Abstract
Turbulent plumes are fascinating to study in large part due to the ability to see the eddies and structures that comprise the exterior structure as they develop in space and time. We perform a laboratory study in which positively buoyant turbulent plumes are generated in a quiescent water tank. Buoyancy is varied by modifying the relative percentages of isopropyl alcohol to water in a mixture placed in a head tank. Photographs captured at steady frame rates record the evolution of the plume as it develops in time and space. A custom algorithm tracks the visible exterior outline of the plume, from which eddies and structures can be identified along the interface between the plume fluid and ambient fluid. Statistical analyses are performed to characterize differences in the distributions of external structures to study their dependence on relative buoyancy between the fluids. Spectral analysis of the edge signal of the plume reveals a \(-\)2.2 slope, indicative of the range of eddy lengths that comprise turbulent plumes. We explore the relationship between buoyancy with both the plume front velocity and plume spread angle. We find the front velocities to be functions of both the buoyancy and source Reynolds number. However, the spread angles were found to vary only with buoyancy of the plumes, thus proportional to their Richardson numbers.
Similar content being viewed by others
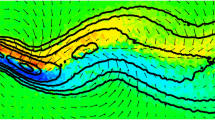
Avoid common mistakes on your manuscript.
1 Introduction
1.1 Motivation
From hydrothermal vents at the ocean floor to chimney smokestacks in the atmosphere, turbulent plumes are found in many natural and industrial scenarios. Understanding the transport mechanisms of plumes is important in hazard management, especially in situations such as underwater oil blowout wells or volcanic ash cloud eruptions. Using the volcanic ash cloud as an example, detailed in situ velocity and concentration measurements of the ash cloud are often prohibitively difficult to collect. Thus, real-time predictions of the fate of the ash remain particularly elusive. By contrast, video recordings in the field can be readily accessible. While field videos may not necessarily show fine scale transport and mixing, they can clearly show large-scale structures (e.g., eddies, billows, cauliform structures) that comprise the exterior structure of the plume, examples of which are shown in Fig. 1.
Cauliform eddy length identification for Mount St. Helens eruption, reproduced from Andrews and Gardner (2009)
Numerous scientists have explored visual large-scale structures of plumes in order to obtain detailed information regarding plume behavior and characteristics. For example, Kitamura and Sumita (2011) used the change in shape and regime on-set times to constrain the buoyancy of the plume, Chojnicki et al. (2014) correlated the morphology of buoyant jets with sources of momentum flux, and Clarke et al. (2009) estimated the erupted mass using video analysis of plume front velocity. We aim to add to this literature by investigating the size distribution of the visual structures comprising the plume exterior. We explore correlations between the dimensions of these external features with the turbulence and buoyancy that drive entrainment and structure formation from the source. By determining relationships linking plume source conditions with their resultant physical structures, we will be able to inform improvements to models that predict fate and transport of natural and anthropogenic plumes from remote photographic or video recordings.
1.2 Background
A vast literature has been established on the physics of plumes (e.g., Kuethe 1935; Rouse et al. 1952; Batchelor 1954; Hoult et al. 1969; Turner 1979; Matulka et al. 2014), buoyant jets (e.g., Rodi 1982; Chojnicki et al. 2014), particle-laden jets (e.g., Shannon et al. 2020), particle-laden plumes (e.g., McConnochie and Cenedese 2023; ODonnell et al. 2024), and thermals (e.g., Turner 1969), in which a fluid either rises due to positive buoyancy (i.e., low-density plume), or sinks due to negative buoyancy (i.e., high density plume), relative to the surrounding fluid. As buoyant fluid rises, shear stress is generated between the plume and ambient. This leads to the development of turbulence, seen as the formation of eddies, or rotating parcels of fluid of varying size and velocity. These eddies can nibble or engulf ambient fluid, thereby entraining relatively quiescent fluid (assuming a stagnant ambient) into the core of the plume (Corrsin and Kistler 1955; Westerweel et al. 2005; Philip and Marusic 2012) and reducing its velocity. Subsequently, the concentration gradient between the plume and ambient is reduced, and the plume spreads laterally, often forming a Gaussian-like distribution of concentration and velocity in the radial direction (inter alia, Turner 1986). Key takeaways from prior studies include the notion that plumes entrain ambient fluid more efficiently than their counterpart momentum-driven jets do (Kaminski et al. 2005; van Reeuwijk and Craske 2015; Saeed et al. 2022), and that the mechanisms that provide this efficient means of transport and mixing rely heavily on interfacial turbulent structures.
Starting plumes, or plumes initiating from zero flux, have seen considerable laboratory and field attention, as they are adequate models for many natural and industrial events including volcanic eruptions and underwater oil well blowouts. In early studies, Turner (1962) determined the ratio of the front velocity for a starting plume to the velocity of a steady plume at corresponding heights to be approximately equal to 0.61. This finding is supported by Scase (2009), who measured a front velocity ratio of 0.65, and Bhamidipati and Woods (2017), with a value of 0.63, providing a means for considering plume source or outlet dynamics from bulk parameters. The shape and spread of plumes provide insight, albeit limited and imperfect, into the interior driving dynamics as well as ambient stratification (Turner 1962; Sparks and Wilson 1982; Sparks 1986). Using quantitative imaging techniques to look at the coarse outline of a distant ash cloud in the field, Sparks and Wilson (1982) showed how the mass flux through a volcano vent could be inferred from sequential photographs of a starting plume. Models exist to approximate the volume of ash clouds, based on two-dimensional images of eruptions from which the overall plume outline, cloud color, and other features are utilized to deduce information about the bulk dynamics of the plume (Sparks and Wilson 1982).
In ash clouds, there is typically a stark visual contrast between the plume and ambient. As a result, turbulent eddies comprising the plume structure can be distinguished through optical techniques. Using video recordings and photographs of the 1980 Mount St. Helens eruption, Andrews and Gardner (2009) used quantitative imaging techniques with feature tracking of image series to measure the turbulent velocity field of the plume exterior during its rise. Due to shadows and bulges highlighting cauliform structures of turbulent eddies, there was sufficient variation in light intensity to spatially resolve the flow field at different times and locations within the eruption. Andrews and Gardner (2009) visually identified cauliform structures of the front face (i.e., camera side) of the plume, appearing in photographs as large clusters of turbulent eddies comprising the plume exterior. The horizontal lengths of these structures are shown in Fig. 1, indicated by black and white lines superimposed on the photographs. It was found that these length scales were aligned with the vent size and boundary layer formation, the latter of which depends upon eruption velocity conditions, shear stress between the plume and ambient, and phase of plume evolution.
To obtain information on detailed characteristics of plumes, laboratory researchers have leveraged different measurement techniques to understand the relationship between the structural evolution and transport processes of a plume. Through the use of a dye technique, Kitamura and Sumita (2011) categorized the shape evolution of a buoyant plume. Using different fluid density as the main driver for the buoyancy flux, Kitamura and Sumita (2011) observed the evolution of the plume from a finger-like shape to a plume head that later transformed into a cone-like self-similar shape. The self-similarity is a result of the entrainment parameter becoming time independent. Rogers and Morris (2009) followed a similar approach of using a density difference to form a starting plume. However, they used shadowgraphy in addition to the dye technique to visualize the plume and analyze the dependence of the plume structure on the plume Richardson number (Ri) based on front velocity \(U_F\). Their study reports that the plume head assumes a confined shape or a dispersed shape depending on whether \(Ri > 1\) or \(Ri < 1\), respectively.
Clarke et al. (2009) leveraged a dye visualization technique to conduct front velocity conventional analysis. By tracking the front of a plume produced by injecting a buoyant mixture via a pump into a freshwater tank, they determined a relationship between the flow front propagation and the vent conditions. This relationship allowed for estimates of two important hazard management parameters for volcanic events: total mass erupted and vent mass flux as a function of time. When measured against mass estimates obtained via independent fieldwork, values obtained using the Clarke et al. (2009) relationship compare favorably. This is significant because it means that the evidence needed to categorize the buoyancy and momentum released for impulsive events can be found through the front displacement. Impulsive events include thermals (releases where buoyancy drives the flow), puffs (releases where momentum drives the flow), and releases driven by both buoyancy and momentum (Clarke et al. 2009).
Instead of tracking the displacement of a single point as a proxy to determine the velocity of the plume, as is the case in front velocity video analysis, particle image velocimetry (PIV; Adrian 1991) allows for determination of the instantaneous velocity measurements of a cross section through an entire plume and body of fluid in the laboratory. Chojnicki et al. (2014) employed PIV in their jet (i.e., “momentum-driven plume”) research to develop a simultaneous understanding of the visible plume boundary, structure and velocity evolution through time. When analyzing the instantaneous velocity fields, they observed large vortex structures that were present throughout the evolution of the plume. These structures subsequently influenced the shape profile of the plume. The vortices became less evident when the instantaneous velocity field was averaged temporally, suggesting that the use of an average velocity leads to a loss of information that could be useful in understanding the transport mechanisms within the plume, and thereby emphasizing the critical role of turbulence as an indicator of plume behavior.
Krug et al. (2014) and Funatani et al. (2004) have used variations of simultaneous PIV and laser induced fluorescence (LIF) to explore the interaction between turbulence, entrainment, and mixing. Specifically, Funatani et al. (2004) recreate a temperature driven buoyancy-flux plume and use simultaneous two-color LIF and PIV to monitor the temperature and velocity distributions, respectively. The temperature distribution is then used as a proxy to determine the presence of entrainment processes in the plume. Effectively, what they observe is a decrease in temperature as the plume width grows with increasing distance from the outlet, demonstrating entrainment of the colder ambient fluid into the plume. Additionally, they noticed the velocity field of the plume increasing in width with distance from the nozzle.
Instantaneous shadowgraph images (a, b) of a dense saline plume; average image intensity (c). Red and green lines (panel a) denote mean width and fluctuation scale, respectively; width measurements denoted in panel b; time-average plume with and standard deviation denoted by red and blue lines, respectively, in panel c. Reproduced from Burridge et al. (2016)
A laboratory study of Burridge et al. (2016) considered the relationship between coherent structures of a steady dense falling saline turbulent plume edge with the bulk transport (i.e., mass, momentum, buoyancy) of the plume. Using fluctuating plume width as a metric of the physical turbulent structure of the plume (Fig. 2), correlations were determined linking visual features of the plume outline with measured entrainment and transport dynamics of known source conditions. This work used shadowgraphy to determine velocities of the plume using feature tracking of coherent structures at the outer edge of the plume. Subsequent laboratory experiments of Bhamidipati and Woods (2017) explored mechanisms by which starting plumes develop from zero flux toward steady state, and in particular, how buoyancy varies in the plume head as opposed to the trailing body through the use of colored dyes in optical measurements. Thus, spatiotemporal records of starting plumes can be used to infer steady-state conditions of the bulk velocity and propagation of the plume.
Recently, Ibarra et al. (2020) employed a combination of direct flow visualization, schlieren photography, shadowgraph photography, and PIV to show that an opaque jet flow can be gauged using visible interface features near the jet exit. They classify the visible turbulence structures as edge curve segments and perform discrete Fourier transformations to convert their lengths to a measure of magnitude with respect to the wavenumber of their occurrence. Plotting the non-dimensionalized curvatures against the non-dimensionalized wavenumbers, they found that segments with the highest amplitudes occur at lower wavenumbers, and these dominant amplitudes fall off at a rate of − 7/3. At higher wavenumbers, the amplitudes are smaller and fall off at a rate of − 5/3, thus showing that the smaller structures are decaying at a faster rate than their larger counterparts.
1.3 Objectives
The main objectives of this research were to develop a methodology for quantifying the distributions and characteristics of the coherent structures that are visible at the margins of a plume and to correlate the distributions of exterior physical structures with the buoyancy conditions of the plume. Whereas the range of flow and buoyancy conditions explored in the present study are relatively narrow, it is our aim that insights obtained from this research can be used to leverage remotely acquired videos or photographs as a means of inferring mass transport and entrainment for natural and anthropogenic disasters.
We performed a series of laboratory experiments in which buoyant plumes were generated in a tank of quiescent water; experimental methods and data collection techniques are presented in Sect. 2. Quantitative imaging techniques were used to identify and quantify the structures that comprise the boundary outlining the visible exterior of the plume, as presented in Sect. 3. We present quantification of front velocity, spread angle, and spectra of the plume shape in Sect. 3, and we conclude our findings in Sect. 4.
2 Experimental facility
2.1 Apparatus
The experimental facility is located at the Johnson Environmental Turbulence Laboratory (JETlab) at The University of Texas at Austin Center for Water and the Environment. The apparatus (Fig. 3) consists of a water tank with an 84.0 cm x 84.0 cm square base, with a 100.0 cm height. The water tank is filled to a depth of 63 cm with tap water of approximately \(20^\circ\)C. A cylindrical head tank with diameter 35.5 cm sits atop the main water tank. The head tank is filled to a height of approximately 15 cm with a buoyant water-alcohol mixture and is placed above the water tank.
The buoyant fluid in the head tank consists of mixtures of tap water, 91% isopropyl alcohol for buoyancy, fluorescein salt for illumination, and AGSCO Corporation silica flour with median diameter \(d_{50}=0.252\) μm for opacity. By volume, the percentage of isopropyl alcohol to water ranged from 10 % to 30 % across tests. The temperature, \(T_p\), of the buoyant fluid is approximately 40 °C. The density of the buoyant fluid mixture, \(\rho _p\), was determined directly in the laboratory while measuring the ingredients, and the viscosity, \(\mu _p\), of the mixture was measured using a Hydramotion Viscolite 700 Portable Viscometer. Three different buoyant solutions were tested, as summarized in Table 1. The relative dimensionless buoyancy between the plume and ambient fluids is defined as \((\rho _p - \rho _a)/\rho _a\), where \(\rho _a\) is the density of the ambient water at \(20^\circ \,\hbox {C}\). Values of relative dimensionless buoyancy range from 2.5 to 6.7 %.
Buoyant fluid was released from the head tank with a manual spigot and subsequently through a 0.0125 m inner diameter (\(d_{0}\)) clear PVC pipe. The pipe was placed in the tank corner and directed the fluid across the tank base to its vertical opening, centered laterally, as depicted in Fig. 3. The pipe outlet through which plume fluid enters the water tank is positioned at a height, \(h_0\), equal to 9.5 cm. Thus, the plume was generated by a combination of buoyancy (i.e., due to the reduced density via alcohol and temperature gradient, relative to the ambient) and head (i.e., placed above the free surface within the water tank). For each of the three buoyancy conditions, 10 replicate experiments were performed. However, some experiments had to be discarded due to inconsistency in data collection, leaving 7 to 8 good trials for each buoyancy condition. For each test, buoyant fluid was released for approximately 20 s. The coordinate system is shown in Fig. 3, with (x, y, z) = (0, 0, 0) at the outlet, with x pointing upward and y and z pointing laterally (or radially) outward from the centerline. Velocity components U, V, and W follow the x, y, and z directions, respectively.
2.2 Measurement techniques
Photographs were recorded at steady frame rates to capture the structural evolution of the plume exterior (Fig. 4). Black lights were mounted externally surrounding the tank, in order for the buoyant plume mixture to sufficiently fluoresce. This allowed a detailed look at the texture (i.e., cauliform structures, eddies, billows) that comprise the outermost visible edge of the plume. Images of the plume were recorded using an iPhone 13 Pro with the Skyflow Time-lapse Shooting application with frame rates of 9, 12, and 16 frames per second in different trials, with a spatial resolution of 3840 pixels by 2160 pixels. Images were recorded over a duration of 5 s in a 58 cm \(\times\) 32 cm measurement region. Spatial calibration of pixels to physical dimensions was completed by taking a photograph of a ruler positioned in the vertical central plane of the plume, and calibrating pixel distances accordingly.
2.3 Identification of Eddy Lengths
Various algorithms have been developed by researchers to track and analyze plume evolution (e.g., Valade et al. 2014; Bombrun et al. 2018). These algorithms focus primarily on following the development of parameters that characterize plume evolution such as plume height, velocity, acceleration, spreading rate, entrainment coefficients, and fine-particle loading. By contrast, the purpose of the algorithm and MATLAB code developed herein is to infer the size distribution of the bulging structures at the margins of the plume over time and space. This is achieved by performing image analysis on the outer edges of the plume. After detecting the edges of the plume, the algorithm uses the edge as a signal to find the sizes of the structures. The sizes of the structures are determined by calculating the distance between adjacent peaks in the signal.
The algorithm for detecting and characterizing eddy lengths comprising the outer edge of the plume begins by cropping the plume images to manually assigned dimensions. This splits the plume into two halves, left and right, enabling independent analysis of each half. Cropping the images also improves the analysis as the field of view is narrowed to focus on the plume. Next, the images are rotated to align the plume edge vertically to facilitate subsequent analysis. The rotated images are assigned a coordinate system with \(x'\) in the vertical direction and \(y'\) in the horizontal direction.
The images are then converted to grayscale and smoothed slightly using the medfilt2 and wiener2 functions in MATLAB to remove additional noise. The graythresh function is used to maximize the contrast of the images (Fig. 5a). This function automatically determines the brightness threshold which is used to binarize the image. However, slight adjustments (i.e., up to 20 %) in the thresholds are made manually to improve accuracy of the extracted outline. The plume edges are binarized (Fig. 5b), and small features around and within the plume are removed using a sequence including the bwareopen, strel, and imclose functions to clean up the edge signal. The boundaries of the plume are then identified using the bwboundaries MATLAB function (Fig. 5.c). The identified points corresponding to the boundary often include several “overlapping” or “non-unique” coordinates in the sense that there are multiple values of x’ for a particular y’. To determine the final edge coordinates, only the first instance of such x’ values were considered. A sample set of points outlining a plume edge is shown in green in Fig. 5.d.
Once the right and left boundary signals are created, the MATLAB findpeaks function is used to identify peaks (i.e., local maxima in the boundary signal; Fig. 6.a). In order to focus on the more pronounced protruding structures, the peaks identified by MATLAB are filtered to ensure that they are consistent with visual evidence in the photographic images. The MinPeakProminence and MinPeakHeight arguments of the findpeaks function are used to filter out nonsignificant peaks. Furthermore, a threshold value is assigned to ensure a minimum distance between two peaks. The algorithm begins the filtering process by comparing adjacent MATLAB-identified peaks and testing whether both adjacent peaks have a value difference of this threshold (e.g., 25 to 35 pixels). If the value difference is smaller than the threshold, the algorithm considers the adjacent peak to be part of the larger structure and will use the previous peak as the basis of comparison with the following adjacent peak. If the value difference with the adjacent peak is equal to or larger than the threshold, the algorithm considers that peak as the ending point of the present structure and starting point of the next structure. The distance between peaks is considered to be an approximation for the length of the feature (Fig. 6.b). The drawback of using the peaks automatically identified by MATLAB lies in the possibility of those peaks being located in the trough between two protruding structures (Fig. 6.a). This is due to the local maxima criteria embedded in the findpeaks function. Though this may add a degree of uncertainty in the total number of structure lengths for the plume, the consistency of the method experienced across all experiments allows for the observation of trends in the data. The optimum values for the MinPeakProminence, MinPeakHeight, and threshold were assigned manually for each plume outline.
3 Results
3.1 Front velocity analysis
Front velocity, \(U_F\), is determined in the early stages of each test as the plumes initiate their rise through the measurement region. This is accomplished by tracking the uppermost pixels that comprise the plume outline, indicating the plume front. Considering the plume front position as a function of time, an example of which is shown in Fig. 7, we see a linear increase of front position over time within the measurement region, as in Rogers and Morris (2009), and therefore steady front velocity (i.e., slope of plume front position over time). Thus, we report temporally-averaged values of \(U_F\) across all trials in Table 2, along with their standard deviation, \(\sigma _F\) for each record.
To estimate the outlet velocity, we rely on empirical relationships linking front velocity with steady plume velocity (recall, e.g., Bhamidipati and Woods 2017; Scase 2009; Turner 1962), such that \(U_0 = U_F/0.63\). With estimates for outlet velocity, we compute Reynolds and Richardson numbers at the outlet. We determine the Reynolds number as \(Re = \rho \overline{U_0} d_0/\mu\). The Richardson number is defined as \(Ri = g' d_o/\overline{U_0}^2\), where reduced gravity \(g' = g (\rho _a - \rho _p)/\rho _a\), and g is gravitational acceleration. The calculated parameters for the experimental cases are presented in Table 2. All quantities presented in the table are the average metrics from the right and left sides of the plume, except for the spread angle \(\theta _{S}\), which is expressed as the sum of the values from both sides.
The front velocity follows a peculiar trend with increasing alcohol content in the buoyant fluid mixture. The mean front velocity for the 2.5 % relative buoyancy tests (case 1) is lowest at approximately 30 cm/s. The 4.6 % relative buoyancy tests (case 2) produced the highest mean front velocity at approximately 43 cm/s, while it is slightly lower for the 6.7 % relative buoyancy tests (case 3) at around 40 cm/s. For the three cases, Re was evaluated to be approximately 5740, 5830, and 4680, respectively, with increasing buoyancy between cases. We initially expected the velocity (and consequently, Re) for the trials with the greatest relative buoyancy to be maximum, as in Kitamura and Sumita (2011). While a decrease in the density of the plume mixture results in a greater buoyancy gradient, and therefore, likely, a faster rise velocity, the experimental setup is such that the buoyant fluid is stored in a head tank above the facility. As the relative buoyancy increases, the reduction in pressure head suggests \(U_0\) should decrease. Therefore, there is a trade-off in regards to how the plume density and pressure head directly affect the front velocity (and in turn, \(U_0\), Re, and Ri).
From visual observations, the plume initially appears to be relatively smooth upon exiting the pipe. We then find turbulent structures start appearing at the plume edges at distances approximately 2 to 4 cm from the source in all cases, indicating a departure from the smooth vertical flow (Fig. 8). To estimate an approximate value at which this transition occurs, we calculate the distance of the first peak point appearing at a plume edge (e.g., the lowermost point of Fig. 6b) from the start of the plume, as it denotes the start of the first identified turbulent structure. Upon averaging these values for all the trials in a case, we obtain distances of 2.20 cm, 2.25 cm, and 2.22 cm for the 2.5 %, 4.6 %, and 6.7 % buoyancy cases, respectively. While the differences between these locations are very small, they follow the trend of the source velocity.
3.2 Plume Spreading
To calculate the spread angles of the plumes, we first determine the lines of best fit for the plume outlines. The angle between this line and the plume centerline can thus be identified as the spread angle of the plume. Considering that a linear fit is not necessarily the best fit for all of the plume outlines, only those images with root-mean-square error (RMSE) values between the line of best fit and plume edge lower than the average RMSE values were considered for analysis in a trial. Figure 9 shows a sample image for determining spread angle. The half angles of the plume for the different cases were found to range from 7 to \(8^\circ\) in all the three cases, when averaged across all images within the trial (i.e., 45 to 80 images). These values are lower than the reported range of \(10.2^\circ \pm 1.72^\circ\) by Kitamura and Sumita (2011). The total spread angles were found to be \(16.25^\circ , 14.94^\circ\), and \(14.28^\circ\) for the 2.5 %, 4.6 %, and 6.7 % relative buoyancy cases, respectively. We obtained similar results on measuring the spread angles formed by performing linear fits of time-averaged images of the different trials, in which we found spread angles of \(16.04^\circ\), \(15.00^\circ\), and \(14.42^\circ\) for the three cases, respectively. The averaged values of the flow parameters are tabulated in Table 2. Intuitively, we expected the angles to decrease with increasing front velocities. The front velocities of cases 2 and 3 are notably higher than case 1, leading to the difference in spread angles being relatively significant. However, the spread angle for case 3 is smaller than case 2, even with a lower front velocity than case 2. We presume this is the result of the relatively high Ri or influence of buoyancy in the case 3 trials, which led to the spread angle being lower than case 2. Considering that the entrainment coefficient is an essential aspect of plumes (McConnochie et al. 2021), empirical relations relating spread angle to the plume entrainment coefficient as provided in Lee and Chu (2003) can be used to determine the entrainment coefficients of the plume in the different cases.
3.3 Statistical Characterization of Eddy Lengths
To understand the structural evolution of the plume, the distribution of the structure sizes were analyzed using statistical methods. The three questions guiding the analysis for the evolution of the structure size distribution are as follows:
-
1.
Does the distribution of the structure lengths change over time?
-
2.
What distribution best fits the size distribution of the structures that occur at the interface between the plume and the ambient fluid?
-
3.
Does the distribution change for plumes with different initial buoyancy conditions or Reynolds number?
To determine time-dependence of the structure lengths, we plotted box plots for the structure sizes as the plume evolves in time (Fig. 10). However, no distinct trend for the change in structure sizes over time was found. We observed this behavior in all trials for all buoyancy conditions, which led to the conclusion that the structure size distributions are not time-dependent, even in the early stages of plume development.
Box plot of structure sizes for images of a sample trial (4.6 % relative buoyancy, trial 4, right side). The central mark of each box represents the median structure size, while the lower and upper edges denote the 25th and 75th percentiles, respectively. The whiskers extend to the furthest data points within the non-outlier range, with outliers represented individually by the ’+’ symbol
As mentioned in Sect. 2.3, the eddy lengths are characterized as the distance between two consecutive peaks identified on the plume outline. For all trials across the different cases, the distribution of the structure sizes was found to be positively skewed. This can be seen in Fig. 11, which shows the distribution of all the structure sizes for the trials with 4.6\(\%\) relative buoyancy. The distribution is found to be best represented as either log-normal or inverse Gaussian, which show a negligible difference in the fit accuracy. We consider the log-normal distribution for this study as the parameters are relatively simpler to evaluate than the inverse Gaussian.
Fig. 12 shows the log-normal distributions of the structure sizes compiled from all buoyancy levels. It can be seen that the maximum number of structures in all cases have lengths between 1 and 2 cm. However, there is a variation in the intensity and width of the distributions. The structure size distribution is widest for trials with a buoyancy difference of 2.5%, and subsequently narrows for the 6.7 % and 4.6 % trials. Thus, the width of the distribution is inversely related to the front velocities, implying that the range of structure sizes in a buoyant plume decreases with increasing front and thereby source velocities.
3.4 Spectra of Plume Edge Signals
Given the range of structure lengths presented in the distributions above, we were interested in further exploring the shape of the edge signal and corresponding distributions of features comprising the plume outline. Spectra of the edge signals (e.g., Fig. 5d) were computed using the fast Fourier transform. For a trial, the spectra were computed for images where the plume reached the top of the measurement region in order to maintain signals of approximately equal size; spectra were then ensemble averaged within each trial (Fig. 13). We then determined the slope (\(M_{y'y'}\)) of the spectra by performing linear fits within a select region of wavenumber (κ) for each spectrum. The averaged slope across all the trials was found to be approximately \(-\)2.23, following a log-log scale (see Table 2). The classic -5/3 slope (Kolmogorov 1941) is also shown in Fig. 13 for comparison, to explore whether the edge signal behaves similarly to that of concentration data within a turbulent plume (e.g., Crimaldi and Koseff 2001). We are not aware of similar results being shown for a spectrum computed of a signal outlining the shape or physical geometry of a turbulent region of flow. We hypothesize that the deviation from the -5/3 slope may be due to the presence of bubbles observed inside the plumes (Fig. 14).
The turbulent kinetic energy of two-phase air-water flows is known to be influenced by the void fraction of the bubbles in the flow. Experimental studies conducted by Lance and Bataille (1991) show that the hydrodynamic interaction between the bubbles increases with void fraction and results in a significant transfer of energy to the fluid. This leads to an increase in the slope of the 1-dimensional spectra of velocity fluctuations from -5/3 to -8/3. A similar result was obtained from the experimental studies of Shawkat et al. (2006). Bryant et al. (2009) reported that the slope of the turbulent energy spectra is lower than -5/3 at the center of a bubble plume due to the production of turbulence by bubbles at smaller scales and reduction of energy at larger scales. The slope recovers to -5/3 toward the edge of the bubble plume. Considering these results and the observation that the bubbles were not significant in our experiments, we hypothesize that the slope of the best fit line obtained from our experiments would be closer to -5/3 without bubbles from air trapped within the pipes that feed the buoyant fluid. Indeed, from this finding, we find evidence of the turbulence cascade in the eddies and structures that comprise this dynamic flow.
4 Conclusions
We have developed a new quantitative imaging technique for studying turbulent plumes that have high visual contrast relative to their surroundings. By using continuous photographic imaging of fluorescent-dyed buoyant plumes, we explored relationships between plume outlet conditions and the optical features comprising the plume exterior. By discerning the outermost edge of the plume from 2D photographs, we investigated the distribution of feature sizes apparent along the plume outline. We found that the distribution of feature lengths tends to follow a log-normal distribution. Importantly, we also found that when taking the spectrum of the edge signal, we find a \(-\)2.23 relationship on a log-log scale. While this differs slightly from the -5/3 relationship observed for spectra of velocity and concentration signals in turbulent flows, it is similar to observations of -8/3 slopes observed for spectra of Lance and Bataille (1991) and Shawkat et al. (2006) measured in 2-phase flows with air bubbles, and therefore indicative of the distribution of physical length scales that comprise the exterior structure of turbulent plumes.
We evaluated front velocities for the different tests and used empirical relationships to estimate source velocities in order to determine outlet Reynolds numbers and Richardson numbers. The front velocity increased as the relative buoyancy between the plume and ambient fluid changed from 2.5 % to 4.6 %, but dropped slightly with a further density difference of 6.7 %. We attributed this peculiar behavior to the balance between the density gradient and the available potential energy for the plume mixture, which have opposing influences on the outlet velocity. We then estimated the spread angles and found that while the angles are primarily sensitive to the front velocities, they are also influenced by the buoyancy conditions of the plume. The spread angles decreased with increasing front velocities for first two buoyancy conditions but further decreased in the third condition with a slightly lower front velocity than the second condition. We attributed this behavior to the high relative buoyancy of the third case.
The distribution of feature lengths comprising the plume exterior was found to be functions of the front velocity. Both the spread angles and ranges of structure sizes decrease with increasing front velocities. However, the structure lengths were found to be independent of time of evolution of the plumes. This work provides a fundamental look at the dependence of the physical eddies and structures that comprise a plume on the driving conditions at the plume outlet, with intended applications where photographs and videos can be remotely acquired. In order to establish this method of edge detection and characterization of plume structures, there is limited variation of the Reynolds and Richardson numbers in the present study. Future experiments with a wider range of Re and Ri will be necessary to analyze variation of the turbulent structures with the presented algorithm. Further exploration into 3D edge structures coupled with increased variability in the plume or jet forcing would provide benefit for understanding the relevance of the turbulent energy cascade and Kolmogorov spectra in relation to eddies and coherent structures found in turbulent flows.
Data availability
The data that accompany this study are available from BJ upon reasonable request.
References
Adrian RJ (1991) Particle-Imaging Techniques for Experimental Fluid Mechanics. Annu Rev Fluid Mech 23:261–304
Andrews BJ, Gardner JE (2009) Turbulent dynamics of the 18 May 1980 Mount St. Helens eruption column. Geol 37(10):895–898. https://doi.org/10.1130/G30168A.1. (ISSN: 1943–2682:0091–7613)
Batchelor GK (1954) Heat convection and buoyancy effects in fluids. Quarterly Journal of the Royal Meteorological Society 80(345):339–358. https://doi.org/10.1002/qj.49708034504 (ISSN: 00359009, 1477870X)
Bhamidipati N, Woods AW (2017) On the dynamics of starting plumes. J Fluid Mech 833:R21469–7645. https://doi.org/10.1017/jfm.2017.762 (ISSN: 0022-1120)
Bombrun M, Jessop D, Harris A, Barra V (2018) An algorithm for the detection and characterisation of volcanic plumes using thermal camera imagery. J Volcanol Geoth Res 352:26–37. https://doi.org/10.1016/j.jvolgeores.2018.01.006 (ISSN: 03770273)
Bryant DB, Seol D-G, Socolofsky SA (2009) Quantification of turbulence properties in bubble plumes using vortex identification methods. Physics of Fluids 21:
Burridge HC, Partridge JL, Linden PF (2016) The Fluxes and Behaviour of Plumes Inferred from Measurements of Coherent Structures within Images of the Bulk Flow. Atmosphere-Ocean 54(4):403–417. https://doi.org/10.1080/07055900.2016.1175337 (ISSN: 0705-5900, 1480-9214)
Chojnicki KN, Clarke AB, Adrian RJ, Phillips JC (2014) The flow structure of jets from transient sources and implications for modeling short-duration explosive volcanic eruptions. Geochemistry, Geophysics, Geosystems 15(12):4831–4845. https://doi.org/10.1002/2014GC005471
Clarke AB, Phillips JC, Chojnicki KN (2009) An investigation of vulcanian eruption dynamics using laboratory analogue experiments and scaling analysis. In: Thordarson T, Self S, Larsen G, Scott K, Rowland A, Hoskuldsson A (eds.) Studies in Volcanology: The Legacy of George Walker. The Geological Society of London on behalf of The International Association of Volcanology and Chemistry of the Earth’s Interior, first edition., pp 155–166. https://doi.org/10.1144/IAVCEl002.8 (ISBN: 978-1-86239-624-1, 978-1-86239-280-9)
Corrsin S, Kistler AL (1955) Free-Stream Boundaries of Turbulent Flows. Technical Report 1244, Johns Hopkins University
Crimaldi JP, Koseff JR (2001) High-resolution measurements of the spatial and temporal scalar structure of a turbulent plume. Experiments in Fluids 31(1):90–102. https://doi.org/10.1007/s003480000263 (ISSN: 0723-4864, 1432-1114)
Funatani S, Fujisawa N, Ikeda H (2004) Simultaneous measurement of temperature and velocity using two-colour LIF combined with PIV with a colour CCD camera and its application to the turbulent buoyant plume. Measurement Science and Technology 15(5):983–990. https://doi.org/10.1088/0957-0233/15/5/030 (ISSN: 0957-0233, 1361-6501)
Hoult DP, Fay JA, Forney LJ (1969) A Theory of Plume Rise Compared with Field Observations. Journal of the Air Pollution Control Association 19(8):585–590. https://doi.org/10.1080/00022470.1969.10466526 (ISSN: 0002-2470)
Rouse H, Yih CS, Humphreys HW (1952) Gravitational Convection from a Boundary Source. Tellus 4(3):201–210. https://doi.org/10.1111/j.2153-3490.1952.tb01005.x
Ibarra E, Shaffer F, Savas O (2020) On the near-field interfaces of homogeneous and immiscible round turbulent jets. Journal of Fluid Mechanics 889(A4):96
Kaminski E, Tait S, Carazzo G (2005) Turbulent entrainment in jets with arbitrary buoyancy. J Fluid Mech 526:361–376. https://doi.org/10.1017/S0022112004003209 (ISSN: 0022-1120)
Kitamura S, Sumita I (2011) Experiments on a turbulent plume: Shape analyses. J Geophys Res 116(B3):B03208. https://doi.org/10.1029/2010JB007633 (ISSN: 0148-0227)
Kolmogorov AN (1941) The local structure of turbulence in incompressible viscous fluid for very large Reynolds numbers. Cr. Acad. Sci. URSS 30:301–305
Krug D, Holzner M, Lüthi B, Wolf M, Tsinober A, Kinzelbach W (2014) A combined scanning PTV/LIF technique to simultaneously measure the full velocity gradient tensor and the 3D density field. Measurement Science and Technology 25(6):065301. https://doi.org/10.1088/0957-0233/25/6/065301 (ISSN: 0957-0233, 1361-6501)
Kuethe AM (1935) Investigations of the Turbulent Mixing Regions Formed by Jets. J Appl Mech 2(3):A87–A95. https://doi.org/10.1115/1.4008625
Lance M, Bataille J (1991) Turbulence in the liquid phase of a uniform bubble air-water flow. Journal of Fluid Mechanics 222:95–118
Lee JHW, Chu VH Turbulent Jets and Plumes: Turbulent buoyant plumes. Springer, (2003)
Matulka A, López P, Redondo JM, Tarquis A (2014) On the entrainment coefficient in a forced plume: Quantitative effects of source parameters. Nonlinear Processes in Geophysics 21(1):269–278. https://doi.org/10.5194/npg-21-269-2014 (ISSN: 1607-7946)
McConnochie CD, Cenedese C (2023) Increased melting of marine-terminating glaciers by sediment-laden plumes. Geophysical Research Letters 50:
McConnochie CD, Cenedese C, McElwaine JN (2021) Entrainment into particle-laden turbulent plumes. Phys. Rev. Fluids 6:123502. https://doi.org/10.1103/PhysRevFluids.6.123502
ODonnell SB, Johnson BA, Breard ECP, Buttles JL, Gardner JE, Mohrig D, Dufek J (2024) Density stratification and buoyancy evolution in pyroclastic density currents. Journal of Geophysical Research: Solid Earth 129(6)
Philip J, Marusic I (2012) Large-scale eddies and their role in entrainment in turbulent jets and wakes. Phys Fluids 24(5):055108. https://doi.org/10.1063/1.4719156
Rogers MC, Morris SW (2009) Natural versus forced convection in laminar starting plumes. Physics of Fluids 21:
Saeed Z, Weidner E, Johnson BA, Mandel TL (2022) Buoyancy-modified entrainment in plumes: Theoretical predictions. Phys Fluids 34(1):015112. https://doi.org/10.1063/5.0065265
Scase MM (2009) Evolution of volcanic eruption columns. Journal of Geophysical Research: Earth Surface 114(F4)
Shannon LK, Viggiano B, Cal RB, Mastin LG, Van Eaton AR, Solovitz SA (2020) Flow development and entrainment in turbulent particle-laden jets. Journal of Geophysical Research: Atmospheres 128:93
Shawkat ME, Ching CY, Shoukri M (2006) On the liquid turbulence energy spectra in two-phase bubbly flow in a large diameter vertical pipe. International Journal of Multiphase Flow 33:300–316
Sparks RSJ (1986) The dimensions and dynamics of volcanic eruption columns. Bulletin of Volcanology 48(1):3–15. https://doi.org/10.1007/BF01073509 (ISSN: 0258-8900, 1432-0819)
Sparks RSJ, Wilson L, (1982) Explosive volcanic eruptions - V. Observations of plume dynamics during the, (1979) Soufriere eruption. St Vincent. Geophysical Journal International 69(2):551–570. https://doi.org/10.1111/j.1365-246X.1982.tb04965.x
Turner JS (1962) The ‘starting plume’ in neutral surroundings. Journal of Fluid Mechanics 13(3):356–368. https://doi.org/10.1017/S0022112062000762 (ISSN: 0022-1120, 1469-7645)
Turner JS (1969) Buoyant Plumes and Thermals. Annual Review of Fluid Mechanics 1(1):29–44. https://doi.org/10.1146/annurev.fl.01.010169.000333 (ISSN: 0066-4189, 1545-4479)
Turner JS (1979) Buoyancy Effects in Fluids. Cambridge University Press,
Turner JS (1986) Turbulent entrainment: The development of the entrainment assumption, and its application to geophysical flows. Journal of Fluid Mechanics 173:431–471. https://doi.org/10.1017/S0022112086001222 (ISSN: 0022-1120, 1469-7645)
Valade SA, Harris AJL, Cerminara M (2014) Plume Ascent Tracker: Interactive Matlab software for analysis of ascending plumes in image data. Comput Geosci 66:132–144
van Reeuwijk M, Craske J (2015) Energy-consistent entrainment relations for jets and plumes. J Fluid Mech 782:333–355. https://doi.org/10.1017/jfm.2015.534 (ISSN: 0022-1120, 1469-7645)
Westerweel J, Fukushima C, Pedersen JM, Hunt JCR (2005) Mechanics of the Turbulent-Nonturbulent Interface of a Jet. Physical Review Letters 95(17):174501. https://doi.org/10.1103/PhysRevLett.95.174501 (ISSN: 0031-9007, 1079-7114)
Rodi W (1982) Turbulent Buoyant Jets and Plumes. Pergamon Press
Acknowledgements
The authors wish to thank financial support provided by the Texas Exes Scholarship and the University of Texas at Austin Fariborz Maseeh Department of Civil, Architectural & Environmental Engineering. We wish to thank Dr. Amanda Clarke for motivating this research and entertaining discussions about the research findings. We thank David Braley for work in constructing and maintaining the experimental facility. Finally, we graciously acknowledge the editor and anonymous reviewer for their thoughtful contributions.
Funding
The authors graciously acknowledge funding provided by the Division of Chemical, Bioengineering, Environmental, and Transport Systems of the US National Science Foundation (Award no. 2231780).
Author information
Authors and Affiliations
Contributions
LF and BK are equally contributing co-first authors of this manuscript. Experimental campaigns were designed by BJ and LF. Preliminary tests were conducted by LF and JH. Data presented within the manuscript was collected by EL, RW, and BJ. Algorithms for eddy length identification and statistical analysis were developed by LF and modified by BK. Data analysis was performed by all authors, with primary analysis performed by BK. The manuscript was written by LF, BK, and BJ, with support of all coauthors.
Corresponding author
Ethics declarations
Ethical approval
Not applicable.
Conflict of interest
The authors declare that they have no financial or personal conflict of interest.
Additional information
Publisher's Note
Springer Nature remains neutral with regard to jurisdictional claims in published maps and institutional affiliations.
Rights and permissions
Springer Nature or its licensor (e.g. a society or other partner) holds exclusive rights to this article under a publishing agreement with the author(s) or other rightsholder(s); author self-archiving of the accepted manuscript version of this article is solely governed by the terms of such publishing agreement and applicable law.
About this article
Cite this article
Kalita, B., Florez, L.P., Landau, E. et al. Characterizing visual structures in a buoyant plume. Exp Fluids 65, 136 (2024). https://doi.org/10.1007/s00348-024-03862-5
Received:
Revised:
Accepted:
Published:
DOI: https://doi.org/10.1007/s00348-024-03862-5