Abstract
The regional climate model RegCM 4.4 at 50 km resolution is used to conduct a sensitivity study over South Asia Coordinated Regional climate Downscaling Experiment domain during the period 1998–2002, in order to investigate the best cumulus convective precipitation scheme, planetary boundary layer (PBL) and land-surface scheme. The inferences obtained from 11 sensitivity experiments include the better performance of community land model version 4.5 (CLM 4.5) over biosphere–atmosphere transfer scheme, Tiedtke as cumulus convective precipitation scheme and University of Washington (UW) as PBL scheme. The simulation with these parameterization schemes well captures the monsoon precipitation pattern over India ~ 7 mm/day and North Eastern Region of India (NER) ~ 12 mm/day, which are comparable to observations with a significant correlation of R2 > 0.93. The observed temperatures are also well simulated by the model. Therefore, RegCM 4.4 with these parameterization schemes is further used to simulate the aerosol fields (aerosol optical depth, AOD and black carbon, BC) and aerosol direct radiative forcing (DRF) for the period 2011–2014 over the same domain with special emphasis on NER. The model captures the seasonality in AOD and BC over the Indian Subcontinent and NER. BC hotspots in the Indo-Gangetic Plain and China are well captured by the model. The observed to simulated BC ratio over Dibrugarh (located in NER) is found to be improved. The model underestimation is significant in the dry season when burning over the region is predominant, which has not been considered by the emission inventories properly. Simulated DRF is found to exhibit seasonality qualitatively as well as a North–South latitudinal gradient.
Similar content being viewed by others
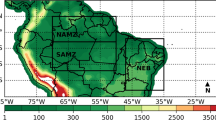
Avoid common mistakes on your manuscript.
1 Introduction
Due to the enhanced anthropogenic activities, the climate change study at varying spatial and temporal scales has become more complex during the last few decades. It is crucial to assess the magnitude of climate change in a finer scale i.e., regionally for impact studies and policy- making. Regional climate models (RCMs) that gained wide interest over the last few decades along with rapid growth in computational power is a potential way to downscale global climate model (GCM) information. Numerous studies have been conducted so far to analyze the improvement of global model results using RCMs (e.g., Dickinson et al. 1989; Giorgi and Marinucci 1991). In present days, RCMs are used globally for downscaling reanalysis and GCM data with various interests. These are one among the strong tools assisting climate change impacts assessment studies in regional to local scales. The World Climate Research Program (WCRP) took an initiative called Coordinated Regional climate Downscaling Experiment (CORDEX) (Giorgi et al. 2012 and references therein) with the intention to generate the regional climate change projections. International Centre for Theoretical Physics (ICTP), Trieste, Italy’s regional climate model was found to perform well in CORDEX protocols when studied over different domains across the world: Africa (Anyah and Semazzi 2007), Asia (Dash et al. 2006; Rahman et al. 2007a, b), Europe (Cossarini et al. 2008; Salon et al. 2008), Middle East (Almazroui 2012; Islam and Almazroui 2012; Artale et al. 2010) and USA (Giorgi et al. 2012; Chen et al. 2003). Earlier studies revealed that evaluation of RCM performance over atmospheric-ocean global climate model (AOGCM) (Giorgi and Mearns 1999) is unavoidable. To capture the climate sensitivity of a region using a climate model, it is essential to correctly incorporate the two-way feedback between meteorology and atmospheric chemistry. The meteorological simulation is vital as that can modify the aerosol properties (chemical, optical and radiative) in all spatial scales. Accurate precipitation, circulation patterns, humidity and temperature only can reproduce a reliable atmospheric chemistry over the simulation region. Hence it is inevitable to investigate whether the model simulated meteorology is adequate or not. As the model contains different cumulus convective precipitation, land surface and boundary layer schemes, the biases produced with reference data sets have to be evaluated.
Along with the development of RegCM from version 1.0 to the current version, several cumulative schemes were imported into the model (Giorgi et al. 2012). However, no cumulative schemes outperform others in terms of simulated climatology. The discrepancies arise with a change in cumulative schemes as they heavily depend on topography and climatic backgrounds. Hence, application of cumulus convective schemes requires several sensitivity tests. The regional simulation outputs are not only influenced by convective schemes but also by several other factors like topography (Gao et al. 2006; Lee et al. 2011), lateral boundary conditions (Oh et al. 2013; Park et al. 2013), planetary boundary layer (PBL) scheme (Guttler et al. 2014), land surface parameterizations (Zou and Xie 2012; Kang et al. 2014). The land surface scheme chosen has a substantial impact on climate model simulations (Wang et al. 2015; Ma et al. 2017). There are several advantages of CLM 4.5 over BATS as the former has more number of soil layers, vegetation fractions and uses subgrid “tiles” approach where separate water and energy balance conducted for each tile. This approach helps in simulating the surface parameters better than BATS scheme (Steiner et al. 2005). Even though PBL can directly influence near-surface temperature and precipitation, which are two mainly studied climate variables, climatological aspects of both observed and modeled PBL studies are not investigated as per need in the scientific community (Giorgi et al. 1993; Dethloff et al. 2001; Shin and Ha 2007; Esau and Zilitinkevich 2010; Guttler et al. 2014). PBL schemes in climate models are broadly divided into two types: as local (uses local characteristics of the PBL) and non-local type (uses global characteristics of the PBL to express turbulent fluxes) (Stensrud 2007). Hence, for a better simulation of climate variables, an optimized experimental design and better performing physical parameterization schemes are necessary. A number of studies were conducted over different regions of the globe in order to find the most suitable cumulus convective precipitation schemes. For example, according to the report by Liu and Ding (2007) Anthes–Kuo scheme performed better over China with a horizontal resolution of 50 km, whereas sensitivity studies over South Asia by Basit et al. (2012), Sinha et al. (2013), Rahman et al. (2007a, b) revealed better performance by different cumulus convective precipitation schemes. Moisture, heat and momentum exchange occur within the PBL due to the influence of turbulent eddies. Hence, the evolution of turbulent motion in climate models that is controlled by PBL parameterization schemes is vital. Earlier studies (e.g. Giorgi et al. 1993; Dethloff et al. 2001; Shin and Ha 2007; Esau and Zilitinkevich 2010) have shown the significance of investigating the PBL as precipitation and near-surface temperature, two widely climate studied parameters are governed by it. Guttler et al. (2014) have investigated the performance of two available PBL schemes in RegCM 4.2. Hence, the selection of the PBL scheme is a key factor in tuning the regional climate simulation into a realistic one. The land cover pattern represents the earth’s surface feature of the region. The rapid changes in the land use, especially because of the perturbations due to human deeds results in ecological imbalances. A better land surface parameterization can improve the model performance drastically. Inbuilt land surface scheme present in the RegCM is biosphere–atmosphere transfer scheme (Dickinson et al. 1993) which is present since the primary RegCM version. Availability of CLM 4.5 (Oleson and Lawrence 2013) as land surface scheme option is the recent advancement in RegCM model physics. CLM provides improvements compared with BATS in land–atmosphere exchanges in terms of moisture, energy and related surface climate feedbacks (Steiner et al. 2009).
Indian sub-continent has different microclimates within the landmass, due to the wide variations in geology and geography. The northern tip of India is the great Himalayas with an alpine climate, whereas the northwestern region has a hot subtropical climate, due to the presence of the Thar Desert. The southern part of India experiences the equatorial climate and semi-arid climate prevails almost throughout the peninsular region. The major portion of the annual precipitation over India is obtained from June to September due to the summer monsoon, popularly known as southwest monsoon. Northeasterly during October to December brings rainfall over the southeastern part of India which is known as winter monsoon or northeast monsoon, also has a small contribution to the annual rainfall. The interesting fact about the southwest monsoon is their regular occurrence during the prescribed time period every year. Year to year variation of southwest monsoon rainfall results in extreme weather events like drought and flood, thus affecting agriculture and water resources all over the country. Hence, due to this complex terrain conditions use of high-resolution RCMs for reproducing the climatic features and to simulate future projections is vital for climate change studies over the Indian region.
During the last decade, several regional climate modeling studies took place over the Indian subcontinent. Devanand et al. (2018) tested Indian summer monsoon rainfall sensitivity to combinations of four cumulus and two microphysics schemes using WRF. They have concluded that the simulated seasonal precipitation is more sensitive to cumulus parameterization schemes than microphysics parameterization. Regional climate model’s ability to represent Indian monsoon was investigated by Lucas-Picher et al. (2011) using four different RCMs and found that precipitation amount and spatial distribution differs substantially in each other. Significant sensitivity of irrigation process to monsoon circulation over Indian subcontinent was reported by Saeed et al. (2009) using Max Planck Institute Regional Model (REMO). Srinivas et al. (2013) evaluated the performance of WRF in simulating regional scale precipitation over seven different homogeneous rainfall zones over India and reported the improved model performance using Betts–Miller–Janjic as convective scheme. Rajendran et al. (2002) using the National Center for Atmospheric Research (NCAR) Community Climate Model, found that model intraseasonal and interannual monsoon variabilities heavily depends on cumulus scheme in the model. Studies using several regional climate models over South Asia have reported that summer monsoon rainfall response to the choice of cumulus convection scheme is highly sensitive (Ratnam and Kumar 2005; Rakesh et al. 2009; Kumar et al. 2012a, b). Ratnam and Kumar (2005) investigated the performance of Mesoscale Model (MM5) model in simulating summer monsoon using three cumulus convective schemes: Betts–Miller–Janjic, Kain–Fritsch and Grell. The model could simulate the large-scale features and the intraseasonal and interannual variabilities using all the three schemes, but the spatial and temporal distribution of the precipitation was found to differ substantially. The same study found that the Kain–Fritsch scheme overestimated the rainfall whereas Grell scheme underestimated the same during two contrasting monsoon years. Kumar et al. (2012a, b) showed that WRF model coupled with Chemistry (WRF-Chem) successfully simulated the general features of the meteorology over India like the seasonal cycle of temperature, water vapour, precipitation and seasonal changes in wind patterns. The study also reported that model overestimates the monsoon rainfall over India and underestimates during other seasons on the usage of Kain Fritsch as cumulus convection scheme. Mukhopadhyay et al. (2010) studied impacts of three different convective closures (Grell–Devenyi, the Betts–Miller–Janjic and Kain Fritsch) on systematic biases of an Indian monsoon precipitation climatology using WRF and found Betts–Miller–Janjic to simulate better the heating profile, seasonal cycle of evaporation and condensation. Ojha et al. (2016) examined the general features of the monsoon meteorology and dynamics using WRF-Chem using Grell as cumulus convective precipitation scheme and reported good agreement of the model with observation. RegCM4 model sensitivity to cumulus convection and land surface parameterization schemes over Indian region is discussed by Nayak et al. (2017). The study concludes that the combination of Grell cumulus convective precipitation scheme with BATS land surface scheme stood out as the best parameterization. Dash et al. (2011) examined the monthly and seasonal Indian summer monsoon using RegCM3 with 55 and 30 km resolutions. Comparisons showed that simulation with higher spatial resolution is in line with observations. In another study on the spatial and temporal variations in Indian summer monsoon rainfall and temperature for the period 1982–2009, Dash et al. (2013) have found that the annual characteristics of both rainfall and temperature in Central India were well in phase with observations. Dash et al. (2014a) projected the summer monsoon changes over the Indian sub-continent and adjoining regions for the twenty-first century under the RCP 4.5 and RCP 8.5 scenarios. The study showed the ability of RegCM4 to generate the less precipitated areas during the historical period simulation. Dash et al. (2014b) examined the effect of domain size on the Indian summer monsoon simulations and suggested to use the CORDEX South Asia domain than smaller Indian domain for model simulations. Das et al. (2015a) reported a positive feedback of the direct radiative effects of anthropogenic aerosols on Indian summer monsoon circulation using the RegCM 4.1. Again, Das et al. (2015b) investigated the sensitivity of dust radiative feedback to the dust absorption property and reported the strengthening of monsoon precipitation due to dust-induced large-scale convergence. Lodh (2015) using RegCM4 studied the impact of Caspian Sea drying on Indian monsoon precipitation and temperature. Halder et al. (2015) examined the land-use and land-cover change on rainfall events and temperature over the Indian region. Raju et al. (2015) conducted a sensitivity test of RegCM 4.3 for four different cumulus convective schemes and found mixed scheme (Emanuel over land and Grell over the ocean) to simulate the summer monsoon precipitation better. Umakanth et al. (2015) conducted a sensitivity of RegCM 4.4 to cumulus convective physics to simulate the seasonal mean and MISOs during the ISM over CORDEX South Asia domain for a 13-year continuous simulation. Tiwari et al. (2015) studied the influence of land surface schemes in climate prediction over the Western Himalaya, where they found CLM to simulate climatology better than BATS.
Earlier studies have reported that Eastern Himalayan foothills covering NER has dominance of various aerosol types with both local and heavy external influences (Pathak et al. 2016; Gogoi et al. 2017; Biswas et al. 2017). NER experiences unique topography with left corridor opened to the outflow of highly populated Indo-Gangetic Plain (IGP) and other three sides being surrounded by hills and mountain ranges. This makes favourable conditions for a complex aerosol environment over the region as the strong anthropogenic activities in IGP, forest fire events in the hills of the north-east adds on to the local anthropogenic emissions from brick kilns, gas fields, oil wells etc (Gogoi et al. 2017). The high contribution of carbonaceous aerosols emitted from these local sources are investigated and reported earlier in detail (Gogoi et al. 2007; Pathak et al. 2016). Gogoi et al. (2017) also reported that the accidental discharges due to blowout/fire, excess gas burn from abandoned oil wells are few other factors, which make high aerosol accumulation over NER. Moreover, the region experiences dense vegetation releasing a large amount of biological aerosols and is prone to very high rainfall, which makes NER a significant zone in aspects of aerosol studies. Thus, this region of South Asia is wide-open to the impacts of atmospheric aerosols and trace gases through climate change, regional air quality degradation and impact on human health. A large number of studies on aerosols in different aspects over the region have been carried out based on both ground-based and satellite observations (Pathak et al. 2010, 2016; Pathak and Bhuyan 2014; Subba et al. 2018 and references therein; Dahutia et al. 2018 etc.). However, modeling studies are still limited except for a location Dibrugarh (Nair et al. 2012; Kumar et al. 2015).
The main objectives of the present study are
-
1.
To study the RegCM model sensitivity to changes in cumulus convective precipitation, boundary layer and land surface schemes. Model’s skill to generate reliable spatial climatology, annual and seasonal cycles of meteorological variables are focused. This will facilitate to attain the second objective of chemistry simulations, with the best parameterization obtained over CORDEX South Asia domain.
-
2.
A comprehensive multiyear (2011–2014) simulation and validation exercise including the spatial and seasonal aerosol black carbon (BC) and aerosol optical depth (AOD) over the region using data collected from both ground-based networks under Aerosol Radiative Forcing Over India NETwork (ARFINET) of Indian Space Research Organisation’s Geosphere Biosphere Program (ISRO-GBP) and MODIS satellite measurements.
-
3.
Evaluation of direct radiative forcing of simulated aerosols over Indian region with emphasis on NER.
-
4.
To analyze the effect of enhancing aerosol emissions in the model simulated AOD and to evaluate its effect on precipitation.
2 Approach
2.1 Model description and experimental design for sensitivity tests
The fourth generation of the Abdus Salam International Centre for Theoretical Physics (ICTP) regional climate model (RegCM4) (Giorgi et al. 2012) has been used in this study. The RegCM’s dynamical core is based on the hydrostatic version of the mesoscale model MM5 of National Center for Atmospheric Research (NCAR) and Pennsylvania State University (Grell et al. 1994). Radiative transfer in RegCM is based on the parameterization of NCAR’s community climate model CCM3 (Kiehl et al. 1996). RegCM consists of the biosphere–atmosphere transfer scheme (BATS; Dickinson et al. 1993) with supplementary amendments to take account subgrid variability of topography and land cover using mosaic-type approach (Giorgi et al. 2003) and community land model (CLM) (Steiner et al. 2009) as land surface packages. We use BATS (Dickinson et al. 1993) and CLM version 4.5 (Oleson and Lawrence 2013) as land-surface scheme (LSS) and both the available PBL schemes of Holtslag et al. (1990) and that of the University of Washington (UW) (Grenier and Bretherton 2001; Bretherton et al. 2004; O’Brien et al. 2012) for the sensitivity analysis. RegCM 4.4 includes several options for cumulus convection: Grell (1993), the Massachusetts Institute of Technology (MIT) scheme (Emanuel 1991; Emanuel and Rothman 1999), Tiedtke scheme (Tiedtke 1989), Kain and Fritsch scheme (Kain and Fritsch 1993) and there is also possibility of using different convective schemes over ocean and continent in the same simulation which is referred to as “mixed convection”. The convection is triggered for Grell scheme when the lifted air parcel attains moist convection. The current study follows Fritsch–Chappell (FC) closure (Fritsch and Chappell 1980) for the Grell scheme where available buoyant energy is released within a specified period. In the Emanuel scheme where the mixing in clouds is highly inhomogeneous and episodic, convection triggers when the level of neutral buoyancy is higher than cloud-based level. Air lifts between these two levels and a fraction of the condensed moisture forms precipitation. Whereas the rest forms cloud that mix with the environment, of which the mixing entrainment and detrainment rate are proportional to vertical gradients of buoyancy in clouds. Tiedtke is a mass flux convection scheme in which the closure assumptions for shallow convection are maintained by the supply of moisture from surface evaporation. Whereas, penetrative and mid-level convection is determined by large-scale moist convergence. Kain and Fritsch scheme is a revised version of Fritsch–Chappell scheme (Fritsch and Chappell 1980) with modifications in detrainment effect and cloud model. The main components of Kain and Fritsch are a trigger function, moist convective updraft, moist convective downdraft, compensating circulation and a closure assumption. Convection triggers when Lifting Condensation Level (LCL) temperature of the ascending parcel exceeds the environmental LCL temperature. The closure assumption of Kain and Frisch scheme is the same as that of Fritsch–Chappell scheme (1980): to reduce convective available potential energy (CAPE) over a specific timescale. The initial conditions and lateral boundary conditions are provided by six hourly fields from ERA-Interim reanalyzes (EIN 75) through a 1000 km buffer zone (Solmon et al. 2015 and references therein). The model domain (Fig. 1) follows CORDEX specifications and covers a large region encompassing Indian sub-continent and adjacent oceanic regions with a 50 km horizontal resolution and 18 vertical sigma-pressure levels. All the integrations start at 00:00 hours UTC on 1 December 1997 and runs unceasingly for 5 years and 1 month until 1 January 2003. The December 1997 is excluded from analysis to allow for the model spin-up time. For obtaining the best parameterization schemes: cumulus convective precipitation, PBL and land surface, we have conducted 11 sensitivity simulations as explained in Table 1. Firstly, four simulations (TDK, GR, EM, KF) are conducted using all the four available cumulus convective precipitation schemes using UW PBL scheme and coupling RegCM with CLM4.5. Another simulation with Tiedtke over land and Emanuel over Ocean is also performed keeping same PBL and land surface scheme. In order to investigate the sensitivity in LSS, RegCM is coupled with BATS keeping rest of the parameters same. PBL scheme Holstag sensitivity under BATS as well as CLM4.5 is also investigated using Tiedtke cumulus convective precipitation scheme. Further, three additional sensitivity simulations (TDKH, TDKM, TDKL) are carried out to investigate the impact of different values like entrainment rate for penetrative convection, critical RH below cloud for evaporation and conversion coefficient from cloud water within the Tiedtke cumulus convective precipitation scheme (Table 2). Here, UW PBL scheme in RegCM coupled with CLM4.5. In the experiment TDKM, we have modified the values of all the three components from default values (experiment TDK in Table 1). In the rest of the experiments: TDKH and TDKL, only entrainment rates for penetrative convection are increased and decreased respectively from the default value. Four observational datasets are used to evaluate the model precipitation and temperature: the Climate Research Unit (CRU; Mitchell and Jones 2005), Tropical Rainfall Measuring Mission (TRMM; Huffman et al. 2007), Global Precipitation Climatology Project (GPCP; Adler et al. 2003) and Indian Meteorological Department (IMD) data set prepared by Rajeevan and Bhate (2009).
CORDEX South Asia domain with topography (m) used for model simulation. Black box shows the Indian region used for Annual cycle validation. Other boxes represent the sub regions—Tibetan Himalayas (TH), Indo-Gangetic Plains (IGP), North Eastern Region of India (NER), Main Land India (MI), North Bay of Bengal (NBOB), South Bay of Bengal (SBOB) and South India (SI) used for RegCM model validation
2.2 Experimental design for simulation of aerosol fields and validation against observations
2.2.1 RegCM4.4 simulations of AOD and BC
For better representation of regional anthropogenic emissions, we use the Regional Emission inventory in ASia (REAS) (Ohara et al. 2007; Nair et al. 2012; Solmon et al. 2015) completed by the Atmospheric Chemistry and Climate Model Inter-comparison Project (ACCMIP) emissions (Lamarque et al. 2010; Solmon et al. 2015). Monthly input boundary conditions for the aerosol run are provided from global simulations using Community Atmosphere Model (CAM) and EC-EARTH (ECWMF-based Earth-system model) following Representative Concentration Pathway (RCP) scenario 2.6. Parameterization schemes for cumulus convection, LSS and PBL are selected performing sensitivity test, which is discussed in the later part of the paper. Simulated aerosols impact on RegCM shortwave radiation scheme through pre-calculated optical properties (Solmon et al. 2006).
2.2.2 Observations: satellite and ground-based
For validations of simulated aerosol fields, the remote sensor Moderate resolution Imaging Spectroradiometer (MODIS) (Remer et al. 2005, 2008) retrieved monthly average Level-3, quality assured (QA) collection 6, 1° × 1° AOD data are utilized. MODIS Level-3 data are cloud screened and have been recommended for scientific investigation (Levy et al. 2013; Dahutia et al. 2018). The retrieved AOD uncertainty derived from MODIS is estimated to be ± (0.05 + 0.15AOD) over land (Remer et al. 2008; Li et al. 2009).
The Aerosol Radiative Forcing over India NETwork (ARFINET) is a large network of aerosol laboratories over South Asian Region covering entire Indian Subcontinent, maintained under the ISRO-GBP Programme. There are 35 ARFINET laboratories across the length and breadth of the country serving the purpose of generating a widespread aerosol database (Babu et al. 2013; Nair et al. 2016). Every ARFINET station either has an in-house built Multi-Wavelength Radiometer (MWR) (Moorthy et al. 1989), which is the major workhorse of ARFINET or handheld sun photometer (MICROTOPS II) for measurement of AOD, both working on filter wheel principle. The reliability between MWR and MICROTOPS II measurements has been established by Kompalli et al. (2010). The backbone of the BC surface measurements over the country are the multi-wavelength Aethalometers (Magee Scientific, USA) under ARFINET. The Aethalometer works on the principle of optical attenuation technique (Hansen et al. 1984). Several studies have already used this method and reported BC measurements over several locations within the ARFINET (Nair et al. 2007, 2008; Beegum et al. 2008; Pathak and Bhuyan 2014; Gogoi et al. 2017 etc. and references therein).
3 Results and discussion
3.1 Model precipitation, circulation climatology and validation
Precipitation is one of the most studied climate variables to evaluate model performance. The spatial distribution of RegCM simulated precipitation for different experiments for the four seasons during 1998–2002 is presented in Fig. 2 along with the TRMM precipitation combined with NCEP wind speed and direction. The results show that the RegCM is able to reproduce the seasonal circulation pattern over South Asia including the evolution of monsoonal pattern. Due to the intense surface heating and hence decrease in the atmospheric pressure of the landmass, a northward moist wind moves from equator to the South Asian region during JJA. During the SON-DJF seasons, the Indian subcontinent receives a winter precipitation. Scarce precipitation during DJF as observed from TRMM is also captured by the RegCM simulations for different experiments. A precipitation band with ~ 6–10 mm/day over the ocean region near to the Southern tip of India during DJF and MAM is well generated in most of the experiments. TDKM experiment generates a wet bias over NER during MAM. The Indian sub-continental maximum precipitation is obtained during the monsoon season. A strong precipitation band ~ 18–20 mm/day is observed near the Southwestern India and NER region. EM and GR experiments fail to capture the high precipitation over NE region during JJA. EM noticeably generates a high precipitation over the Bay of Bengal (BoB) region during that period (JJA). The model precipitation bias in percentage with TRMM dataset is presented in Fig. 3. During the DJF all the experiments show a very minimal bias less than 10% with the TRMM pattern over the Indian landmass. During MAM, the rainfall pattern of NER simulated by TDK, KF, TDKH and TDKL experiments goes well with the TRMM pattern while EM, GR, TDEM create a dry bias. TDKM different from all other schemes creates a wet bias over NER. KF, EM, TDKM, TDKH and TDKL create a wet bias over Southern India. GR, KF, TDKM experiments simulate a wet bias over Eastern India, whereas EM, TDEM, TDKH and TDKL inhibit this. TDK generates a marginal dry bias over the region. During monsoon, when dominant rainfall occurs throughout the Indian Subcontinent, cumulus convective schemes behave in dissimilar ways over different regions. All the convective schemes other than the GR overestimate precipitation over South India while other experiments create a dry bias. All the experiments create a dry bias over central India, comparatively, KF and TDKM simulate well. In EM and GR experiments, an intense dry bias covers NER. Over Eastern Himalayan regions, all experiments unanimously show a dry bias. Noticeably all the schemes except GR and EM generate a dry bias over the western Indian region. An underestimated peak monsoon precipitation during the mature phase in Indian region has also been reported by Giorgi et al. (2012). During post-monsoon season TDKH, TDK generated rainfall go well with the observations throughout the Indian mainland (MI) with only a minimal bias. GR produces a drier climatology during the SON all over India. KF and TDKM behavior is noble other than a bias over North India for the former and over IGP and NER for the later. TDK and TDEM even though well behave with observations over South India, has a wet bias over Central, North and NER. The performances of different schemes are further discussed based on Taylor’s diagram.
3.2 Temperature climatology and validation
Simulated seasonal air temperatures were validated against Climate Research Unit (CRU; Mitchell and Jones 2005) dataset with 0.5° × 0.5° resolution and is only available over continents. During DJF, the observed temperature is higher over South Indian region (> 24 °C), reduces gradually moving northwards and Tibetan Himalayas experience lowest temperature (< 12 °C). There is a warm bias of ~ 2–4 °C over the central Indian and a cold bias over the NER region for all schemes (Fig. 4). During JJA even though underestimation of temperature over northwestern India and NER exist, TDEM and TDK experiments comparatively perform well over other regions. GR experiment underestimates the temperature all over India during JJA. Whereas all other experiments exhibit a cold bias over South India and warm bias over north India. TDEM and TDK exhibit higher spatial warm bias over Central and North India than the rest of India. A cold systematic bias of a few degrees has been reported over the Indian region by Giorgi et al. (2012).
3.3 Taylor analysis
With the Taylor diagram shown in Fig. 5, the impact of cumulus convective schemes on precipitation and the modulations brought by changes in convective parameters over different regions of the Indian subcontinent and adjacent areas can be examined. Thus the better performing convective scheme over different regions can be found out. Taylor analysis has been carried out over Tibetan Himalayas, Indo-Gangetic Plain (IGP), Main Land India, North East India, Northern BoB, Southern BoB and South India. This analysis makes an implication that none of the precipitation schemes is finest over the across all sub-regions of the domain. TDKM simulation, even though shows a good correlation with observation, it overestimates for the precipitation over the Himalayan region. TDEM, TDKH and TDKL show better results comparatively. IGP is a hotspot for aerosols over South Asia, as the pollution rate is extremely high in that region (Gautam et al. 2009, 2010; Tripathi et al. 2005). Hence, precipitation simulation over this region has to be considered important as it plays a major role in the aerosol removal from the atmosphere. TDK simulation shows a very good correlation of > 0.96 also with less deviation from observation and also has a perfect annual cycle. Over the Indian mainland, all schemes generate rainfall other than GR goes well with the observation. NER is a rainfall hotspot region of the world and also unique with its topography. TDK and TDKM rainfall simulations show good agreements with the observations whereas GR and EM performances are poor. All the model simulations have a substantial discrepancy with the observations over the southern part of India with R < 0.75. The main reason for this is the less precision in simulating the southwest monsoon precipitation entering the subcontinent through South India. Over the North Bay of Bengal (BoB), all the experiments underestimate the precipitation, whereas over the south BoB Grell and KF experiments are found to perform well. Considering the temperature, model experiments exhibit less disagreement with the observations. GR shows a less standard deviation from observations over IGP, NER and North BoB.
Figure 5 further illustrates that simulation of regional temperature and precipitation varies highly based on the choice of cumulus convective parameterization schemes. The intense low-level westerlies during monsoon simulated using TDK scheme is in good agreement with the observed pattern as discussed in Fig. 2. The TDK scheme exhibits a realistic precipitation and temperature over a large part of the domain and is in good agreement with observations over most of the sub-regions. The observed phase of annual precipitation cycle is well simulated by TDK scheme over IGP, Mainland India and NER. Extensive sensitivity study of RegCM to cumulus convective precipitation schemes with reference to precipitation and temperature reveals that seasonal and monthly mean spatial distribution, as well as magnitude, are better simulated by TDK experiment compared to the other experiment. In Tiedtke scheme the shallow, mid-level and deep convection are represented by several cloud types and downdrafts. Performance of Tiedtke scheme is better due to its different closure assumptions for shallow and deep, mid-level convection (Bhatla et al. 2016). Even though there is a difference in spatial distribution between observation across the other subregions, the Tiedtke scheme does well capture the seasonality. It is worthwhile to mention that KF is also performing well spatially in all the regions except the NER. However, as NER is the focused region for the present chemistry simulation, we are not considering KF as the best parameterization scheme. Instead, Tiedtke which is performing much better over NER, IGP and MI is considered further for aerosol field simulations. It is worthwhile to mention that NER is affected by pollutants including aerosols transported from IGP and MI compared to rest of the regions mentioned above.
3.4 Annual precipitation cycle
The annual precipitation cycle for all RegCM experiments are compared with CRU, IMD, GPCP and TRMM (1998–2002) over India is shown in Fig. 6. The right onset time of monsoon is vital as it is the major contributor to the annual rainfall over Indian sub-continent. From the figure, it is clear that there is an early monsoon onset in most of the RegCM experiments (EM, KF, TDKM, TDKH, TDKL), whereas the GR experiment inhibits the precipitation, with a dry bias in JJA. There is a delayed monsoon evolution in the TDEM precipitation, thus possessing a high wet bias during the post-monsoon period. Whereas TDK experiment in spite of exhibiting a wet bias in the MAM months (~ 2 mm/day) over the observations, captures the right onset of monsoon and peak level precipitation over the entire Indian region. Thus, comparatively, better annual precipitation cycle is obtained using the TDK experiment. Bhatla et al. (2016) using RegCM 4.3 examined the sensitivity of cumulus convection scheme in the simulation of Pre-onset, Onset and Post-onset periods of Indian Summer Monsoon over CORDEX South Asia domain. The study reported that composite onset simulation is in good agreement with observation using Tiedtke scheme. Srivastava and Sherin (2018), investigated the role of physical and dynamical parameterizations on meteorology and aerosol characteristics over India using WRF-CHEM. The study revealed that the Grell 3D and Kain–Fritsch cumulus parameterization schemes are not able to capture the meteorological observations (rainfall, winds, and Relative Humidity) over the region. The experiment considering Grell–Freitas cumulus parameterization was found to be simulating meteorological parameters and BC well in comparison with observations. Rakesh et al. (2009) reported that the WRF model overestimates the summer monsoon rainfall over Indian region using Kain–Fritsch as cumulus scheme. It is evident that the same parameterization scheme behaves in different ways when implemented in different models as these strongly depend on the other physical parameterizations as well.
3.5 Regional model sensitivity to land surface schemes and PBL schemes
In this section, we examine the performances of PBL schemes over the study region (Figs. 7, 8). Since two different PBL schemes are implemented in RegCM 4.4: Diagnostic Holtslag scheme (Holtslag et al. 1990) and prognostic UW scheme (Grenier and Bretherton 2001), four different experiments using the best convection scheme, Tiedtke obtained from the above results coupling with BATS and CLM 4.5 respectively are performed. The two experiments using Holtslag scheme with BATS and CLM 4.5 are TDKBH and TDKCH and the other two using UW scheme are TDKBU and TDK respectively. The impact of PBL is very significant during the JJA as both the experiments TDKBH and TDKCH underestimate the precipitation all over India significantly (figure not shown). To finalize the better simulation from TDK, TDKBU, TDKBH and TDKCH the annual precipitation cycle over India are analyzed along with the observations as presented in Fig. 8.
BATS exhibits a significant overestimation of precipitation from January to May, with ~ 4–5 mm/day in April and May, whereas the CLM experiment phenomenally reduces this overestimation in MAM. The contradiction in BATS and CLM simulated precipitations can be due to the difference in the number of soil layers present in both the schemes. As there are soil layers with less depth in the BATS scheme, surface temperature reacts to the solar heating strongly and thus surface heat gets driven to the convective precipitation simulation. As CLM 4.5 includes several soil layers and it considers the soil depth up to few meters, the upper soil temperature doesn’t react as if in the BATS scheme, reducing the surface heat pumping and thus inhibits the precipitation. Thus the RegCM simulation using Tiedtke as cumulus convective precipitation scheme coupled with CLM 4.5 is the suitable combination than coupling with BATS. The annual cycles of precipitation for both the experiments using Holstag as PBL scheme underestimate precipitation significantly during June (~ 3 mm/day). The TDKBH experiment overestimates precipitation during September and October.
Both the Holstag experiments fail to generate the maximum JJA rainfall over the region, whereas TDK even though exhibits a minimal monthly bias follows the observational pattern well. Thus the TDK experiment in which Tiedtke is used as cumulus convective precipitation scheme coupled with CLM 4.5 using UW as PBL scheme comes out as the suitable combination over the domain. From spatial plot rainfall bias with TRMM (figure not shown), CLM is found to lessen the rainfall in comparison to BATS using UW PBL scheme, almost all over the Mainland by ~ 1 mm/day. During MAM and JJA, CLM inhibits around ~ 4 mm/day rainfall in NER. CLM creates wet bias over IGP and NER during SON with respect to BATS. The Correlation plot of monthly average CRU temperature with TDK and TDKBU experiment, (Fig. 7) is to investigate the model capability of generating near-surface temperature. CLM 4.5 coupled TDK simulation clearly shows a better correlation (0.98), whereas, TDKBU experiment even though has a good correlation (0.96), is not apposite since there is an overestimation of temperature throughout the simulation while compared with observations. Thus the TDK experiment with UW scheme is considered as the best combination for RegCM 4.4 coupled with CLM 4.5.
3.6 Simulation of aerosol fields
The aerosol fields: AOD and BC are simulated over the Indian subcontinent for the period 2011–2014 using RegCM 4.4 model coupled with CLM 4.5. In this simulation, we have considered Tiedtke cumulus convective parameterization and UW PBL Schemes, which are found to be the best schemes over the region as discussed above keeping all other parameters the same. Wet deposition is an important sink of BC that determines its lifetime and atmospheric burden (Bond et al. 2013). Appreciable differences in the absolute values of simulated precipitation with observations can significantly affect the wet scavenging of the aerosols (Srivastava and Sherin 2017). Hence, it is very important that the cumulus parameterization scheme is able to capture the variabilities in meteorological parameters over the study region. Similarly, the choice of PBL scheme generates high variability in atmospheric chemistry, diffusion, advection and deposition. Shallow wintertime atmospheric boundary layer is an important factor contributing to high surface aerosol concentrations in winter over South Asia by trapping pollutants near the surface. The nonlocal boundary layer scheme used in RegCM4 tends to overestimate vertical mixing in very stable conditions (Giorgi et al. 2012). RegCM4 with non-local boundary scheme failed to represent the very stable nighttime conditions and therefore overestimates the vertical aerosol transport and underestimates the nocturnal BC (Nair et al. 2012). Therefore, the sensitivity with the advanced RegCM 4.4 version helps in the selection of cumulus and PBL scheme that has to be chosen wisely for aerosol simulations over South Asia. Our analyses showed that use of Tiedtke and UW scheme as cumulus convective and PBL schemes respectively are closer to observed precipitation over India, especially over NER. Features like annual rainfall cycle over India, high monsoon rainfall over NER, perfect rainfall pattern over IGP are simulated by the combination of Tiedtke and UW scheme. This will aid in perfect wet scavenging/removal mechanism over BC hotspot regions like IGP, which even impacts the aerosol environment over NER.
We have validated the simulated AOD with MODIS (Moderated Resolution Imaging Spectroradiometer) satellite retrievals over the Indian subcontinent, while ground-based ARFINET AOD and BC concentrations are used to validate the simulations over the NER only.
3.6.1 RegCM simulated AOD and validation against MODIS retrieved AOD over India
Total extinction due to the existence of aerosols in the vertical column of atmosphere is determined by columnar spectral aerosol optical depth (AOD), which portrays the climate impact of aerosols. Hence in-depth understanding about the spatio-temporal variation of simulated AOD over Indian region is crucial. Studies for validating large-scale simulated aerosols are based on satellite observations due to their ability to provide aerosol information at regional to global scale with high temporal resolution. Figure 9 shows the spatio-temporal distribution of seasonal AOD simulated by RegCM at 350–640 nm (left column), MODIS AOD at ~ 550 nm (middle column) and the bias between MODIS and RegCM AOD (right column) over Indian Subcontinent. Spatio-temporal AOD variation is well depicted by the model thereby simulation of AOD hot spots like IGP during DJF associated with anthropogenic emission and dust activities, high AOD over the Arabian Sea during JJA, low AOD over the Indian Ocean etc. Simulated and Satellite retrieved AOD possess less discrepancy during the MAM, the bias being within ± 0.2. MODIS shows highest AOD values (~ 1.1) over northwestern India during monsoon. This is associated with enhanced dust activity over west Asian regions as reported earlier (Moorthy et al. 2005; Zhu et al. 2007). This strong north westerly advection of mineral dust is well represented by RegCM simulations, even though a small bias exists (Solmon et al. 2015). The extreme bias between the simulated and MODIS retrieved AOD exists over the IGP region during DJF, where RegCM underestimates MODIS by ~ 0.5. The primary reason for this might be the inadequacy of the emission inventories including the dominant burning events and other anthropogenic activities occurring over the region during DJF. This makes the DJF AOD underestimation high over regions where anthropogenic aerosol loading is higher than natural aerosols. Similar underestimation of AOD over Indian region during DJF was reported in the earlier studies (Nair et al. 2012; Reddy et al. 2004; Dickerson et al. 2002) revealing the inadequacy of emission inventories. Also as stated by Nair et al. (2012), imprecision of surface albedo representation in MODIS retrieval algorithm (Jethva et al. 2009) over Indian region adds up to the AOD disagreement.
3.6.2 RegCM simulated black carbon over the Indian subcontinent
Black carbon (BC), one of the most tentative components of aerosols system over South Asian region (Kinne et al. 2006; Koch et al. 2009) has strong radiative effects due to its high light absorbing nature. Many reports are available on spatio-temporal distribution of BC and its contribution to radiative forcing and thus to climate over the South Asia region (Pathak and Bhuyan 2014 and references therein; Subba et al. 2018 and references therein). However, due to inaccessibility to various places of the complex terrain of Indian Subcontinent, ground-based measurements are insufficient. Also, BC measurements by satellites are non-existent. As such, models are the best tool to obtain regular information on spatio-temporal variability of BC. A few model-based studies on BC including validation against observation over the Indian subcontinent are available (Goto et al. 2011; Nair et al. 2012; Kumar et al. 2015). Spatial and seasonal distribution of RegCM simulated BC reported in those studies are in agreement with the current simulations presented in Fig. 10. The model is capable of capturing the BC hotspots in the IGP region, particularly during the dry season, thus revealing its capability of replicating the real BC seasonal scenario. However, there exists a strong difference in magnitude between observation and simulation. According to Nair et al. (2012), absolute magnitudes of BC mass concentration were underestimated by RegCM by two to five times, with high underestimation in DJF. Model inability to replicate the diurnal variation of BC was found to be the foremost reason for this and is associated with the usage of non-local boundary layers in the model. Also the year average emission files lack the incorporation of local emission activities.
3.6.3 RegCM simulated AOD and BC validation using ARFINET measurements over NER
NER is a unique biodiversity region located at sub-Himalayan ranges surrounded by Tibetan plateau at North, Indo Myanmar hills at East, Garo–Khasi–Jayantia and Naga hills to the South and a left corridor opened to highly polluted IGP region and rest of Indian subcontinent. Even though the NER is a significant part of aerosol loading region of South Asia, no full-fledged aerosol modeling studies over this region have been conducted. In terms of aerosol burden, this region stands as second highest in South Asia next to IGP (Pathak et al. 2016). Hence, in the present study, Modeled AOD validation is carried out using ARFINET measured AOD over four distinct locations in NER [Agartala (AGA), Shillong (SHN), Imphal (IPH) and Dibrugarh (DBR)]. Pathak et al. (2016) have extensively studied the AOD distribution and ARF estimation over these locations and found a west-east gradient, with higher values in western locations. Figure 11 represents the spatial variation of AOD over different locations for ARFINET measured and simulated AOD during DJF and MAM. Both modeled and measured AOD are higher during MAM than in DJF at all the NER locations.
The observed AOD throughout all the locations ranges from 0.3 to 0.73 in DJF and 0.6 to 0.8 in MAM, whereas the simulated AOD lies between 0.21 and 0.31 and 0.34 to 0.47 respectively. Highest values for both observation (AOD ~ 0.8) and simulation (AOD ~ 0.47) are obtained at AGA, but with a high bias. Even though seasonality of AOD is well captured by the model, in terms of magnitude the simulation needs further improvement. Similar to observation by Pathak et al. (2016), the simulated AOD also exhibits east–west asymmetry with higher AOD over western NER locations, AGA and SHN than the eastern locations DBR and IPH. Overall, RegCM is capable of simulating the real Aerosol optical features over the Indian subcontinent. Still, the inconsistencies in magnitude existing between the simulated and observed AOD can mainly be assigned to the factors like, (1) emission inventories: as NER together with most of the Indian locations, is highly influenced by the local burning and anthropogenic activities whereas emission scenario is not completely capable of recreating the real scenario. It should also be kept in mind that emission files are yearly averaged. (2) As NER is the highest rain receiving region in India, replicating observed precipitation with high reliability using model simulation is highly challenging. Wet deposition is one of the main aerosol removal processes and surface wetness is a controlling factor of aerosol production. Along with rainfall, other meteorological parameters also can influence the aerosol simulation (e.g., wind, PBL). Hence, the model deficiency in simulating meteorology precisely can impose a bias in aerosol concentration and optical properties ultimately. Therefore, this inability to simulate same rainfall pattern and magnitude also adds to the model discrepancy.
Even though Nair et al. (2012) have carried out a detailed investigation of RegCM simulated BC concentration over Indian region by comparing with ARFINET observations, only one station, DBR from NER was included in that. Earlier studies over this region, based on the rich ground-based measurements under ARFINET (Pathak et al. 2016; Babu et al. 2013; Gogoi et al. 2017) revealed a distinct aerosol spatio-temporal variability. As such current simulations of BC are compared with the above mentioned four ARFINET stations in NER (Table 3). Measured BC concentration peaks during DJF with the highest value at AGA (5 µg/m3). The model simulations are found to be underestimated by the measurements at all the four stations throughout the year. Highest underestimation is observed during DJF over IPH than in other stations where the measured and simulated BC ratio is ~ 6.7. On the other hand, over DBR and SHN measured and simulated BC ratio throughout all the seasons lies below 3. During JJA and SON, the observed BC is closer to the observations. This systematic discrepancy is mainly due to the lack of precise emission inventory as mentioned above.
3.7 Evaluation of model-simulated direct radiative forcing
The direct radiative forcing (DRF), which is the means to study the climate implication of aerosols, represents the perturbed radiation flux due to the presence of aerosols, at top of the atmosphere (TOA), in the atmosphere (ATM) and at the surface (SUR). These are estimated with the change in net solar flux considering with and without aerosol conditions. Direct atmospheric forcing (DRFATM) is the difference between the radiative forcing at the top of the atmosphere and at the surface:
3.7.1 Direct radiative forcing over Indian sub-continent
Earlier studies have revealed the importance of studying DRF over the region as they contribute to the weakening of the Asian monsoon system (Lau et al. 2006 and references therein). The seasonal mean aerosol-induced DRFTOA is shown in Fig. 12. Over most of the Indian region aerosols induced DRFTOA cooling is exhibited in the range − 12 to 0 W/m2 throughout all the seasons. On contrary, over the Tibetan plateau and western Chinese region, a warming is induced due to the high surface albedo. During DJF the DRFTOA is less in the region, ranging from 0 to − 4 W/m2 over western India and − 4 to − 8 W/m2 over IGP, East and NER region. During MAM, the TOA cooling increases in the NER (− 8 to − 12 W/m2) compared to other regions. DRFTOA in JJA cooling increases over the west and North West India and adjacent Arabian Sea regions (< − 12 W/m2) compared to the remaining subcontinent. This is mainly due to the high dust loading over the West and North West India during the JJA. DRFTOA is almost 0 or less negative during SON over most part of India, that can be attributed to the increasing BC mass fraction (Pathak et al. 2010 and references therein). Slightly positive or negative TOA forcing over NER locations during post-monsoon and winter is reported before which was correlated to the increase in BC mass fraction (Pathak et al. 2010, 2016). During DJF, seasonal atmospheric forcing (DRFATM) over IGP, North India, East and NER ranges between 0 and 20 W/m2 whereas over the rest of the sub-continent it is < 10 W/m2 (Fig. 13). Atmospheric forcing increases throughout the Indian subcontinent during MAM, with highest over IGP. This may be assigned to the steady increase in simulated AOD during this season. DRFATM reaches as high as > 40 W/m2 over the North Western region during JJA when simulated AOD is also appreciable. The IGP region too experiences significant atmospheric forcing ranging from 40 to 50 W/m2. DRFATM during SON is comparatively less over all the aerosol hot spot regions. The North Indian regions experience high DRFTOA and DRFATM compared to South India throughout the year. This latitudinal gradient is mainly due to the high enhanced anthropogenic activities over North than South India. A detailed comparison of DRF estimated using Santa Barbara Discrete Ordinates Radiative Transfer (SBDART) model (Ricchiazzi et al. 1998) model over the South Asian region has been discussed by Subba et al. (2018) and hence not repeated here. They have reported, on an average, similar surface cooling over NE and South India (~ − 31 W/m2). The study also reported IGP and west India experiencing a surface dimming of ~ − 40 W/m2 annually and has higher atmospheric warming (~ + 32 W/m2) than NE and South India. This latitudinal gradient is captured by current simulation as discussed above.
3.7.2 Direct radiative forcing over NER
With the simulated AOD scenario over NER possessing consistency with the observations, here we try to estimate the radiative forcing due to simulated aerosols over the region. Several previous studies (Pathak et al. 2010, 2016; Gogoi et al. 2017; Biswas et al. 2017) have reported the DRF for composite aerosols and for BC only over the ARFINET stations in NER using SBDART model. The model simulated seasonal variation of DRF at TOA, SURF and in the ATM over AGA, SHN, IPH and DBR are shown in Fig. 14. All the stations experience less negative TOA forcing and surface forcing in DJF than in MAM mainly due to the high BC fraction in DJF than in MAM (Pathak et al. 2010, 2016). High atmospheric forcing over SHN and AGA during MAM (23 W/m2 and 21 W/m2) than in DJF (14 W/m2 and 16 W/m2) can be attributed to the higher AOD in MAM (0.47 and 0.44) than in DJF (0.3 and 0.31). The radiative forcing dependency on aerosol over DBR and other three locations has been reported earlier by Pathak et al. (2010, 2016) and the current study backs the same conclusion. Highest negative surface forcing in MAM is experienced over SHN (− 32 W/m2) and AGA (− 31 W/m2) than the eastern locations IPH (− 27 W/m2) and DBR (− 23 W/m2). Atmospheric forcing symbolizes the amount of radiative flux perturbed due to the existence of various aerosol species, that further results in atmospheric heating. Hence, the atmospheric heating rate is a significant sign of climate implication of aerosols, which can be calculated as (Liou 1980; Quijano et al. 2000)
where, g is the acceleration due to gravity, Cp the specific heat capacity of air at constant pressure and P the atmospheric pressure. A constant value of 300 hPa is depicted to ΔP in the equation as most of the aerosol-induced heating is confined to lower vertical levels. The atmosphere over AGA, SHN, IPH and DBR are found to be heated by 0.45–0.7 K/day, 0.40–0.69 K/day, 0.28–0.57 K/day and 0.25–0.64 K/day respectively throughout the year. The seasonality of heating rate follows the forcing in the atmosphere and thus simulated seasonal AOD. Hence due to the high atmospheric forcing values in MAM, all the locations experience a significant increase in MAM heating rate than the DJF. During MAM, heating rates of 0.65 K/day and 0.62 K/day are experienced in SHN and AGA, whereas < 0.52 K/day heating rates were noticeable over the other two locations. Even though the heating rates obtained over all the four locations are underestimated by the values reported by Pathak et al. (2016), spatio-temporal variation over the region is well captured by the model.
3.8 Model sensitivity to enhanced regional emissions and effect on monsoon precipitation
Interactive coupling of chemistry/aerosol in regional climate models is a relevant topic over Indian subcontinent as aerosols have significant effects on the South Asian monsoon (Lau et al. 2006). Several previous studies revealed significant underestimation of aerosols in emission inventories over Indian subcontinent in comparison with observations (Nair et al. 2012; Kumar et al. 2012b; Michael et al. 2014). The accurate parameterization of aerosol emissions would help to decrease the uncertainty in aerosol concentrations over the domain and will enhance our understanding of the effects of anthropogenic aerosols on the climate over CORDEX South Asia.
In the current experiment, anthropogenic emissions over the CORDEX South Asia domain have been increased by 100% (exp_DBL) and are utilized to study the effect of enhancement in regional emissions. The results are compared with the standard experiment (exp_STD) as discussed in Sect. 3.6 and MODIS retrieved AOD over different sub-regions: Tibetan Himalayas (TH), Indo-Gangetic Plains (IGP), North East (NE), Main Land India (MI), North Bay of Bengal (NBOB), South Bay of Bengal (SBOB) and South India (SI) (Table 4). The influence of enhancement in aerosol emission leads to an appreciable increase in AOD over entire Indian sub-continent and adjacent oceanic region with an increase of 38–97% seasonally over various sub-regions. Despite the underestimation in comparison with MODIS AOD, significant improvement in model performance in simulating AOD is exhibited by exp_DBL than in exp_STD over all sub-regions during DJF and MAM. During DJF, AOD over SI and SBOB during MAM is improved by 97% and 86% respectively. Noteworthy influence of enhanced emission is experienced over IGP during JJA and SON with an increase of AOD by 55% and 77% respectively. On the other hand, doubling emissions overestimate AOD over NER by 48% and 68% during JJA and SON. Simulated AOD in exp_DBL is also high over ML, NBOB, SBOB, and SI than MODIS retrieved AOD during SON. Overall, the results show that as simulated AOD is very sensitive to aerosol emissions, updating in emission inventory over the region will further improve model credibility. Though exp_DBL experiment reduced the underestimation, still the model underestimates the AOD over hot spots regions like IGP, revealing that emission inventories miss few of the significant local sources over the domain. Also, the AOD bias with observation is not the same over all the subregions during all the seasons. Hence, the exp_DBL simulated AOD overestimates the observed AOD from MODIS during SON over many sub-regions.
Figure 15 shows the change in JJA precipitation due to the fast climatic response because of doubling the aerosol emission with respect to the standard experiment. The model precipitation response to the increase in emission is highly sensitive. Doubling the emission increases the summer monsoon precipitation over northwest India and SBOB whereas a reduction is observed over west India, SI and portion of IGP. Hence, the precipitation variation over Indian sub-continent is mainly due to the enhancement of aerosol emissions inside the domain. Weakening of Asian summer monsoon due to enhancing anthropogenic aerosols is already reported by several studies (Ramanathan et al. 2005; Bollasina et al. 2011; Ganguly et al. 2012). Thus, it is essential to improve the emission inventories due to their potential to trigger a change in monsoon precipitation. Simulating the aerosol emission scenario and its feedback on dynamics precisely will improve the RegCM model performance over South Asia.
The present study can be a reference for fine tuning the RegCM4.4-CLM 4.5 coupled model for studying regional aerosol environment. Also, RegCM is one of the finest tools for assessing aerosol feedback to climate change. Up to a certain grid-spacing performance of a regional climate model gets better with the increase in the horizontal resolution (Wang et al. 2007). Even though Maurya et al. (2018) shown that RegCM performs better for Indian Summer Monsoon simulation when intermediate resolutions are used as compared to high and low-resolution experiments, the choice of resolution for the simulation of aerosols using RegCM is not yet studied. Hence, more sensitivity studies on the impact of horizontal resolution in aerosol studies over CORDEX framework will be performed in a future perspective that will provide valuable information for long-term studies further.
4 Conclusions
Sensitivity tests for 5 years 1998–2002, to fine tune the RegCM 4.4 model for chemistry simulation over the CORDEX South Asia with a specific thrust on the Indian sub-continent has been performed. Simulated temperature and precipitation using different precipitation schemes at 50 km resolution over the Indian sub-continent are compared with several observational datasets. Tiedtke cumulous parameterization scheme with default values was found as the best one over the region with PBL scheme of UW when coupled with CLM 4.5 land surface model. Chemistry simulation with the obtained best parameterization during 2011–2014 has been performed and a spatio-temporal distribution of aerosol optical and radiative properties is presented over the Indian subcontinent in general, with emphasis on NER in particular. Model sensitivity to enhanced aerosol emissions and the summer monsoon precipitation response to it are also looked into. Conclusions revealed by the study are summarized below.
-
The best agreement with observational data over entire India is obtained considering the Tiedtke as cumulous convective precipitation scheme. The right onset of monsoon and the right temporal peak of precipitation considering annual cycle over India is only observed in that experiment.
-
CLM 4.5 outperforms the BATS simulated precipitation, hence can be considered as the primary option for the land surface scheme over the domain with better representation of precipitation and temperature. The UW scheme performs well than the Holstag scheme in the domain, as it simulates well during the peak monsoon months whereas Holstag experiments show a high dry bias.
-
The RegCM with Tiedtke as cumulus convective precipitation scheme, UW scheme as PBL scheme coupled with CLM 4.5 as the land surface scheme is better performing for the simulations over CORDEX South Asia domain and is used further multiyear (2011–2014) aerosol field simulations.
-
RegCM captures the AOD and BC seasonality and spatial variability well over the Indian region, while it is underestimated magnitude wise, especially over anthropogenically active regions. Aerosol-induced atmospheric radiative forcing is highest over the western and northwestern part of India, associated with high aerosol loading during JJA.
-
Simulated TOA and ATM forcing over India are higher over northwestern India and IGP during JJA due to the high dust loading and dominant anthropogenic activities respectively. SHN and AGA experience high ATM forcing during MAM (23 W/m2 and 21 W/m2) than in DJF (14 W/m2 and 16 W/m2) and is associated with high AOD in MAM.
-
Maximum heating rates of 0.65 K/day and 0.62 K/day are experienced in SHN and AGA during MAM. Model is successful in simulating the seasonality in AOD, radiative forcing and heating rates over the four locations in NER.
-
Enhanced aerosol emissions helped in reducing model underestimation of AOD over hot spots regions like IGP. Hence, improving the aerosol emission over the Indian region is necessary for robust aerosol field simulation.
-
Model simulated precipitation shows high sensitivity to aerosol emissions. A reduction in summer monsoon precipitation is observed over west India, SI and portion of IGP due to increased aerosol emissions.
References
Adler RF, Huffman GJ, Chang A, Ferraro R, Xie P, Janowiak J, Rudolf B, Schneider U, Curtis S, Bolvin D, Gruber A, Susskind J, Arkin P, Nelkin E (2003) The version-2 global precipitation climatology project (GPCP) monthly precipitation analysis (1979–present). J Hydrometeorol 4:1147–1167. https://doi.org/10.1175/15257541(2003)004%3C1147:TVGPCP%3E2.0.CO;2
Almazroui M (2012) Dynamical downscaling of rainfall and temperature over the Arabian Peninsula using RegCM4. Clim Res 52:49–62. https://doi.org/10.3354/cr01073
Anyah RO, Semazzi FHM (2007) Variability of East African rainfall based on multiyear RegCM3 simulations. Int J Climatol 27:357–371. https://doi.org/10.1002/joc.1401
Artale V, Calmanti S, Carillo A, Dell’Aquila A, Hersmann M, Pisacane G, Ruti PM, Sannino G, Struglia MV, Giorgi F, Bi X, Pal JS, Rauscher S (2010) An atmosphere-ocean regional climate model for the Mediterranean area: assessment of a present climate simulation. Clim Dyn 35:721–740. https://doi.org/10.1007/s00382-009-0691-8
Babu SS, Manoj MR, Moorthy KK, Gogoi MM, Nair VS, Kompalli SK, Satheesh SK, Niranjan K, Ramagopal K, Bhuyan PK, Singh D (2013) Trends in aerosol optical depth over Indian region: potential causes and impact indicators. J Geophys Res 118:11794–11806. https://doi.org/10.1002/2013JD020507
Basit A, Shoaib SR, Irfan N, Avila R (2012) Simulation of monsoon precipitation over South-Asia using RegCM3. ISRN Meteorol 12:754902. https://doi.org/10.5402/2012/754902
Beegum SN, Moorthy KK, Nair VS, Babu SS, Satheesh SK, Vinoj V, Reddy RR, Gopal KR, Badarinath KVS, Niranjan K, Pandey SK, Behera M, Jeyaram A, Bhuyan PK, Gogoi MM, Singh S, Pant P, Dumka UC, Kant Y, Kuniyal JC, Singh D (2008) Characteristics of spectral aerosol optical depths over India during ICARB. J Earth Syst Sci 117(S1):303–313. https://doi.org/10.1007/s12040-008-0033-y
Bhatla R, Ghosh S, Mandal B, Mall RK, Sharma K (2016) Simulation of Indian summer monsoon onset with different parameterization convection schemes of RegCM-4.3. Atmos Res 176–177:10–18. https://doi.org/10.1016/j.atmosres.2016.02.010
Biswas J, Pathak B, Patadia F, Bhuyan PK, Gogoi MM, Babu SS (2017) Satellite-retrieved direct radiative forcing of aerosols over North-East India and adjoining areas: climatology and impact assessment. Int J Climatol 37:4756. https://doi.org/10.1002/joc.5325
Bollasina MA, Ming Y, Ramaswamy V (2011) Anthropogenic aerosols and the weakening of the south Asian summer monsoon. Science 334:502–505. https://doi.org/10.1126/science.1204994
Bond TC, Doherty SJ, Fahey DW, Forster PM, Berntsen T, DeAngelo BJ, Flanner MG, Ghan S, Kärcher B, Koch D, Kinne S, Kondo Y, Quinn PK, Sarofim S, Schultz M, Venkataraman C, Zhang H, Zhang S, Bellouin N, Guttikunda S, Hopke PK, Jacobson MZ, Kaiser JW, Klimont Z, Lohmann U, Schwarz JP, Shindell D, Storelvmo T, Warren SG, Zender S (2013) Bounding the role of black carbon in the climate system: a scientific assessment. J Geophys Res Atmos 118:1–173. https://doi.org/10.1002/jgrd.50171
Bretherton CS, McCaa JR, Grenier H (2004) A new parameterization for shallow cumulus convection and its application to marine subtropical cloud-topped boundary layers. I. Description and 1D results. Mon Weather Rev 132:864–882. https://doi.org/10.1175/1520-0493(2004)132%3C0864:ANPFSC%3E2.0.CO;2
Chen M, Pollard D, Barron EJ (2003) Comparison of future climate change over North America simulated by two regional models. J Geophys Res Atmos 108:4348. https://doi.org/10.1029/2002JD002738
Cossarini G, Salon S, Libralato S, Gao XJ, Giorgi F, Solidoro C (2008) A downscaling experiment for the lagoon of Venice. Part II: testing potential impacts of changes in precipitation patterns on biogeochemical properties. Clim Res 38:43–59. https://doi.org/10.3354/cr00758
Dahutia P, Pathak B, Bhuyan PK (2018) Aerosols characteristics, trends and their climatic implications over northeast India and adjoining South Asia. Int J Climatol 38:1234–1256. https://doi.org/10.1002/joc.5240
Das S, Dey S, Dash S (2015a) Impacts of aerosols on dynamics of India summer monsoon using a regional climate model. Clim Dyn 44(5–6):1685–1697
Das S, Dey S, Dash S, Diuliani G, Solmon F (2015b) Dust aerosol feedback on the Indian summer monsoon: sensitivity to absorption property. J Geophys Res Atmos 120(18):9642–9652
Dash SK, Shekhar MS, Singh GP (2006) Simulation of Indian summer monsoon circulation and rainfall using RegCM3. Theor Appl Climatol 86:161–172. https://doi.org/10.1007/s00704-006-0204-1
Dash SK, Rai S, Mohanty UC, Panda SK (2011) Monthly and seasonal Indian summer monsoon simulated by RegCM3 at high resolutions. In: Attri S, Rathore L, Sivakumar M, Dash S (eds) Challenges and opportunities in agrometeorology. Springer, Berlin. https://doi.org/10.1007/978-3-642-19360-6_2
Dash SK, Mamgain A, Pattnayak KC, Giorgi F (2013) Spatial and temporal variations in Indian summer monsoon rainfall and temperature: an analysis based on RegCM3 simulations. Pure Appl Geophys 170:1–20. https://doi.org/10.1007/s00024-012-0567-4
Dash SK, Mishra SK, Pattnayak KC, Mamgain A, Mariotti L, Coppola E, Giorgi F, Giuliani G (2014a) Projected seasonal mean summer monsoon over India and adjoining regions for the twenty-first century. Theor Appl Climatol 122:581–593. https://doi.org/10.1007/s00704-014-1310-0
Dash SK, Pattnayak KC, Panda SK, Vaddi D, Mamgain A (2014b) Impact of domain size on the simulation of Indian summer monsoon in RegCM4 using mixed convection scheme and driven by HadGEM2. Clim Dyn 44:961. https://doi.org/10.1007/s00382-014-2420-1
Dethloff K, Abegg C, Rinke A, Hebestadt I, Romanov VF (2001) Sensitivity of Arctic climate simulations to different boundary-layer parameterizations in a regional climate model. Tellus 53:1–26. https://doi.org/10.1034/j.1600-0870.2001.01073.x
Devanand A, Ghosh S, Paul S, Karmakar S, Niyogi D (2018) Multi-ensemble regional simulation of Indian monsoon during contrasting rainfall years: role of convective schemes and nested domain. Clim Dyn 50:4127–4147. https://doi.org/10.1007/s00382-017-3864-x
Dickerson RR, Andreae MO, Campos T, Mayol-Bracero OL, Neusuess C, Streets DG (2002) Analysis of black carbon and carbon monoxide observed over the Indian Ocean: implications for emissions and photochemistry. J Geophys Res 107(D19):8017. https://doi.org/10.1029/2001JD000501
Dickinson RE, Errico RM, Giorgi F, Bates GT (1989) A regional climate model for the western United States. Clim Change 15:383–422. https://doi.org/10.1007/BF00240465
Dickinson RE, Sellers HA, Kennedy PJ (1993) Biosphere atmosphere transfer scheme (BATS) version 1E as coupled to the NCAR community climate model. Technical note NCAR/TN-3871 STR, p 72. https://doi.org/10.5065/D67W6959
Emanuel KA (1991) A scheme for representing cumulus convection in large-scale models. J Atmos Sci 48:2313–2335. https://doi.org/10.1175/1520-0469(1991)048%3C2313:ASFRCC%3E2.0,CO;2
Emanuel KA, Rothman MZ (1999) Development and evaluation of a convection scheme for use in climate models. J Atmos Sci 56:1766–1782. https://doi.org/10.1175/1520-0469(1991)056%3C1766:DAEOAC%3E2.0.CO;2
Esau I, Zilitinkevich S (2010) On the role of the planetary boundary layer depth in climate system. Adv Sci Res 4:63–69. https://doi.org/10.5194/asr-4-63-2010
Fritsch JM, Chappell CF (1980) Numerical prediction of convectively driven mesoscale pressure systems. Part I: convective parameterization. J Atmos Sci 37:1722–1733
Ganguly D, Rasch PJ, Wang H, Yoon JH (2012) Climate response of the south Asian monsoon system to anthropogenic aerosols. J Geophys Res Atmos. https://doi.org/10.1029/2012JD017508
Gao X, Xu Y, Zhao Z, Pal JS, Giorgi F (2006) On the role of resolution and topography in the simulation of East Asia precipitation. Theor Appl Climatol 86:173–185. https://doi.org/10.1007/s00704-005-0214-4
Gautam R, Liu Z, Singh RP, Hsu NC (2009) Two contrasting dust-dominant periods over India observed from MODIS and CALIPSO data. Geophys Res Lett 36:L06813. https://doi.org/10.1029/2008GL036967
Gautam R, Hsu NC, Lau KM (2010) Premonsoon aerosol characterization and radiative effects over the Indo-Gangetic Plains: implications for regional climate warming. J Geophys Res 115:D17208. https://doi.org/10.1029/2010JD013819
Giorgi F, Marinucci MR (1991) Validation of a regional atmospheric model over Europe: sensitivity of wintertime and summertime simulations to selected physics parameterization and lower boundary conditions. Q J R Meteorol Soc 117:1171–1206. https://doi.org/10.1002/qj.49711750204
Giorgi F, Mearns LO (1999) Introduction to special section: regional climate modeling revisited. J Geophys Res 104:6335–6352. https://doi.org/10.1029/98JD02072
Giorgi F, Mearns LO (2003) Probability of regional climate change based on the reliability ensemble averaging (REA) method. Geophys Res Lett 30:1629. https://doi.org/10.1029/2003GL017130
Giorgi F, Marinucci MR, Bates GT, Canio GD (1993) Development of a second-generation regional climate model (RegCM2). Part II: convective processes and assimilation of lateral boundary conditions. Mon Weather Rev 121:2814–2832. https://doi.org/10.1175/1520-0493(1993)121%3C2814:DOASGR%3E2.0.CO;2
Giorgi F, Coppola E, Solmon F, Mariotti L, Sylla MB, Bi X, Elguindi N, Diro GY, Nair V, Giuliani G, Turuncoglu UU, Cozzini S, Guttler I, O’Brien TA, Tawfik AB, Shalaby A, Zakey AS, Steiner F, Stordal F, Sloan LC, Brankovic C (2012) RegCM4: model description and preliminary tests over multiple CORDEX domains. Clim Res 52:7–29. https://doi.org/10.3354/cr01018
Gogoi MM, Bhuyan PK, Moorthy KK (2007) Possible impact of a major oil-well fire on aerosol optical depth at Dibrugarh. Curr Sci 92(8):1047–1049
Gogoi MM, Babu SS, Moorthy KK, Bhuyan PK, Pathak B, Subba T, Chutia L, Kundu SS, Bharali C, Borgohain A, Guha A, De BK, Singh B, Chin M (2017) Radiative effects of absorbing aerosols over northeastern India: observations and model simulations. J Geophys Res 122:1132–1157. https://doi.org/10.1002/2016JD025592
Goto D, Takemura T, Nakajima T, Badarinath KVS (2011) Global aerosol model-derived black carbon concentration and single scattering albedo over Indian region and its comparison with ground observations. Atmos Environ 45:3277–3285. https://doi.org/10.1016/j.atmosenv.2011.03.037
Grell G (1993) Prognostic evaluation of assumptions used by cumulus parameterizations. Mon Weather Rev 121:764–787
Grell GA, Dudhia J, Stauffer DR (1994) A description of the fifth generation Penn State/NCAR mesoscale model (MM5). NCAR Technical note NCAR/TN-380 + STR
Grenier H, Bretherton CS (2001) A moist PBL parameterization for large-scale models and its application to subtropical cloud-topped marine boundary layers. Mon Weather Rev 129:357–377. https://doi.org/10.1175/1520-0493(2001)129%3C0357:AMPPFL%3E2.0.CO;2
Guttler I, Brankovic C, O’Brien TA, Coppola E, Grisogono B, Giorgi F (2014) Sensitivity of the regional climate model RegCM4.2 to planetary boundary layer parameterization. Clim Dyn 43:1753–1772. https://doi.org/10.1007/s00382-013-2003-6
Halder S, Dirmeyer P, Saha K (2015) Sensitivity of the mean and variability of indian summer monsoon to land surface schemes in RegCM4: understanding coupled land-atmosphere feedbacks. J Geophys Res 120:9437–9458. https://doi.org/10.1002/2015JD023101
Hansen ADA, Rosen H, Novakov T (1984) The Aethalometer—An instrument for the real-time measurement of optical absorption by aerosol particles. Sci Total Environ 36:191–196
Holtslag AAM, Brujin EIFD, Pan HL (1990) A high-resolution air mass transformation model for short-range weather forecasting. Mon Weather Rev 118:1561–1575. https://doi.org/10.1175/1520-0493(1990)118%3C1561:AHRAMT%3E2.0.CO;2
Huffman GJ, Adler RF, Bolvin DT, Gu G, Nelkin EJ, Bowman KP, Hong Y, Stocker EF, Wolff DB (2007) The TRMM multi-satellite precipitation analysis: quasi-global, multi-year, combined-sensor precipitation estimates at fine scale. J Hydrometeorol 8:38–55. https://doi.org/10.1175/JHM560.1
Islam MN, Almazroui M (2012) Direct effects and feedback of desert dust on the climate of the Arabian Peninsula during the wet season: a regional climate model study. Clim Dyn 39:2239–2250. https://doi.org/10.1007/s00382-012-1293-4
Jethva H, Satheesh SK, Srinivasan J, Moorthy KK (2009) How good is the assumption about visible surface reflectance in MODIS aerosol retrieval over land? A comparison with aircraft measurements over an urban site in India. IEEE Trans Geosci Remote Sens 47:1990–1998. https://doi.org/10.1109/TGRS.2008.2010221
Kain JS, Fritsch JM (1993) Convective parameterization for mesoscale models: the kain-fritsch scheme. In: Emanuel KA, Raymond J (eds) The representation of cumulus convection in numerical models. Meteorological Monographs. American Meteorological Society, Boston, MA. https://doi.org/10.1007/978-1-935704-13-3_16
Kang S, Im ES, Ahn JB (2014) The impact of two land-surface schemes on the characteristics of summer precipitation over East Asia from the RegCM4 simulations. Int J Climatol 34: 3986–3997. https://doi.org/10.1002/joc.3998
Kiehl JT, Hack JJ, Bonan GB, Boville BA, Breigleb BP, Williamson D, Rasch P (1996) Description of the NCAR community climate model (CCM3). NCAR Technical note NCAR/TN-420 + STR, p 152
Kinne S et al (2006) An AeroCom initial assessment—optical properties in aerosol component modules of global models. Atmos Chem Phys 6:1815–1834. https://doi.org/10.5194/acp-6-1815-2006
Koch D et al (2009) Evaluation of black carbon estimations in global aerosol models. Atmos Chem Phys 9:9001–9026. https://doi.org/10.5194/acp-99001-2009
Kompalli SK, Babu SS, Moorthy KK (2010) Inter-comparison of aerosol optical depth from the multi-wavelength solar radiometer with other radiometric measurements. Indian J Radio Space Phys 39:364–371. http://nopr.niscair.res.in/handle/123456789/10930
Kumar R, Naja M, Pfister GG, Barth MC, Brasseur GP (2012a) Simulations over South Asia using the Weather Research and Forecasting model with Chemistry (WRF-Chem): set-up and meteorological evaluation. Geosci Model Dev 5:321–343. https://doi.org/10.5194/gmd-5-321-2012
Kumar R, Naja M, Pfister GG, Barth MC, Wiedinmyer C, Brasseur GP (2012b) Simulations over South Asia using the Weather Research and Forecasting model with Chemistry (WRFChem): chemistry evaluation and initial results. Geosci Model Dev 5:619–648. https://doi.org/10.5194/gmd-5-619-2012
Kumar R, Barth MC, Pfister GG, Nair VS, Ghude SD, Ojha N (2015) What controls the seasonal cycle of black carbon aerosols in India? J Geophys Res Atmos 120:7788–7812. https://doi.org/10.1002/2015JD023298
Lamarque JF, Bond TC, Eyring V, Granier C, Heil A, Klimont Z, Lee D, Liousse C, Mieville A, Owen B, Schultz MG, Shindell D, Smith SJ, Stehfest E, Van Aardenne J, Cooper OR, Kainuma M, Mahowald N, McConnell JR, Naik V, Riahi K, van Vuuren DP (2010) Historical (1850–2000) gridded anthropogenic and biomass burning emissions of reactive gases and aerosols: methodology and application. Atmos Chem Phys 10:7017–7039. https://doi.org/10.5194/acp-10-7017-2010
Lau KM, Kim MK, Kim KM (2006) Asian summer monsoon anomalies induced by aerosol direct forcing: the role of the Tibetan Plateau. Clim Dyn 26:855–864. https://doi.org/10.1007/s00382-006-0114-z
Lee SW, Lee DK, Chang DE (2011) Impact of horizontal resolution and cumulus parameterization scheme on the simulation of heavy rainfall events over the Korean Peninsula. Adv Atmos Sci 28:1–15
Levy RC, Mattoo S, Munchak LA, Remer LA, Sayer AM, Patadia F, Hsu NC (2013) The collection 6 MODIS aerosol products over land and ocean. Atmos Meas Tech 6:989–3034. https://doi.org/10.5194/amt-6-2989-2013
Li Z, Zhao X, Kahn R, Mishchenko M, Remer L, Lee KH, Wang M, Laszlo I, Nakajima T, Maring H (2009) Uncertainties in satellite remote sensing of aerosols and impact on monitoring its long-term trend: a review and perspective. Ann Geophys 27:2755–2770. https://doi.org/10.5194/angeo-27-2755-2009
Liou KN (1980) An introduction to atmospheric radiation. Harcourt Brace Jovanovich, New York, p 392
Liu Y, Ding Y (2007) Sensitivity study of the South China Sea summer monsoon in 1998 to different cumulus parameterization schemes. Adv Atmos Sci 24:360–376. https://doi.org/10.1007/s00376-007-0360
Lodh A (2015) Impact of Caspian Sea drying on Indian monsoon precipitation and temperature as simulated by RegCM4 model. Hydrol Curr Res 6:217. https://doi.org/10.4172/2157-7587.1000217
Lucas-Picher P, Christensen JH, Saeed F, Kumar P, Asharaf S, Ahrens B, Wiltshire AJ, Jacob D, Hagemann S (2011) Can regional climate models represent the Indian monsoon? J Hydrometeorol 12:849–868. https://doi.org/10.1175/2011JHM1327.1
Ma Y, Zeng XM, Zhang Y (2017) Impact of the choice of land surface scheme on a simulated heatwave event: the case of Sichuan–Chongqing area, China. Adv Meteorol. https://doi.org/10.1155/2017/9545896
Maurya RKS, Sinha P, Mohanty PR, Mohanty UC (2018) RegCM4 model sensitivity to horizontal resolution and domain size in simulating the Indian summer monsoon. Atmos Res 210:15–33. https://doi.org/10.1016/j.atmosres.2018.04.010
Michael M, Yadav A, Tripathi SN, Kanawade VP, Gaur A, Sadavarte P, Venkataraman C (2014) Simulation of trace gases and aerosols over the Indian domain: evaluation of the WRF-Chem model. Geosci Model Dev 7:431–482. https://doi.org/10.5194/gmdd-7-431-2014
Mitchell TD, Jones PD (2005) An improved method of constructing a database of monthly climate observations and associated high-resolution grids. Int J Climatol 25:693–712. https://doi.org/10.1002/joc.1181
Moorthy K, Nair K, Prabha R, Murthy BVK (1989) Multiwavelength solar radiometer network and features of aerosol spectral optical depth at Trivandrum. IJRSP 18:194–201
Moorthy KK, Sunilkumar SV, Pillai PS, Parameswaran K, Nair PR, Ahmed YN, Ramgopal K, Narasimhulu K, Reddy RR, Vinoj V, Satheesh SK, Niranjan K, Rao BM, Brahmanandam PS, Saha A, Badarinath KVS, Kiranchand TR, Latha KM (2005) Wintertime spatial characteristics of boundary layer aerosols over peninsular India. J Geophys Res 110:D08207. https://doi.org/10.1029/2004JD005520
Mukhopadhyay P, Taraphdar S, Goswami BN, Krishnakumar K (2010) Indian summer monsoon precipitation climatology in a high-resolution regional climate model: impacts of convective parameterization on systematic biases. Weather Forecast 25:369–387. https://doi.org/10.1175/2009WAF2222320.1
Nair VS et al (2007) Winter time aerosol characteristics over the IndoGangetic Plain (IGP): impacts of local boundary layer processes and long-range transport. J Geophys Res 112:D13205. https://doi.org/10.1029/2006JD008099
Nair VS, Babu SS, Moorthy KK (2008) Spatial distribution and spectral characteristics of aerosol single scattering albedo over the Bay of Bengal inferred from shipborne measurements. Geophys Res Lett 35:L10806. https://doi.org/10.1029/2008GL033687
Nair VS, Solmon F, Giorgi F, Mariotti L, Babu SS, Moorthy KK (2012) Simulation of South Asian aerosols for regional climate studies. J Geophys Res 117:D04209. https://doi.org/10.1029/2011JD016711
Nair VS, Babu S, Manoj MR, Moorthy KK, Chin M (2016) Direct radiative effects of aerosols over South Asia from observations and modeling. Clim Dyn 47:1–18. https://doi.org/10.1007/s00382-016-3384-0
Nayak S, Mandal M, Maity S (2017) Customization of regional climate model (RegCM4) over Indian region. Theor Appl Climatol 127:153–168. https://doi.org/10.1007/s00704-015-1630-8
O’Brien TA, Chuang PY, Sloan LC, Faloona IC, Rossiter DL (2012) Coupling a new turbulence parametrization to RegCM adds realistic stratocumulus clouds. Geosci Mod Dev 5:989–1008. https://doi.org/10.5194/gmd-5-989-2012
Oh SG, Suh MS, Cha DH (2013) Impact of lateral boundary conditions on precipitation and temperature extremes over South Korea in the CORDEX regional climate simulation using RegCM4. Asia Pac J Atmos Sci 49:497–509. https://doi.org/10.1007/s0013143-013-0044-8
Ohara T, Akimoto H, Kurokawa J, Horii N, Yamaji K, Yan X, Hayasaka T (2007) An Asian emission inventory of anthropogenic emission sources for the period 1980–2020. Atmos Chem Phys 7:4419–4444. https://doi.org/10.5194/acp-7-4419-2007
Ojha N, Pozzer A, Rauthe-Sch¨och A, Baker AK, Yoon J, Brenninkmeijer CAM, Lelieveld J (2016) Ozone and carbon monoxide over India during the summer monsoon: regional emissions and transport. Atmos Chem Phys 16:3013–3032. https://doi.org/10.5194/acp-16-3013-2016
Oleson K, Lawrence DM (2013) Technical description of version 4.5 of the community land model (CLM). NCAR Technical note NCAR/TN-503 + STR, National Center for Atmospheric Research, Boulder, CO. https://doi.org/10.5065/D6RR1W7M
Park JH, Oh SG, Suh MS (2013) Impacts of boundary conditions on the precipitation simulation of RegCM4 in the CORDEX East Asian domain. J Geophys Res 118:1652–1667. https://doi.org/10.1002/jgrd.50159
Pathak B, Bhuyan PK (2014) Absorbing and scattering properties of boundary layer aerosols over Dibrugarh, Northeast India. Int J Remote Sens 35:5527–5543. https://doi.org/10.1080/01431161.2014.926424
Pathak B, Kalita G, Bhuyan K, Bhuyan PK, Moorthy KK (2010) Aerosol temporal characteristics and the resulting impact on radiative forcing at a location in the northeast India. J Geophys Res 115:D19204. https://doi.org/10.1029/2009JD013462
Pathak B, Subba T, Dahutia P, Bhuyan PK, Moorthy KK, Gogoi MM, Babu SS, Chutia L, Ajay P, Biswas J, Bharali C, Borgohain A, Dhar P, Guha A, De BK, Banik T, Chakraborty M, Kundu SS, Sudhakar S, Singh SB (2016) Aerosol characteristics in north-east India using ARFINET spectral optical depth measurements. Atmos Environ 125:461–473. https://doi.org/10.1016/j.atmosenv.2015.07.038
Quijano AL, Sokolik IN, Toon OB (2000) Radiative heating rates and direct radiative forcing by mineral dust in a cloudy atmospheric conditions. J Geophys Res 105:207–219. https://doi.org/10.1029/2000JD900047
Rahman MM, Islam MN, Ahmed AU, Afroz R (2007a) Comparison of RegCM3 simulated meteorological parameters in Bangladesh: part I-preliminary result for rainfall. Sri Lankan J Phys 8:1–9. https://doi.org/10.4038/sljp.v8i0.208
Rahman MM, Islam MN, Ahmed AU, Afroz R (2007b) Comparison of RegCM3 simulated meteorological parameters in Bangladesh: part II-preliminary result for temperature. Sri Lankan J Phys 8:11–19. https://doi.org/10.4038/sljp.v8i0.209
Rajeevan M, Bhate J (2009) A high resolution daily gridded rainfall dataset (1971–2005) for mesoscale meteorological studies. Curr Sci 96(4):558–562
Rajendran K, Nanjundiah RS, Srinivasan J (2002) Comparison of seasonal and intraseasonal variation of tropical climate in NCAR CCM2 GCM with two different cumulus schemes. Meteorol Atmos Phys 79:57–86
Raju PVS, Bhatla R, Almazroui M, Assiri M (2015) Performance of convection schemes on the simulation of summer monsoon features over the CORDEX South Asia domain using RegCM-4.3. Int J Climatol 35:4695–4706. https://doi.org/10.1002/joc.4317
Rakesh V, Singh R, Joshi PC (2009) Intercomparison of the performance of MM5/WRF with and without satellite data assimilation in short-range forecast applications over the Indian region. Meteorol Atmos Phys 105:133–155. https://doi.org/10.1007/s00703-009-0038-3
Ramanathan V, Chung C, Kim D, Bettge T, Buja L, Kiehl JT, Washington WM, Fu Q, Sikka DR, Wild M (2005) Atmospheric brown clouds: impacts on South Asian climate and hydrological cycle. Proc Natl Acad Sci USA 102:5326–5333
Ratnam VJ, Kumar KK (2005) Sensitivity of the simulated monsoon of 1987 and 1988 to convective parameterization schemes in MM5. J Clim 18:2724–2743
Reddy MS, Boucher O, Venkataraman C, Verma S, Le’on J-F, Bellouin N, Pham M (2004) General circulation model estimates of aerosol transport and radiative forcing during the Indian Ocean experiment. J Geophys Res 109:D16205. https://doi.org/10.1029/2004JD004557
Remer LA, Kaufman YJ, Tanré D, Mattoo S, Chu DA, Martins JV, Li RR, Ichoku C, Levy RC, Kleidman RG, Eck TF, Vermote E, Holben BN (2005) The MODIS aerosol algorithm, products and validation. J Atmos Sci 62:947–973. https://doi.org/10.1029/2001GL013204
Remer LA, Kleidman RG, Levy RC, Kaufman YJ, Tanré D, Mattoo S, Martins JV, Ichoku C, Koren I, Yu H, Holben B (2008) Global aerosol climatology from the MODIS satellite sensors. J Geophys Res 113:D14S07. https://doi.org/10.1029/2007JD009661
Ricchiazzi P, Yang S, Gautier C, Sowle D (1998) SBDART: a research and teaching software tool for plane-parallel radiative transfer in the Earth’s atmosphere. Bull Am Meteorol Soc 79:2101–2114. https://doi.org/10.1175/1520-0477(1998)079%3C2101:SARATS%3E2.0.CO;2
Saeed F, Hagemann S, Jacob D (2009) Impact of irrigation on the South Asian summer monsoon. Geophys Res Lett 36:1–7. https://doi.org/10.1029/2009GL040625
Salon S, Cossarini G, Libralato S, Gao XJ, Solidoro C, Giorgi F (2008) A downscaling experiment for the lagoon of Venice. Part I: validation of the present day precipitation climatology. Clim Res 38:31–41. https://doi.org/10.3354/cr00757
Shin SH, Ha KJ (2007) Effects of spatial and temporal variations in PBL depth on a GCM. J Clim 20:4717–4732. https://doi.org/10.1175/JCLI4274.1
Sinha P, Mohanty UC, Kar SC, Dash SK, Kumari S (2013) Sensitivity of the GCM driven summer monsoon simulations to cumulus parameterization schemes in nested RegCM3. Theor Appl Climatol 112:285–306. https://doi.org/10.1007/s00704-012-0728-5
Solmon F, Giorgi F, Liousse C (2006) Aerosol modeling for regional climate studies: application to anthropogenic particles and evaluation over a European/African domain. Tellus Ser B 58:51 – 72. https://doi.org/10.1111/j.1600-0889.2005.00155.x
Solmon F, Nair VS, Mallet M (2015) Increasing Arabian dust activity and the Indian summer monsoon. Atmos Chem Phys 15:8051–8064. https://doi.org/10.5194/acp-15-8051-2015
Srinivas CV, Hariprasad D, Bhaskar Rao DV, Anjaneyulu Y, Baskaran R, Venkatraman B (2013) Simulation of the Indian summer monsoon regional climate using advanced research WRF model. Int J Climatol 33:1195–1210. https://doi.org/10.1002/joc.3505
Srivastava R, Sherin SH (2017) Spatio-temporal variations of black carbon and optical properties in a regional climate model. Int J Climatol 37:1432–1443. https://doi.org/10.1002/joc.4787
Srivastava R, Sherin SH (2018) Impact of dynamical and microphysical schemes on black carbon prediction in a regional climate model over India. Environ Sci Pollut Res 25:14844. https://doi.org/10.1007/s11356-018-1607-0
Steiner AL, Pal JS, Giorgi F, Dickinson RE, Chameides WL (2005) The coupling of the Common Land Model (CLM0) to a regional climate model. Theor Appl Climatol 82:225–243. https://doi.org/10.1007/s00704-005-0132-5
Steiner AL, Pal JS, Rausscher SA, Bell JL, Diffenbaugh NS, Boone A, Sloan LC, Giorgi F (2009) Land surface coupling in regional climate simulations of the West Africa monsoon. Clim Dyn 33:869–892. https://doi.org/10.1007/s00382-009-0543-6
Stensrud D (2007) Parameterization schemes: keys to understanding numerical weather prediction models. Cambridge University Press, Cambridge, p 459
Subba T, Gogoi MM, Pathak B, Ajay P, Bhuyan PK, Solmon F (2018) Assessment of 1D and 3D model simulated radiation flux based on surface measurements and estimation of aerosol forcing and their climatological aspects. Atmos Res 204:110–127. https://doi.org/10.1016/j.atmosres.2018.01.012
Tiedtke M (1989) A comprehensive mass-flux scheme for cumulus parameterization in large-scale models. Mon Weather Rev 117:1779–1800. https://doi.org/10.1175/1520-0493(1989)117%3C1779:ACMFSF%3E2.0.CO;2
Tiwari PR, Kar SC, Mohanty UC, Dey S, Sinha P, Raju PVS, Shekhar MS (2015) The role of land surface schemes in the regional climate model (RegCM) for seasonal scale simulations over Western Himalaya. Atmósfera 28:129–142. https://doi.org/10.1016/S0187-6236(15)30005-9
Tripathi SN, Dey S, Tare V, Satheesh SK, Lal S, Venkataramani S (2005) Enhanced layer of black carbon in a north Indian industrial city. Geophys Res Lett 32:L12802. https://doi.org/10.1029/2005GL022564
Umakanth U, Kesarkar AP, Raju A, Rao S (2015) Representation of monsoon intraseasonal oscillations in regional climate model: sensitivity to convective physics. Clim Dyn. https://doi.org/10.1007/s00382-015-2878-5
Wang Y, Zhou L, Hamilton K (2007) Effect of convective entrainment/detrainment on the simulation of the tropical precipitation diurnal cycle. Mon Weather Rev 135:567–585. https://doi.org/10.1175/MWR3308.1
Wang X, Yang M, Pang G (2015) Influences of two land-surface schemes on RegCM4 precipitation simulations over the Tibetan Plateau. Adv Meteorol. https://doi.org/10.1155/2015/106891
Zhu A, Ramanathan V, Li F, Kim D (2007) Dust plumes over the Pacific, Indian, and Atlantic oceans: climatology and radiative impact. J Geophys Res 112:D16208. https://doi.org/10.1029/2007JD008427
Zou J, Xie Z (2012) The effects of the land-surface process parameterization of the RegCM4 on climate simulation in East Asia. Acta Meteor Sinica 70(6):1312–1326 (in Chinese)
Acknowledgements
The authors are thankful to the RegCM, ERA-Interim, TRMM, CRU, IMD, GPCP and MODIS science team. The ground based data are obtained from four ARFINET stations maintained by ISRO under its Geosphere-Biosphere Programme, in North-East India. BP is a Junior Associate in the ICTP, Trieste and thankful to ICTP for providing access to their computational system. AP is grateful to ISRO-GBP ARFI for providing him fellowship to undertake the work. This work is partly supported by the UGC SAP DRS II programme. PKB is an Emeritus Professor under UGC. Authors are sincerely grateful to the anonymous referees for their valuable suggestions in improving the manuscript.
Author information
Authors and Affiliations
Corresponding author
Additional information
Publisher’s Note
Springer Nature remains neutral with regard to jurisdictional claims in published maps and institutional affiliations.
Rights and permissions
About this article
Cite this article
Ajay, P., Pathak, B., Solmon, F. et al. Obtaining best parameterization scheme of RegCM 4.4 for aerosols and chemistry simulations over the CORDEX South Asia. Clim Dyn 53, 329–352 (2019). https://doi.org/10.1007/s00382-018-4587-3
Received:
Accepted:
Published:
Issue Date:
DOI: https://doi.org/10.1007/s00382-018-4587-3