Abstract
Circulation patterns associated with cold events over East Asia during the winter months of 1948–2014 are classified into four regimes by applying a k-means clustering method based on the area-weighted pattern correlation. The earliest precursor signals for two regimes are anticyclonic anomalies, which evolve into Ural and central Siberian blocking-like circulation patterns. The earliest precursor signals for the other two regimes are cyclonic anomalies, both of which evolve to amplify the East Asian trough (EAT). Both the blocking-like circulation patterns and amplified EAT favor the initialization of cold events. On average, the blocking-related regimes tend to last longer. The lead time of the earliest precursor signal for the central Siberian blocking-related regime is only 4 days, while those for the other regimes range from 16 to 18 days. The North Atlantic Oscillation plays essential roles both in triggering the precursor for the Ural blocking-related regime and in amplifying the precursors for all regimes. All regimes preferentially occur during the positive phase of the Eurasian teleconnection pattern and the negative phase of the El Niño–Southern Oscillation. For three regimes, surface cooling is primarily due to reduced downward infrared radiation and enhanced cold advection. For the remaining regime, which is associated with the southernmost cooling center, sensible and latent heat release and horizontal cold advection dominate the East Asian cooling.
Similar content being viewed by others
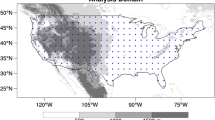
Avoid common mistakes on your manuscript.
1 Introduction
Extreme cold events are one of the most frequent climate disasters that occur over East Asia in boreal winter (Ding and Krishnamurti 1987; Zhang et al. 1997; Chen et al. 2004). These cold events often lead to sharp declines in surface air temperature and may have significant widespread snow accumulations. Either of these can have destructive impacts on agriculture, transportation, and other social or economic activities. Therefore, exploring the mechanisms behind the extreme cold events and identifying effective precursor signals are of high importance.
The possible mechanisms for cold events over East Asia and corresponding precursor signals have been explored in some previous studies. Analysis of 16 severe East Asian cold events that occurred during the winters of 1965–1975 revealed that the Rossby wave train propagating across the Eurasian continent was the major cause (Joung and Hitchman 1982). Further, the onset of this wave train could be traced back 6–7 days to geopotential height (HGT) anomalies over the North Atlantic Ocean. Zhang et al. (1997) pointed out that, prior to the onset of cold events, a cyclonic anomaly in the Rossby wave train, originating from the west of the East Asian trough (EAT), moved eastward and gradually developed into a major trough that replaced the EAT. They also showed that the lifetimes of East Asian cold events during 1979–1995 varied from 5 to 14 days, with an average lifetime of about 7 days (around 9 days for severe events). By studying 357 wintertime cold events over East Asia during the time period 1958–2000, Jeong et al. (2006) found that cold events were induced by large-scale negative potential vorticity (PV) anomalies in the lower stratosphere over northern Eurasia. These PV anomalies could be observed roughly 12 days prior to the outbreak of the cold events. Park et al. (2014) identified 332 cold events during the 52 winters from 1954 to 2005 and classified these events into two types: wave-train type events and blocking type events, both of which were associated with middle-tropospheric anticyclonic anomalies around the Ural Mountains. They further found that the blocking-type cold events tended to be more intense and had longer lifetimes than the wave-train type ones. In addition, the precursor signals for the wave-train and blocking type events could be observed in the lower stratosphere about 2 weeks and 1 week prior to the outbreak of the cold events, respectively. As reported by Wei et al. (2011) and Nath et al. (2016), these precursor signals in the lower stratosphere could propagate downward via planetary wave activity, and subsequently affect the middle and lower-level circulation, and the surface temperature.
Large-scale circulation modes such as the North Atlantic Oscillation (NAO), the Eurasian (EU) teleconnection pattern, and the El Niño–Southern Oscillation (ENSO), are crucial to the establishment and subsequent development of the wintertime cold events over East Asia. Hurrell (1995) demonstrated that the NAO is positively correlated with the wintertime surface temperature over East Asia. Luo et al. (2016a, b) investigated the relationship between the circulation pattern over the North Atlantic Ocean and the Ural blocking centered at 60°E (Diao et al. 2006). They revealed that Ural blocking, which usually leads to cold events over East Asia, mainly occurs during the positive phase of the NAO (NAO\(^{+}\)), especially during the decay stage of the NAO\(^{+}\) events. As the NAO\(^{+}\) events decay, the Ural blocking is excited due to the energy dispersion of Rossby waves (Luo et al. 2007). The EU teleconnection pattern is negatively correlated with the East Asian temperature (Wallace and Gutzler 1981; Gong et al. 2001; Sung et al. 2009). During the positive phase of the EU (EU\(^{+}\)), there are three centers of activity over the Eurasian continent: a cyclonic anomaly over western Europe, an anticyclonic anomaly over Siberia, and a cyclonic anomaly over Japan. Sung et al. (2009) found out that three days after the appearance of the EU\(^{+}\)-related circulation pattern, a remarkable enhancement can be observed in the northerly winds over the coastal areas of East Asia, which lead to a sharp decrease in the surface temperature. In addition, ENSO is positively correlated with East Asian temperature (Zhang et al. 1997; Chen et al. 2000). Wang et al. (2000) linked the East Asian wintertime conditions to ENSO through the Pacific–East Asian teleconnection pattern in the lower troposphere. During the negative phase of ENSO (ENSO\(^{-}\)), the Pacific–East Asian teleconnection pattern generates a cyclonic anomaly over the western North Pacific Ocean, which strengthens the northerly coastal winds (upstream of the cyclonic anomaly), thereby increasing the severity of the cold events.
Based on these previous studies, it can be concluded that the different types of cold events may be associated with diverse generation mechanisms with different precursor signals, and that the large-scale climate modes play important roles in modulating cold events over East Asia. However, there are several issues arising from these differences that remain unresolved. First, although the anticyclonic anomaly over the Ural Mountains is critical to East Asian wintertime climate, the anticyclonic anomaly over central Siberia (Lee and Jhun 2006; Huang et al. 2017a) and the cyclonic anomaly over the Eurasian continent (Joung and Hitchman 1982; Zhang et al. 1997) also appear to play important roles. Whether the mechanisms and precursor signals for the cold events associated with these anticyclonic or cyclonic anomalies are the same or not is an important unanswered question. Second, whether the favorable large-scale circulation modes (i.e., NAO, EU, and ENSO) for the different types of cold events are the same or not is also unclear. Third, the cold events over East Asia have often been attributed to cold advection from high latitudes (Lau and Li 1984; Takaya and Nakamura 2005a; Park et al. 2011; Woo et al. 2012; Nath et al. 2014). However, as pointed by Yao et al. (2017), radiative cooling plays a more important role than cold advection in the wintertime surface cooling over the Eurasian continent. Therefore, when analyzing the surface cooling in the different types of East Asian cold events, it is essential to compare the relative contributions of advective and radiative cooling as well as the contributions from any other physical processes.
To address the above issues, the aims of this study are threefold: (1) to obtain an accurate classification of cold events over East Asia and identify the earliest precursor signal and the associated lead time for each type of cold event, (2) to elucidate the impacts of the large-scale circulation modes (i.e. NAO, EU, and ENSO) on the generation and evolution of each type of cold event, (3) to diagnose the contributions of different physical processes to the surface cooling during each type of cold event. This paper is organized as follows: Sect. 2 describes the data and methods used for estimating climate indices, calculating the wave activity flux, identifying cold events over East Asia, and classifying these cold events into different regimes. Section 3 presents a brief introduction to cold events, a sensitivity analysis of the clustering method, and the circulation patterns and the corresponding surface temperature anomalies under different regimes. Section 4 analyzes the time evolutions of the circulation and surface temperature anomalies of cold events belonging to the different regimes. Section 5 discusses the relationships between the different regimes and the large-scale circulation modes. Section 6 discusses the contributions of different physical processes to the surface temperature variations for cold events under different regimes. Section 7 summarizes the major findings of this study.
2 Data and methods
2.1 Data
The data used in this study is from the National Centers for Environmental Prediction and the National Center for Atmospheric Research (NCEP–NCAR) reanalysis (Kalnay et al. 1996) for the period from 1948 to 2015. Variables used include the daily HGT and horizontal winds on the 500 hPa pressure surface, air temperature and horizontal winds on the 850 hPa pressure surface, near-surface air temperature at 2 m height, surface downward long wave radiation flux, surface latent and sensible heat fluxes, and the monthly mean HGT on the 500 hPa pressure surface. This study focuses on the cold events during the winter months. Because Huang et al. (2016a) demonstrated that March is an important month for studying the East Asian winter monsoon and therefore indispensable to the study of the cold events over East Asia, we define winter to include December, January, February, and March (DJFM). Note that the winter of a specific year refers to December of that year and January, February, and March of the following year. The analysis period runs from December of 1948 to March of 2015 and includes 67 winters with a total of 8123 days.
2.2 Climate indices and wave activity flux
Several indices are used to track the variations of the large-scale circulation modes. The EAT index is defined as the area-averaged HGT on the 500 hPa surface over the region of 110–145°E, 25–45°N (Wang and He 2012). After Barnston and Livezey (1987), the daily NAO index is calculated as follows. First, the monthly and daily HGT anomalies at 500 hPa are obtained by removing their respective climatological annual cycle during the period 1950–2000. Second, the loading pattern of the NAO is obtained as the leading mode of the monthly HGT anomalies at 500 hPa north of 20°N during 1950–2000 based on Rotated Empirical Orthogonal Function (REOF) analysis. Note that the base period 1950–2000 is used to keep the loading pattern consistent with that of the NAO available from the National Weather Service Climate Prediction Center (http://www.cpc.ncep.noaa.gov/products/precip/CWlink/pna/nao_loading.html). Third, the daily NAO index during 1948–2015 is estimated by projecting the daily HGT anomalies onto the loading pattern of the NAO. Fourth, the daily NAO index is normalized to have a mean of zero and a standard deviation of one during the base period. The Niño3 index, which is defined as the area-averaged sea surface temperature anomaly over the region of 150–90°W, 5°S–5°N, is used for tracking the variability of ENSO. In our study, the monthly Niño3 index is taken from http://www.esrl.noaa.gov/psd/gcos_wgsp/Timeseries/, which is constructed based on the Hadley Centre Sea Ice and Sea Surface Temperature analysis version 1 (Rayner et al. 2003). For the present study, the Niño3 index for a specific day is taken from the associated monthly value of the index. Following Wallace and Gutzler (1981), the EU index is computed by:
where \(Z^{*}\) denotes normalized HGT anomalies on the 500 hPa surface.
In this study, the wave activity fluxes are used to track the origin and propagation of wave energy for cold events. According to Takaya and Nakamura (2001), the horizontal wave activity fluxes \({\mathbf{{W}}_h}\) are calculated by:
where \((\lambda ,\phi )\) are the longitude and latitude, p is the pressure, \(p_0\) is the reference pressure (\(p_0=1000\,{\text{hPa}}\)), a is the radius of Earth, \(\psi\) is geostrophic streamfunction with the prime denoting a perturbation from climatological mean, (u, v) are the climatological mean zonal and meridional winds, and \(\vert U\vert\) is the magnitude of horizontal winds (\(=\sqrt{u^2+v^2}\)).
2.3 Identification of cold events
The cold events over East Asia are identified as follows: First, the daily surface temperature anomalies for each grid point are estimated by removing the average annual cycle for DJFM over the period 1948–2014. Second, the area-averaged land surface temperature anomalies over East Asia (90–150°E, 20–50°N; LSTA) are calculated. Third, a cold event is identified as a period when the LSTA is less than or equal to –2 K for at least five consecutive days. Using this definition, 103 cold events are detected during DJFM 1948–2014.
2.4 Clustering method
A clustering method is used to classify the 103 cold events into different types. Recently, to study the non-stationary relationship between the Siberian High and the Arctic Oscillation, Huang et al. (2016b) proposed the following k-means clustering method based on the area-weighted pattern correlation (AWPC):
where (X, Y) are the samples with horizontal spatial distributions of a variable for two different times, (n, m) and (i, j) are the numbers and corresponding indices of grid points along the zonal and meridional directions, respectively, and \(W_{i,j}\) is the area weight for the grid cell (i, j). The major advantages of the AWPC-based k-means clustering method are that: (1) it classifies the samples based on the phase, which is more suitable for climate analysis than the other clustering methods based on the amplitude; (2) the use of area weights can eliminate the “over-weighting” of the polar cells in the regular longitude-latitude grids often used in climate research; (3) it avoids the introduction of uncertainties during the initialization process that some other clustering methods, such as distance-based k-means (Lloyd 1982) and Self-Organizing Maps (Kohonen 1989) methods, tend to introduce as a result of their random initialization of the centroids. We also carry out additional analyses comparing the classification results from the AWPC-based k-means and distance-based k-means methods in the supplementary material (See Sect. 1 and Figs. S1–4 in Supplementary Material), and nearly all of the stated advantages of the AWPC-based k-means method are confirmed.
Using the AWPC-based k-means clustering method, we classify N samples into M clusters (M is a user-defined parameter with the constraint that \(M\ll N\)). The algorithm for the AWPC-based k-means clustering method includes the following steps. First, the AWPC between each pair of the N samples is calculated and used to define M initial centroids, so that each sample is associated with \(N-1\) AWPCs. A threshold value, AWPC_cr, is used to determine whether two samples are numerically similar. Specifically, two samples are viewed as similar when their AWPC ≥ AWPC_cr; otherwise they are regarded as dissimilar. The first initial centroid is estimated as the average of the sample with the largest number of similar samples (LN) and its LN similar samples (a total of \(LN+1\) samples). The second initial centroid is identified in the same way, but after removing the \(LN+1\) samples that define the first initial centroid. This procedure is repeated until the M initial centroids, each corresponding to a data cluster, are determined. Second, each sample is classified into the cluster with which the sample has the largest AWPC. We define a metric called the within-group AWPC, which is estimated as the average of the AWPCs between all the samples and their corresponding centroids. Third, the M initial centroids are updated by averaging only the samples assigned to each cluster. Fourth, the second and third steps are iterated until the difference in the within-group AWPC between two consecutive iterations is less than \(10^{-6}\).
3 Classification of cold events
3.1 Introduction to the cold events
In this section, we briefly present the basic characteristics of the observed cold events. There are 103 cold events during the analysis period, 75 of which have lifetimes ranging from 5 to 10 days, 20 have lifetimes ranging from 11 to 20 days, and remaining 8 have lifetimes ranging from 21 to 29 days. The average lifetime of all 103 cold events is about 9.50 days.
Composite means of a surface temperature anomalies (shading; units: K) and horizontal wind anomalies on the 850 hPa surface (vectors; units: m s\({^{-1}}\)) and b HGT anomalies on the 500 hPa surface (contour and shading; units: gpm) for the 103 cold events in DJFM 1948–2014. Shading indicates that the composite value meets the 95% confidence level based on two-tailed Student’s t tests. Wind anomalies are plotted if they are significant in at least one direction (zonal or meridional)
Figure 1 illustrates the composite mean anomalies of surface temperature, horizontal winds at 850 hPa, and HGT at 500 hPa during the 103 cold events. Note that the anomalies of temperature are estimated with respect to the annual cycle during 1948–2014, while those of the other variables are estimated by removing the long-term mean over all the winter months. For each spatial point, the significance of the composite mean of the cold event days is tested against the composite mean of the remaining days during DJFM 1948–2014 based on a two-tailed Student’s t test. When cold events are present, the cold anomalies can be observed over large parts of East Asia (Fig. 1a). In addition, the cooling amplitudes over Mongolia and eastern China exceed 5 K with the maximum cooling amplitude exceeding 6 K over northern China. These large temperature anomaly magnitudes can be explained by the significant intensification of the northerlies which drive cold advection over the coastal areas of East Asia and the surrounding oceans (Fig. 1a). Concurrently, warming conditions are evident over the coastal areas of the Kara Sea, which is closely related to the intensification of the southerlies that drive warm advection near the Ural Mountains (around 60°E). It is clear that the observed changes in surface temperature and horizontal winds at 850 hPa are tightly coupled with the changes in the HGT at 500 hPa. The composited map of the HGT anomalies at 500 hPa exhibits a dipole structure over the eastern parts of the Eurasian continent (Fig. 1b). Near the Ural Mountains, a significant anticyclonic anomaly with comparatively large amplitude is present, suggesting that the probability of the development of Ural blocking (Diao et al. 2006) is increased. To the southeast, downstream of the Ural blocking-like positive height anomaly, a cyclonic anomaly is centered over northeastern parts of East Asia, indicating that the EAT is significantly intensified and displaced southward. Both of these effects are conducive to cold air invasion and the occurrence of the East Asian cold events.
3.2 Parameter selection in the AWPC-based k-means clustering method
To classify the cold events, we apply the AWPC-based, k-means clustering method described in Sect. 2.4 to the composite HGT anomaly at 500 hPa associated with each cold event, which is estimated as the time average of the daily HGT anomaly during the cold event. The HGT anomaly for each event is regarded as an independent sample, so that the total number of samples (N) is 103. The region under consideration by the AWPC-based k-means clustering method is 0–180°E, 30–89.5°N, which covers the extratropical part of the Eurasian continent and surrounding oceans. Note that the region under consideration is much larger than the occurrence area of the 103 cold events. It was pointed by Luo et al. (2016a, b) that the cold events over East Asia are often accompanied by Ural blocking. Therefore, regions east of the 0° meridian are included in the clustering analysis. Although the NAO\(^{+}\) events usually precede the Ural blocking by 4–7 days (Luo et al. 2016a, b), the regions over the North Atlantic Ocean are not wholly considered because the lead times for different precursor signals are different (a detailed discussion can be found in Sect. 4.1). In addition, the time period used for clustering is when the cold events are present, during which the precursor signals from the North Atlantic Ocean have already propagated to the Eurasian continent and concurrent signals in the North Atlantic Ocean are therefore unlikely to exert strong effects on East Asian cold events. Further, cold events over East Asia are also influenced by downstream pressure ridges (Takaya and Nakamura 2005b; Cheung et al. 2015; Cheung and Zhou 2016). Therefore, the eastern boundary for the clustering analysis is set to the 180° meridian.
As mentioned in Sect. 2.4, there are two user-defined parameters in the AWPC-based k-means clustering method: (1) the number of clusters (M) and (2) the threshold value of the AWPC (AWPC_cr). To estimate the optimal combination of these two parameters, we employ a grid search method, wherein M ranges from 2 to 6 and AWPC_cr ranges from 0.3 to 0.7 with an interval of 0.05. Table 1 lists the computational results for the within-group AWPC as a function of M and AWPC_cr. A larger value of within-group AWPC means a better classification performance, while a large number of clusters can introduce redundancy among the cluster centroids. The principle goal of the parameter selection is to achieve the target classification accuracy for the within-group AWPC using the smallest number of clusters. In this study, the target classification accuracy is set to 0.6, a level where the similarity between each sample and the corresponding centroid can be considered to be relatively high. The smallest number of clusters (M) that achieves the target accuracy is four. However, there are five different choices for the parameter AWPC_cr, namely 0.45, 0.55, 0.6, 0.65, and 0.7, that lead to a within-group AWPC exceeding 0.6. To further constrain the optimal AWPC_cr, a metric called the minimum-group AWPC (min-group AWPC) is introduced and defined as the minimum value of the within-group AWPC among the M clusters. Table 2 lists the min-group AWPC as a function of M and AWPC_cr. Since the optimal number of clusters (M) has been determined to be 4, the optimal value for AWPC_cr has to be selected from the five possible choices (0.45, 0.55, 0.6, 0.65, and 0.7). Since a larger min-group AWPC corresponds to a more accurate classification, the optimal value for AWPC_cr is 0.65 because it gives the largest value for the min-group AWPC of 0.59. Under this optimal pair of parameter values, the within-group AWPCs for the four clusters are 0.60, 0.59, 0.60, and 0.61, indicating that the classification accuracy is uniform and relatively high.
3.3 Four circulation regimes for cold events over East Asia
Figure 2a–d shows the composite mean HGT anomalies at 500 hPa for the four regimes identified by the AWPC-based k-means clustering method. The numbers of cold events belonging to regimes 1–4 are 43, 22, 19 and 19, respectively.
Composite means of a–d HGT anomalies on the 500 hPa surface (contour and shading; units: gpm) and e–h surface temperature anomalies (shading; units: K) and horizontal wind anomalies on the 850 hPa surface (vectors; units: m s\({^{-1}}\)) for the four different regimes identified by the AWPC-based k-means clustering method during DJFM 1948–2014. Shading indicates that the composite value meets the 95% confidence level based on two-tailed Student’s t tests. Wind anomalies are plotted if they are significant in at least one direction (zonal or meridional). The number of cold events belonging to each regime and the associated within-group AWPC are shown at the top right corner of the panels in the left column
Regime 1, which has the largest number of cold events and accounts for 41.7% of all cold events, has a circulation pattern characterized by a dipole HGT anomaly structure over the Eurasian continent. This dipole has an anticyclonic anomaly near the Ural Mountains and a cyclonic anomaly over East Asia and the surrounding oceans (Fig. 2a). The area-weighted pattern correlation coefficient between the horizontal distribution of the HGT anomalies for regime 1 and that for the composite mean of all the 103 cold events (Fig. 1b) is 0.91, further indicating that regime 1 is the dominant regime. The circulation pattern here is very similar to the Ural blocking circulation pattern identified by Diao et al. (2006). As pointed out by Luo et al. (2016a, b), the Ural blocking circulation pattern is crucial for the occurrence of cold events over East Asia.
Regime 2, which accounts for 21.4% of the cold events, also features a dipole structure of HGT anomalies over the eastern parts of the Eurasian continent (Fig. 2b). However, when compared with regime 1, the center of the anticyclonic anomaly for this regime is located farther to the southeast, and the amplitudes of the negative HGT anomalies associated with the cyclonic anomaly over the northeastern parts of East Asia are much smaller. Further, there are two additional cyclonic anomalies centered over northern Europe and the Chukchi Sea in this regime that are not present in regime 1. The presence of the cyclonic anomaly over northern Europe significantly intensifies the southerlies along 60°E upstream of the anticyclonic anomaly near the Ural Mountains.
Regime 3, which accounts for 18.4% of the cold events, has a structure similar to regime 1, in that it is also characterized by a widespread cyclonic anomaly over East Asia and the Sea of Okhotsk (Fig. 2c). However, unlike regime 1, the center of this cyclonic anomaly extends over the oceanic regions farther to the northeast. In addition, under this regime, positive HGT anomalies associated with an anticyclonic anomaly also can be observed west of the Ural Mountains. Compared with regimes 1 and 2, the center of the positive HGT anomalies is shifted to the Norwegian Sea and their amplitudes are much smaller.
Regime 4, which has an occurrence frequency equal to that of regime 3, has a distinctive widespread anticyclonic anomaly anchored over the North Pacific Ocean (Fig. 2d). Simultaneously, an anticyclonic anomaly located near the Ural Mountains is also evident. Under this regime, significant negative HGT anomalies are rarely observed over the northeastern parts of East Asia, indicating that the EAT is weaker during this regime than it is under the other regimes. The weak EAT is closely related to the presence of the powerful anticyclonic anomaly over the North Pacific Ocean, which inhibits the southward extension of the EAT.
3.4 Long-term variations in the four circulation regimes
Figure 3a shows interannual variations in the number of cold events and the number of associated cold days during 1948–2014. As reported by many previous studies (Watanabe and Nitta 1999; Wang et al. 2009; Huang et al. 2016a), there was a continuous weakening in the East Asian winter monsoon that began around 1987/1988. This weakening is also reflected in the numbers of identified cold events and cold days. Specifically, during the period preceding 1987 (1948–1987), the time-averaged numbers of cold events and cold days were 1.75 and 17.58, respectively, while following 1987 (1988–2014), these numbers were reduced to 1.22 and 10.22.
a Interannual variations in the numbers of cold events (red bars; right ordinate) and the associated cold days (units: days; blue bars; left ordinate) during DJFM 1948–2014, and b those belonging to the four different regimes. The total numbers of all cold events and the associated cold days are shown at the top right corner of a. In b, the ratio and the correlation coefficient between the number of cold days associated with each regime and that associated with all events are shown in parentheses and on the right, respectively
We further estimate the numbers of cold events and cold days for the four different regimes, and the results are shown in Fig. 3b. During 1948–1987, the time-averaged numbers of cold events (cold days) for the four regimes were 0.70 (8.65), 0.38 (3.68), 0.35 (2.98), and 0.33 (2.28), while during 1988–2014, these numbers decreased to 0.56 (4.85), 0.26 (2.15), 0.19 (1.85), and 0.22 (1.37), respectively. Therefore, all four circulation regimes play roles in the reduction of the numbers of cold events and the associated cold days around 1987/1988.
Additionally, the numbers of cold days associated with the cold events for regimes 1–4 account for about 48.7, 20.9, 17.3, and 13.1%, respectively, of all the cold days associated with the 103 cold events (Fig. 3). The correlation coefficient between the interannual variation in the number of cold days associated with all events and that associated with each regime is calculated. The corresponding correlation coefficients for regimes 1–4 are 0.67, 0.38, 0.40, and 0.35, respectively, all of which are statistically significant at the 99% confidence level based on two-tailed Student’s t tests. Therefore, while regime 1 makes a relatively large contribution to variations in cold days associated with the identified cold events, the contributions from the other three regimes cannot be neglected.
3.5 Surface temperature anomalies of the four circulation regimes
This section examines the structure of the surface temperature anomalies associated with each circulation regime. All four cold event regimes are associated with significant cold anomalies of comparably large amplitudes over East Asia (Fig. 2e–h). However, the horizontal distributions of the surface temperature anomalies and anomalous winds on the 850 hPa surface are quite different.
The distribution of surface temperature anomalies for regime 1 (Fig. 2e) is similar to that for the composite mean of all cold events, owing to its dominance of the occurrence frequency. The cooling center in regime 1 is located over Mongolia and northern China, with cold temperature anomaly amplitudes exceeding 5 K. The cooling process in this regime is dominated by the northerly anomalies over East Asia, which transport cold air from higher latitudes and help to maintain cold temperatures over East Asia. The northerly anomalies over southern China under regime 1 are relatively weaker than those under the other three regimes. The cooling center in this regime is therefore the northernmost among the four regimes (Fig. 2e–h). The mean lifetime of cold events belonging to regime 1 is about 11.09 days with a standard deviation of 6.74 days (Fig. 4a). Almost half of the regime 1 cold events (44.2%; Fig. 4b) lasted for more than 10 days. The quasi-stationary characteristic of the Ural blocking can explain the longer average lifetime of the cold events belonging to this regime (Yao et al. 2017). This quasi-stationarity of the Ural blocking and the minimum duration (5 days) used for identifying cold events over East Asia partially explain why this regime had the highest occurrence frequency.
The cooling center for regime 2 is located over Inner Mongolia and has an amplitude exceeding 7 K (Fig. 2f). The amplitude of the cooling over southern China in this regime is greater than 5 K, which exceeds that in regime 1 (Fig. 2e). This southward extension of the cooling in regime 2 relative to regime 1 is accompanied by stronger northerly anomalies over East Asia. Like regime 1, there also are warm temperature anomalies over the Kara Sea and surrounding coastal areas under regime 2, but their amplitudes are substantially larger. These relatively large warm anomalies near the Kara Sea can be explained by the presence of strong southerly anomalies along 60°E. The average lifetime of the cold events in regime 2 is 9.32 days with a standard deviation of 5.20 days (Fig. 4a). Note that this average lifetime is the second longest among the four regimes, indicating the possible existence of blocking circulation over central Siberia (Fig. 2b).
The cooling center of regime 3 is located primarily over eastern China, with cooling amplitudes over both northern and southern China exceeding 5 K (Fig. 2g). It should be noted that the amplitude of the cooling over East Asia under regime 3 is the smallest among the four regimes. Cooling amplitudes larger than 4 K are also evident over land areas east of the Caspian Sea. This cooling is mainly due to the far westward placement of the positive HGT anomalies at 500 hPa (Fig. 2c) that causes an enhancement of the cold advection along 60°E. Compared to the other regimes, regime 3 has no apparent warming near the Kara Sea, which is also due to the westward placement of the positive HGT anomalies (Fig. 2c). The cold events belonging to this regime have an average lifetime of 8.89 days with a standard deviation of 6.24 days (Fig. 4a).
Under regime 4, the most prominent feature of the composite surface temperature anomalies is that the cooling center is located over southern China (Fig. 2h) and its amplitude exceeds 7 K. Corresponding to the anomalous circulation pattern at the 500 hPa level (Fig. 2d), there is also a powerful anticyclonic anomaly at the 850 hPa level (Fig. 2h). As a consequence of the cold advection downstream of this surface anticyclonic anomaly, cold anomalies with amplitudes that exceed 1 K can be observed over the region east of 150°W. Due mainly to the warm advection upstream of the surface anticyclonic circulation, warm anomalies with amplitudes larger than 1 K can be observed over the region of 150–180°E, 30–45°N. The mean lifetime of the cold events under this regime is the shortest among the four regimes (6.74 days with a standard deviation of 1.41 days) and all cold events belonging to this regime have lifetimes shorter than 10 days (Fig. 4). The short lifetime of events in this regime is likely due to the presence of the anticyclonic anomaly over the North Pacific Ocean, which inhibits the southward movement of the EAT and cuts off the supply of cold air.
4 Time evolution of circulation and temperature anomalies
This section explores the characteristics of the circulation patterns and surface temperature anomalies before and during the cold events in each regime. The focus is on the period prior to the onset of cold events in an attempt to better understand their origins and causes.
4.1 Time evolution of middle-tropospheric geopotential height anomalies
The composite time evolutions of the HGT anomalies and associated wave activity fluxes on the 500 hPa surface at 2 day intervals from day –18 to day 4 for each regime type are shown in Figs. 5, 6, 7 and 8. Note that the composite on day 0 represents the average over all start days for the cold events belonging to each regime, while that on day –N or N is the average over all the days N days before or after the onset of cold events. The statistical significance of the composite fields is tested against the remaining winter days during 1948–2014 based on two-tailed Student’s t tests. To support the analysis of the evolution of the circulation anomalies, we also calculate the concurrent composite time evolutions of the NAO and EAT indices for the four regimes (Fig. 9a, b). The NAO index is used to explore whether upstream effect in the North Atlantic Ocean has a role in the initialization of the East Asian cold events, while the EAT index is used to examine whether the EAT is enhanced, which is usually favorable to the cold air invasion from higher latitudes to East Asia.
Composite time evolutions of HGT anomalies (contour and shading; units: gpm) and wave activity fluxes (vector; units: m\({^{2}}\) s\({^{-2}}\)) on the 500 hPa surface for regime 1 from day –18 to day 4 (with an interval of 2 days) with respect to the onset of cold events. Shading indicates that the composite value meets the 95% confidence level based on two-tailed Student’s t tests. Wave activity fluxes are plotted if they are significant in at least one direction (zonal or meridional)
Same as Fig. 5 but for regime 2
Same as Fig. 5 but for regime 3
Same as Fig. 5 but for regime 4
Under regime 1, the anticyclonic anomaly near the Ural Mountains during cold events (Fig. 2a) can be traced back to an anticyclonic anomaly over the Laptev Sea on day –16 (Fig. 5b), while the cyclonic anomaly located in East Asia can be traced back to a cyclonic anomaly near Lake Baikal on day –14 (Fig. 5c). On day –18, there is wave activity flux that emanates from Greenland and propagates to the Laptev Sea and the surrounding coastal areas (Fig. 5a), which gives rise to the initialization of the anticyclonic anomaly over the Laptev Sea on day –16. The downward propagation of wave activity flux from the above anticyclonic anomaly further leads to the generation of the cyclonic anomaly near Lake Baikal on day –14. From the timing of these two anomalies, the anticyclonic anomaly originating from the Laptev Sea can be regarded as the earliest precursor signal for the cold events belonging to regime 1. Since the wave activity flux favorable to the development of this anticyclonic anomaly primarily emanates from Greenland (one of the centers of activity for the NAO), it is inferred that the circulation anomalies over the North Atlantic Ocean are indispensable to the generation of the circulation patterns favorable to the regime 1 cold events. While the initial signals of these anticyclonic (cyclonic) precursor anomalies are quite weak, due to the continuous transport of wave activity flux from the North Atlantic Ocean, these signals gradually strengthen. This continuous wave activity flux also triggers the generation of a cyclonic anomaly over Europe, which by day –12 is located upstream of the anticyclonic anomaly over the Laptev Sea (Fig. 5d). This cyclonic anomaly over Europe and the downstream anticyclonic-cyclonic dipole together constitute a Rossby wave train, which propagates slowly toward the southeast after day –10. By the onset of cold events, the cyclonic anomaly over Lake Baikal has moved to the coastal areas of East Asia, which favors the southward displacement and rapid enhancement of the EAT (Fig. 9a). It should be noted that on day –18, there is a dipole structure of the HGT anomalies over the North Atlantic Ocean, with a cyclonic anomaly around Greenland and an anticyclonic anomaly to its south (Fig. 5a), which resembles the NAO\(^{+}\) circulation pattern. Moreover, the composite NAO index ranges from 0.25 to 0.32 during the time period between day –18 and day –15 (Fig. 9b). Subsequently, the NAO\(^{+}\)-like pattern gradually decays and the NAO index becomes negative after day –13, when the Ural blocking-like circulation pattern dominates over the Eurasian continent. Therefore, the NAO\(^{+}\)-like circulation pattern clearly plays an important role in the formation of the precursor anticyclonic anomaly, subsequent cyclonic anomaly, and thus the Ural blocking circulation pattern that triggers the subsequent cold events over East Asia. This evolution is consistent with the conclusion drawn by Luo et al. (2016a, b) that the Ural blocking events always lag the NAO\(^{+}\) events by about 4–7 days.
Under regime 2, few precursor circulation signals are significant before day –4 (Fig. 6). On day –4, two simultaneous anomalies appear, one being an anticyclonic anomaly that forms over the coastal areas of the Kara Sea and the other a cyclonic anomaly centered over Mongolia (Fig. 6h). These anomalies subsequently form a dipole structure after the anticyclonic anomaly moves over central Siberia and the cyclonic anomaly moves over East Asia and surrounding oceans during the cold events (Fig. 2b). Further, the two cyclonic anomalies over northern Europe and the Chukchi Sea can also only be traced back to cyclonic anomalies near the Arctic Ocean on day –4. The cyclonic anomaly over the Norwegian Sea, the anticyclonic anomaly around the Kara Sea and the cyclonic anomaly near Mongolia on day –4 constitute a Rossby wave train. Due to the presence of the wave activity flux emanating from the North Atlantic Ocean, the HGT anomalies associated with this wave train get stronger, especially the anticyclonic anomaly over central Siberia. Note that for regime 2 the center of the anticyclonic anomaly in the dipole structure on day –2 is located more than 10° east of the location of the analogous structure in regime 1 (Figs. 5i, 6i). Meanwhile, there is also a significant dipole structure over the North Atlantic Ocean, with an anticyclonic anomaly near the southern coast of Greenland and a cyclonic anomaly to its south, which resembles the negative phase of the NAO (NAO\(^{-}\)). The start of rapid enhancement in the EAT can be observed on day –2 (Fig. 9a), primarily because the cyclonic anomaly over Mongolia moves into the EAT region. Therefore, the lead time of the precursor signals for regime 2 is only 4 days, considerably shorter than the roughly 16 days for regime 1. Although an NAO\(^{-}\)-like pattern is present prior to the occurrence of the cold events under this regime, the earliest circulation anomalies that can be taken as precursor signals are present over the Arctic Ocean and Eurasian continent, suggesting that the NAO-related circulation pattern only plays a secondary role in the initialization of circulation patterns favorable to the cold events under regime 2.
Under regime 3, the cyclonic anomaly related to the cold events (Fig. 2c) can be traced back to a cyclonic anomaly that appears to the southeast of the Caspian Sea on day –16 (Fig. 7b). However, from day –16 to day –10, the wave activity flux to the cyclonic anomaly is relatively weak, so that the amplitude and position of this cyclonic anomaly remain nearly unchanged. In contrast, although the anticyclonic anomaly centered over the Norwegian Sea (Fig. 2c) is a large distance from East Asia, its presence is crucial for the generation of the cold events over East Asia under this regime and it can be traced back to an anticyclonic anomaly near the East Siberian Sea on day –12 (Fig. 7d). After its initialization, this anticyclonic anomaly moves upstream along the Arctic coastline of northern Russia until day –8 when it reaches 45°E (Fig. 7f). At that time, there is another cyclonic anomaly located over western Europe, part of a Rossby wave train that also includes the anticyclonic anomaly originating from the East Siberian Sea and the cyclonic anomaly originating from the Caspian Sea. Note that on day –8, due mainly to the propagation of this wave train, the cyclonic anomaly near the Caspian Sea begins to move southeastward. Further, as a consequence of the flux of wave activity from the North Atlantic Ocean, the cyclonic anomaly begins to amplify. On day –4, there is a dipole structure consisting of an anticyclonic anomaly to the southwest of Greenland and a cyclonic anomaly over western North America, which is similar to the NAO\(^{-}\) pattern (Fig. 7h). This NAO\(^{-}\)-like pattern slowly propagates downstream and reaches its peak on day 0 (Fig. 9b). In particular, there is additional wave activity flux from the anticyclonic anomaly to the Rossby wave train over the Eurasian continent, so that the dipole structure over the North Atlantic Ocean plays a role in the amplification of the circulation pattern favorable to the initialization of cold events under this regime. On day 0, the cyclonic anomaly covers almost the entire East Asian region (Fig. 7j), which significantly intensifies the EAT and triggers the start of cold events under this regime. Therefore, the precursor signal for regime 3 cold events is a cyclonic anomaly originating from the Caspian Sea with a lead time of about 16 days.
Regime 4 is characterized by two anticyclonic anomalies, one centered to the east of the Ural Mountains and the other over the North Pacific Ocean (Fig. 2d). The anticyclonic anomaly centered east of the Ural Mountains can be traced back to an anticyclonic anomaly that appears over the Barents Sea on day –8 (Fig. 8f), while the anticyclonic anomaly over the North Pacific Ocean can be traced back to an anticyclonic anomaly that appears over the coastal areas of northern China on day –10 (Fig. 8e). It is worth noting that there is also a persistent cyclonic anomaly centered at 90°E near Lake Balkhash, which lasts from day –18 to day 0. On day –8, a wave train emanating from the northeastern parts of North America to the Ural Mountains appears. This wave train intensifies and transports wave activity flux downstream between day –8 and day –6, significantly intensifying the anticyclonic anomaly over the coastal areas of northern China. The upstream wave activity flux from the North Atlantic Ocean is cut off around day –4 (Fig. 8h). After this change, the dipole structure over East Asia, comprised of an anticyclonic anomaly near the Ural Mountains and a cyclonic anomaly to its southeast, propagates southeastward and carries wave energy to the anticyclonic anomaly over the North Pacific Ocean, which has shifted from its initial location over the coastal areas of northern China. The presence of the anticyclonic anomaly over the North Pacific Ocean weakens the intensity of the EAT. As a consequence, the EAT index increases prior to the onset of the cold events (Fig. 9a). After day 0, the cyclonic anomaly near Lake Balkhash moves into the vicinity of the EAT (Fig. 8j), significantly enhancing the intensity of the EAT and effectively initiating the regime 4 cold events. In addition, a dipole structure can be observed on day 0 around Greenland which resembles the NAO\(^{+}\) pattern. However, unlike regimes 1–3, there is no significant NAO-related circulation pattern before day 0, suggesting that the NAO may not play a significant role in the initialization of the circulation pattern favorable to the generation of cold events under regime 4. Therefore, the precursor signal for regime 4 is the cyclonic anomaly centered over Lake Balkhash, which has a lead time of 18 days.
Based on the above analyses of the evolutions of HGT anomalies and wave activity fluxes, the earliest precursor signals for the cold events belonging to regimes 1–4 are, respectively, the anticyclonic anomaly over the Laptev Sea, the anticyclonic anomaly near the Kara Sea and three cyclonic anomalies (two located in the Arctic Ocean and the other located in Mongolia), the cyclonic anomaly over the Caspian Sea, and the cyclonic anomaly centered over Lake Balkhash. The lead times of these precursor signals are 16, 4, 16, and 18 days, respectively. In particular, the earliest precursor anticyclonic anomaly for regime 1 evolves into a Ural blocking-like pattern, while that for regime 2 develops into a central Siberian blocking-like pattern. Therefore, the first two regimes are closely related to blocking activities over the Eurasian continent, while the last two regimes are associated with cyclonic anomalies over the Eurasian continent.
In addition, the circulation anomalies over the North Atlantic Ocean play important roles in the generation and/or amplification of the precursor anomalies for the cold events under all four regime types. In particular, under regime 1, an NAO\(^{+}\)-like circulation pattern precedes the occurrence of cold events by 18 days and excites the Ural blocking-like pattern, which favors cold air invasion from higher latitudes to East Asia. However, the NAO-related circulation pattern is not critical for the generation of the earliest precursor circulation pattern favorable to the occurrence of cold events belonging to regimes 2–4. Nevertheless, following the appearance of the earliest precursor signals under regimes 2–4, there is wave activity flux from the North Atlantic Ocean that favors the amplification of the circulation pattern over the Eurasian continent and leads to the occurrence of the East Asian cold events.
4.2 Time evolution of surface temperature anomalies
Following the same compositing method for estimating the time evolutions of the EAT and NAO indices in Sect. 4.1, we composite the time evolution of the LSTA. The time evolutions of LSTAs for the four regimes have some similar characteristics. While they do not reach the temperature criterion of a cold event (–2 K), rapid drops in LSTA occur in all four regime types two or three days prior to the onset of the subsequent cold event (Fig. 9c).
While there are similarities, the time evolutions of the horizontal distributions of surface temperature anomalies under the four regimes are different. Figure 10 illustrates the composite time evolutions of surface temperature anomalies in each regime from day –16 to day 4 at an interval of 4 days.
Time evolutions of the composite surface temperature anomalies for the four different regimes from day –16 to day 4 (with an interval of 4 days) relative to the onset of cold events. Only composite values that meet the 95% confidence level based on two-tailed Student’s t tests are plotted. Each row represents the day relative to the onset of the cold events under each regime, while each column represents the time evolution of each regime
By day 0, cold anomalies with amplitudes exceeding 7 K can be observed over Mongolia and northern China under all the four regimes. Further, the areas east of the Caspian Sea also experience severe cooling effects under regime 3, with surface temperature anomaly amplitudes larger than 7 K. These Caspian Sea cold anomalies are closely related to the northerly anomalies upstream of the cyclonic anomalies there (Fig. 7j). By day –4, cold anomalies also can be observed around central Siberia in all the four regimes. Specifically, the maximum cooling amplitude over these regions, which only exceeds 4 K under regime 2, exceeds 7 K under regimes 1, 3, and 4. In addition, the spatial extent of the significant cold anomalies under regime 2 is smaller than the widespread coverage seen under the other regimes. From the horizontal distributions of the cold anomalies on day –4 and day 0 for all regimes, it is inferred that central Siberia is the preferred transfer station for temperature anomalies prior to the cold events over East Asia. Note that on day –8, the cold anomalies under regimes 1, 3, and 4 cover the western and central parts of Russia, although the cooling center under regime 1 is shifted a bit northward relative to the other two. Regime 2 is unique in that there are no significant temperature anomalies over the Eurasian continent on day –8. This is consistent with the analysis in Sect. 4.1 that found that regime 2 has the shortest lead time for its earliest precursor signal. While on day –12 there are scattered temperature anomalies over the Eurasian continent for all the four regimes, they are not well organized and not identifiable as a cold event precursor. Therefore, the temperature anomalies before day –8 are not further analyzed.
5 Relationships with large-scale circulation modes
This section investigates the links that the four cold event regimes have to the large-scale circulation modes including the NAO, EU, and ENSO. Note that the role of the atmospheric variability originating from Arctic areas is not examined in this paper due to the fact that the relationship between the Arctic circulation and the mid-latitude weather is complex and controversial (Barnes and Screen 2015).
The composite NAO indices during regimes 1–4 cold events are −0.34, −0.30, −0.50, and 0.33, respectively, all of which are statistically significant at the 99% confidence level (Table 3). Although the composite NAO indices in regimes 1–3 are negative, there are still 44 cold events (accounting for about 42.7% of the 103 cold events) that occurred during NAO\(^{+}\) (Fig. 11). In particular, the ratio of the cold events associated with NAO\(^{+}\) under regime 1 is about 44.2%, slightly larger than that of all the events. As discussed by Luo et al. (2016b), Ural blocking can occur under multiple NAO states (NAO\(^{+}\), NAO\(^{-}\), NAO\(^{+}\)-to-NAO\(^{-}\), or neutral NAO). The similar frequencies of NAO\(^{+}\) and NAO\(^{-}\) under regime 1 are therefore unsurprising, since the cold events over East Asia tend to emerge during the mature or decaying stages of Ural blocking (Cheung et al. 2013; Luo et al. 2016a). By comparison, the proportions of cold events associated with NAO\(^{-}\) under regimes 2 and 3 are 68.2 and 68.4%, respectively, which are relatively higher than that under regime 1 (55.8%). This is consistent with the observation that cold events belonging to these two regimes closely follow the NAO\(^{-}\)-like circulation patterns (Sect. 4.1). Under regime 4, there are 63.2% cold events occurred during NAO\(^{+}\). This difference from the other three regimes is consistent with the concurrence of the NAO\(^{+}\)-like circulation pattern and cold events under this regime (Sect. 4.1).
As revealed by many previous studies (Wallace and Gutzler 1981; Gong et al. 2001; Liu et al. 2014; Park et al. 2014), the EU teleconnection pattern plays an important role in East Asian winter climate. The composite EU indices for regimes 1–4 are 0.44, 0.45, 0.26, and 0.21, respectively, all of which are statistically significant at the 99% confidence level (Table 3). In total, 87 of the cold events identified occurred during EU\(^{+}\) and only 16 cold events occurred during EU\(^{-}\) (Fig. 11). This tendency for cold events over East Asia to occur during EU\(^{+}\) is consistent with previous studies (Sung et al. 2009; Lim and Kim 2016). During the period from day 0 to day 4, Rossby wave activity is evident over the Eurasian continent under all regimes (Figs. 5, 6, 7, 8). However, the distance between the centers of the individual circulation anomalies within the wave train and those used for defining the EU index varies from regime to regime. These differences likely explain the different amplitudes of the EU indices among the four regimes.
Considering the relationship of the cold events to ENSO, the composite Niño3 indices for the four regimes are –0.17 (not significant at the 90% confidence level), –0.34 (99% confidence level), –0.24 (90% confidence level), and –0.44 (99% confidence level). In total, 74 cold events occurred during ENSO\(^{-}\) and 29 cold events occurred during ENSO\(^{+}\) (Fig. 11). Therefore, cold events over East Asia preferentially occur during ENSO\(^{-}\), which is consistent with results from previous studies (Zhang et al. 1997; Chen et al. 2000; Wu and Leung 2009). The linkage between ENSO\(^{-}\) and cold events over East Asia can likely be explained by the Pacific–East Asian teleconnection pattern (Wang et al. 2000), which can induce a cyclonic anomaly over the western North Pacific Ocean during ENSO\(^{-}\). Northerly wind anomalies along the northwest boundary of this cyclonic anomaly can effectively intensify the wintertime northerly over East Asia and surrounding oceans, favoring the development of the cold surge. It should be noted that the largest amplitude in the composite Niño3 index under regime 4 is closely related to the anticyclonic anomaly over the North Pacific Ocean. This anticyclonic anomaly can be linked to the negative sea surface temperature anomalies over the tropical central Pacific Ocean through the Pacific/North American (PNA) teleconnection pattern (Renwick and Wallace 1996; Hoerling et al. 1997). Through its modulation of the North Pacific anticyclonic anomaly, ENSO plays an important role in the formation of the horizontal distribution of the temperature anomalies over East Asia.
6 Contributions of different physical processes to surface temperature variations
This section is devoted to estimating the contributions of different physical processes to changes of the surface temperature over East Asia and surrounding areas during cold events for each regime type. Following Yao et al. (2017), the surface temperature tendency can be divided into four different components: horizontal temperature advection (T\(_{advection}\)), adiabatic warming/cooling by vertical motions (T\(_{adiabatic}\)), surface downward infrared radiation (IR) flux, and sensible and latent heat (SLH) flux. The contributions of the downward IR flux and the SLH flux are estimated on the surface, while the T\(_{advection}\) and T\(_{adiabatic}\) are calculated approximately at the 850 hPa level according to Yanai et al. (1973) and Yanai and Tomita (1998):
where T is temperature, \(\theta\) is potential temperature, p is pressure, \({\varvec{\vec {V}}}_h\) is the horizontal wind vector and \(\omega =\frac{dp}{dt}\) is the velocity of vertical motion, and \(\nabla _h\) is the gradient operator on an isobaric surface. In order to directly compare the physical processes, the units of T\(_{advection}\) and T\(_{adiabatic}\) processes are converted from \(K\,s^{-1}\) to \(W\,m^{-2}\) based on the method of Yao et al. (2017). Figure 12 shows the composite anomalies of horizontal temperature advection, adiabatic process, downward IR flux, and SLH flux (a positive value means warming effect) during cold events belonging to different regimes.
Composite mean anomalies of horizontal temperature advection (T\(_{advection}\)) at 850 hPa, adiabatic warming/cooling by vertical motions (T\(_{adiabatic}\)) at 850 hPa, surface downward infrared radiation (IR) flux, and surface sensible and latent heat (SLH) flux for the four different regimes. Only composite values that meet the 95% confidence level based on two-tailed Student’s t tests are plotted
6.1 Contribution of horizontal temperature advection
Under all regimes, there are significant cold advection anomalies over the coastal regions of East Asia and the surrounding oceans (Fig. 12), which are closely related to the intensified northerly anomalies over this region (Fig. 2e–h). Comparing the two regions, the cold advection anomalies are of much larger amplitude over the surrounding oceans than over the coastal areas. It should be noted that in regime 4 cold events the surrounding ocean areas with cold advection anomaly amplitudes exceeding 60 W m\(^{-2}\) are much smaller than those in the other three regimes. This is due to the presence of the anticyclonic anomaly over the North Pacific Ocean during regime 4 cold events that weakens the northerly wind anomalies and reduces cold advection.
The horizontal distribution of cold advection anomalies over East Asian land areas is closely related to the cooling center of the cold events for each regime. Under regime 1, the maximum cold advection anomalies over land areas are located in northern China with amplitudes larger than 40 W m\(^{-2}\), which is consistent with the geographical location of the cooling center under this regime (Fig. 2e).
Under regime 2, the maximum cold advection anomalies are also located in northern China, but cold advection anomalies with amplitudes of 20–40 W m\(^{-2}\) penetrate into southern China. As a consequence, cold temperature anomalies with amplitudes larger than 5 K also extend into southern China under this regime (Fig. 2f).
Under regime 3, the significant cold advection anomalies are confined to northern China, with amplitudes ranging from 20 to 40 W m\(^{-2}\). The relatively smaller spatial extent and amplitude of cold advection anomalies under this regime result in the weakest cooling over East Asian land areas among the four regimes (Fig. 13). This distribution of the cold advection anomaly partially explains why cold events in this regime have the smallest cooling intensity (Fig. 2g).
Area-averaged composite anomalies of horizontal temperature advection (T\(_{advection}\)) at 850 hPa, adiabatic warming/cooling by vertical motions (T\(_{adiabatic}\)) at 850 hPa, surface downward infrared radiation (IR) flux, and surface sensible and latent heat (SLH) flux over land areas of East Asia for the four different regimes
Under regime 4, cold advection anomalies with amplitudes larger than 40 W m\(^{-2}\) cover the coastal areas of eastern China between 30° and 40°N. These cold advection anomalies are larger than those associated with the other three regimes over the same region (Fig. 13). In addition, a local maximum in cold advection anomalies is also evident over the southwestern portion of East Asia with an amplitude larger than 60 W m\(^{-2}\). This is evidence of the southward advance of the cold advection anomaly, which induces a southward shift in the cooling center under this regime (Fig. 2h).
6.2 Contribution of adiabatic process
In all four regimes, the contributions of the adiabatic process to surface temperature anomalies are characterized by a dipole structure over East Asian land areas. This dipole features the positive anomalies (warming effects) over northeastern East Asia and negative anomalies (cooling effects) over southwestern East Asia (Fig. 12). The warming effects over the northeastern parts of East Asia are closely related to the descending motion in the atmosphere (Fig. S5), due mainly to an expansion of surface anticyclonic anomalies associated with an enhanced Siberian High to East Asian coast during cold events (Fig. S6). In contrast, the cooling effects over the southwestern parts of East Asia are mainly caused by the ascending motion in the atmosphere over this region (Fig. S5). These ascending motions can be explained by increased atmospheric instability related to the enhanced vertical temperature contrast between the colder air aloft (in the middle and lower troposphere) and the warmer air near the land surface. The area-averaged values of the adiabatic process over East Asian land areas for the four regimes are 0.98, 2.79, 0.93, and −3.40 W m\(^{-2}\), respectively (Fig. 13). The relatively small area-averaged contribution of the adiabatic process to the surface temperature variations over East Asia is primarily attributable to compensation between the positive anomalies and negative anomalies in the dipole structure.
6.3 Contribution of downward IR flux
In all regimes, there are significant negative anomalies in the downward IR flux over East Asian land areas (Fig. 12). In particular, under regimes 1–3, areas with significant negative IR flux anomalies cover nearly all of East Asian land areas, considerably more widespread than the significant anomalies contributed by other processes. However, under regime 4, the region of significant negative downward IR flux anomalies is confined to Mongolia and northern China. Since downward IR flux has a warming effect on surface temperature, the decreased downward IR flux is conducive to the cooling process over land areas of East Asia. The area-averaged values of the downward IR flux anomalies over East Asian land areas for the four regimes are −19.10, −16.30, −21.22, and −7.70 W m\(^{-2}\), respectively (Fig. 13). The reduced coverage and the small amplitude of the area-averaged negative IR flux anomaly over East Asian land areas under regime 4 are closely related to the more widespread cloud coverage relative to the other regimes. This more widespread cloud coverage during regime 4 cold events, especially in southern China, can be understood as follows: First, the cooling center under regime 4 is located in southern China, where atmospheric moisture is relatively more abundant. Second, as described in Sect. 6.2, the increased atmospheric instability in this region favors ascending air mass motions. Both of these features are conducive to the development of more extensive cloud cover under regime 4. It should be noted that the dominant role of the downward IR flux on the surface cooling during the first three regimes is consistent with the results given by Yao et al. (2017), who pointed out that radiative cooling is extremely important for the development of Eurasian wintertime cold extremes.
6.4 Contribution of SLH flux
In all regimes, there are significant negative SLH flux anomalies (cooling effects) over the oceans surrounding East Asia, with maximum amplitudes all exceeding 120 W m\(^{-2}\). These extremely high heat fluxes from the sea surface to the lower troposphere are caused by the sharp contrast between the underlying warmer and moister ocean surface and the colder and drier air masses advected over it from the high latitudes.
The SLH flux situation over land areas of East Asia is rather different than that over the surrounding oceans. Specifically, the area-averaged values of the SLH flux anomalies over East Asian land areas for the four regimes are 4.53, 2.82, 5.42, and −13.12 W m\(^{-2}\), respectively (Fig. 13). A further investigation reveals that the warming effects associated with positive SLH flux anomalies in the first three regimes can be attributed to the latent heat flux (Fig. S7). These positive latent heat flux anomalies can be explained by the fact that the underlying surface experiencing the most severe cooling under these three regimes is drier than it is under regime 4. Under regime 4, the negative anomalies in SLH flux over the land areas can be attributed to the variations in both the latent heat flux over the coastal areas and the sensible heat flux over the northern parts of East Asia and coastal areas (Fig. S7).
6.5 A brief summary for the contributions from different physical processes
Comparing the four different physical processes that modulate the variations in surface temperature over land areas of East Asia (Figs. 12, 13), it can be concluded that: (1) Under all the regimes, the downward IR flux and the horizontal temperature advection always drive cooling effects over land areas during the cold events. (2) Under all the regimes except regime 4, the downward IR flux and horizontal temperature advection play the dominant and secondary roles in the energy budget, respectively. In addition, under regimes 1–3, the adiabatic process and the SLH flux act to warm the land surface, but their contributions are less important. (3) Under regime 4, all four physical processes act to cool the land surface. However, the contributions of the SLH flux and the horizontal temperature advection are dominant and comparable, while the contributions by the downward IR flux and the adiabatic cooling are of secondary importance. The southernmost location of the cooling center distinguishes regime 4 from all other regimes.
7 Summary and concluding remarks
In this study, 103 wintertime cold events over East Asia are identified during the winters of 1948–2014. By applying the AWPC-based k-means clustering method to the HGT anomalies on the 500 hPa surface associated with these cold events, four cold event regimes are recognized and the possible mechanisms and earliest precursor signals are explored. Based on the earliest precursors, half of the regimes correspond to anticyclonic anomalies that develop over the Eurasian continent, while the other half correspond to cyclonic anomalies that develop over the Eurasian continent.
The two regimes with anticyclonic precursor are closely related to blocking highs around the Ural Mountains (regime 1) and central Siberia (regime 2). These two regimes tend to last for longer time periods due to the quasi-stationary nature of the blocking high. However, the presence of a blocking high does not necessarily mean a long lead time between the earliest precursor signal and the onset of the cold event in East Asia. The blocking-high precursor signal for regime 1 can be observed about 16 days prior to the onset of the cold events, but the one for regime 2 only becomes significant roughly 4 days prior to the onset of the cold event. This is the shortest lead time among all four regimes and can be mainly attributed to the close proximity of the blocking location that leads to a short invasion path for the cold air downstream of the blocking high.
The cyclonic precursor anomalies for regimes 3 and 4 trigger the East Asian cold events by intensifying the EAT. The lifetimes of these two regimes are shorter than those associated with the anticyclonic precursors. In particular, cold events belonging to regime 4 have the shortest lifetimes among all regimes, due mainly to the influence of an anticyclonic anomaly over the North Pacific Ocean. However, while their lifetimes are shorter, the lead times of the earliest precursor signals for these two regimes are relatively long. Specifically, the appearances of the precursor signals for regimes 3 and 4 can be observed over the Caspian Sea and Lake Balkhash with lead times of 16 and 18 days, respectively.
All four cold event regimes are closely related to the large-scale circulation modes. Although the cold events seem to have no specific preference for the phase of the NAO, the NAO plays an important role in the generation and/or amplification of the circulation patterns favorable to the generation of cold events in each regime. For regime 1, the NAO\(^{+}\) plays an essential role in initiating the earliest precursor signal, which gradually develops into a Ural blocking-like circulation pattern that subsequently triggers the cold events over East Asia. For regimes 2–4, the significant NAO-related circulation patterns appear after the appearance of the earliest precursor signals. However, the NAO makes a significant contribution to the amplification of the precursor signals for these three regimes. It should be noted that many previous studies have reported that the Arctic Oscillation (AO) plays an important role in the variability of the East Asian winter monsoon, including temperature anomalies over East Asia (Gong et al. 2001; Wu and Wang 2002; Chen et al. 2005, 2013; Jeong and Ho 2005; Chen and Kang 2006; Suo et al. 2009; Huang et al. 2017b). Hurrell (2003), Vallis et al. (2004) and Wang et al. (2017) have shown that the NAO can be viewed as the regional manifestation of the AO over the North Atlantic Ocean. Therefore, our finding that NAO variability contributes to the formation of cold events partially reflects this relationship between the AO and temperature anomalies over East Asia. In this study, we emphasize the role of the NAO rather than that of the AO because the wave activity fluxes that initiate or amplify the earliest precursors of the four identified regimes emanate from the North Atlantic region. All four regimes tend to occur during EU\(^{+}\) and ENSO\(^{-}\). In particular, the ENSO\(^{-}\) can induce an anticyclonic anomaly over the North Pacific Ocean through the PNA teleconnection pattern, which leads to the southward displacement of the cooling center and inhibits the maintenance of the cold events over East Asia.
Under regimes 1–3, the downward IR flux and the horizontal cold advection play the dominant and secondary roles in surface cooling, respectively. By contrast, under regime 4, all physical processes are important in cooling East Asian land surface. Due to the presence of the anticyclonic anomaly over the North Pacific Ocean during regime 4, the loss of the sensible and latent heat from the land surface and the horizontal cold advection primarily act to modulate the cooling over East Asia.
As mentioned in Sect. 1, previous studies have examined the circulation patterns related to the East Asian cold events. These studies have emphasized the influences of Ural blocking (Park et al. 2014; Luo et al. 2016a, b), central Siberian blocking (Lee and Jhun 2006; Huang et al. 2017a), the NAO (Luo et al. 2016a, b), and the EAT (Joung and Hitchman 1982; Zhang et al. 1997). The following aspects distinguish our work from these previous studies. First, we examine four different types of cold events identified via a unified framework that applies the AWPC-based k-means clustering method to the geopotential height anomalies associated with each cold event. Our use of this unified framework enables a systematic and objective evaluation of characteristics that may differ among different types of cold events, including the earliest precursors, the lead times associated with these earliest precursors, and the causes for surface cooling based on heat budget analyses. By contrast, previous studies have only identified and examined one or at most two types of East Asian cold events. Moreover, most of these studies have focused on the role of a specific phenomenon (e.g., blocking highs, the NAO, or the EAT) on East Asian cold events, whereas our approach facilitates a more comprehensive analysis. Second, the remarkable reduction in lead time (16 days to 4 days) for the earliest precursor associated with central Siberian blocking-related cold events relative to Ural blocking-related cold events has never been reported. This finding benefits understanding of the relationships between upstream blocking activities and East Asian wintertime climate. Third, while Luo et al. (2016a, b) have emphasized the essential role played by the NAO during the formation of Ural blocking-related cold events, our results reveal that the NAO plays important roles in the development of central Siberian blocking-related and cyclonic anomaly-related cold events as well. Fourth, we have identified and analyzed a separate class of cold events centered over southern China in this study, whereas previous studies have focused almost exclusively on cold events centered over Mongolia or northern China. The relative abundance of moisture in the atmosphere above southern China means that cold surges in this region are conducive to the occurrence of the extreme rainfall or snowfall. Our explicit examination of this type of cold event is thus helpful for understanding and potentially predicting the occurrence of extreme wintertime precipitation in this region.
References
Barnes EA, Screen JA (2015) The impact of Arctic warming on the midlatitude jet-stream: Can it? Has it? Will it? Wiley Interdiscip Rev Clim Change 6(3):277–286. doi:10.1002/wcc.337
Barnston AG, Livezey RE (1987) Classification, seasonality and persistence of low-frequency atmospheric circulation patterns. Mon Weather Rev 115(6):1083–1126. doi:10.1175/1520-0493(1987)115<1083:CSAPOL>2.0.CO;2
Chen TC, Huang WR, Yoon Jh (2004) Interannual variation of the East Asian cold surge activity. J Clim 17(2):401–413. doi:10.1175/1520-0442(2004)017<0401:IVOTEA>2.0.CO;2
Chen W, Kang L (2006) Linkage between the Arctic Oscillation and winter climate over East Asia on the interannual timescale: roles of quasi-stationary planetary waves. Chin J Atmos Sci 30:863–870 (in Chinese)
Chen W, Graf HF, Huang R (2000) The interannual variability of East Asian winter monsoon and its relation to the summer monsoon. Adv Atmos Sci 17(1):48–60. doi:10.1007/s00376-000-0042-5
Chen W, Yang S, Huang RH (2005) Relationship between stationary planetary wave activity and the East Asian winter monsoon. J Geophys Res Atmos. doi:10.1029/2004JD005669
Chen W, Lan X, Wang L, Ma Y (2013) The combined effects of the ENSO and the Arctic Oscillation on the winter climate anomalies in East Asia. Chin Sci Bull 58(12):1355–1362. doi:10.1007/s11434-012-5654-5
Cheung HHN, Zhou W (2016) Simple metrics for representing East Asian winter monsoon variability: Urals blocking and western Pacific teleconnection patterns. Adv Atmos Sci 33(6):695–705. doi:10.1007/s00376-015-5204-6
Cheung HHN, Zhou W, Lee SM, Tong HW (2015) Interannual and interdecadal variability of the number of cold days in Hong Kong and their relationship with large-scale circulation. Mon Weather Rev 143(4):1438–1454. doi:10.1175/MWR-D-14-00335.1
Cheung HN, Zhou W, Shao Y, Chen W, Mok HY, Wu MC (2013) Observational climatology and characteristics of wintertime atmospheric blocking over Ural–Siberia. Clim Dyn 41(1):63–79. doi:10.1007/s00382-012-1587-6
Diao Y, Li J, Luo D (2006) A new blocking index and its application: blocking action in the northern hemisphere. J Clim 19(19):4819–4839. doi:10.1175/JCLI3886.1
Ding Y, Krishnamurti TN (1987) Heat budget of the Siberian high and the winter monsoon. Mon Weather Rev 115(10):2428–2449. doi:10.1175/1520-0493(1987)115<2428:HBOTSH>2.0.CO;2
Gong D, Wang S, Zhu J (2001) East Asian winter monsoon and Arctic Oscillation. Geophys Res Lett 28(10):2073–2076. doi:10.1029/2000GL012311
Hoerling MP, Kumar A, Zhong M (1997) El Niño, La Niña, and the nonlinearity of their teleconnections. J Clim 10(8):1769–1786. doi:10.1175/1520-0442(1997)010<1769:ENOLNA>2.0.CO;2
Huang W, Chen R, Wang B, Wright JS, Yang Z, Ma W (2017a) Potential vorticity regimes over East Asia during winter. J Geophys Res Atmos 122(3):1524–1544. doi:10.1002/2016JD025893
Huang W, Chen R, Yang Z, Wang B, Ma W (2017b) Exploring the combined effects of the Arctic Oscillation and ENSO on the wintertime climate over East Asia using self-organizing maps. J Geophys Res Atmos. doi:10.1002/2017JD026812
Huang W, Wang B, Wright JS (2016a) A potential vorticity-based index for the East Asian winter monsoon. J Geophys Res Atmos 121(16):9382–9399. doi:10.1002/2016JD025053
Huang W, Wang B, Wright JS, Chen R (2016b) On the non-stationary relationship between the Siberian High and Arctic Oscillation. PLoS One. doi:10.1371/journal.pone.0158122
Hurrell JW (1995) Decadal trends in the North Atlantic oscillation: regional temperatures and precipitation. Science 269(5224):676–679. doi:10.1126/science.269.5224.676
Hurrell JW (2003) Climate variability: North Atlantic and Arctic Oscillation. In: Holton JR (ed) Encyclopedia of atmospheric sciences. Academic Press, Cambridge, pp 439–445. doi:10.1016/B0-12-227090-8/00109-3
Jeong JH, Ho CH (2005) Changes in occurrence of cold surges over East Asia in association with Arctic Oscillation. Geophys Res Lett. doi:10.1029/2005GL023024
Jeong JH, Kim BM, Ho CH, Chen D, Lim GH (2006) Stratospheric origin of cold surge occurrence in East Asia. Geophys Res Lett. doi:10.1029/2006GL026607
Joung CH, Hitchman MH (1982) On the role of successive downstream development in East Asian polar air outbreaks. Mon Weather Rev 110(9):1224–1237. doi:10.1175/1520-0493(1982)110<1224:OTROSD>2.0.CO;2
Kalnay E, Kanamitsu M, Kistler R, Collins W, Deaven D, Gandin L, Iredell M, Saha S, White G, Woollen J, Zhu Y, Leetmaa A, Reynolds R, Chelliah M, Ebisuzaki W, Higgins W, Janowiak J, Mo KC, Ropelewski C, Wang J, Jenne R, Joseph D (1996) The NCEP/NCAR 40-year reanalysis project. Bull Am Meteorol Soc 77(3):437–471. doi:10.1175/1520-0477(1996)077<0437:TNYRP>2.0.CO;2
Kohonen T (1989) Self-organization and associative memory. Springer, Berlin
Lau KM, Li MT (1984) The monsoon of East Asia and its global associations—a survey. Bull Am Meteorol Soc 65(2):114–125. doi:10.1175/1520-0477(1984)065<0114:TMOEAA>2.0.CO;2
Lee HS, Jhun JG (2006) Two types of the Asian continental blocking and their relation to the east Asian monsoon during the boreal winter. Geophys Res Lett. doi:10.1029/2006GL027948
Lim YK, Kim HD (2016) Comparison of the impact of the Arctic Oscillation and Eurasian teleconnection on interannual variation in East Asian winter temperatures and monsoon. Theor Appl Climatol 124(1):267–279. doi:10.1007/s00704-015-1418-x
Liu Y, Wang L, Zhou W, Chen W (2014) Three Eurasian teleconnection patterns: spatial structures, temporal variability, and associated winter climate anomalies. Clim Dyn 42(11):2817–2839. doi:10.1007/s00382-014-2163-z
Lloyd S (1982) Least squares quantization in PCM. IEEE Trans Inf Theory 28(2):129–137. doi:10.1109/TIT.1982.1056489
Luo D, Lupo AR, Wan H (2007) Dynamics of Eddy-driven low-frequency dipole modes. Part I: A simple model of North Atlantic Oscillations. J Atmos Sci 64(1):3–28. doi:10.1175/JAS3818.1
Luo D, Xiao Y, Yao Y, Dai A, Simmonds I, Franzke CLE (2016a) Impact of Ural blocking on winter warm Arctic–Cold Eurasian anomalies. Part I: Blocking-induced amplification. J Clim 29(11):3925–3947. doi:10.1175/JCLI-D-15-0611.1
Luo D, Xiao Y, Diao Y, Dai A, Franzke CLE, Simmonds I (2016b) Impact of Ural blocking on winter warm Arctic–Cold Eurasian anomalies. Part II: The link to the North Atlantic oscillation. J Clim 29(11):3949–3971. doi:10.1175/JCLI-D-15-0612.1
Nath D, Chen W, Wang L, Ma Y (2014) Planetary wave reflection and its impact on tropospheric cold weather over Asia during January 2008. Adv Atmos Sci 31(4):851–862. doi:10.1007/s00376-013-3195-8
Nath D, Chen W, Zelin C, Pogoreltsev AI, Wei K (2016) Dynamics of 2013 sudden stratospheric warming event and its impact on cold weather over Eurasia: role of planetary wave reflection. Sci Rep. doi:10.1038/srep24174
Park TW, Ho CH, Yang S (2011) Relationship between the Arctic Oscillation and cold surges over East Asia. J Clim 24(1):68–83. doi:10.1175/2010JCLI3529.1
Park TW, Ho CH, Deng Y (2014) A synoptic and dynamical characterization of wave-train and blocking cold surge over East Asia. Clim Dyn 43(3):753–770. doi:10.1007/s00382-013-1817-6
Rayner NA, Parker DE, Horton EB, Folland CK, Alexander LV, Rowell DP, Kent EC, Kaplan A (2003) Global analyses of sea surface temperature, sea ice, and night marine air temperature since the late nineteenth century. J Geophys Res Atmos. doi:10.1029/2002JD002670
Renwick JA, Wallace JM (1996) Relationships between North Pacific wintertime blocking, El Niño, and the PNA pattern. Mon Weather Rev 124(9):2071–2076. doi:10.1175/1520-0493(1996)124<2071:RBNPWB>2.0.CO;2
Sung MK, Lim GH, Kwon WT, Boo KO, Kug JS (2009) Short-term variation of Eurasian pattern and its relation to winter weather over East Asia. Int J Climatol 29(5):771–775. doi:10.1002/joc.1774
Suo L, Tan B, Huang J (2009) Further exploration on causes of temperature anomalies associated with the abnormal northern annular mode. Chin Sci Bull 54(12):2101–2106. doi:10.1007/s11434-009-0045-2
Takaya K, Nakamura H (2001) A formulation of a phase-independent wave-activity flux for stationary and migratory quasigeostrophic eddies on a zonally varying basic flow. J Atmos Sci 58(6):608–627. doi:10.1175/1520-0469(2001)058<0608:AFOAPI>2.0.CO;2
Takaya K, Nakamura H (2005a) Mechanisms of intraseasonal amplification of the cold Siberian high. J Atmos Sci 62(12):4423–4440. doi:10.1175/JAS3629.1
Takaya K, Nakamura H (2005b) Geographical dependence of upper-level blocking formation associated with intraseasonal amplification of the Siberian high. J Atmos Sci 62(12):4441–4449. doi:10.1175/JAS3628.1
Vallis GK, Gerber EP, Kushner PJ, Cash BA (2004) A mechanism and simple dynamical model of the North Atlantic oscillation and annular modes. J Atmos Sci 61(3):264–280. doi:10.1175/1520-0469(2004)061<0264:AMASDM>2.0.CO;2
Wallace JM, Gutzler DS (1981) Teleconnections in the geopotential height field during the Northern Hemisphere winter. Mon Weather Rev 109(4):784–812. doi:10.1175/1520-0493(1981)109<0784:TITGHF>2.0.CO;2
Wang B, Wu R, Fu X (2000) Pacific–East Asian teleconnection: how does ENSO affect East Asian climate? J Clim 13(9):1517–1536. doi:10.1175/1520-0442(2000)013<1517:PEATHD>2.0.CO;2
Wang H, He S (2012) Weakening relationship between East Asian winter monsoon and ENSO after mid-1970s. Chin Sci Bull 57(27):3535–3540. doi:10.1007/s11434-012-5285-x
Wang L, Huang R, Gu L, Chen W, Kang L (2009) Interdecadal variations of the East Asian winter monsoon and their association with quasi-stationary planetary wave activity. J Clim 22(18):4860–4872. doi:10.1175/2009JCLI2973.1
Wang L, Ting M, Kushner PJ (2017) A robust empirical seasonal prediction of winter NAO and surface climate. Sci Rep 7(1):279. doi:10.1038/s41598-017-00353-y
Watanabe M, Nitta T (1999) Decadal changes in the atmospheric circulation and associated surface climate variations in the northern hemisphere winter. J Clim 12(2):494–510. doi:10.1175/1520-0442(1999)012<0494:DCITAC>2.0.CO;2
Wei K, Chen W, Zhou W (2011) Changes in the East Asian cold season since 2000. Adv Atmos Sci 28(1):69–79. doi:10.1007/s00376-010-9232-y
Woo SH, Kim BM, Jeong JH, Kim SJ, Lim GH (2012) Decadal changes in surface air temperature variability and cold surge characteristics over northeast Asia and their relation with the Arctic Oscillation for the past three decades (1979–2011). J Geophys Res Atmos. doi:10.1029/2011JD016929
Wu B, Wang J (2002) Winter Arctic Oscillation, Siberian high and East Asian winter monsoon. Geophys Res Lett 29(19):3-1–3-4. doi:10.1029/2002GL015373
Wu MC, Leung WH (2009) Effect of ENSO on the Hong Kong winter season. Atmos Sci Let 10(2):94–101. doi:10.1002/asl.215
Yanai M, Tomita T (1998) Seasonal and interannual variability of atmospheric heat sources and moisture sinks as determined from NCEP–NCAR reanalysis. J Clim 11(3):463–482. doi:10.1175/1520-0442(1998)011<0463:SAIVOA>2.0.CO;2
Yanai M, Esbensen S, Chu JH (1973) Determination of bulk properties of tropical cloud clusters from large-scale heat and moisture budgets. J Atmos Sci 30(4):611–627. doi:10.1175/1520-0469(1973)030<0611:DOBPOT>2.0.CO;2
Yao Y, Luo D, Dai A, Simmonds I (2017) Increased quasi stationarity and persistence of winter Ural blocking and Eurasian extreme cold events in response to Arctic warming. Part I: insights from observational analyses. J Clim 30(10):3549–3568. doi:10.1175/JCLI-D-16-0261.1
Zhang Y, Sperber KR, Boyle JS (1997) Climatology and interannual variation of the East Asian winter monsoon: results from the 1979–95 NCEP/NCAR reanalysis. Mon Weather Rev 125(10):2605–2619. doi:10.1175/1520-0493(1997)125<2605:CAIVOT>2.0.CO;2
Acknowledgements
We thank two anonymous reviewers for comments and suggestions that have substantially improved the manuscript. This work was jointly supported by the National Basic Research Program of China (2015CB953703, 2014CB441302), the National Natural Science Foundation of China (41505063), and the China Meteorological Welfare Research Fund (GYHY201406007).
Author information
Authors and Affiliations
Corresponding author
Electronic supplementary material
Below is the link to the electronic supplementary material.
Rights and permissions
About this article
Cite this article
Yang, Z., Huang, W., Wang, B. et al. Possible mechanisms for four regimes associated with cold events over East Asia. Clim Dyn 51, 35–56 (2018). https://doi.org/10.1007/s00382-017-3905-5
Received:
Accepted:
Published:
Issue Date:
DOI: https://doi.org/10.1007/s00382-017-3905-5