Abstract
Mutations in the pivotal metabolic isocitrate dehydrogenase (IDH) enzymes are recognized to drive the molecular footprint of diffuse gliomas, and patients with IDH mutant gliomas have overall favorable outcomes compared to patients with IDH wild-type tumors. However, survival still varies widely among patients with IDH mutated tumors. Here, we aimed to characterize molecular signatures that explain the range of IDH mutant gliomas. By integrating matched epigenome-wide methylome, transcriptome, and global metabolome data in 154 patients with gliomas, we identified a group of IDH mutant gliomas with globally altered metabolism that resembled IDH wild-type tumors. IDH-mutant gliomas with altered metabolism have significantly shorter overall survival from their IDH mutant counterparts that is not fully accounted for by recognized molecular prognostic markers of CDKN2A/B loss and glioma CpG Island Methylator Phenotype (GCIMP) status. IDH-mutant tumors with dysregulated metabolism harbored distinct epigenetic alterations that converged to drive proliferative and stem-like transcriptional profiles, providing a window to target novel dependencies in gliomas.
Graphical Abstract

Similar content being viewed by others
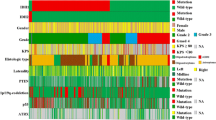
Avoid common mistakes on your manuscript.
Introduction
Gliomas are the most common and lethal brain tumors. Gain-of-function mutations in the isocitrate dehydrogenase genes (IDH1/2) are used to differentiate astrocytoma and oligodendrogliomas from the more deadly glioblastoma (IDH wild-type) [5, 6]. Mutations in IDH1/2 result in the accumulation of the oncometabolite R-2-hydroxyglutarate that drives widespread restructuring of the DNA methylome (glioma CpG-island methylator phenotype, GCIMP) [23, 33]. In general, patients with tumors that have mutations in IDH1 and IDH2 have favorable prognoses overall; however, some patients with IDH mutant gliomas can have poor outcomes [35]. While homozygous deletion of CDKN2A/B and variability in GCIMP level can identify some IDH mutant tumors with poor outcomes, these do not capture all patients.
The clinical and genetic footprint of gliomas is predicated on the metabolic IDH enzymes. Metabologenomic characterization of gliomas is currently limited by the paucity of resources with matched comprehensive genomic, epigenomic, and metabolomic data. Integration of these datatypes could importantly reveal novel clinical and biological insights as in other cancers. To address this, we performed matched DNA methylation profiling, RNA sequencing, and global metabolomic profiling on a cohort of 154 patients with diffuse gliomas with detailed clinical annotation. We characterize for the first time a group of metabolically dysregulated IDH-mutant gliomas with prognostic relevance as they confer distinct clinical behavior and molecular alterations that inform on biological behavior and novel therapies.
Methods
Clinically annotated patient samples
We assembled a valuable cohort of diffuse gliomas from adult (age ≥ 18) patients that underwent surgery at the University Health Network (UHN; Toronto, Ontario, Canada). In total, we analyzed 154 glioma samples that had sufficient biospecimen (> 500 mg fresh-frozen tissue) with comprehensive matched clinical annotation for meaningful multiplatform molecular analyses. These specimens were taken at the time of surgical resection, immediately snap-frozen in liquid nitrogen, and stored at -80ºC in our UHN Biobank. Clinical data, including but not limited to: patient demographic information (sex, age), date of surgery, tumor IDH-status, tumor 1p/19q codeletion status, WHO grade, longitudinal follow-up, and vital status, were collected for each patient. Overall survival was defined as the time from the initial surgery to date of death. Central pathology review was performed for each case whereby hematoxylin and eosin (H&E) slides and results of molecular subtyping were reviewed by two experienced neuropathologists to confirm the clinical diagnosis in accordance with the 2021 WHO Brain Tumor Classification [18]. Collection of all samples and clinical data were carried out in accordance with local institutional ethics and review board guidelines.
Metabolic profiling
Metabolomic studies were performed at Metabolon Inc. using a nontargeted platform able to quantify a wide array of metabolites with a high degree of confidence using three independent platforms: ultrahigh performance liquid chromatography/tandem mass spectrometry (UHPLC/MS–MS2) for basic species, UHPLC/MS–MS2 for acidic species, and gas chromatography/mass spectrometry (GC/MS) [10, 12]. Sample processing has been previously described in detail [12]. Independent quantification of specific metabolites of interest was also performed using conventional high-pressure liquid chromatography/tandem mass spectrometry (HPLC–MS/MS). In brief, proteins were precipitated from 100 μL of homogenized tumor tissue in sterile water. The precipitate extract was subsequently split into aliquots and reconstituted in various solutions as previously described for subsequent analysis on all three platforms. Both tumor samples and healthy controls were randomized to various platform run days. Aliquots used for UHPLC/MS–MS2 were analyzed using an LTQ mass spectrometer (MS) (ThermoFisher) using electrospray ionization. The MS instrument scanned 99–1000 m/z and alternated between MS and MS/MS scans at a speed of approximately six scans per second (3 MS and 3 MS/MS scans). Specimens used for GC/MS were separated on a 5% phenyldimethyl silicone column using helium as the carrier gas with a temperature increase from 60 to 340 ºC and analyzed on a Thermo-Finnigan Trace DSQ MS (ThermoFisher) operating a unit mass resolving power with electron impact ionization and a 50–750 atomic mass unit scan range. For HPLC/MS-MS analyses, aliquots were separated using a Surveyor MSplus pump (ThermoFisher) equipped with a 3 μm particle 2.1 × 100 mm Aquasil C18 column (ThermoFisher), gradient-eluted, and analyzed using ESI LTQ MS. The instrument scanned 99–1500 m/z, alternated between positive and negative polarity within a given injection of a sample. All metabolites were identified by comparing ion features in the tumor samples with a reference library of chemical standard entries using automated software developed at Metabolon [10, 12]. Metabolome cohorts were generated using unsupervised hierarchical clustering on GeneCluster 3.0 and principal component analysis [14].
For statistical analyses of our metabolomics data, missing values were assumed to be below the technical limits of detection and resolved using minimum value imputation. Statistical analysis of log-transformed metabolite concentration data was done using R version 3.6.3 (http://cran.r-project.org/), a free, open-source software [25].
DNA and RNA extraction and processing
DNA and RNA were extracted from the same tissue samples from each respective patient. DNA was extracted from the tumor as well as matched normal tissue (whole blood) using the DNeasy Blood and Tissue Kit (Qiagen, USA) and quantified using the Nanodrop 1000 Instrument (ThermoScientific, USA). Total RNA was isolated from tumor samples using the RNeasy Mini Kit (Qiagen, USA) and quantified using the PicoGreen assay. RNA integrity was assessed using the Agilent 2100 Bioanalyzer (RNA; Agilent, US) and samples with RNA Integrity Number (RIN) > 7 were selected for further library preparation and sequencing.
Genome-wide DNA methylation profiling and detection of copy-number alterations
Illumina Infinium MethylationEPIC BeadChip array (lllumina, San Diego, USA) was used to obtain genome-wide DNA methylation profiles of all tumors using 250–500 ng of bisulfite-converted DNA (EZ DNA Methylation Kit, Zymo, California, USA). Raw methylation files (*.idat) were imported, processed, and normalized (ssNoob) using minfi (v1.34) [1]. Probes that failed to hybridize (detection p value > 0.01) in one or more samples were removed from further downstream analyses. Probes that overlapped with known single-nucleotide polymorphisms, cross-reactive probes, and probes that localized on X and Y chromosomes were also removed from all unsupervised analyses. Differentially methylated probes were identified using limma-based modeling approaches [26]. When comparing gliomas of different metabolic groups, CpG sites were considered differentially methylated if the absolute mean differences in β value > 0.1 and adjusted p value (FDR-corrected) < 0.05. Probe annotation was performed using the UCSC Genome Browser (hg38 assembly).
Copy-number alterations were identified from the Infinium MethylationEPIC BeadChip array data using the previously described minfi and conumee pipelines as part of the R Bioconductor package (v3.13) [13, 19]. Briefly, DNA methylation array data were loaded, preprocessed, and normalized using the minfi package. Copy-number analysis was subsequently performed using the conumee R package, which compared each tumor sample to a set of user-provided normal reference samples. A set of copy-number plots for the entire genome, individual chromosomes, and specific gene loci of interest were generated and exported as text files for visualization using the Integrated Genome Viewer and for use in subsequent analysis [27].
Classification of G-CIMP and CDKN2A/B status
GCIMP status for each tumor was determined by its methylation profile using a Random Forest (RF) model as previously described [9, 23]. CDKN2A/B status (homozygous deletion) was inferred through copy-number variation collected through DNA methylation analysis using a previously established methodology: a log2 copy ratio of ≤ − 11 was defined as homozygous deletion and − 1.1 < log2 copy ratio ≤ − 0.4 as hemizygous deletion [8, 15].
RNA sequencing
mRNA libraries were generated using NEB Ultra II directional mRNA library prep kit in accordance with the manufacturer’s instructions. Libraries were sequenced on the Illumina HiSeq 2500 high output flow cell (2 × 126 bp), sequenced with 3 samples per lane to obtain approximately 70 million reads per sample. Raw sequencing data (FastQ) were processed and aligned to the human reference genome (GRCh38) using STAR (v2.6.0a) [11]. SamTools (v1.3) was used to remove duplicate reads and sort all remaining reads [16]. The package Rsubread (v1.5.0) was used to compute raw gene-expression counts for each sample using featureCounts [17]. The edgeR package (v3.22.3) was used to normalize the raw gene-expression counts by counts-per-million (CPM) and perform TMM (trimmed mean of M) normalization to remove genes with low counts using a CPM-cutoff [28]. The CPM-cutoff values were determined empirically by identifying the minimum value required to achieve the ideal normalization across samples while filtering out noise. The best CPM-cutoff was determined to be 1 using only protein-coding genes.
TCGA database
The Cancer Genome Atlas (TCGA) is a publicly available database containing genomic, epigenomic, transcriptomic, and proteomic data spanning 20000 primary cancers and normal samples spanning 33 cancer types (https://portal-gdc-cancer-gov.myaccess.library.utoronto.ca/). DNA methylation and matched RNA expression data for diffuse gliomas was obtained from the TCGA data portal along with relevant clinical data including gender, age, histological type, GCIMP status, survival, and outcome.
Survival analysis
Kaplan–Meier survival plots were generated to compare overall survival between independent groups. Log-rank tests were used to test the null hypothesis that there is no survival difference between independent subgroups with statistical significance set at p < 0.05. Univariable and multivariable hazard ratios (HRs) with 95% CI and p values for clinical variables and subgroups were calculated by fitting Cox Proportional Hazards Models.
Epigenetic mitotic clock analysis
Molecular mitotic-like clocks are used to provide an approximate estimate of the relative number of cell divisions of a tissue in an individual. Errors in maintenance of DNA methylation during cell division may accumulate in tissues that match its stem cell division rate and chronological age, and higher mitoses in the stem cell pool may lead to epigenetic cellular heterogeneity that may predispose tissues to further neoplastic transformation [37]. Epigenetic mitotic clocks are calculated using DNA methylation-based age-correlative mathematical models to examine regions of the genome that are either fully methylated or unmethylated in multiple fetal tissues but subsequently gain or lose methylation as a function of mitotic age. We used several previously described methods: epiTOC, epiTOC2, and solo-WCGW (or HypoClock) to calculate the epigenetic mitotic clock of gliomas in our different metabolic groups [32, 37, 38]. The epiTOC model calculates a weighted average of methylation across 354 CpGs on the 850 k array at gene promoters corresponding to the PRC2 complex that are unmethylated in fetal tissue, and accumulate more methylation with age and increasing cell division [37]. The epiTOC2 model estimates mitotic age, while taking the patient’s chronological age into consideration, using a weighted group of 151 CpGs from the epiTOC model that are the most likely to undergo changes in DNA methylation with age [32]. The solo-WCGWs are a subset of CpGs at the WCGW motif (where W equates to an A or T base) that are hypomethylated in fetal tissues and gradually gain methylation with increasing age and cell division. There are 6214 solo-WCGWs that are found on the EPIC array. Of these, 648 are uniformly hypomethylated across multiple different tissue types, as previously described. A weighted average of these 648 CpG sites was used to derive the HypoClock score [38].
Determination of stemness
Signatures to quantify stemness have been derived from a number of publicly available molecular profiles from normal and cancer cells. We compared the stemness indices of our gene-expression signatures from our glioma cohort to three independent mRNA-derived gene sets associated with stemness that have been previously published using single sample gene set enrichment analysis (ssGSEA) [20, 21, 36]. The score derived from ssGSEA corresponds to the degree to which the input gene signature is coordinately up- or downregulated within a sample [2].
Results
We performed a comprehensive, integrative epigenomic, genomic, and metabolomic analyses of diffuse human gliomas in 154 patients with sufficient tissue and clinical data. The average age of patients in this cohort was 42 years (range 21–78 years) and 64% of patients were males. A total of 68% of the patients had IDH-mutant tumors confirmed by sequencing. The patients were divided into two separate cohorts: a discovery cohort made up of 64 patients, 70% IDH-mutant tumors, and 30% wild type, followed by a verification cohort composed of the remaining 90 patients, 67% of whom were IDH-mutants and 33% were IDH-wildtype. The detailed clinical characteristics of these cohorts are presented in Supplementary Table 1, online resource.
We first sought to comprehensively characterize the global metabolic profile by performing single-shot metabolomic profiling of a cohort of 64 gliomas of both IDH mutant and IDH wild-type genotypes. In total, we quantified 676 metabolites that mapped to corresponding macromolecules of amino acids, carbohydrates, cofactors and vitamins, energy, lipid, nucleotide, and peptides. Of the 676 metabolites, 76 xenobiotic metabolites were removed to limit the effects of exogenous confounders. Unsupervised analyses of global metabolomic data by hierarchical clustering and examination of principal components did not clearly separate the gliomas by IDH mutation status or histopathological grade (Fig. 1A, B and Supplementary Fig. 1, online resource).
Global metabolomic profiling reveals a group of IDH mutant gliomas that resemble IDH wild-type tumors with poor outcomes. a, Principal component analysis using all quantitated metabolites of 64 diffuse gliomas. Samples are colored according to IDH mutation status. b, Heatmap showing hierarchical cluster results using all quantitated metabolites. Metabolites are mapped to respective macromolecules using side annotation. Annotation to IDH mutation status, CNS WHO grade and 1p/19q codeletion status are shown at the top. c, Volcano plot showing differentially accumulated metabolites between IDH mutant and IDH wild-type gliomas. d, Dot plot showing relative abundance of each metabolite in mutant samples versus wild-type samples. Metabolites are grouped and colored by corresponding macromolecular categories. Horizontal red lines represent thresholds at Log2FC 1 and -1. e, Hierarchical clustering of samples using abundance of 2HG and sphingadienine. f, Dot plot showing relative abundance of 2R/S-hydroxyglutarate measured by high-pressure liquid chromatography–tandem mass spectrometry (HPLC–MS/MS) in IDH mutant and wild-type samples stratified by abundance of sphingadienine. g, Kaplan–Meier survival distributions of gliomas stratified by sphingadienine level and IDH-status. P value reported is from log-rank test
Only 6.5% (39/600) metabolites were differentially abundant between IDH mutant and IDH wild-type gliomas (Fig. 1C, D and Supplementary Fig. 2, online resource). Interestingly, while metabolic profile of IDH mutant and wild-type gliomas were largely similar, there were two main differentiating metabolites: 2-hydroxyglutrate in IDH mutant tumors and sphingadienine in IDH wild types. Hierarchical clustering using these two most differentiating metabolites revealed two groups of tumors, one group entirely made of IDH-mutant tumors, and second group with both IDH-wildtype and IDH-mutant tumors. IDH-mutant tumors that clustered together with IDH-wildtype tumors included both 1p/19q codeleted and intact tumors and metabolically resembled IDH wild-type gliomas with moderately elevated sphingadienine and lower 2-hydroxyglutarate levels (Fig. 1E). We confirmed this by independently quantifying 2-hydroxyglutarate by high-pressure liquid chromatography–tandem mass spectrometry (HPLC–MS/MS) (Fig. 1F). Importantly, this subset of IDH-mutant gliomas that metabolically resembled IDH-wildtype gliomas with moderately elevated sphingadienine and lower 2-hydroxyglutarate levels had significantly shorter overall survival when compared to their IDH mutant counterparts with high 2-hydroxylutarate and low sphingadienine (Fig. 1G).
To further explore and verify the metabolic alterations among IDH mutant tumors, we profiled the global metabolome of a second independent cohort of 90 IDH mutant and IDH wild-type gliomas across a range of WHO grades. A total of 622 metabolites were analyzed after the removal of xenobiotics. Unsupervised analyses by principal components and consensus clustering using all metabolites demonstrated two stable and robust metabolic groups of gliomas. One was composed entirely of IDH mutant tumors, whereas IDH mutant tumors and wild-type glioblastomas clustered together in the second group (Fig. 2A, B). The IDH mutant tumors that clustered with IDH wild-type glioblastomas (herein referred to as metabolically dysregulated IDH mutants) were a diverse group: they included both 1p/19q codeleted (55%) and intact tumors (45%), both primary (35%) and recurrent samples (65%), and represented both WHO Grade 3 (55%) and Grade 4 (44%) tumors. When comparing the dysregulated tumors to their non-dysregulated IDH mutant counterparts, we found that the group was enriched for WHO Grade 4 tumors (44 vs 12%, p = 0.001752), and tumors that had received treatment with alkylating agents and radiotherapy (65 vs 22% p = 9.758e-08). Most importantly however, 35% of IDH mutant tumors within this metabolically dysregulated cluster had never received prior treatment, and 55% were classified as WHO Grade 3, indicating that the observed metabolic differences between the IDH mutant tumors in both clusters were not a direct consequence of prior alkylating treatment nor grade alone, but rather a general dysregulation of metabolism.
Shift of global metabolism results in similarity of some IDH mutant gliomas to IDH wild-type glioblastomas. a, Heatmap showing consensus cluster results using all quantitated metabolites of a separate cohort of 90 gliomas. IDH mutant gliomas that cluster with IDH wild-type gliomas are referred to as metabolically dysregulated IDH mutant tumors. Metabolites are mapped to respective macromolecules using side annotation. Annotation to IDH mutation status, CNS WHO grade, 1p/19q codeletion status and treatment using alkylating chemotherapy are shown at the top. b, Principal Component Analysis of samples from Fig. 2A. Ellipsoids represent standard deviation. c, Volcano plot showing differentially accumulated metabolites between metabolically dysregulated and non-dysregulated IDH-mutant gliomas. d, Dot plot showing relative abundance of each metabolite in metabolically dysregulated IDH mutant versus non-dysregulated counterparts. Horizontal red lines represent thresholds at Log2FC 1 and -1. e, Scatterplot showing abundance of each metabolite for metabolically dysregulated IDH mutant gliomas (y-axis) and IDH wild-type gliomas (x-axis) in comparison to non-dysregulated IDH mutant gliomas. Metabolites are colored by relationship to macromolecules. f, Kaplan–Meier survival distributions of gliomas stratified by metabolic groups of IDH-mutant tumors. P value reported is from log-rank test
Nearly 30% of metabolites were differentially abundant between IDH mutant gliomas with and without dysregulated metabolism (186/622, Log2FC > 1 or < − 1 and FDR < 0.05, Fig. 2C). The shift in metabolic profile in the metabolically dysregulated IDH mutant cohort was not limited to a few metabolites, but rather the full spectrum of metabolites from all macromolecules (Fig. 2D). This shift in metabolism was highly similar to the changes observed when comparing IDH wild-type glioblastomas to IDH mutant gliomas without dysregulated metabolism (Fig. 2E). We again noted and verified in this second cohort that the IDH mutant gliomas with dysregulated metabolism had statistically significant shorter overall survival in comparison to non-dysregulated IDH-mutant gliomas (median OS 118.9 months vs 173.6 months, p = 0.048, Fig. 2F). The differences in outcomes were not explained by prior treatment status or WHO grade of the tumors in each group (Supplementary Fig. 3A, B, online resource). As well, these tumors were not more aggressive from the onset of disease, as there was no difference in the time to initial recurrence when comparing metabolically distinct groups of IDH mutant gliomas (median 36.8 months vs median 45.4 months, p = 0.54, Cox Proportional Hazards Regression, Supplementary Fig. 3C, online resource). Overall, metabologenomic characterization in two separate cohorts led us to identify a subset of IDH-mutant gliomas that are metabolically and phenotypically distinct to their counterparts with significantly shortened overall survival.
We next sought to better understand the metabolically dysregulated IDH-mutant gliomas by performing mRNA-sequencing and DNA methylation profiling in a subset of tumors with matched metabolomic data. We first examined mRNA abundance data and found that metabolically dysregulated IDH-mutant gliomas were generally separable from their non-dysregulated counterparts and IDH wild-type tumors by Principal Component Analyses (Fig. 3A). Metabolically dysregulated IDH-mutant gliomas and IDH-wildtype glioblastomas were both characterized by elevated expression of a common set of pathways involved in cell-cycle regulation and cell proliferation when compared to the non-dysregulated IDH-mutant gliomas by Gene Set Enrichment Analyses using gene-expression data (Fig. 3B). Similarly, metabolically dysregulated IDH-mutant gliomas also uniquely showed enrichment of several pathways involved in cell-cycle regulation, cellular motility, and cellular division that was not shared with IDH-wildtype gliomas, providing unique potential vulnerabilities for this subcohort. The differences in gene expression was not driven by a highly unstable genome as the extent of large-scale copy-number alterations were similar in IDH-mutant gliomas with and without metabolic dysregulation (Fig. 3C).
Metabolically reprogrammed IDH-mutant gliomas also harbor distinctive transcriptomic and methylome signatures indicative of global molecular rewiring. a, Principal component analysis using mRNA expression data of all protein-coding genes. First 3 principal components are plotted. Samples are colored by metabolic grouping. Ellipsoids represent standard deviation. b, Network of activated gene circuits by mRNA data according to metabolic groups. Nodes represent pathways and edges represent shared genes between pathways. Pathways within orange box are upregulated in metabolically dysregulated IDH mutant gliomas vs non-dysregulated counterparts. Pathways within purple box are upregulated in IDH wild-type gliomas vs non-dysregulated IDH mutant gliomas. Pathways within intersection of orange and purple box are shared pathways between IDH mutant dysregulated and wild-type gliomas. c, Genome-wide copy-number variability inferred from DNA methylation data stratified by metabolic groups. d, Heatmap showing consensus cluster results using the top 15,000 variably methylated CpG sites across all gliomas. One consensus cluster group is almost entirely composed of metabolically dysregulated IDH mutant tumors. e, Boxplots showing the average degree of methylation across all CpGs in the genome for each metabolic group. f, Volcano plot showing distribution of differences in DNA methylation between IDH-mutant metabolically dysregulated and non-dysregulated gliomas. g, Odds ratios and 95%CI (gray lines) of probes from Fig. 3F relative to the distribution of probes found on the 850 K EPIC array. Probes are separated by their genomic regions. h, Heatmap of the top 300 differentially methylated regions between groups of gliomas. Metabolic groups of IDH mutant gliomas can be separated from each other and from IDH-wildtype tumors. i, Boxplots showing single sample GSEA scores for three different stemness gene-expression signature stratified by metabolic groups of gliomas
Next, we analyzed the epigenotypic differences between metabolic groups of gliomas. Unbiased consensus clustering of all glioma samples in our cohort with matched DNA methylation and metabolic data revealed three robust methylation-derived groups of gliomas, one composed entirely of IDH wild-type tumors and two separate clusters of IDH-mutant tumors (Fig. 3D). Metabolically dysregulated IDH mutant tumors were almost exclusively identified in one of these IDH-mutant groups (Odds Ratio = 52.5, 95%CI 13.0–272.1, p = 1.94e-12), and showed an intermediate degree of methylation across the genome that was statistically different compared to IDH-mutant and IDH-wildtype tumors (Fig. 3E). When compared to IDH-wildtype tumors, the metabolically dysregulated IDH-mutant gliomas showed relative global hypermethylation (Supplementary Fig. 4A, B, online resource). By contrast, metabolically dysregulated IDH mutant gliomas were characterized by relative global hypomethylation at intergenic regions compared to non-dysregulated IDH-mutant gliomas, but highly prominent CpG-island hypermethylation (Fig. 3E, F). The differences in methylation between metabolic groups of IDH mutant gliomas could be captured in the plasma of patients using a cell-free DNA methylated immunoprecipitation technique we have previously applied to brain tumors (Figs. 3H and Supplementary Fig. 5, online resource), which recapitulated the distinct methylation grouping derived from the tissue samples, potentially providing an avenue for non-invasive detection and longitudinal tracking of this metabolically dysregulated molecular subtypes of IDH-mutant gliomas [22, 29].
Homozygous deletion of CDKN2A/B (cyclin-dependent kinase inhibitor 2A/B) portends a poor prognosis in IDH-mutant gliomas. Astrocytomas harboring homozygous deletion of CDKN2A/B are categorized as CNS WHO Grade 4 tumors in the most recent 2021 World Health Organization (WHO) classification of CNS tumors [4, 18, 30] To examine the interplay between metabolic status and CDKN2A/B status, we characterized the copy-number status of CDKN2A/B among the metabolically dysregulated IDH-mutant tumors. We found that homozygous deletion of CDKN2A/B occurred in 11% (N = 3/27) of IDH-mutant metabolically dysregulated tumors, and while this was greater in proportion than that which occurred in IDH-mutant non-dysregulated tumors (3% (N = 1/29), p = 0.0015), over half of the dysregulated tumors did not harbor this alterations and loss of CDKN2A/B was not exclusive to this group. Moreover, CDKN2A/B status did not show a clear survival benefit upon stratification of our data set (median OS 112.4 months vs 159 months, p = 0.84, Supplementary Fig. 4D, online resource). Hemizygous CDKN2A/B status also has clinical relevance in gliomas with overall shorter survival when compared to CDKN2A/B intact IDH mutants [15]. CDKN2A/B hemizygous deletion was also abundant in the metabolically dysregulated group (33% N = 9/27 vs 7% N = 2/29, p = 0.01541) in our cohort.
Importantly, the CpG-island methylator phenotype (GCIMP)-low pattern of diffuse astrocytomas has been shown to be prognostically unfavorable, and was almost exclusively found in the methylation cluster composed of metabolically dysregulated IDH-mutant gliomas (N = 7/27, p = 0.009) (Fig. 3D) [23, 31]. However, the GCIMP-low tumors represented only 35% of the metabolically dysregulated tumors in this cohort, suggesting that metabolic dysregulation is not simply explained by the GCIMP-low status alone. Given the association between GCIMP status and stemness, we next looked to examine stemness associated signatures between IDH-mutant groups [31]. Using 3 independent mRNA-derived stemness signatures, we found that IDH-mutant metabolically dysregulated gliomas had significantly higher stemness scores when compared to non-dysregulated counterparts and similar stemness indices to IDH-wildtype tumors (Fig. 3E) [20, 21, 36].
To demonstrate that our findings are generalizable in a completely independent cohort, we applied the DNA methylation signature from our cohort to methylome data of 683 gliomas from The Cancer Genome Atlas. Two distinct groups of IDH-mutant gliomas of mixed 1p/19q codeleted and non-codeleted genomic make-up were identified (Fig. 4A). One group had methylation signature similar to the metabolically dysregulated IDH mutant gliomas, with relative global hypomethylation at intergenic regions but highly prominent CpG-island hypermethylation in comparison to their other IDH mutant counterparts (Fig. 4B) together with a non-specific hypermethylation relative to IDH wild-type gliomas (Supplementary Fig. 4C, D, online resource). All of the previously described GCIMP-low tumors in the TCGA cohort classified with this group that clustered with metabolically dysregulated IDH-mutant gliomas (Fig. 4A). Once again, the majority of the metabolically dysregulated IDH-mutant gliomas (62%) were not GCIMP-low. Of note, we also found that the metabolically dysregulated IDH-mutant gliomas in the TCGA had significantly shorter overall survival compared to their counterparts (Fig. 4C), and in fact, metabolic dysregulation was the strongest independent predictor of outcome among IDH-mutant gliomas on multivariable Cox regression analysis (HR 4.34, 95%CI 2.04–9.25), even after controlling for established prognostic factors in diffuse gliomas including 1p/19q codeletion status, GCIMP status, CDKN2A/B homozygous deletion, MGMT promoter methylation status, and 2021 WHO grade (Supplementary Table 2, online resource). When comparing the dysregulated status of IDH-mutants within traditional IDH-mutant groups [oligodendroglioma (1p/19q codeleted) and astrocytoma (1p/19q intact)], we see a robust difference in overall survival. IDH-mutant codeleted tumors had a median overall survival of 134.2 months, while their metabolically dysregulated counterparts were 78.2 months (p = 0.0016). We saw a similar trend among the non-codeleted IDH-mutant gliomas, with median overall survival for the dysregulated tumors significantly lower than the non-dysregulated tumors (23.3 vs 105.1 months, respectively, p < 0.0001; Supplementary Fig. 6, online resource).
Independent validation of metabolically dysregulated IDH mutant gliomas. a, Heatmap of consensus matrix from consensus hierarchical clustering of DNA methylation data from the TCGA using features from Fig. 3D. b, Odds ratios and 95%CI (gray lines) of differentially methylated probes among metabolically dysregulated IDH mutant gliomas from the TCGA relative to the background distribution of all probes. Probes are separated by their genomic regions. Genome-wide copy-number alterations inferred from DNA methylation data stratified by metabolic groups. c, Kaplan–Meier survival distributions of gliomas from the TCGA stratified by Consensus Cluster groups from (A). P value reported is from log-rank test. d, Network of activated gene circuits of TCGA gliomas by classification of metabolic groups. Nodes represent pathways and edges represent shared genes between pathways. Pathways within orange box are upregulated in metabolically dysregulated IDH mutant gliomas vs non-dysregulated counterparts. Pathways within purple box are upregulated in IDH wild-type gliomas vs non-dysregulated IDH mutant gliomas. Pathways within green box are upregulated in non-dysregulated IDH mutant gliomas. Nodes in pink are shared between IDH mutant dysregulated and IDH wild-type tumors, and nodes in light green are shared between IDH mutant dysregulated and non-dysregulated counterparts. e, Boxplots showing scores for three different stemness gene-expression signatures among TCGA samples stratified by metabolic groups. f, Boxplots showing the distribution of using epigenetic mitotic clocks in Toronto cohort samples with epiTOC model (left), epiTOC2 model (middle), and HypoClock model (right). Comparisons that do not reach statistical significance (p < 0.05) are denoted. g, Boxplots showing the distribution of using epigenetic mitotic clocks with orientation as in (F), now using samples from the TCGA. Comparisons that do not reach statistical significance (p < 0.05) are denoted
DNA methylation profiling has been utilized in the clinical setting to differentiate between tumor types and to sub-stratify tumors into clinically meaningful groups. The DFKZ classifier subdivides IDH-mutant astrocytomas into two groups: an MC Astrocytoma IDH-mutant lower grade and high grade [7]. We noted a significant overlap between our dysregulated astrocytomas and the high-grade methylation class within our validation cohort (N = 8/10; Supplementary Fig. 7, online resource). This prompted us to further explore the use of DNA methylation as a tool to differentiate dysregulated IDH mutant gliomas from the non-dysregulated tumors within our TCGA cohort. To this end, we completed a differential methylation analysis of dysregulated and non-dysregulated oligodendrogliomas and astrocytomas, followed by supervised hierarchical clustering. This revealed differences in DNA methylation patterns that could potentially be utilized in the clinical setting (Supplementary Fig. 8, online resource).
We then examined the mRNA abundance data of diffuse gliomas from the TCGA, and similar to findings from our discovery cohort, we found that metabolically dysregulated IDH mutant gliomas harbored enrichment of cellular pathways that converged on cell proliferation and invasion on gene-expression analyses (Fig. 4D). As well, metabolically dysregulated gliomas from the TCGA cohort also showed higher stem-like transcriptomes (Fig. 4E).
These findings prompted us to next examine different regions of the genome that either gain or lose methylation from “ground-zero” fetal states as a function of mitotic age (i.e., epigenetic mitotic clocks). We found that metabolically dysregulated IDH mutant gliomas showed a higher degree of divergence of methylation from expected fetal levels at these regions when compared to their IDH mutant counterparts, even after correcting for the chronological age of patients, indicative of a higher total number of stem cell divisions per stem cell (Fig. 4F). These patterns were almost identical in samples from the TCGA (Fig. 4G). Overall, the findings from our own cohorts and validation in the TCGA cohort point to discovery of a subset of IDH-mutant gliomas that are characterized by aberrant epigenome-wide alterations in combination with stem-like and proliferative transcriptomes and globally dysregulated metabolism, with short survival and classification that encompasses previously described prognostic markers in IDH-mutant gliomas.
Discussion
Using a cross-platform analysis, we have uncovered a novel group of IDH-mutant gliomas that share metabolic features with IDH-wildtype tumors, and have a worse prognosis than their IDH-mutant counterpart. These metabolically dysregulated IDH mutant gliomas encompass both 1p/19q codeleted and non-codeleted tumors, and harbor distinct molecular alterations both at the epigenomic and genomic levels. The recognized prognostic markers of CDKN2A/B homozygous deletion and GCIMP-low are enriched in metabolically dysregulated IDH-mutant gliomas, but do not account for more than half of these tumors. CpG-island hypermethylation in dysregulated IDH-mutant gliomas is associated with stem-like and proliferative transcriptome, and in line with this, the metabolically dysregulated IDH-mutant tumors have significantly shorter survival than their non-dysregulated IDH-mutant counterparts. In fact, metabolic dysregulation was the strongest prognostic factor of outcome among IDH-mutant gliomas.
The genomic and epigenomic hallmarks of diffuse gliomas have been thoroughly investigated in prior studies [3, 23, 24, 31, 33,34,35]. These landmark studies identified that the genomic subtypes of diffuse gliomas were anchored in tumorigenic mutations of metabolic genes IDH1 and IDH2. Despite this, a few studies have focused on understanding the metabolic underpinning gliomas, with only a handful of existing reports examining global metabolic profiles of these tumors [10, 24]. To our knowledge, only one of these has looked at global metabolic profiles in the context of genomic determinants of gliomas, and this study was limited to mainly glioblastomas [24].
The robust inclusion of IDH-mutant gliomas in our study facilitated the discovery of two metabolic groups of IDH-mutant gliomas, interestingly not stratified based on 1p/19q codeletion. One group displayed strikingly divergent metabolism that was highly similar to that of IDH-wildtype glioblastomas. Comparing these two metabolic groups of IDH-mutant tumors revealed that 30% of metabolites were differentially abundant overall.
The distinct upstream molecular alterations at the epigenomic and genomic levels in metabolically dysregulated IDH-mutant gliomas are indicative of more widespread molecular rewiring in this group. Moreover, while the group encompassed tumors with several negative prognostic features that are currently recognized, namely CDKN2A/B homozygous deletions and CpG-island hypermethylation status, more than half of the IDH-mutant metabolically dysregulated tumors were CDKN2A/B wild types, and were GCIMP-high. Indeed, on our multivariable Cox regression analysis, recurrent, GCIMP, and CDKN2A/B status were not independently associated with outcome, whereas metabolic dysregulation status in comparison was the strongest factor associated with overall survival. While tumors with higher grade and prior treatment were enriched in this group, there were substantial proportion of tumors that were treatment naïve and lower grade, indicating that the process of metabolic dysregulation is not a direct consequence of treatment or grade.
The metabolic heterogeneity we describe among IDH-mutant gliomas has considerable implications in the management of patients. Although genome-based classifications are now commonly adopted in clinical practice, it is important to remember that the genotype of a tumor is not always reflective of the phenotype and behavior, which was indeed evident in the results we presented here. The metabolic profile provides unique information on glioma phenotypes, which, as we demonstrated, can facilitate a more comprehensive understanding of glioma biology when these data are coupled with genomic data. To this end, we have also showcased the ability of our dysregulated metabolic group to be determined through methylation profiling, a technique that is becoming more accessible clinically. As well, the impact of the metabolic heterogeneity that we describe here should be investigated when targeted treatments based on metabolic dependencies are being considered. In line with this, we found that metabolically dysregulated IDH-mutant gliomas activate cell-cycle pathways, similar to IDH-wildtype tumors, to drive cellular proliferation. However, several of the cell-cycle pathways enriched among in the metabolically dysregulated pathways were not shared with IDH-wildtype gliomas, potentially rendering these tumors susceptible to unique therapeutic vulnerabilities that warrant further investigation.
Data availability
Raw methylation (idat files) from Universiy Health Network (UHN) for the IDH-Mutant Gliomas have been deposited to the European Genome-Phenome Archive with the dataset identifier of EGAS00001006961 (https://wwwdev.ebi.ac.uk/ega/studies/EGAS00001006961). The Cancer Genome Atlas (TCGA) dataset is open access. Additional idats for the IDH wild type cohort, RNAseq data and metabolomic data from this study can be made available upon request to our corresponding author (gelareh.zadeh@uhn.ca).
References
Aryee MJ, Jaffe AE, Corrada-Bravo H, Ladd-Acosta C, Feinberg AP, Hansen KD et al (2014) Minfi: a flexible and comprehensive Bioconductor package for the analysis of Infinium DNA methylation microarrays. Bioinformatics 30:1363–1369. https://doi.org/10.1093/bioinformatics/btu049
Barbie DA, Tamayo P, Boehm JS, Kim SY, Moody SE, Dunn IF et al (2009) Systematic RNA interference reveals that oncogenic KRAS-driven cancers require TBK1. Nature 462:108–112. https://doi.org/10.1038/nature08460
Barthel FP, Johnson KC, Varn FS, Moskalik AD, Tanner G, Kocakavuk E et al (2019) Longitudinal molecular trajectories of diffuse glioma in adults. Nature 576:112–120. https://doi.org/10.1038/s41586-019-1775-1
Brat DJ, Aldape K, Colman H, Figrarella-Branger D, Fuller GN, Giannini C et al (2020) cIMPACT-NOW update 5: recommended grading criteria and terminologies for IDH-mutant astrocytomas. Acta Neuropathol 139:603–608. https://doi.org/10.1007/s00401-020-02127-9
Brat DJ, Verhaak RGW, Aldape KD, Yung WKA, Salama SR, Cooper LAD et al (2015) Comprehensive, Integrative Genomic Analysis of Diffuse Lower-Grade Gliomas. N Engl J Med 372:2481–2498. https://doi.org/10.1056/NEJMoa1402121
Brennan CW, Verhaak RGW, McKenna A, Campos B, Noushmehr H, Salama SR et al (2013) The somatic genomic landscape of glioblastoma. Cell 155:462–477. https://doi.org/10.1016/j.cell.2013.09.034
Capper D, Jones DTW, Sill M, Hovestadt V, Schrimpf D, Sturm D et al (2018) DNA methylation-based classification of central nervous system tumours. Nature 555:469–474. https://doi.org/10.1038/nature26000
Capper D, Stichel D, Sahm F, Jones DTW, Schrimpf D, Sill M et al (2018) Practical implementation of DNA methylation and copy-number-based CNS tumor diagnostics: the Heidelberg experience. Acta Neuropathol 136:181–210. https://doi.org/10.1007/s00401-018-1879-y
Ceccarelli M, Barthel FP, Malta TM, Sabedot TS, Salama SR, Murray BA et al (2016) Molecular Profiling Reveals Biologically Discrete Subsets and Pathways of Progression in Diffuse Glioma. Cell 164:550–563. https://doi.org/10.1016/j.cell.2015.12.028
Chinnaiyan P, Kensicki E, Bloom G, Prabhu A, Sarcar B, Kahali S et al (2012) The metabolomic signature of malignant glioma reflects accelerated anabolic metabolism. Cancer Res 72:5878–5888. https://doi.org/10.1158/0008-5472.CAN-12-1572-T
Dobin A, Davis CA, Schlesinger F, Drenkow J, Zaleski C, Jha S et al (2013) STAR: ultrafast universal RNA-seq aligner. Bioinformatics 29:15–21. https://doi.org/10.1093/bioinformatics/bts635
Evans AM, DeHaven CD, Barrett T, Mitchell M, Milgram E (2009) Integrated, nontargeted ultrahigh performance liquid chromatography/electrospray ionization tandem mass spectrometry platform for the identification and relative quantification of the small-molecule complement of biological systems. Anal Chem 81:6656–6667. https://doi.org/10.1021/ac901536h
Feber A, Guilhamon P, Lechner M, Fenton T, Wilson GA, Thirlwell C et al (2014) Using high-density DNA methylation arrays to profile copy number alterations. Genome Biol 15:R30. https://doi.org/10.1186/gb-2014-15-2-r30
de Hoon MJL, Imoto S, Nolan J, Miyano S (2004) Open source clustering software. Bioinformatics 20:1453–1454. https://doi.org/10.1093/bioinformatics/bth078
Kocakavuk E, Johnson KC, Sabedot TS, Reinhardt HC, Noushmehr H, Verhaak RGW (2023) Hemizygous CDKN2A deletion confers worse survival outcomes in IDHmut-noncodel gliomas. Neuro Oncol 25:1721–1723
Li H, Handsaker B, Wysoker A, Fennell T, Ruan J, Homer N et al (2009) The Sequence Alignment/Map format and SAMtools. Bioinformatics 25:2078–2079. https://doi.org/10.1093/bioinformatics/btp352
Liao Y, Smyth GK, Shi W (2019) The R package Rsubread is easier, faster, cheaper and better for alignment and quantification of RNA sequencing reads. Nucleic Acids Res 47:e47. https://doi.org/10.1093/nar/gkz114
Louis DN, Perry A, Wesseling P, Brat DJ, Cree IA, Figarella-Branger D et al (2021) The 2021 WHO Classification of Tumors of the Central Nervous System: a summary. Neuro Oncol 23:1231–1251. https://doi.org/10.1093/neuonc/noab106
Mah CK, Mesirov JP, Chavez L (2018) An accessible GenePattern notebook for the copy number variation analysis of Illumina Infinium DNA methylation arrays. F1000Research 7. doi: https://doi.org/10.12688/f1000research.16338.1
Malta TM, Sokolov A, Gentles AJ, Burzykowski T, Poisson L, Weinstein JN et al (2018) Machine Learning Identifies Stemness Features Associated with Oncogenic Dedifferentiation. Cell 173:338-354.e15. https://doi.org/10.1016/j.cell.2018.03.034
Müller F-J, Laurent LC, Kostka D, Ulitsky I, Williams R, Lu C et al (2008) Regulatory networks define phenotypic classes of human stem cell lines. Nature 455:401–405. https://doi.org/10.1038/nature07213
Nassiri F, Chakravarthy A, Feng S, Shen SY, Nejad R, Zuccato JA et al (2020) Detection and discrimination of intracranial tumors using plasma cell-free DNA methylomes. Nat Med 26:1044–1047. https://doi.org/10.1038/s41591-020-0932-2
Noushmehr H, Weisenberger DJ, Diefes K, Phillips HS, Pujara K, Berman BP et al (2010) Identification of a CpG island methylator phenotype that defines a distinct subgroup of glioma. Cancer Cell 17:510–522. https://doi.org/10.1016/j.ccr.2010.03.017
Prabhu AH, Kant S, Kesarwani P, Ahmed K, Forsyth P, Nakano I et al (2019) Integrative cross-platform analyses identify enhanced heterotrophy as a metabolic hallmark in glioblastoma. Neuro Oncol 21:337–347. https://doi.org/10.1093/neuonc/noy185
RC T (2013) R: A Language and Environment for Statistical Computing
Ritchie ME, Phipson B, Wu D, Hu Y, Law CW, Shi W et al (2015) limma powers differential expression analyses for RNA-sequencing and microarray studies. Nucleic Acids Res 43:e47. https://doi.org/10.1093/nar/gkv007
Robinson JT, Thorvaldsdóttir H, Winckler W, Guttman M, Lander ES, Getz G et al (2011) Integrative genomics viewer. Nat Biotechnol 29:24–26
Robinson MD, McCarthy DJ, Smyth GK (2010) edgeR: a Bioconductor package for differential expression analysis of digital gene expression data. Bioinformatics 26:139–140. https://doi.org/10.1093/bioinformatics/btp616
Shen SY, Singhania R, Fehringer G, Chakravarthy A, Roehrl MHA, Chadwick D et al (2018) Sensitive tumour detection and classification using plasma cell-free DNA methylomes. Nature 563:579–583. https://doi.org/10.1038/s41586-018-0703-0
Shirahata M, Ono T, Stichel D, Schrimpf D, Reuss DE, Sahm F et al (2018) Novel, improved grading system(s) for IDH-mutant astrocytic gliomas. Acta Neuropathol 136:153–166. https://doi.org/10.1007/s00401-018-1849-4
de Souza CF, Sabedot TS, Malta TM, Stetson L, Morozova O, Sokolov A et al (2018) A Distinct DNA Methylation Shift in a Subset of Glioma CpG Island Methylator Phenotypes during Tumor Recurrence. Cell Rep 23:637–651. https://doi.org/10.1016/j.celrep.2018.03.107
Teschendorff AE (2020) A comparison of epigenetic mitotic-like clocks for cancer risk prediction. Genome Med 12:56. https://doi.org/10.1186/s13073-020-00752-3
Turcan S, Rohle D, Goenka A, Walsh LA, Fang F, Yilmaz E et al (2012) IDH1 mutation is sufficient to establish the glioma hypermethylator phenotype. Nature 483:479–483. https://doi.org/10.1038/nature10866
Wang J, Cazzato E, Ladewig E, Frattini V, Rosenbloom DIS, Zairis S et al (2016) Clonal evolution of glioblastoma under therapy. Nat Genet 48:768–776. https://doi.org/10.1038/ng.3590
Yan H, Parsons DW, Jin G, McLendon R, Rasheed BA, Yuan W et al (2009) IDH1 and IDH2 mutations in gliomas. N Engl J Med 360:765–773. https://doi.org/10.1056/NEJMoa0808710
Yan X, Ma L, Yi D, Yoon J, Diercks A, Foltz G et al (2011) A CD133-related gene expression signature identifies an aggressive glioblastoma subtype with excessive mutations. Proc Natl Acad Sci U S A 108:1591–1596. https://doi.org/10.1073/pnas.1018696108
Yang Z, Wong A, Kuh D, Paul DS, Rakyan VK, Leslie RD et al (2016) Correlation of an epigenetic mitotic clock with cancer risk. Genome Biol 17:205. https://doi.org/10.1186/s13059-016-1064-3
Zhou W, Dinh HQ, Ramjan Z, Weisenberger DJ, Nicolet CM, Shen H et al (2018) DNA methylation loss in late-replicating domains is linked to mitotic cell division. Nat Genet 50:591–602. https://doi.org/10.1038/s41588-018-0073-4
Author information
Authors and Affiliations
Corresponding authors
Ethics declarations
Conflict of interest
The authors declare no potential conflicts of interest.
Additional information
Publisher's Note
Springer Nature remains neutral with regard to jurisdictional claims in published maps and institutional affiliations.
Supplementary Information
Below is the link to the electronic supplementary material.
Rights and permissions
Springer Nature or its licensor (e.g. a society or other partner) holds exclusive rights to this article under a publishing agreement with the author(s) or other rightsholder(s); author self-archiving of the accepted manuscript version of this article is solely governed by the terms of such publishing agreement and applicable law.
About this article
Cite this article
Nassiri, F., Ajisebutu, A., Patil, V. et al. Metabologenomic characterization uncovers a clinically aggressive IDH mutant glioma subtype. Acta Neuropathol 147, 68 (2024). https://doi.org/10.1007/s00401-024-02713-1
Received:
Revised:
Accepted:
Published:
DOI: https://doi.org/10.1007/s00401-024-02713-1