598
Havenith G, Fiala D, Błazejczyk K, Richards M, Bröde P, Holmér I, Rintamaki H, Benshabat Y, Jendritzky G (2012a) The UTCI-clothing model. Int J Biometeorol 56:461–470. https://doi.org/10.1007/S00484-011-0451-4
Havenith G, Fiala D, Błazejczyk K, Richards M, Bröde P, Holmér I, Rintamaki H, Benshabat Y, Jendritzky G (2012b) The UTCI-clothing model. Int J Biometeorol 56:461–470. https://doi.org/10.1007/S00484-011-0451-4/FIGURES/7
Höppe P (1999) The physiological equivalent temperature – a universal index for the biometeorological assessment of the thermal environment. Int J Biometeorol 1999 43:2. https://doi.org/10.1007/S004840050118
Janjic Z (2002) Nonsingular implementation of the Mellor-Yamada level 2.5 scheme in the NCEP Meso model. NCEP Office Note
Jendritzky G (2008) The universal thermal climate index UTCI–goal and state of COST Action 730. 18th international conference on biometeorology, Tokyo
Jiang Y, Luo Z, Wang Z, Lin B (2019) Review of thermal comfort infused with the latest big data and modeling progresses in public health. Build Environ 164:106336. https://doi.org/10.1016/J.BUILDENV.2019.106336
Jimy Dudhia (1989) Numerical Study of Convection observed during the winter monsoon experiment using a mesoscale two-dimensional model. J Atmos Sci 46:3077–3107
Kadaverugu R (2023) A comparison between WRF-simulated and observed surface meteorological variables across varying land cover and urbanization in south-central India. Earth Sci Inf 16:147–163. https://doi.org/10.1007/S12145-022-00927-Z/FIGURES/9
Kain JS (2004) The Kain–Fritsch Convective parameterization: an update. J Appl Meteorol Climatol 43:170–181. https://doi.org/10.1175/1520-0450(2004)043
Kántor N, Unger J (2011) The most problematic variable in the course of human-biometeorological comfort assessment - the mean radiant temperature. Cent Eur J Geosci 3:90–100. https://doi.org/10.2478/S13533-011-0010-X/MACHINEREADABLECITATION/RIS
Kotharkar R, Dongarsane P (2024) Investigating outdoor thermal comfort variations across local climate zones in Nagpur, India, using ENVI-met. Build Environ 249:111122. https://doi.org/10.1016/J.BUILDENV.2023.111122
Kumar P, Sharma A (2022a) Assessing outdoor thermal comfort conditions at an urban park during summer in the hot semi-arid region of India. Mater Today Proc 61:356–369. https://doi.org/10.1016/J.MATPR.2021.10.085
Kumar P, Sharma A (2022b) Assessing the monthly heat stress risk to society using thermal comfort indices in the hot semi-arid climate of India. Mater Today Proc 61:132–137. https://doi.org/10.1016/J.MATPR.2021.06.292
Kumar P, Sharma A (2022c) Assessing the outdoor thermal comfort conditions of exercising people in the semi-arid region of India. Sustain Cities Soc 76:103366. https://doi.org/10.1016/J.SCS.2021.103366
Kusaka H, Hara M, Takane Y (2012) Urban Climate Projection by the WRF Model at 3-km horizontal Grid Increment: Dynamical Downscaling and Predicting Heat stress in the 2070’s August for Tokyo, Osaka, and Nagoya Metropolises. J Meteorological Soc Japan Ser II 90B:47–63. https://doi.org/10.2151/JMSJ.2012-B04
Mlawer EJ, Taubman SJ, Brown PD, Iacono MJ, Clough SA (1997) Radiative transfer for inhomogeneous atmospheres: RRTM, a validated correlated-k model for the longwave. J Geophys Research: Atmos 102:16663–16682. https://doi.org/10.1029/97JD00237
Naskar PR, Mohapatra M, Singh GP, Das U (2024) Spatiotemporal variations of UTCI based discomfort over India. J Earth Syst Sci, 133(1), 47. https://doi.org/10.1007/s12040-024-02261-y
Nishi Y, Gagge AP (1977) Effective temperature scale useful for hypo- and hyperbaric environments. Aviat Space Environ Med 48:97–107. https://doi.org/10.1097/00006534-197801000-00129
Norman MJTPCJSPGE (1995) The ecology of tropical food crops. Cambridge University Press, pp 149–251
Park S, Tuller SE, Jo M (2014) Application of universal thermal climate index (UTCI) for microclimatic analysis in urban thermal environments. Landsc Urban Plan 125:146–155. https://doi.org/10.1016/J.LANDURBPLAN.2014.02.014
Pleim JE (2006) A simple, efficient solution of Flux–Profile relationships in the atmospheric surface layer. J Appl Meteorol Climatol 45:341–347. https://doi.org/10.1175/JAM2339.1
Prasad PSH, Satyanarayana ANV (2023) Assessment of outdoor thermal comfort using landsat 8 imageries with machine learning tools over a metropolitan city of India. Pure Appl Geophys 1–17. https://doi.org/10.1007/S00024-023-03328-5/FIGURES/9
Ren J, Shi K, Li Z, Kong X, Zhou HA, Ren J, Shi K, Li Z, Kong X, Zhou H (2023) A review on the impacts of urban heat islands on outdoor thermal comfort. Buildings 13:1368. https://doi.org/10.3390/BUILDINGS13061368
Rothfusz LP (1990) The heat index equation (or, more than you ever wanted to know about heat index). In: Tech. Attachment, SR/SSD 90– 23, NWS S. Reg. Headquarters, Forth Worth, TX, 1990
Sen J, Nag PK (2019) Effectiveness of human-thermal indices: Spatio–temporal trend of human warmth in tropical India. Urban Clim 27:351–371. https://doi.org/10.1016/J.UCLIM.2018.11.009
Shukla KK, Attada R, Kumar A, Kunchala RK, Sivareddy S (2022) Comprehensive analysis of thermal stress over northwest India: climatology, trends and extremes. Urban Clim 44:101188. https://doi.org/10.1016/J.UCLIM.2022.101188
Siple P, Society CP-P of the AP (1945) Undefined Measurements of dry atmospheric cooling in subfreezing temperatures. JSTORPA Siple, CF PasselProceedings of the American Philosophical Society, 1945•JSTOR
Staiger H, Matzarakis A (2010) Estimating down-and up-welling thermal radiation for use in mean radiant temperature
Sultana S, Satyanarayana ANV (2018) Urban heat island intensity during winter over metropolitan cities of India using remote-sensing techniques: impact of urbanization. Int J Remote Sens 39:6692–6730. https://doi.org/10.1080/01431161.2018.1466072
Sultana S, Satyanarayana ANV (2019) Impact of urbanisation on urban heat island intensity during summer and winter over Indian metropolitan cities. Environ Monit Assess 191:1–17. https://doi.org/10.1007/S10661-019-7692-9/FIGURES/9
Sultana S, Satyanarayana ANV (2022) Impact of urbanization on surface energy balance components over metropolitan cities of India during 2000–2018 winter seasons. Theor Appl Climatol 148:693–725. https://doi.org/10.1007/S00704-022-03937-5/TABLES/13
Sultana S, Satyanarayana ANV (2023) Impact of land use land cover on variation of urban heat island characteristics and surface energy fluxes using WRF and urban canopy model over metropolitan cities of India. Theor Appl Climatol 152:97–121. https://doi.org/10.1007/S00704-023-04362-Y/FIGURES/15
Tewari M, CF, WW, DJ, LMA MKE (2004) Implementation and verification of the unified Noah land-surface model in the WRF model [presentation. In 20th conference on weather analysis and forecasting/16th conference on numerical weather prediction American Meteorological Society: Seattle, WA, US
Yaglou C, Health DM-AI (1957) Undefined control of heat casualties at military training centers. cabdirect.orgCP Yaglou. D MinaedArch Indust Health, 1957•cabdirect.org
Yeo LB, Ling GHT, Tan ML, Leng PC (2021) Interrelationships between land use land cover (LULC) and human thermal comfort (HTC): a comparative analysis of different spatial settings. Sustainability 2021 13:382. https://doi.org/10.3390/SU13010382
Yuh-Lang Lin RDF and HDO (1983) Bulk parameterization of the snow field in a cloud model. J Appl Meteorol Climatol 22:1065–1092
Zare S, Hasheminejad N, Shirvan HE, Hemmatjo R, Sarebanzadeh K, Ahmadi S (2018) Comparing Universal Thermal Climate Index (UTCI) with selected thermal indices/environmental parameters during 12 months of the year. Weather Clim Extrem 19:49–57. https://doi.org/10.1016/J.WACE.2018.01.004
Zeng D, Wu J, Mu Y, Deng M, Wei Y, Sun W (2020) Spatial-temporal pattern changes of UTCI in the China-Pakistan economic corridor in recent 40 years. Atmos, 11(8), 858. https://doi.org/10.3390/atmos11080858
Zhao Y, Zhong L, Ma Y, Fu Y, Chen M, Ma W, Zhao C, Huang Z, Zhou K (2021) WRF/UCM simulations of the impacts of urban expansion and future climate change on atmospheric thermal environment in a Chinese megacity. Clim Change 169:1–17. https://doi.org/10.1007/S10584-021-03287-7/FIGURES/6
Acknowledgements
The first author of the manuscript, P.S. Hari Prasad, gratefully acknowledges the Indian Institute of Technology Kharagpur for conducting the research work, and the Govt. of India is providing a research fellowship.
Funding
No funding is available for this work.
Author information
Authors and Affiliations
Contributions
P.S. Hari Prasad and Dr. A.N.V. Satyanarayana contributed to the conception and design of the study. Material preparation, data collection, and analysis were performed by P.S.Hari Prasad and continuously guided by Dr. A.N.V. Satyanarayana. The first draft of the manuscript was written by P.S. Hari Prasad, and Dr. A.N.V. Satyanarayana made corrections and modifications. All authors read and approved the final manuscript.
Corresponding author
Ethics declarations
Conflict of interest
On behalf of all authors, the corresponding author states that there is no conflict of interest.
Competing interest
The authors declare that they have no known competing financial interests or personal relationships that could have appeared to influence the work reported in this paper.
Additional information
Publisher’s Note
Springer Nature remains neutral with regard to jurisdictional claims in published maps and institutional affiliations.
Electronic supplementary material
Below is the link to the electronic supplementary material.
Rights and permissions
Springer Nature or its licensor (e.g. a society or other partner) holds exclusive rights to this article under a publishing agreement with the author(s) or other rightsholder(s); author self-archiving of the accepted manuscript version of this article is solely governed by the terms of such publishing agreement and applicable law.
About this article
Cite this article
Prasad, P.S.H., Satyanarayana, A.N.V. Assessment of universal thermal climate index (UTCI) using the WRF-UCM model over a metropolitan city in India. Int J Biometeorol (2024). https://doi.org/10.1007/s00484-024-02714-5
Received:
Revised:
Accepted:
Published:
DOI: https://doi.org/10.1007/s00484-024-02714-5